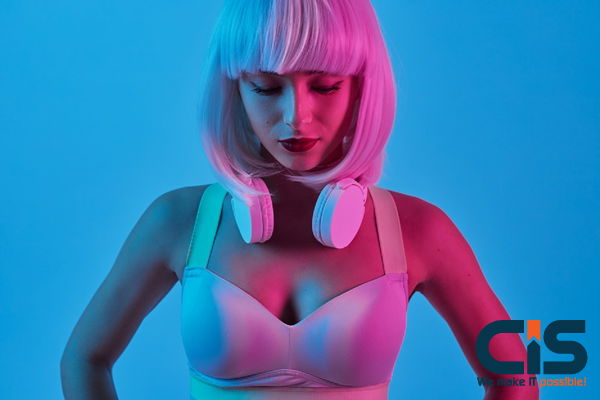
Data management and architecture continue to develop at an astonishingly fast rate, reaching new heights of sophistication never seen before. Every day 2.5 quintillion bytes of new data is generated globally - 90% of this was produced within the past few years alone. Organizations are becoming more aware of what information they gather, organize, and handle as data becomes the backbone for machine learning algorithms and provides insight across industries. Reading this article will enable you to gain greater knowledge of both the vast field of big data as well as its challenges, should you believe they're minor or nonexistent.
Big Data
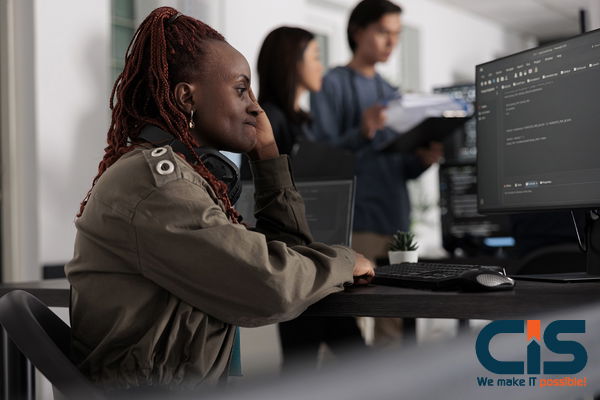
What is Big Data? To understand the challenges presented by big data, we must comprehend its definition. When hearing "Big Data," many may wonder how it differs from more conventional "data." Traditionally speaking, "data" refers to any unprocessed characters or symbols which can be recorded onto media or transmitted electronically using computer signals; raw data however cannot be utilized until processed somehow.
"Big Data" refers to an increasingly unmanageable volume of information generated from an interconnected world, making its management an important challenge that companies need to face quickly if they want to remain not only competitive but viable options in their sector.
However, with big data comes big infrastructure. Scalability of essential facilities, technology and energy required for processing data processing is just as vital to its processing as is its content - without properly managed and scalable infrastructure there would be no such challenge to contend with. Before we delve further into its difficulties let's review five vital aspects of Big Data that make up its foundation - or "V's".
Big Data: The Five 'V's
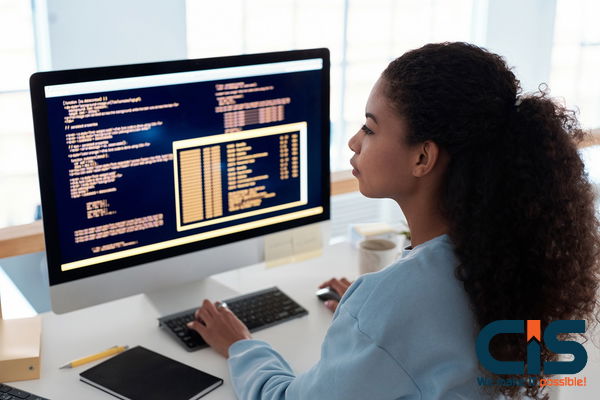
Big Data (short for "big information") refers to any collection of information too large and complex for traditional databases to store effectively, typically consisting of five components referred to collectively as Big Data:
- Volume: It is defined as the volume of data produced over a given period.
- Velocity: This measures how quickly data is produced, collected and analyzed.
- Variety: Variety refers to different forms of structured, semi-structured and unstructured data sets.
- Value: Transforming data into useful insights
- Veracity: Reliability in terms of quality and accuracy
What Does Facebook Do With Their Big Amount Of Data?
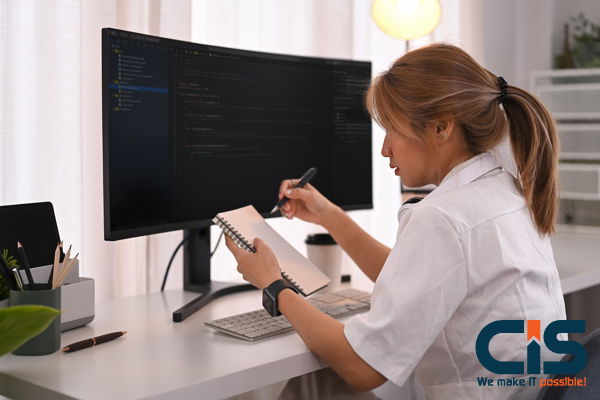
Facebook collects enormous quantities of user data - estimated in petabytes. The company uses this information in various forms - comments, likes, interests, friends and demographics among them - in various ways such as ads display.
- Create personalized news feeds and sponsor ads.
- Find recent pictures with high levels of engagement here for Photo Tag Suggestions.
- Crisis or disaster situations require regular safety check-ins with authorities to assess potential vulnerabilities and risks.
Case Study On Big Data
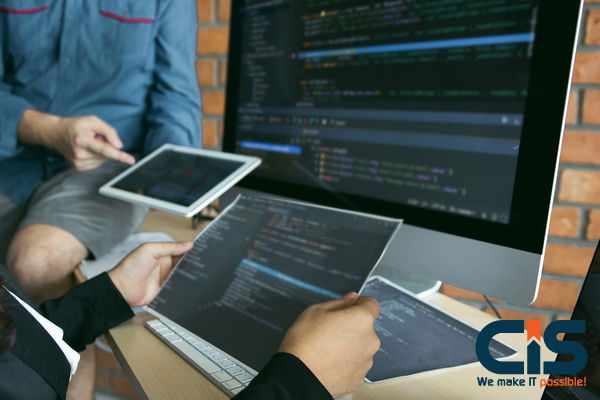
As Internet user numbers exploded over the past decade, Google faced increasing challenges storing so much user data on traditional servers. With thousands of search queries being raised each second and retrieval consuming hundreds of megabytes and billions of CPU cycles, retrieval needed an extensive, distributed and highly fault-tolerant file system in which to store queries; in response, they developed their Google File System (GFS).
GFS architecture comprises one master machine and multiple chunk servers or slave machines, such as chunk servers. The master contains metadata while chunk servers/slave machines store data distributedly. When an API client wants to read some data, they contact their master who then provides metadata information which they then send read/write requests out to all their slave machines for response generation. Files are split up into small chunks that are distributed among various chunk servers or slave machines, featuring features like:
- Each piece in Hadoop version 2 and later contains 64 MB (128 MB from version 1) of data.
- By default, each piece will be replicated three times across various chunk servers.
- If a server crashes, its data file remains on other chunk servers.
Why Can Big Data Be Challenging?
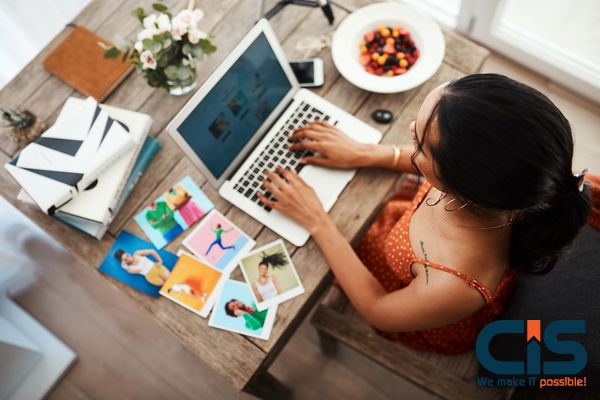
First and foremost, it helps to define "big data." No set amount differentiates "normal" from "big" data - big data is relative and constantly growing around the globe, meaning its definition has to remain fluid and relative. Big data presents unique challenges. Now, let's consider its specific issues and potential solutions.
Read More: Big Data: What Is It? Who Uses It? And How Much Does It Cost?
Challenges And Solutions Of Big Data
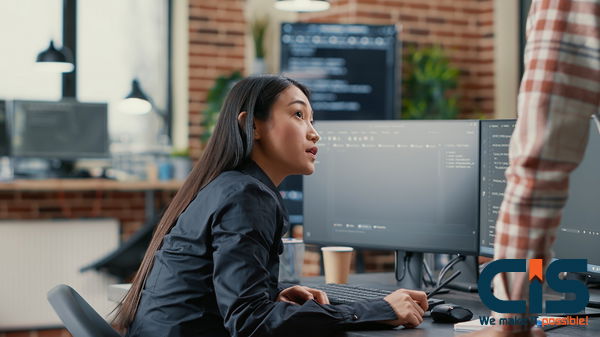
Here are a few common challenges of Big Data your organization could experience while attempting to implement a big data solution, along with potential solutions:
Organizing And Manipulating Large Volumes Of Information
Big data is, as it implies, an ever-increasing volume of information collected daily by businesses worldwide. Traditional data centers can eventually no longer accommodate this data influx, prompting business leaders to worry. According to 43 per cent of IT decision-makers working within technology industries, an overwhelming data influx could easily overwhelm infrastructure resources.
Companies are taking measures to meet this challenge by moving their IT infrastructure into the cloud, where storage solutions can scale dynamically as more storage needs arise, while big data software specializes in managing vast volumes of information and providing quick access and query capabilities.
Integrating Data From Multiple Sources
Businesses often face difficulty managing data due to its abundance and diversity. A business could possess analytics data from several websites, sharing data on social media channels such as Facebook or Instagram and user information from CRM software such as CRM Online CRM Plus - none of it conforms to standard structures; integration and reconciliation might be needed in order to extract insights that allow for reports.
Businesses face this challenge head-on by using software for data integration, ETL processing and business intelligence that combines disparate sources into one structure so accurate reports can be created. To address it successfully.
Ensuring Data Quality
Machine learning processes that depend on big data also need clean, accurate information in order to produce meaningful insights and predictions that match up with our expectations. Corrupt or incomplete information could produce unexpected outcomes; unfortunately, as sources, types, and quantities of data increase, it can become harder to tell whether all your sources contain sufficient quality for accurate insights.
There are solutions available to address this challenge. Data governance applications provide solutions by helping organize, secure and validate the data used in big data projects as well as cleaning corrupted or incomplete sets from them. Furthermore, quality software provides solutions by validating and cleaning up before it enters processing.
Protecting Data
Many companies handle sensitive information that must remain safe. Companies handling sensitive data often become the target of hackers. To safeguard it from potential attackers, businesses often hire cybersecurity specialists who stay abreast of best practices for protecting systems. Examples may include:
- Competitors could leverage company information in order to grab more market share of an industry.
- Financial data that could give hackers entry to accounts.
- The personal information of customers could be misused to commit identity theft.
No matter if it is outsourced to consultants or managed in-house, data must always be encrypted so it cannot be read without an encryption key. Identity and access authorization controls on resources, so only authorized users have access. Implement endpoint protection software to ward off malware attacks as well as real-time monitoring that detects threats immediately and stops threats promptly if detected.
Selecting Appropriate Big Data Tools
As soon as a business decides to start dealing with data, tools are readily available for them to do just that. At the same time, however, this abundance of options presents itself as a daunting challenge: big data software comes in various varieties with capabilities often overlapping between versions, so how do you ensure you choose appropriate tools?
At times, hiring an outside consultant to assess which tools would fit best with what your business plans to accomplish with big data may be the optimal approach. A knowledgeable data professional can take an in-depth look at both current and future requirements before selecting an enterprise data streaming or ETL solution to collect all available sources into aggregate form for further processing, configure cloud services appropriately, scale dynamically according to workload needs if necessary and scale dynamically once set up with tools tailored for their purposes - your system should run seamlessly without much maintenance required over time.
Optimizing Systems And Costs Efficiently
Start building your big data solution without first creating an effective plan, and you risk spending unnecessary funds to store and process information that either doesn't pertain to the business needs, is unnecessary, or redundantly duplicates existing systems. Big data doesn't equate with necessarily processing all available information at the same time - all it means is more data needs processing.
Your company must start its data project with goals in mind and a plan on how you'll utilize available data in order to reach those goals. Once implementing a solution is underway, team members need to determine exactly which types of data and schemas they need in order to avoid going down an unnecessary route with their project. In addition, policies must also be put in place regarding purging out unwanted old information that no longer serves its intended purpose from your system.
Lack Of Qualified Data Professionals
Many companies run into difficulties when working with big data because their current staff have never encountered it before - an experience gained only with time, training and experience. Working without sufficient staff training may result in dead ends, disrupted workflow, errors during processing or dead ends altogether.
There are various solutions to the data management challenge. One option is hiring a big data specialist who can oversee and train your data team until everyone is up-to-speed; you could hire this individual either full-time as an employee or hire them on as a consultant to train the team before leaving as necessary, depending on budgetary considerations.
Alternatively, if there's time to plan, provide training to existing team members so they will possess the necessary abilities once your big data project starts moving forward. Thirdly, select one of the self-service analytics or business intelligence solutions designed for professionals without an extensive data science background.
Institutional Resistance
Another form of resistance can pose a hurdle to data projects: resistance to change. Large organizations tend to be resistant to change. Leaders might not see value in big data analytics and machine learning projects or they may not want to invest their time or funds on something they deem unimportant or expensive enough for them.
As daunting a challenge as this may be, you don't need to struggle alone if it comes to big data usage in business. Start small with smaller projects with smaller teams until results demonstrate its value to other leaders as you gradually transition your company toward being data driven. Another approach would be placing expert big data experts into leadership positions so they can assist your transformation journey.
Top Tips For Handling Big Data And Understanding It Effectively
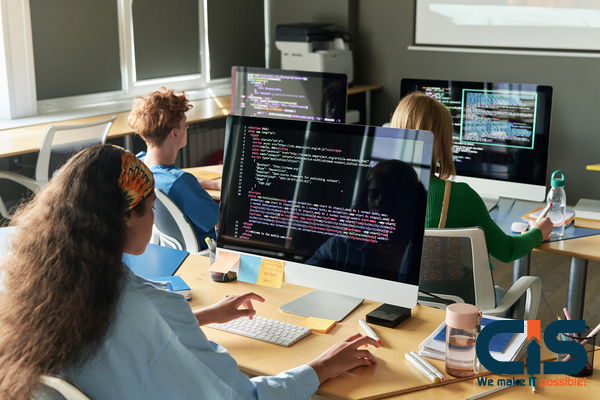
These tips cover three aspects: infrastructure requirements for managing data, analysis of said data and strategic motivations behind it all. Read out top tips for handling Big Data:
- Security: Be certain the infrastructure housing your data is secure to avoid analyzing lost or compromised information, which has long been one of the major headaches for regulated industries and one of the primary concerns for both customers and executives alike.
- Performance: Continuous assessment of environmental factors impacting data center environments is paramount to its performance. Heat, humidity, liquid leakage and thermal fluctuations that cause system failure and downtime must all be managed carefully to avoid system malfunction and downtime. Make use of historical data as planning material by closely watching these environmental variables that might impede optimal operation in real-time and automating preventative actions as required - monitor them all to plan for additional capacity requirements in your data center.
- Inventory/Asset Management: Data centers must remain flexible due to environmental conditions, workload fluctuations, maintenance needs, failures and depreciation - factors which must all be managed for audit requirements as well as potential regulatory implications. An automated asset tracking and management system would prove invaluable in keeping them running effectively and smoothly.
- Integrate: Avoid purchasing "best of breed" solutions at the expense of integrated ones, since data needs to communicate between multiple platforms in order to allow action to be taken either systematically or physically.
- BI & "Big Data" Analytics: One of the key aspects of managing big data is being able to capture it at just the right times and analyze it immediately - an expensive endeavor. As data warehousing applications have developed over time, integration and configuration processes have become easier but still require work. BI applications offer solutions designed for this process but will take an expert to execute successfully.
- Adapt: Continuous process adaptation is crucial in an agile business. Too often, processes become static, inhibiting response. Make sure the right personnel or systems are receiving and analyzing relevant data in order to create an actionable management information dashboard.
- Set Your Data Center Strategy: Large companies are increasingly planning 3-5-year strategies for their data centers to accommodate Big Data implications; otherwise, there could be serious cost and scaling implications that halt company expansion altogether.
- Manage Energy: Energy usage and environmental effects from data centers have become one of the primary drivers behind adaptation in the IT sector. Big data requires "Big Power", while governments and society demand strict governance over how these data centers utilize resources. Sustainability is paramount when harnessing big data for maximum profit potential.
- What You Cannot Find, You Cannot Manage: Unmanageable assets must be located and monitored closely so as to remain secure and readily available for management purposes. As regulations surrounding consumer and corporate data security increase, asset security violations become one of the top violations.
- Focus: Please don't get overwhelmed by Big Data hype; its immense growth brings with it many unique challenges; instead, remain focused on your organization and search out data that best supports its strategies and initiatives.
Want More Information About Our Services? Talk to Our Consultants!
Conclusion
Now that you understand the five 'Vs of Big Data and its case study, challenges, and potential solutions relating to it - as well as industry applications of this form of information collection - it is time for you to build your knowledge further and become industry-ready. Many organizations now utilize big data analytics services in their decision-making while creating insights for strategic business decisions and supporting insights with big data analyses.
Implementing big data technology into your business can be transformative and make you more competitive by giving access to insights not available elsewhere in your industry. While implementation will undoubtedly face its share of obstacles and hurdles, knowing what they are will allow you to navigate them without hindering its digital transformation efforts.