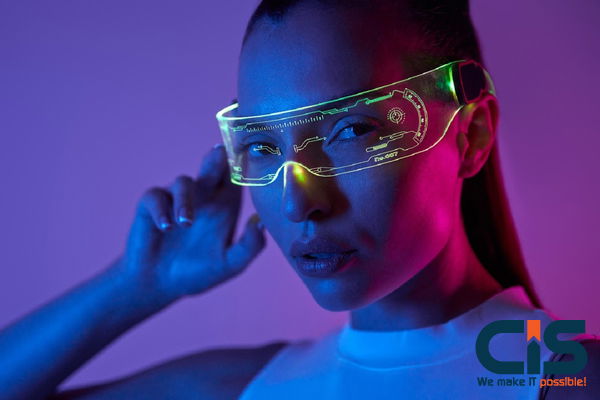
In an era where over 79% of companies acknowledge that supply chain management (SCM) is integral to their competitive advantage, the need for efficiency and responsiveness has never been more critical.
As the landscape of global trade grows increasingly complex, organizations are turning to cutting-edge technologies to streamline operations. One such innovation making waves is machine learning (ML). According to Statista, in 2025, the machine learning market is expected to grow to a size of US$113.10 billion.
With advanced algorithms and data analytics, machine learning applications are revolutionizing how organizations approach SCM. Machine learning engineers and developers are at the forefront of this transformation, creating intelligent systems that anticipate demand fluctuations, automate procurement, and enhance logistics management.
This blog aims to explore the pivotal role of machine learning in reshaping supply chain management. We'll delve into ten specific ways that ML is enhancing efficiency, responsiveness, and decision-making within the supply chain.
From predictive analytics to supplier risk management, these advancements not only promise to optimize processes but also safeguard companies against future disruptions. Join us as we uncover how machine learning can empower your supply chain to thrive in a dynamic marketplace.
Read Also: Machine Learning: Boon or Bane? Cost, Gain, and Impact Revealed!
Enhanced Demand Forecasting
Expert Insights
Machine learning engineers leverage sophisticated algorithms that analyze historical sales data, market trends, and external variables to forecast customer demand with remarkable accuracy. By utilizing machine learning applications, companies can sift through vast amounts of data, identifying patterns that human analysts might miss. This allows for predictive analytics that not only anticipates customer needs but does so with a level of precision that can significantly impact inventory management.
Experience in Action
Consider the case of a leading retail giant that integrated machine learning-powered forecasting tools into its supply chain operations. By adopting these advanced analytics, the company was able to reduce stockouts by an impressive 30%. This transformation wasn't just about numbers; it represented a shift in how they approached inventory, allowing for quicker restocking and enhanced product availability.
Why It Matters
Improved demand forecasting directly benefits both businesses and customers. For companies, accurate demand predictions translate into optimized inventory planning, reducing excess stock and minimizing waste.
For customers, this means enhanced satisfaction as products are readily available when needed. As machine learning developers continue to refine these applications, organizations can expect even greater reliability in their forecasting capabilities, paving the way for a more responsive supply chain.
Inventory Optimization
Expert Insights
Machine learning applications are revolutionizing how businesses approach inventory management. According to Statista, by 2025, the global supply chain management software industry is expected to generate US$20.97 billion in sales. By utilizing predictive analytics and real-time tracking, companies can effectively minimize overstock and understock scenarios.
Machine learning engineers create algorithms that analyze sales patterns, seasonal trends, and other variables to recommend optimal inventory levels. This data-driven approach ensures that organizations maintain the right amount of stock at all times, aligning supply with actual market demand.
Experience in Action
A prominent manufacturing firm implemented machine learning-based optimization tools to revamp its inventory strategy. As a result, they achieved a remarkable 20% reduction in inventory costs.
The machine learning developers behind this solution integrated predictive maintenance schedules and inventory tracking systems that adjusted automatically to sales fluctuations. This shift not only improved operational efficiency but also freed up capital that could be redirected to other important areas of the business.
Why It Matters
Balancing supply and demand is critical for any organization looking to thrive in a competitive landscape. By leveraging machine learning applications for inventory optimization, businesses can significantly reduce waste, lower costs, and ultimately enhance profitability.
The reliability of these ML-driven solutions helps companies respond quickly to changing market conditions, ensuring that they are not overstocked with slow-moving items or understocked on fast-selling products.
Prioritizing inventory optimization through machine learning empowers teams to make informed decisions that drive success. As machine learning engineers and developers continue to innovate and refine these applications, the benefits will only become more pronounced. Embracing this technology is no longer a luxury, it's a strategic imperative that can transform supply chain operations.
Supplier Selection and Evaluation
Expert Insights
Machine learning applications are transforming how companies evaluate and select their suppliers. By utilizing robust data analysis, machine learning engineers can assess supplier performance across various metrics, including delivery times, product quality, and compliance with contractual obligations. This data-driven approach allows organizations to build stronger partnerships by identifying reliable suppliers while minimizing risks.
Experience in Action
A leading automotive company leveraged machine learning to refine its supplier scoring system. By analyzing historical performance data, the machine learning developers were able to pinpoint inefficiencies and opportunities for improvement. The implementation of these advanced analyses resulted in a notable 15% reduction in late deliveries, significantly enhancing the company's supply chain reliability.
Why It Matters
A seamless and effective supply chain depends on having solid relationships with suppliers. By employing machine learning applications for supplier selection and evaluation, businesses can lower risks associated with supplier failures and enhance overall supply chain performance.
With the backing of skilled machine learning software engineers and developers, companies can make informed business decisions that lead to more reliable supplier partnerships, ultimately driving growth and customer satisfaction.
In today's competitive landscape, the use of machine learning in supplier evaluations is not just a technological advancement-it's a strategic necessity. Firms that embrace these solutions can ensure reliability and efficiency in their supply chains, embodying the values of simplicity and dependable performance that resonate with their brand ethos.
Logistics and Transportation Management
Expert Insights
Machine learning applications are revolutionizing logistics and transportation management. With the help of machine learning engineers, businesses can employ sophisticated algorithms that analyze a myriad of data points to optimize delivery routes and improve scheduling. This not only enhances fleet efficiency but also significantly cuts down on average time and fuel costs.
Experience in Action
One notable example comes from a leading logistics provider that harnessed the power of machine learning to streamline their operations. By integrating predictive analytics into their delivery systems, they managed to reduce transportation costs by an impressive 25%.
The machine learning developers on the project meticulously fine-tuned the algorithms, allowing for real-time adjustments based on traffic patterns and other variables. This initiative not only saved costs but also improved the overall speed of their services.
Why It Matters
In today's fast-paced market, quicker deliveries at lower costs can provide businesses with a substantial competitive edge. The application of machine learning in logistics empowers organizations to respond more effectively to customer demands, ultimately enhancing reliability and customer satisfaction.
By investing in machine learning applications, companies can ensure that their transportation strategies are both efficient and adaptable, aligning perfectly with core values of simplicity and teamwork.
Moreover, the collaborative efforts of skilled machine learning engineers and developers make it possible to create solutions that drive growth and operational excellence in supply chain management.
Quality Control and Maintenance
Expert Insights
Machine learning applications are redefining quality control in supply chain management. By leveraging predictive maintenance, organizations can anticipate equipment failures before they occur, leading to significant reductions in downtime.
For machine learning engineers and developers, the incorporation of real-time quality inspections into production processes is now more feasible and effective than ever. This proactive approach not only enhances operational efficiency but also ensures that products meet the highest standards right from the start.
Experience in Action
One compelling case study comes from a leading manufacturing firm that adopted machine learning-enabled systems for their quality control processes. By implementing predictive analytics and automated inspections, they were able to boost their product quality by an impressive 18%. This success story highlights how machine learning applications empower teams to make data-driven decisions, minimizing human errors and optimizing resource utilization.
Why It Matters
The significance of integrating machine learning into quality control cannot be overstated. Early detection of potential issues allows companies to maintain reliability in their products, bolstering customer trust and satisfaction. In today's competitive market, ensuring quality through advanced technology is crucial for maintaining a strong reputation and driving growth.
For those involved in machine learning development, the potential to improve quality while cutting costs is an exciting opportunity. With ML, organizations can streamline their quality assurance processes, fully embodying the principles of reliability and efficiency that are vital in supply chain management. As we continue to advance in this digital era, embracing machine learning applications will be essential for companies striving to achieve excellence in their operations.
Enhanced Decision Making and Analytics
Expert Insights
Machine learning (ML) is revolutionizing decision-making in supply chain management by processing vast datasets effectively. This technology empowers machine learning engineers and developers to create models that analyze complex patterns within the data. The outcome is actionable valuable insights that support strategic decisions. With machine learning applications, businesses can identify trends, forecast demand, and optimize inventory-all in real-time.
Experience in Action
One notable example comes from a fast-moving consumer goods (FMCG) company that integrated machine learning into their decision-making processes. By employing ML-integrated dashboards, they were able to accelerate their decision-making speed by an impressive 40%.
These dashboards provide stakeholders with visual data representations, allowing for quick interpretation and more informed choices. The combination of automation and intelligent analytics has made their operations more agile and responsive to market changes.
Why It Matters
In today's competitive landscape, data-driven decisions are essential for maintaining an edge. Companies that embrace machine learning applications can enhance their agility and precision, leading to better performance and reduced risks.
The ability to act quickly on accurate insights helps organizations adapt to dynamic market conditions, keeping them one step ahead. For machine learning developers, this ongoing evolution in analytics signifies a critical shift toward using technology to empower teams and drive results.
Incorporating machine learning into decision-making processes not only boosts efficiency but also supports a culture of reliability and teamwork. By harnessing the power of data, businesses can ensure that every decision is grounded in analysis rather than assumption. As machine learning continues to advance, its applications in enhancing decision-making will inevitably shape the future of supply chain management.
Risk Management and Mitigation
Risk management is a critical aspect of supply chain management, and machine learning applications are revolutionizing how companies identify and mitigate potential threats. Machine learning engineers and developers are harnessing advanced algorithms to simulate various scenarios, allowing organizations to foresee potential disruptions before they occur. This capability is not merely theoretical; it's proven effective in real-world applications.
Expert Insights
By modeling numerous "what-if" scenarios, machine learning frameworks can detect manifold risks such as supplier failures, demand spikes, or logistic delays. In practice, these ML models analyze vast datasets, recognizing patterns that might indicate an impending disruption. This proactive approach is transforming risk management from reactive to anticipatory.
Experience in Action
Take the case of a leading logistics company that integrated machine learning into its risk management strategies. By employing sophisticated ML applications, they were able to predict potential disruptions caused by both internal and external factors.
As a result, they successfully avoided losses estimated at $1 million. This success underscores the vital role that machine learning developers play in building systems that enhance operational resilience.
Why It Matters
In today's fast-paced market, resilient supply chains are essential for business continuity. By leveraging machine learning to innovate risk management strategies, companies can safeguard their operations against unexpected challenges. For businesses, this means not only protecting their bottom line but also enhancing their reliability in a constantly changing environment.
Investing in machine learning applications for risk management ensures that organizations are not just surviving potential disruptions but are equipped to thrive amidst them, reinforcing their competitive edge. The future of supply chain management lies in the hands of machine learning engineers who are dedicated to crafting solutions that prioritize security and efficiency.
Automation of Routine Tasks
Expert Insights
The integration of machine learning applications in supply chain management is significantly enhancing efficiency through the automation of repetitive tasks. By enabling machine learning engineers and developers to design systems that handle routine functions-such as order processing and inventory updates-organizations can streamline operations and reduce human error.
Experience in Action
A prominent distribution center implemented ML-driven automation, resulting in a remarkable 50% increase in processing speed. This advancement not only expedited order fulfillment but also improved overall operational accuracy. Such use of machine learning applications demonstrates how tailored solutions by skilled machine learning developers can lead to substantial productivity gains in supply chains.
Why It Matters
By automating mundane tasks, companies empower their employees to concentrate on more strategic initiatives that drive business growth. This shift enables teams to innovate and improve customer service rather than getting bogged down with legacy processes.
Ultimately, the role of machine learning engineers in developing these solutions creates a more agile workforce, facilitating a culture of reliability and efficiency. In today's fast-evolving market, embracing automation with machine learning is not just a trend; it's a vital step toward sustaining competitive advantage.
Customer Experience Enhancement
Expert Insights
Applications of machine learning are transforming how companies communicate with their clientele. By leveraging sophisticated algorithms, machine learning engineers and developers can create systems that personalize customer experiences based on data analysis.
This targeted approach leads to quicker response times and improved customer satisfaction. From chatbots that understand customer preferences to intelligent recommendation systems, the impact of machine learning on customer experience is profound.
Experience in Action
Consider an e-commerce brand that implements machine learning-driven personalization strategies. This company utilized data analytics to understand individual shopping behaviors and preferences, resulting in tailored recommendations for each customer. They consequently saw a startling 25% rise in client retention. This demonstrates the significant ROI achievable through effective machine learning applications in the supply chain.
Why It Matters
Enhancing customer experience is not just about making a sale; it's about building relationships. Satisfied consumers are more inclined to stick with your brand and promote it, which leads to long-term success.
As machine learning engineers and developers continue to innovate, businesses can expect increasingly effective tools that prioritize customer needs and streamline interactions. Ultimately, organizations that embrace these technologies will stand out in a competitive marketplace, ensuring they not only meet but exceed customer expectations.
Read More: Revolutionize Software: How Much Can Machine Learning Boost Your Gains?
Sustainability and Ethical Supply Chains
Expert Insights
Machine learning (ML) is playing a pivotal role in creating sustainable and ethical supply chains. By optimizing resource usage and minimizing waste, machine learning applications allow businesses to operate more efficiently.
For instance, a machine learning engineer can develop algorithms that predict demand, ensuring that production levels are closely aligned with actual market needs. This precision reduces overproduction and waste, thus promoting sustainability in the supply chain.
Experience in Action
Consider a leading retail chain that leveraged machine learning for route optimization. By employing advanced ML algorithms, they were able to analyze data related to delivery routes, traffic patterns, and customer locations.
As a result, they achieved a 10% reduction in carbon emissions. This is just one example of how machine learning developers can implement innovative solutions that not only enhance operational efficiency but also contribute positively to the environment.
Why It Matters
Implementing sustainable practices through machine learning is not merely about compliance with global regulations; it significantly improves a company's brand image. Customers today are increasingly aware of corporate social responsibility, and a commitment to sustainability can differentiate a business in a crowded market.
By integrating machine learning applications into supply chain processes, companies not only fulfill ethical obligations but also foster a culture of reliability and simplicity-qualities that resonate with consumers.
The transformation towards sustainability in supply chain management is driven by the profound capabilities of machine learning. As businesses continue to embrace these technologies, the partnership between machine learning engineers and developers will be crucial in pioneering eco-friendly and ethical practices. This alignment not only secures operational advantages but also creates a community geared toward sustainable growth and innovation.
CTA - Discuss Ethical Supply Chain Strategies Now
Conclusion
In conclusion, machine learning is undeniably transforming supply chain management in profound ways. From improving forecasting accuracy to enhancing resource allocation, the impacts of machine learning applications are evident across various sectors. Businesses that embrace these innovations, led by skilled machine learning engineers and developers, will find themselves better equipped to navigate the complexities of supply chain logistics, ultimately promoting growth and operational efficiency.
We encourage businesses looking to stay competitive and sustainable to explore the transformative potential of machine learning. Engaging with talented machine learning developers can enhance your organization's capabilities and streamline operations. If you are ready to take the next step and integrate cutting-edge machine learning applications into your supply chain processes, connect with the experts at CISIN.
Our team of dedicated machine learning engineers is here to collaborate on your projects, unlocking new possibilities and driving success in the digital age. For those seeking to leverage supply chain industry expertise, consider our Artificial Intelligence Development Services to revolutionize your business. Contact us right now to take advantage of the chance to improve your operations.