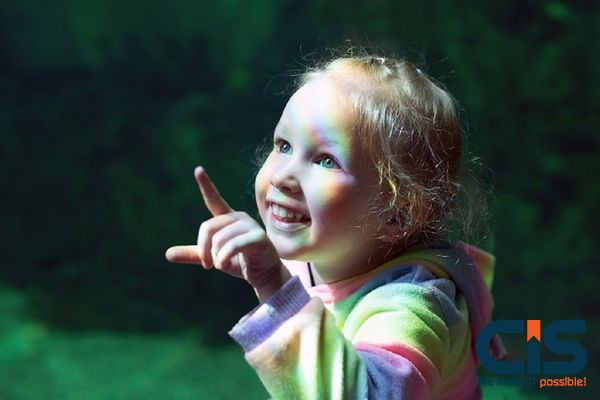
AI can be used in many ways. The AI automatically improves defect detection by using image processing to classify defects on various industrial products.
What is AI in Manufacturing?
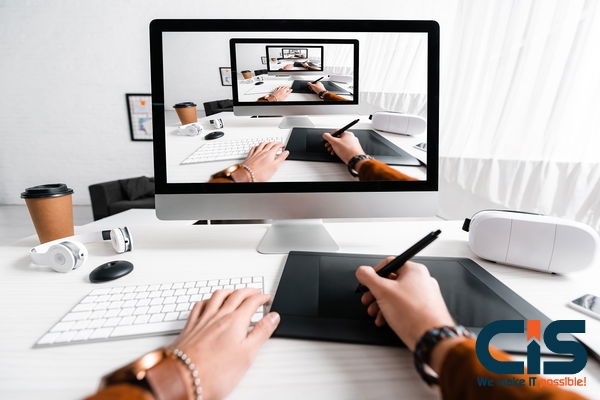
Artificial intelligence can be used in many ways to improve manufacturing. Artificial intelligence solutions such as machine learning and deep neural networks are being used by manufacturers to analyze data better and to make more informed decisions.
Artificial intelligence is frequently cited as a manufacturing application. AI can be used to analyze production data and improve maintenance planning. The result is less expensive maintenance of production lines.
AI can be used in many ways, such as more accurate demand forecasting and reduced material waste. Manufacturing and artificial intelligence (AI), a combination of both, are closely linked because humans and machines work together in an industrial environment.
Artificial Intelligence Segments that Impact Manufacturing
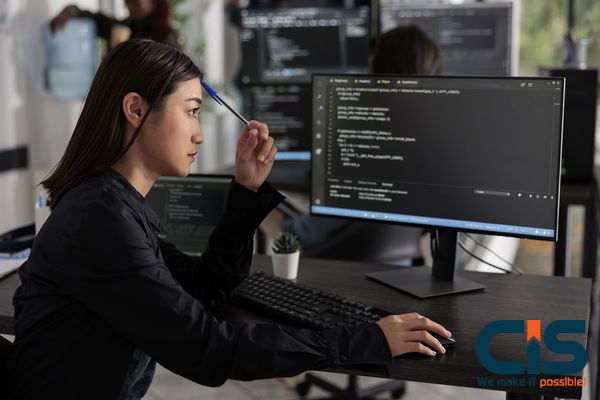
According to experts AI refers collectively to learning systems that can be perceived as intelligent. These include image and video recognition and prescriptive modeling, intelligent automation and advanced simulations, complex analytics, and many other capabilities. AI applications in manufacturing are centered around these technologies.
- Machine Learning: Using algorithms and data to learn automatically from patterns without being explicitly programmed.
- Deep learning: Is a subset of Machine Learning that utilizes neural networks to analyze images, videos, and other data.
- Autonomous objects (AI agents): Agents that can perform tasks independently, like collaborative robots and connected vehicles.
The AI market for manufacturing will grow by 57 percent, from $ 1.1 billion in 2020 to 16.7 billion by 2026. This growth is primarily attributed to extensive data availability, increased industrial automation, improved computing power, and higher capital investments.
What is the use of AI in Manufacturing?
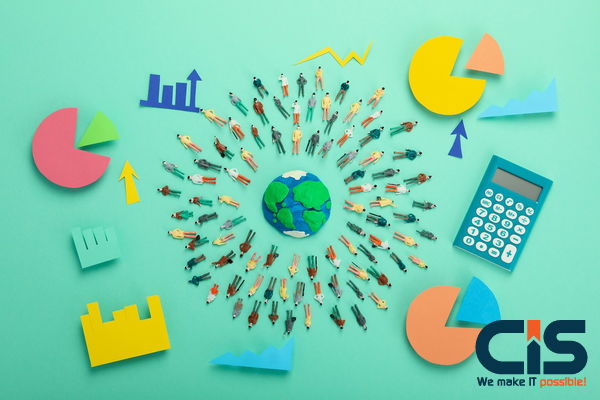
Here are some examples of artificial intelligence in the manufacturing industry:
- In the first phase, you would teach AI how to perform a particular task by observing what humans do. This will not only lead to progress but also will allow for expansion. With enough practice and time, it will eventually learn to do many tasks independently.
- The next step is crowdsourcing. This method allows you to collect public data to train AI. This can be done instantly, and the AI will compare its results with anything it already has. This will result in an AI that can access collective knowledge and "hive-mind" or know what others think.
- Unsupervised learning makes it possible to supervise AI. It can learn without having to be told. How will it learn new information? The technique they would use is called reinforcement learning.
AI and the Industrial Sector
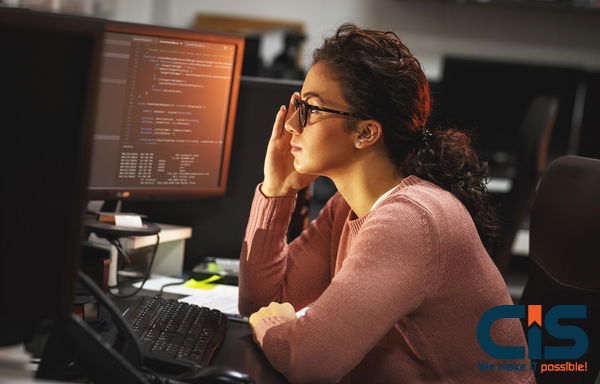
Prevention of Future Problems
Artificial Intelligence (AI) helps manufacturers predict when and if the equipment will break down so maintenance can be scheduled before it happens.
Create a New Idea
Machine learning algorithms mimic the engineering design process in generative design. This technique allows manufacturers to create hundreds of different design options quickly.
Predicting Raw Material Prices
AI software can predict the prices of commodities more accurately than human beings and improves over time.
Aspect Analyses
Edge analytics is a method of delivering quick and decentralized insights using data sets collected from sensors.
Quality Controls
Quality assurance is the process of maintaining a certain level of quality for a product or service. AI systems that use machine vision can detect deviations because most flaws will be obvious.
Robotics
Manufacturing robots are industrial robots that automate repetitive operations. They eliminate or reduce human errors drastically and refocus human workers' attention on profitable areas.
Process Improvement
AI software development can help organizations achieve sustainable production by optimizing their processes.
Enhancement of Shop Floor Performance
The digital twin allows you to examine and track the entire production cycle to identify any potential problems with quality or performance.
AI in Manufacturing
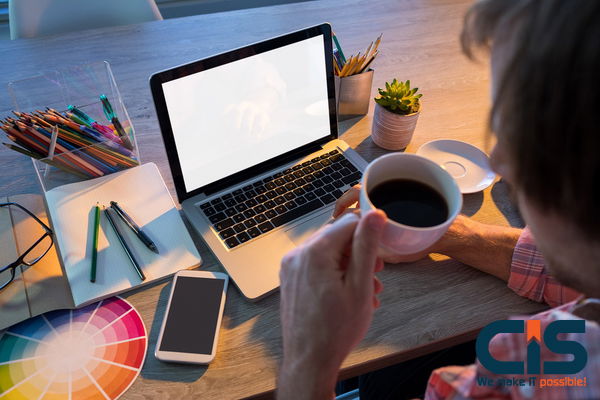
AI has a significant impact on manufacturing. Artificial intelligence improves the manufacturing process on many levels.
Artificial Intelligence for Logistics
Overstocking and understocking continue to be a problem. Overstocking can lead to waste and reduced profits. Businesses can gain money, sales, and customers by adequately stocking products.
AI Robots: Robotic Process Automation
Robotics in manufacturing, also known as industrial robots, allows for automation, reduces or eliminates human errors, and reallocates labor from monotonous tasks to more valuable activities.
The potential applications of robots in the manufacturing industry are numerous. Several industrial robots are equipped with machine vision, which allows them to maneuver precisely, even in chaotic environments.
Want More Information About Our Services? Talk to Our Consultants!
Manage Supply Chains with Artificial Intelligence
AI can help factories manage the entire supply chain, from forecasting capacity to inventory management. Businesses can be alerted to any disruptions in their supply chains by establishing real-time, predictive models for monitoring and assessing suppliers.
AI Autonomous Vehicles
They are automating the factory floor with self-driving vehicles, including the assembly line and conveyor belts. Self-driving ships and trucks can help optimize deliveries, operate around the clock, and complete them more quickly.
Connect car sensors can provide real-time data on traffic, road conditions, and accidents. This information helps plan delivery routes and reduce accidents. The change improves the safety and speed of delivery.
Artificial Intelligence for Factory Automation
The factory operators rely on intuition and their knowledge to manually adjust equipment while watching various indicators on multiple screens. Operators in this system will now be responsible for testing and troubleshooting the system and their regular duties.
Some business owners ignore the importance of generating or minimizing a return on their investment or minimizing it, which can lead to undesirable results.
AI in IT operations
AIOps is an essential tool for achieving this goal. AIOps is a Gartner-defined approach to IT automation using big data and machine intelligence.
AIOps can be very helpful for automating large data sets. AIOps can be used for IT service management, event correlation, analysis, performance, anomaly detection, and cause determination.
AI Design and Production
AI software can generate iterations that are better than the original design. This software is also known as generative software and asks for designers to provide inputs such as:
- Basic Ingredients
- Mass and Measurements
- Processing Techniques
- Financial and other resource limitations
- Based on the inputs, an algorithm could generate a variety of possible layouts.
Internet of Things and Artificial Intelligence
Internet-of-Things devices (IoTs) have sensors and produce a large amount of data in real-time. This concept is called the Industrial Internet of Things (IIoT) in manufacturing It solutions. AI combined with IIoT in the factory can improve output and precision.
AI for Warehouse Management
AI can automate many aspects of warehouse management. The data manufacturers can help them monitor the stockrooms they manage and improve logistics.
Automating quality control and inventory can reduce costs, increase productivity, and require fewer workers to perform the task. As a result, manufacturers can increase revenue and profits.
AI Process Automation
Artificial intelligence software can optimize business processes and maintain production levels indefinitely. Manufacturers can use AI-powered technology for process mining to identify and eliminate inefficiencies.
For example, in manufacturing, meeting customer needs requires you to meet them on various levels, such as promptly and accurately delivering products.
AI Predictive Maintenance
Manufacturers use AI to analyze sensor data and predict failures and accidents. Artificial intelligence helps production facilities predict future breakdowns of operational machinery. This allows for scheduled preventative maintenance. AI-enabled predictive maintenance allows factories to increase productivity and reduce repair costs.
AI-Based Product Development
AI-based products allow producers to test multiple models before producing AR and VR.
Maintenance and bug fixing should be simplified. Manufacturers may enhance and accelerate their innovation with AI-based product creation, creating new, more progressive items that hit the market ahead of the competition.
AI-based Connected Factory
Sensor- and cloud-equipped "connected" or intelligent factories are the future. Innovative manufacturing practices can help:
- Shop floor vision in real-time
- Watch how you are using your resources.
- Remote systems that operate without the need for hands are easy to install
- Be prepared to act quickly.
AI for Quality Assurance and Inspections
AI-driven fault identification uses computer vision to identify flaws. This technology employs high-resolution cameras that observe each step in production. This system would detect flaws that a human eye might miss and initiate immediate efforts to correct them. This means fewer products must be recalled, and fewer will go to waste.
Workplace hazards can be avoided when abnormalities such as poisonous gasses are detected immediately.
AI for Variation in Purchasing Prices
A change in input prices can significantly impact a producer's profits. Estimating raw material costs and selecting vendors are the most challenging aspects of production.
It is also easier to keep track of all parts bought from different vendors and manage them in one location.
AI Order Management
Order management requires flexibility, including in terms of the consumer, market, and demand. It also involves a shift in manufacturing strategies. AI systems and manufacturing robots can:
- Use inventory sensors to generate purchase orders instantly.
- Manage the complexity of multiple order types through different sales channels.
- Order and Inventory Management can be streamlined and improved to improve clarity.
AI in Cybersecurity
Studies show that manufacturing companies are the ones who suffer the most significant losses due to cyberattacks. Even a tiny interruption in production can have disastrous consequences. As the IoT grows, so will its dangers. The number of cyberattacks against innovative industries is increasing.
Artificial intelligence and algorithms for risk detection can help protect industrial sites and reduce their vulnerability.
Immerman explained that when we pull large amounts of data at high frequency from the tooling process, we look at it a thousand times closer to standard levels to detect potential microfractures or chips which were not previously visible. With this information, we can apply machine learning to the data and identify problems before they occur, saving our customers time and money.
It was once easy to tell when a machine would fail, but it wasn't always possible. The breakage was so significant that it was necessary to shut the machine down, regardless of the minor flaws. This process is quaint due to the increased ability to retrieve new data.
AI is becoming increasingly crucial as competitors compete for limited skilled workers, and knowledge of institutions may be lost with retirees.
Immerman said that as more businesses understand the benefits of machine learning, they can see how it can go beyond simply fixing broken parts. Humans have tracked cycle times and part counts for years. Platform platforms such as MachineMetrics aggregate data and learn AI can suggest reasons machines are down. It can also help improve quoting and scheduling. Sales forecasts may even need to be adjusted.
Digital Transformation Personas
Many companies have access to deep data insights. They are ready to invest but lack the direction to know what investment level will yield maximum value.
A report "Capturing Industry 4.0's true value" breaks down three different types of businesses poised to enter the AI race.
The "cautious start-ups" consist of businesses just starting to investigate a journey toward digital transformation. The companies need help gaining a bigger picture because they want to implement a digital transformation strategy across the entire organization.
The "frustrated experimenters," who have begun dabbling with some success, remain frustrated by a lack of clear understanding of measuring or achieving ROI.
The "ready-to-scalers," however, struggle to achieve full benefits at scale. It is essential to refocus on the benefits they are aware of and respond to customer, and business needs changes.
Read More: What Is Machine Learning? Different Fields Of Application For ML
Extrapolating Insights From Data
Data analysis is the key to a successful digital transformation, whether you are a company just starting or a large enterprise.
leader, said that the cloud-enabled manufacturers use data-driven AI applications, which reduce manual work, eliminate human error and improve operational efficiency. Embedded data-driven intelligence is essential in an industry that demands efficiency in everything from production lines to supply chains.
Prioritizing efforts to quickly and securely utilize this mountainous manufacturing data will help businesses create better products, remain competitive, allow AI-led innovations, and better prepare for the rapidly changing future.
AI integration has allowed manufacturers to realize their data's value and the intelligent solutions that can be used to monetize it to reduce operational costs and improve mission-critical workflows. The quantity has identified four methods by which manufacturers can integrate cutting-edge AI in all aspects of their manufacturing ecosystem.
First, engage with manufacturers to monitor and regulate production operations. The most difficult challenge for manufacturers is collecting machine and plant data to measure and see everything along the value chain
Second, AI helps organizations to solve challenges in the supply chain. Organizations use AI to help them overcome problems with demand forecasting and planning. They also leverage historical data and geopolitical and weather scenarios.
The third element is optimizing the business operation. An AI-powered solution such as Document AI automates the validation of documents and streamlines workflows to reduce confusion and speed up processing. This helps regulate business operations and drastically decreases processing time. The data can enhance customer experience and reduce the time it takes to acquire new customers.
Expert explained that "data generated by customer interactions can be used for customer profiling and churn predictions, as well as other use cases which help to enhance revenue." Businesses can use AI and machine learning to enhance the customer experience with features like chatbots or personalized recommendations.
Sustainability is the fourth and most significant segment in which AI has advanced. Singh said, "Efficiency is linked to sustainability, as many businesses strive to incorporate this into their processes." Energy footprints are primarily affected by the efficiencies that can be brought to the supply chain and manufacturing workflows. AI has the broadest range of applications across all industries. The whole purpose of AI and machine learning in manufacturing is to improve efficiency.
AI and Sustainability: The Role of AI is Changing
AI will play an increasingly important role in the sustainability of a company's operations as more and better legislation is passed.
AI Expert, admitted that five years ago, the chances were low for the sustainability director to know anyone working on the floor. The situation has changed dramatically in recent years as the sustainability issue has gained more prominence and attention. Artificial intelligence must now follow.
With supply chains still not operating at maximum capacity--and perhaps never getting back to pre-pandemic levels--manufacturers are considering using more recycled materials to meet customer needs because they can be more readily available. However, there are often significant differences in specifications between recycled and raw materials.
When working with raw materials, manufacturers have a variable that is controlled. They know where the material comes from and what it will do to specific processing. The specifications of a recycled product are much more variable. The manufacturer may not know where this material comes from or whether it is of lower quality. It will fit in the final part but taking longer to refine how it fits. This is a procedure that must be completed.
AI can provide a detailed view of how raw and recycled materials interact, which is crucial to ensure that everything functions together.
The AI was used to show how useful it can be if properly implemented and tailored for specific purposes within the manufacturing process.
AI Journey: The Next Step
AI is no longer exciting, but it still needs to be proven to manufacturers that AI can bring tangible benefits to their business. They must spend time, resources, and money to prove their value. Where does AI go next?
Every manufacturer is asking themselves this question as they continue to face delays, issues, and problems caused by the global pandemic.
A leader in intelligent manufacturing solutions delivered via the cloud), said: "Our customers' focus has been on survival for three years. This is understandable, given that unprecedented uncertainty has disrupted plans." As we get further from the global manufacturing upheaval, many companies will be able to benefit from new artificial intelligence solutions.
Customers benefit from AI advances when they identify previously unapparent patterns. Comparing data across companies and industries became possible as AI and ML developed.
Foster said that the goal of machine learning is to discover patterns previously unknown. Combining contextual data with something allows us to find better information that can be used widely.
Foster said that a Plex algorithm created with AI might be able to see patterns from one client that are similar to those in other areas. The flags help users discover similarities they might not have noticed and give them insights they would never have imagined.
Foster said, "This intelligent configuration tool is one we can test with customers internally to determine if it is worth exploring." When we are satisfied that it works, we will make it available to all customers.
Foster explains that the next frontier could be adaptive AI or AI, which writes itself. The next frontier is adaptive AI, or AI that writes itself. Foster explained.
While this may seem a general idea, Foster said the technology is not out of reach and can allow businesses to gain valuable insights without hiring specialized data analysts.
Want More Information About Our Services? Talk to Our Consultants!
The Conclusion of the Article is:
Integrating AI into manufacturing as quickly as possible is essential to reap its benefits. To do so, you must invest a lot of resources and time. You'll also have to upgrade your employees. Finishing pilot projects quickly and moving out of the pilot stage is essential. The time to incorporate AI in production is rapidly running out for those still interested.
AI has become a significant part of manufacturing, with yearly growth. There are skill sets in shortage, and training is an excellent way to fill them. AI engineers can use a variety of intelligent agents to create applications. Machine learning experts are also in demand. They are taught supervised and unsupervised learning techniques, mathematics and heuristics, and modeling. Deep learning experts are in high demand and are trained to understand TensorFlow - an open-source library for conducting machine learning research and researching deep neural networks.