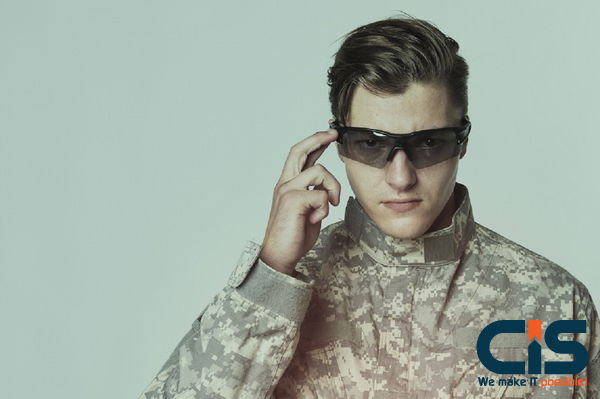
What is Artificial Intelligence?
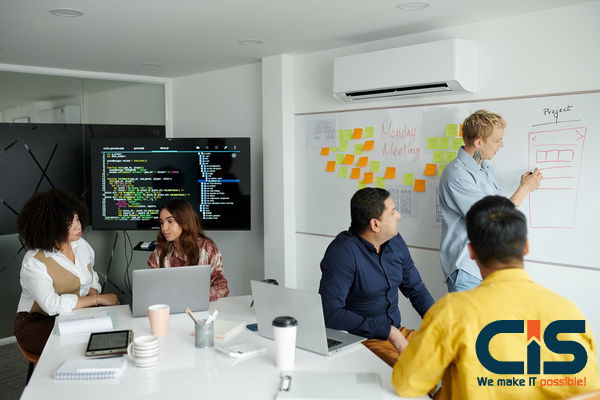
AI in manufacturing refers to machines' ability to mimic human intelligence in performing human-like tasks--responding to events internally and externally, anticipating them, even. Machines can detect unexpected events or worn-out tools and react immediately.
Historians trace human development from the Stone Age through the Bronze and Iron Ages by measuring human control over the natural environment, tools, and technologies. We live in an age of machine-learning AI systems that allow individuals to decide their application - they don't take over from humans! AI works best as an aid for creative people doing more; rather than replacing humans with its work, its ideal applications should allow people to pursue what they are uniquely good at, such as manufacturing products in factories or designing them from a design standpoint.
Industrial robotics require constant supervision; as their AI intelligence improves, this makes the relationship between humans and robots safer and more effective. AI and machine learning are often used interchangeably; AI incorporates machine learning as one subfield of its practice.
Want More Information About Our Services? Talk to Our Consultants!
Robots for Production
Artificial intelligence is frequently referenced when discussing intelligent industrial robots used in manufacturing industries. Machine learning technology is one of the most valuable aspects of industrial robots; these machines can learn from past errors to anticipate and identify future issues or malfunctions independently.
Companies have increasingly adopted digital technology in their manufacturing processes. According to a BCG/MIT Sloan Management/MIT Sloan Management study, 84% of executives viewed intelligent machines as providing a competitive advantage.
Robots have proven effective in automating production processes such as welding, soldering, and spraying with their cameras and sensors for accurate identification of individual parts, picking, placing, and assembling of components accurately, picking or assembling items accurately, as well as being used for highly automated processes like laser welding soldering spraying, etc.
Robot-assisted inspection can also be integrated into production lines, which is instrumental when dealing with products with prominent form factors. Programmable robot arms can help assemble heavy components while aiding employees during production - precision, and quality are of the utmost importance since even minor product defects could lead to severe injuries for employees and customers alike.
Inspection Of The Cleanroom Using Ai
High-quality manufacturing is necessary when producing complex medical devices, such as infusion pumps, laboratory instruments, or single-use products. Such items must adhere to stringent hygienic guidelines for production.
Cleanrooms use systematic ventilation and filtering to minimize environmental risks and particle production. If even one hair is detected in sterile packaging, entire batches could be recalled; this would incur huge costs and cause thousands of products to be recalled from shelves. To prevent contamination during production, robots and automation technologies are used.
Robot-assisted inspections utilize built-in cameras to look for defects in components or other objects and identify objects by visual features like markings, notches, or other visual characteristics. The robot can locate and identify these features using visual cues such as markings or notches that serve as landmarks for identification. Artificial intelligence evaluates and compares images in milliseconds, providing real-time feedback as to whether all parts are assembled correctly or whether any deviations will be reported by the robot at an early stage in production.
Also Read: AI Enables What's Next in Manufacturing
Data Analysis In The Iiot
Machine learning helps transform robot-assisted inspections, setting the foundation for the Industrial Internet of Things. Industrial robots collect and process a wealth of data sets that assist in automating and programming more efficiently; AI applications can utilize these linked sets more easily in learning patterns more rapidly.
Predictive maintenance is an example; sensors gather data about machine performance and track maintenance requirements, enabling repairs before defects appear and downtimes avoided, saving money. Data analytics provide a 360-degree perspective of products that allows agile engineering to reduce risks quickly and react swiftly to market fluctuations, saving both time and money.
Smart Factory: More than Science Fiction
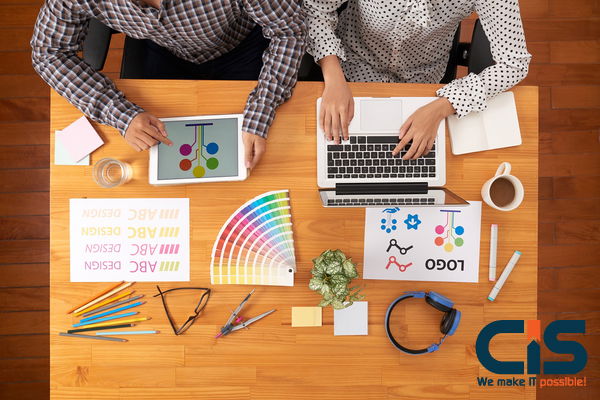
Time and money must be dedicated to integrating disruptive artificial technologies into an intelligent factory, taking small steps to implement new processes gradually before assessing AI's value in various business sectors and applications. No single company should attempt this undertaking by themselves.
What impact will robot-controlled manufacturing have on product design and development processes? Artificial Intelligence makes a lot of sense when it comes to production. How can an IT infrastructure effectively handle data flow analysis?
Robot-assisted automation solutions bring many advantages: increased precision, reduced downtime, consistency, and accuracy; it also decreases time-to-market and quality; eventually, robots may live alongside humans and demonstrate real AI. Artificial intelligence and automation have already become part of many production processes. Over time, AI/Automation will enable even more advanced products.
How Has Ai In Manufacturing Evolved?
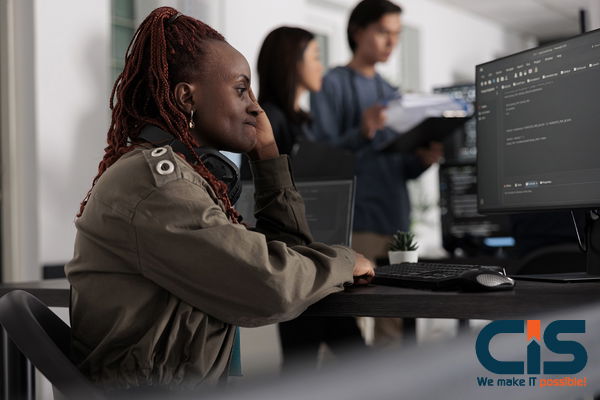
AI can be applied in manufacturing for nondestructive testing (NDT), measurement, and other processes. AI has also been employed in product design; however, adoption in fabrication remains at an early stage; machine tools remain relatively dumb despite reports about automated shop tooling; many factories still rely on outdated equipment with limited or mechanical interfaces for operating processes.
Newer fabrication systems feature human-computer interfaces and electronic sensors to provide feedback about raw material supply, system status, power consumption, and many other factors. A machine or computer screen helps people visualize their actions; as AI's potential in manufacturing becomes clear, its future looks promising.
Nearer-term scenarios involve real-time monitoring of machining operations and status inputs such as tool wear. This application of AI is known as Predictive Maintenance, where algorithms analyze continuous streams of sensor data in real-time for patterns and apply analytics to forecast issues in advance.
Machine sensors may detect when something unusual happens - for instance, an acoustic detector listening for belts and gears beginning to wear or an instrument monitoring the wear on tools - This information could then be used to create an analytical model that estimates their remaining life expectancy. Additive fabrication has quickly become a go-to practice on shop floors worldwide, leading to the addition of numerous sensors designed to track changes that impact materials and fabrication technologies.
The Current Status of AI in Manufacturing
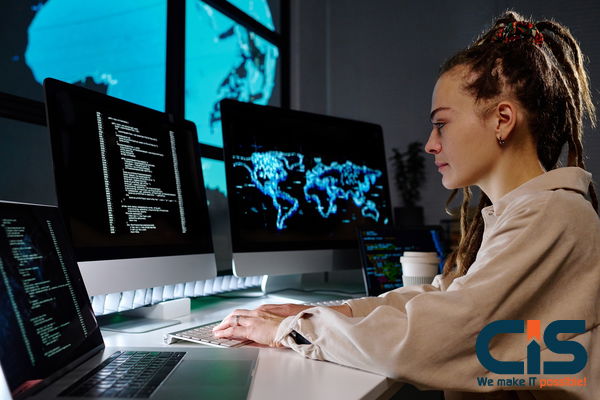
AI allows for much more precise manufacturing process design as well as more accurate diagnostics and resolution of defects during fabrication processes, thanks to Digital Twins. A Digital Twin is a virtual copy of physical parts, such as machine tools or parts, serving to diagnose defects during the fabrication process more accurately than simply by looking at a CAD model of them; instead, it acts like an exact digital copy that depicts their behavior if there were an issue (all parts contain defects); AI is needed for their implementation into manufacturing process maintenance and design processes.
Large enterprises stand to benefit significantly from adopting AI and possessing the financial strength necessary to fund these innovations. However, some of the most innovative AI applications have come from more minor to medium-sized enterprises (SMEs) like contract designers or manufacturers supplying technology-intensive industries like aerospace.
End-to-end engineering expertise can be integrated into a manufacturing process via onboard AI tooling, with the knowledge to direct installation, adoption, sensors, and analytics for detecting operational and maintenance issues (which may involve "unsupervised models" trained to search out odd or "wrong" aspects within sensor feedback).
A real-world example of this concept can be seen with the Digital Reconfigurable Additive Manufacturing facilities for Aerospace) project, launched. Autodesk joined a consortium of companies working with the Manufacturing Technology Centre (MTC) to prototype a "digital learning factory." Digital twinned additive-manufacturing process chains will allow reconfiguration to meet different user needs while testing different hardware and software options; developers are creating an additive manufacturing knowledge base that aids technology and process adoption.
Autodesk plays an essential role in DRAMA as an integral player in design, simulation, and optimization, taking complete account of manufacturing's downstream processes. Deliberating how those effects manifest on each part is critical information humans can automate before applying generative design tools to bring digital designs to physical reality.
How AI Could Transform the Manufacturing Industry
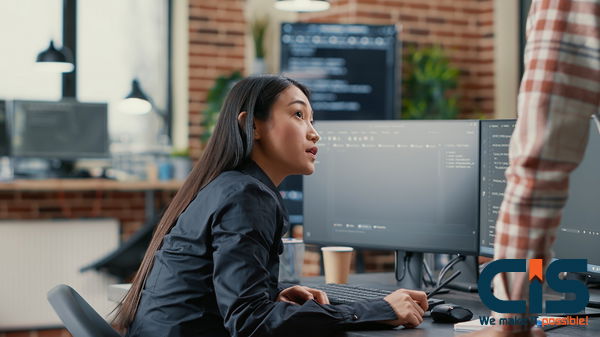
This scenario allows manufacturers to package end-to-end work processes to sell to manufacturers effectively. This could involve everything from software and physical machinery in the factory, their digital twin, ordering systems that exchange data with supply-chain systems in the factory, analytics monitoring production methods and collecting data as inputs move through, as well as analytics for monitoring manufacturing methods and collecting information as inputs enter. In other words, creating "factory in a box" systems.
Factory in a Box
An NDT system would enable a manufacturer to examine parts being produced today and compare them with parts produced yesterday, as well as analyze NDT performed on each process in their line. Feedback provided would enable the manufacturer to understand precisely which parameters were used in making those parts, along with any defects detected from sensor data.
Idealized, that process would involve loading materials at one end and receiving parts at the other, with people only needed for maintaining systems, as most work can eventually be handled by robots. Unfortunately, in reality, people still design and make decisions, oversee manufacturing operations, work in several line functions, and make critical line decisions themselves - but thanks to a system that helps them understand what effects their decisions have.
Machine Learning and Autonomous AI
AI's power lies in its capacity for self-organizing systems like machine learning, neural networks, and deep learning to learn without human interference, rapidly discovering patterns that would otherwise escape analysis by human analysts. Today's manufacturing practices, however, still depend heavily on human experts directing AI application development by encoding previous systems they've engineered with their expertise - human experts bring ideas of what has occurred, what went wrong, and what has gone well.
Autonomous AI will soon build on this repository of expert knowledge to offer employees in, say, additive manufacturing the benefits of operational feedback. At the same time, AI analyzes sensor data for preventive maintenance and to refine processes. That could eventually evolve into innovations like self-correcting machines; when tools wear out, adaptive systems adjust themselves automatically while suggesting replacement of worn components.
Factory Planning and Layout Optimization
AI applications go beyond fabrication processes alone; AI can help in other ways as well, including factory planning. Facility layout decisions depend on variables ranging from operator safety and process flow efficiency to reconfiguration needs that adapt quickly for short-run projects or frequently evolving processes.
Sensors Capture Data for Real-Time AI Analysis
When adopting new technologies that involve considerable uncertainty, such as additive manufacturing, NDT is an essential step after the part has been produced. Nondestructive testing can be costly, mainly if it involves capital equipment like CT scanners (used to evaluate the structural integrity of manufactured parts). Sensors installed in machines can connect with models developed from abundant data collected during manufacturing for specific parts.
Once sensor data are available, machine-learning models can be built from it--for instance, to correlate with defects identified during CT scanning. Sensor data could then be used to flag any parts likely to contain defects without undergoing CT scanning on every piece that comes off the line. Thus, only the part with likely defects must be examined further.
Operational metrics also allow businesses to track how people are using equipment closely. When designing machinery, manufacturing engineers make assumptions about its usage; when used by humans, there may be extra steps or missed steps that cannot be accurately captured using human analysis alone - sensors capture this data accurately for AI analysis.
AI can play an integral role in adapting manufacturing processes and tooling to different environmental conditions where they might be applied, such as humidity. Additive-manufacturing developers have noticed that some machines don't function as designed in certain countries, with humidity sensors monitoring conditions within factories discovering surprising things like people leaving doors open when leaving to smoke outside, causing moisture issues in supposedly moisture-controlled environments.
Effective use of sensor data requires the creation of practical artificial intelligence models. Once these AI models have been trained to recognize what is happening within the data, they must understand its implications, what causes issues, how to detect their causes, and how best to respond. At present, machine-learning models use sensor data to predict when an issue will arise, alert human troubleshooters when this occurs, and react in real-time if issues do arise - and ultimately create proactive ways of solving manufacturing processes through AI systems.
Generative Design
Artificial Intelligence plays an essential role in generative design - a process wherein design engineers submit requirements for a project and then use design software to generate multiple iterations based on AI algorithms. Autodesk recently collected materials data related to additive manufacturing. It uses that information to power its generative-design model prototype that understands how material properties change according to how manufacturing affects individual features and geometry.
Generative design is an adaptable optimization technique. While traditional optimization approaches take more general approaches to part optimization, generative-design algorithms can take a much more specific approach by targeting one feature at a time based on materials testing or collaboration with universities. Although designs may be idealized, manufacturing processes occur in reality so that conditions might change during production processes; an effective generative design algorithm incorporates this level of understanding.
Generative design uses software to generate optimal designs and specifications and then distributes these to multiple facilities with compatible tooling. This enables smaller facilities in various geographic locations to manufacture parts for multiple products simultaneously, such as aerospace one day and then other essential products the next - saving both distribution and shipping costs in the process. Generative design has become an essential concept within automotive production lines as an example of such a design strategy.
Flexible and Reconfigurable Processes and Factory Floors
AI can also optimize manufacturing processes and make them more flexible and reconfigurable. Current demand can help determine factory floor layout and generate processes; similar models can then be compared with each other and analyzed further - such as whether to invest in more large additive machines that may cost less but become unnecessary when demand slows; "what-if" analysis is a widespread application of AI.
Models will be utilized to optimize both shop floor layout and process sequencing. For instance, applying thermal treatment on additive parts directly from the 3D printer could be done quickly or retempered, requiring another heat cycle. Engineers could perform what-if analyses to assess what equipment a facility should possess or outsource parts of its operations to another business nearby.
These AI applications could alter the business case that determines whether a factory focuses solely on one captive process or diversifies to take on multiple products and projects simultaneously, making the factory more resilient; as an example, aerospace manufacturing operations that have experienced a downturn could adapt by producing medical components as well.
Also Read: How AI Can Be Used in Manufacturing in 2022?
Manufacturing and AI: Applications and Benefits
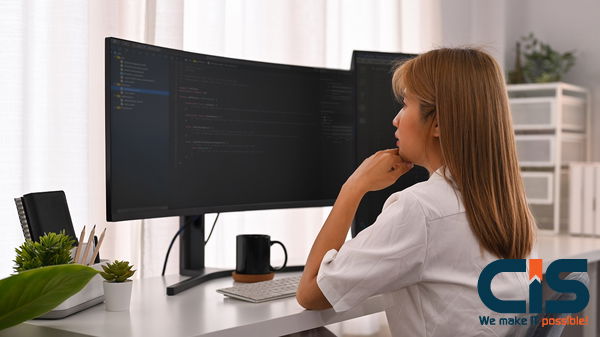
AI will become increasingly integrated into manufacturing over time - already, this evolution is in full effect, from design, process improvement, wear-reduced machines, and optimizing energy consumption - to optimizing energy consumption. All of these elements of manufacturing will utilize artificial intelligence (AI). And AI's presence has already been felt.
Machines are becoming more intelligent and integrated, both with supply chain management and business automation systems. An ideal situation would see materials coming in. Parts coming out with sensors monitoring every link of the supply chain - freeing up essential manufacturing resources to focus on innovation and creating new ways to design and manufacture components--rather than mundane, repetitive work that can be automated.
As with any fundamental shift, AI adoption has met resistance. The knowledge and skills required can be costly and scarce - many manufacturers don't possess these capabilities themselves - which makes justifying investments to create something new or improve processes difficult as these specialized manufacturers see themselves as experts at what they specialize in. Furthermore, many are risk-averse when scaling factories up.
This can make the concept of "factory in a box" more attractive to companies. More enterprises, particularly SMEs, can comfortably adopt an end-to-end packaged process in which software works seamlessly with tooling while using sensors and analytics for continuous improvement. Furthermore, adding digital twin capability means engineers can test out a potential manufacturing process before making their final decision - further decreasing the risk associated with the decision.
AI in manufacturing also plays an integral role in predictive maintenance. Engineers can utilize it to equip factory machines with pre-trained AI models using data collected by these tools; as soon as new patterns of cause-and-effect arise on-site, these models will learn and be ready to prevent potential issues from arising.
AI also plays an integral part in quality inspection, which generates large amounts of data ideal for machine learning analysis. Consider additive manufacturing: one build produces as much as a terabyte of information about how the machine made parts, on-site conditions, and any issues discovered during production; human analysts would be incapable of processing such massive volumes of information quickly enough; AI can do it now! What works with additive tools can efficiently work across subtraction manufacturing, casting, injection molding, and many other manufacturing processes.
Want More Information About Our Services? Talk to Our Consultants!
Conclusion
Intelligent components can notify manufacturers when their part has reached the end of its useful life or needs inspection rather than monitoring these points externally. Instead, this component checks in periodically with AI systems to report normal status until conditions deteriorate. At this point, it begins demanding attention from its maker. This strategy cuts down on data traffic within systems, which at scale could become an impediment to analytical processing performance.
Additive manufacturing processes provide AI with its most immediate opportunity for value addition, being more expensive and smaller volume products than their counterparts. However, as people develop and perfect AI over time, its utility may expand across all aspects of manufacturing value chains.