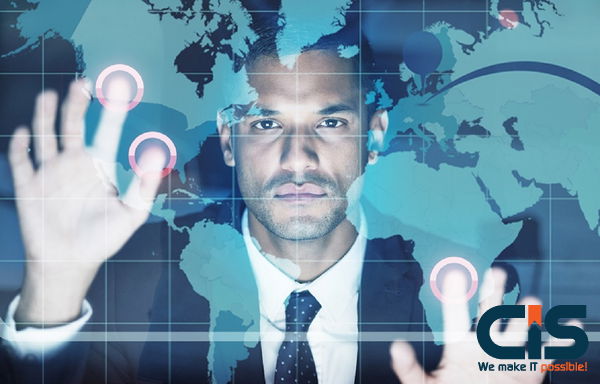
At an increasingly important stage in technology's history, being able to manage and analyze data effectively has become more of an art than a science. One exciting technological development over the past decade is big data: an important responsibility of technological specialists and data analysts that gather large amounts of information before compiling reports that are easy for people to interpret. Therefore, understanding big data has become essential.
Everyone who uses Big Data technology and techniques wants to understand it better. Yet, many use its name without actually understanding what it entails or its potential benefits. We will cover each Big Data perspective thoroughly in this blog - making this resource invaluable to those wanting to become experts with this subject.
Introduction To Big Data
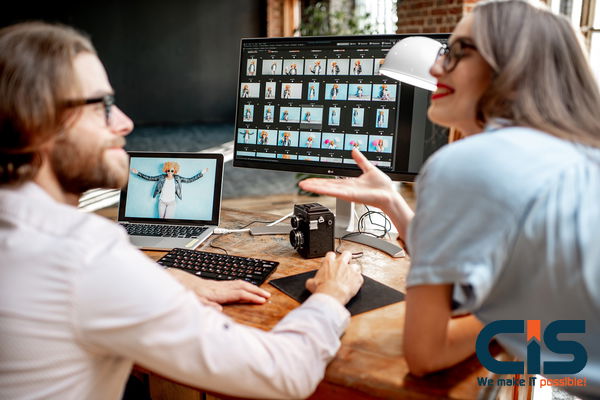
What is Big Data? Understanding Big Data requires understanding its definition. Understanding this could bring up questions on its difference from regular data we encounter every day; raw data simply does not have much use unless processed into usable form by an algorithm or computer software program. Big Data refers to an enormous collection of unstructured information generated by businesses, usually coming from websites, transactions and emails.
Big Data Classifications
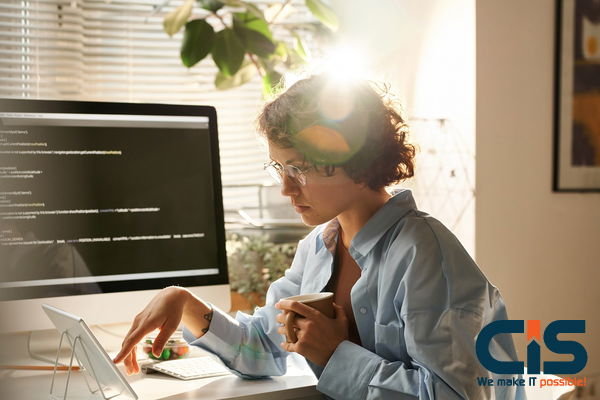
Big Data Can Be Organized, Unorganized or Semi-organized When categorizing big data based on how it is stored, and it may fall under three distinct forms:
- Structured Data: Information that can be easily accessed, processed, and stored is considered structured data. An example would be a table such as Student that stores fields related to each student in rows and columns of its rows/columns format.
- Unstructured Data: Any formless or formatless information can be considered unstructured data and can make processing and managing difficult. Examples may include data sources containing images, texts or videos.
- Semi-Structured Data: Semi-structured data includes both structured and unstructured elements. It typically has a formal form but doesn't correspond with tables; examples would include data stored within an XML file.
Characteristics Of Big Data
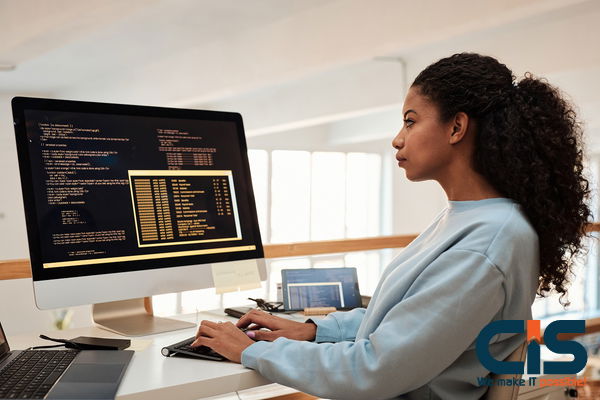
After getting acquainted with Big Data's core concepts, let's delve deeper into characteristics of Big Data. These characteristics include Volume, Velocity, Variety, Variability and Veracity as their hallmarks; let's study what these terms denote.
- Volume: It is defined by an enormous size of data which determines its volume. Volume can also indicate whether data is big or not.
- Velocity: Velocity measures the speed at which data generation occurs and processing. It demonstrates how rapidly information can be created and utilized in analysis.
- Variety: Variety refers to the heterogeneity of data being examined. Nowadays, this can include photos, videos, emails and audio.
- Variability: Data inconsistency may compromise our efforts in managing or processing it effectively, thus leading to variations.
- Veracity: Veracity refers to the trustworthiness and organization of data. Since large datasets exist today, it becomes essential that accurate quality control procedures be in place in order to protect both accuracy and quality in regard to these records.
Big Data Benefits In Business
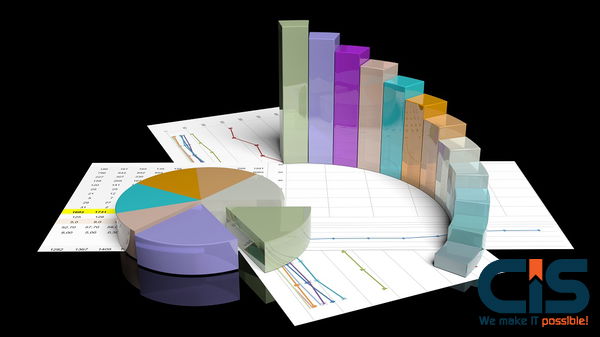
With digital trends emerging and consumer behavior evolving rapidly, businesses must adapt by training employees on Big Data tools for effective use of this vast amount of information to reach consumer insights and input for their own businesses. To keep pace with consumer insights and input for growth opportunities. But why have organizations turned toward Big Data? Here are primary benefits of Big Data for companies today.
- Time Saving: Big Data technologies like Hadoop offer fast techniques for quickly identifying sources and analyzing them quickly for quick decision-making. This ensures accurate results at any point in time.
- Cost Saving: Learning Big Data techniques will enable cost savings by efficiently storing huge volumes of information. As you gain experience using Big Data techniques, this will demonstrate your cost-efficient data management abilities.
- Customer Service: To improve feedback systems that effectively evaluate customer responses. It also enables people to manage both online and offline customer interactions appropriately.
- Consumer Insights: Big Data analytics tools have shed new light on consumer insights that could aid product creation and development for the market. This data could assist companies with creating innovative new offerings on the market.
- Relevant And Reliable: Web analytics using big data is a useful way to comprehend relevant data sets better, while customer monitoring using newer technologies has become even more reliable and trustworthy.
- Security: Big Data technologies offer secure options for performing data analysis with leading partners and advanced infrastructures.
- Operational Efficiency: Big Data technologies aid in the identification and filtering of usable information while offloading irrelevant data for easier management, leading to higher operational efficiencies overall.
- Real-Time Monitoring: Big Data technologies facilitate real-time system monitoring in real time to detect issues as soon as they arise and determine any causes of system failures, too.
- Risk Identification:Big data provides early and accurate detection of risks related to products and services. Risk portfolios can quickly be reviewed for any problems that might occur.
- Predictive Analysis: Predictive analysis allows an organization to scour social media and online spaces in search of consumer feedback and responses, helping you stay ahead of competitors.
Gain Expert Knowledge In Big Data With Significant Facts
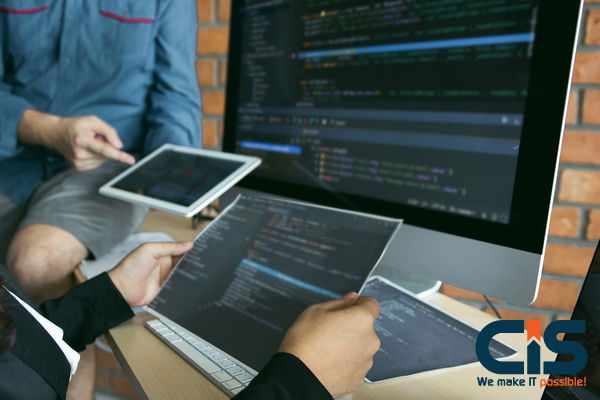
Big Data technologies come with various facts that will aid your understanding. These provide insights on what aspects your organization should keep in mind when creating and adopting Big Data strategies.
Big Data Is Everywhere
Big data has become ubiquitous in our highly digital world, thanks to the Internet of Things (IoT). Now, every item connected digitally generates new sources of data, which continue to stream into companies through these items - creating vast quantities of Big data every day, which affects every industry and organization imaginable - thus necessitating organizations to understand this phenomenon and use this resource appropriately to their benefit.
The Big Data Culture
IT giants must understand that adopting Big Data technologies represents a culture shift. Making an organization data-driven will require both strategic and operational changes; using data more effectively by employees requires cultural adaptation to take place as well. When we learn Big Data technologies, our minds need to adapt quickly in working with vast datasets.
People Are Key In Big Data Analytics
People are the heart of Big Data technologies in an organization. Data management strategies will only succeed if people in that organization become familiar with Big Data technologies and are ready to implement strategies based on them. Thus, employees need to acquire Big Data skills within an organization.
Big Data Engineers Are Needed Now
According to predictions, there is currently an acute shortage of Big data engineers and this trend will only worsen as companies rapidly adopt Big Data technologies and require well-trained talent for implementation. Large organizations typically rely on existing resources learning Big Data technologies while hiring experts for external training as needed.
Funding And Investment Considerations For Investment In The Field
There has been a drastic surge in funding available for Big data start-ups worldwide and funding available from venture capital firms and governments has only furthered this field's expansion. Thus, should you learn Big Data, there will be endless opportunities in this arena. However, using big data presents several unique challenges. When conducting statistical data analyses on big data sets, extreme caution must be exercised as figures may be misleading and misinterpretation or misanalysis may provide incorrect insight of information leading to wrong decisions being taken.
Big data solutions incur substantial expense and, to get maximum return on your investment, budget alignment must take place for optimal implementation of solutions. Furthermore, adaptability must also play a part in this endeavor: existing systems need to fit seamlessly alongside newer ones for efficient operation.
Read More: Big Data Challenges: Worth the Investment?
Trending Big Data Technologies
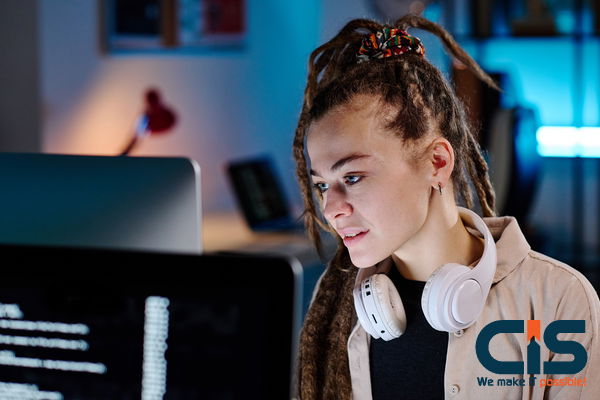
Companies are investing a great deal in big data technologies, and the market continues to experience strong growth. Big data and analytics are now mainstream in the IT world, and spending on banking, insurance, investment services and healthcare industries is projected to experience maximum expansion. Popular technologies adopted include data analytics used for risk management, fraud detection and customer service - with others trending such as:
- Hadoop Ecosystem: Apache Hadoop has quickly become one of the world's favorite Big Data technologies, used by companies and individuals alike worldwide. Products built around Hadoop continue to increase rapidly while vendors support this ecosystem of products and support services for Hadoop-related needs. If you want to start learning Big Data, starting out with Hadoop would be ideal as its foundation provides ample room for exploration.
- Apache Spark: Another component of the Hadoop ecosystem can be found worldwide and widely utilized. Spark serves as Hadoop's Big data processing engine - considerably quicker than Hadoop itself. Many vendors of Hadoop also allow products using Spark for processing data sets in Hadoop environments.
- NoSQL Databases: These special databases specialize in unstructured data usage and storage. MongoDB, Cassandra and other no-SQL solutions have proven fast performance over time.
- R Software: R is an open-source programming language designed for statistical analysis. Data scientists commonly turn to it due to its user-friendly IDE.
- Predictive Analytics: Predictive analytics refers to a technology that uses data mining and modeling alongside machine learning in order to predict future behaviors or events, typically within marketing, finance, credit scores or fraud detection industries.
- Prescriptive Analytics: Prescriptive analytics guides businesses in reaching desired results through prescriptive action planning.
- Data Lakes: Organizations have increasingly implemented data lakes to collect information from various sources and store it safely when using it for business. They allow organizations to keep all their records at one central place when searching for particular data sets or using it themselves.
- Artificial Intelligence: AI has rapidly advanced over the last several years and now encompasses data analytics, deep learning and machine learning as a part of AI's field of operation. Analytics tools play a central role in making artificial intelligence usable; their presence grows continuously, too.
- Big Data Governance Solutions: Data Governance has taken on even greater significance due to modern security concerns, comprising processes related to integrity, usability and availability.
- Big Data Security Solutions: As companies increasingly turn to big data analytics for business needs, safeguarding repositories against hackers and threats has become essential - leading them to seek data security solutions as an answer.
- Blockchain: The technology underlying Bitcoin digital currency, acts as a distributed database with unique properties - its data cannot be altered once stored within its structure.
Numerous Well-Known Big Data Tools Available
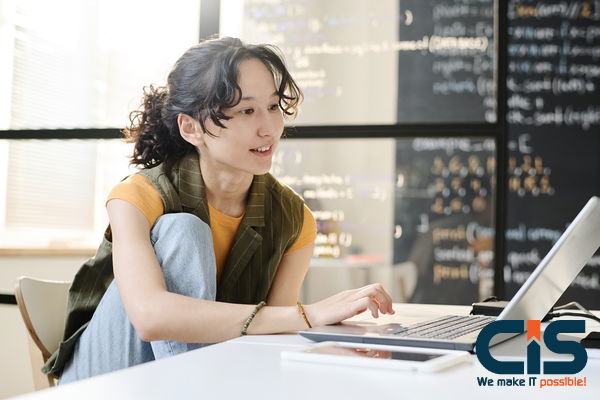
Today there are various tools in the market you should become acquainted with if you wish to understand Big Data. Organizations commonly utilize such tools for effective data analysis to increase cost efficiency and time savings - examples being:
Hadoop
Apache Hadoop, commonly used interchangeably with Big data, is an open software framework based on Java that is designed for distributed storage of large datasets on clusters. Scalability for your datasets and fault tolerance for your hardware are among its hallmark features; making Hadoop an invaluable solution when handling concurrent tasks that include both unstructured and structured information.
Apache Hive
It is another widely-used big data tool used for querying and managing large datasets, providing query capabilities with structured data models for modeling and interaction purposes. Supported query languages allow data modeling and interaction as well as tasks defined using Java and Python tasks defined for analysis of datasets using their interface for data modelling & interaction purposes. Designed mainly to query structured data only while simplifying complex maps reduces programming for its users.
Storm
Apache Storm is an open-source data processing application with real-time capabilities for streaming data processing in real-time streams, offering distributed fault tolerance with real-time computation capabilities and parallel processing across clusters of machines - one of the easiest big data tools to work with.
MongoDB
Written in C++, it is an invaluable tool for handling rapidly varying data, from mobile applications and content management systems, for instance. MongoDB features high availability as well as index support, which makes analysis of large datasets easy while developing applications.
HPCC Systems
It offers effective methods for data analysis. As an alternative to Hadoop, HPCC acts as a platform that facilitates querying, transforming and manipulating information while offering both scalability and high performance with its built-in distributed system.
Cassandra
Apache Cassandra is an efficient database for efficiently handling large datasets. Featuring a fault tolerant design with replicated data across multiple nodes, Cassandra boasts excellent scalability, performance, and availability characteristics that enable efficient management.
Want More Information About Our Services? Talk to Our Consultants!
Conclusion
Big Data technologies represent the future of the IT world and the tech market. No industry can flourish without making use of these powerful tools and technologies; additionally, talent demand for Big Data analysis solutions continues to soar with this surge. In particular, professionals could benefit greatly by learning Big Data technologies; consequently making big data part of how the world changes today. Cisin understands this reality and assists learners of Big Data through services.