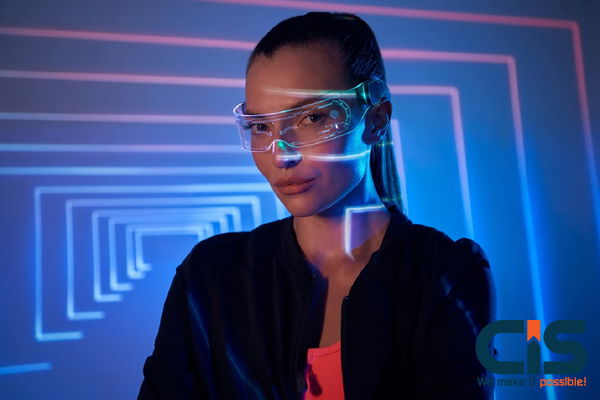
Companies believe Artificial Intelligence will revolutionize their industry. Yet, only one-quarter of large companies have an AI definition or strategy, with less than half of AI projects currently in production at these same firms. The reasons may include missing or inaccurate data, limited data-science knowledge, or organizational hurdles that prevent projects from moving forward as planned, possibly also due to interdisciplinarity requirements of AI projects. Download the recent Luenendonk Study "Artificial Intelligence: Fields of Application and Challenges of AI Projects within Large Companies and Corporations."
AI Projects: Poor Data Quality Needs Revenge
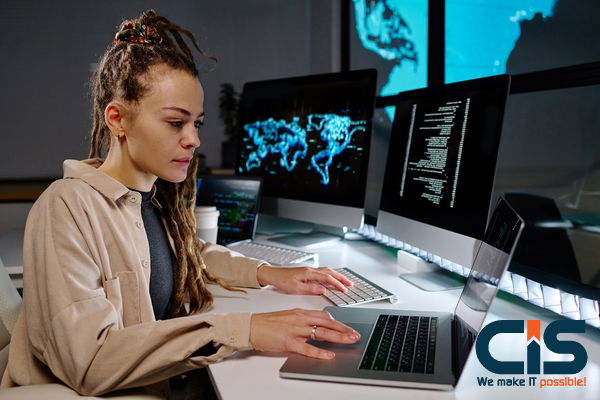
"Data Is the New Oil" has become an increasingly common phrase. This analogy works because oil quality in many companies may be inadequate compared to that of data; pipelines may not be compatible, or companies might need more time before sharing data freely with one another; established businesses often need assistance managing legacy IT systems.
Existing solutions can be integrated as long as there is clean data management and an organized architecture, though implementation presents significant obstacles. Many IT personnel dislike data architectures as they make calculating business cases directly tricky.
AI projects will only be successful if data quality research and architecture are thoroughly understood. More than 70% of companies consider data a fundamental or significant challenge; over half said they did not possess sufficient expertise in data science to implement projects involving this field.
Want More Information About Our Services? Talk to Our Consultants!
Lack of Strategy Slows Down AI Implementation
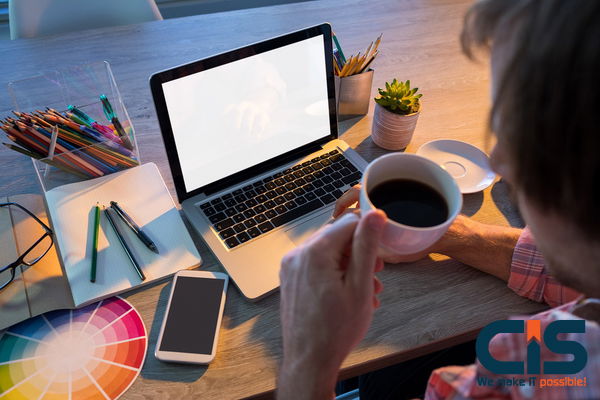
Companies need a comprehensive strategy for modernizing IT systems and developing artificial intelligence expertise. Establishing organizational requirements is essential to ensuring managers and experts fully grasp solutions related to AI solutions. At the same time, an interdisciplinarity strategy may support the implementation efforts of different departments within a company.Companies that follow a plan can only become data-driven businesses over time.
AI Projects: Many use cases already exist
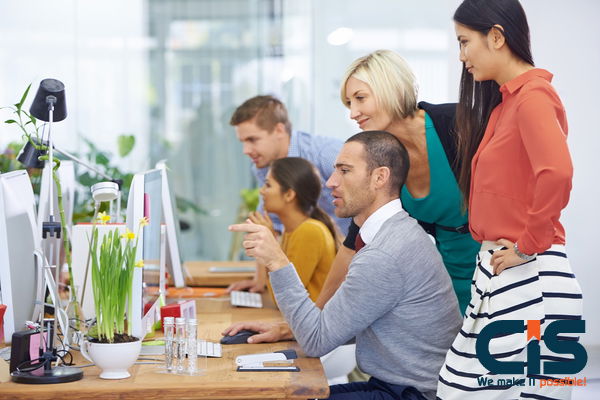
Artificial intelligence isn't as mysterious or abstract as many believe; AI is used across many business areas, from customer service and marketing and sales production to development and production management. A study from UConn included specific examples of AI's implementation within customer service, marketing & sales, production & development, and customer service and production/development environments. Most respondents also felt AI had high future potential across most aspects of business, including being crucial for marketing & customer service activities.
Sales grow as fields of action expand. Businesses expect happier customers and higher sales by providing customers with personalized and intelligent interactions 24 hours a week, seven days a week. Most interviewees noted that AI could be used for decision-making; however, AI may not always be the ideal tool for this task.
How to Create an AI Marketing Strategy
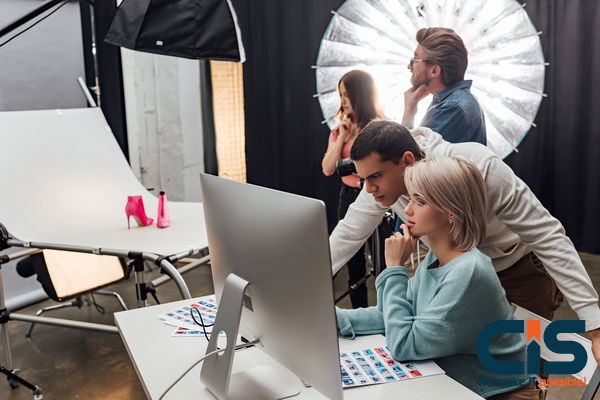
Artificial intelligence (AI) can be an invaluable marketing asset. Marketing involves understanding customer needs, matching products, and persuading people to purchase. AI can significantly amplify these functions; chief marketing officers are increasingly adopting this technology.
According to an American Marketing Association study conducted over one quarter, implementation had increased by 27%, and three of their top five goals related to improving existing products/services, creating new ones, and maintaining relationships. early adopters showed three top goals explicitly related to marketing: improving existing/services/creating new ones/enhancing relationships/enhancing customer relations/improvements were among three.
Also Read: 7 Types Of Artificial Intelligence (AI)
Today's AI
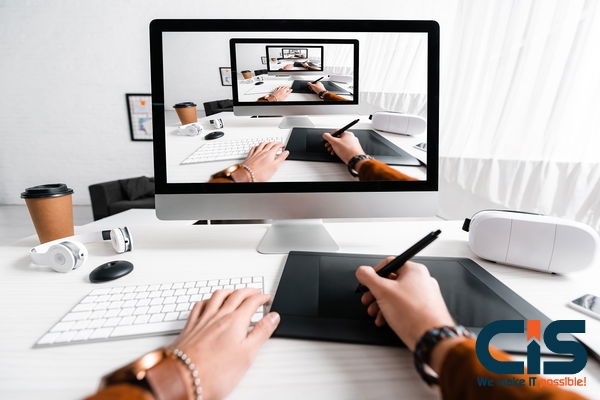
AI has become an invaluable asset to firms today, being used in everything from digital ad placement (also referred to as "programmatic purchasing") and improving forecast accuracy (e.g., sales forecasting) to augment human effort during structured tasks like customer service or augment human effort in structured tasks like customer care (see the sidebar "Established AI Applications in Marketing." For a list, see "Well-Established AI Applications in Marketing."
AI can streamline sales processes using highly detailed individual information, such as geolocation data, to create tailored product or service offerings that suit individuals perfectly. AI bots, for instance, could give testimonials to customers after filling their cart - for instance: "Great Purchase!" James from Vermont bought this mattress, etc. - increasing conversions by fivefold!
Artificial Intelligence-enabled agents from Amelia (formerly IPsoft) and Interactions are always on hand to meet customer requests 24/7, efficiently managing fluctuating service volumes more effectively than human agents.
AI agents such as these can answer simple inquiries regarding delivery times or appointment scheduling, as well as escalate more complex matters to human agents for resolution. AI can even support human agents by analyzing customer tones and suggesting different responses; furthermore, it can coach agents on how best to meet customers' requirements or suggest interventions by managers.
The Framework
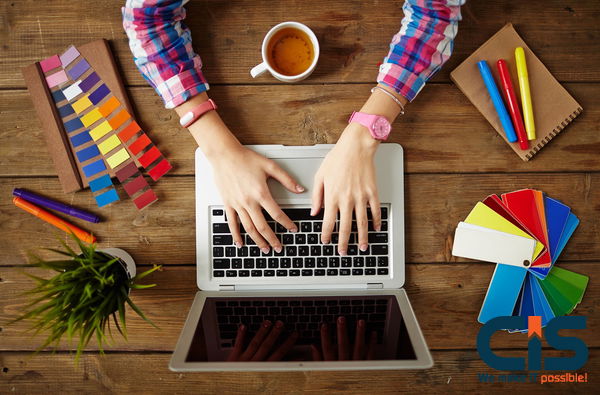
Marketing AI can be divided into two broad categories, depending on its intelligence level and use case. Chatbots and recommendation engines, for instance, can fit into any one or more categories depending on their usage within an app or platform. Let's first examine the two types.
Automatism Of Tasks
These applications are designed to perform repetitive and structured tasks that require low intelligence. They utilize predetermined operations or follow predetermined rules based on input; however, they cannot manage complex issues such as customer requests. A system that sends an email greeting to every new customer would fall under this category, as would simpler chatbots like those found on Facebook Messenger and other social media platforms - these bots may help customers with fundamental interactions via guided decision trees but cannot recognize customer intent, customize responses or learn over time.
Machine Learning Is A Growing Field
These algorithms are trained using large amounts of data to make complex predictions and decisions - their models can decipher texts, recognize images, segment customers, and predict how they'll respond to promotions such as coupons. Machine Learning has already found application in online advertising campaigns, recommendation engines for eCommerce stores, and sales propensity model CRM systems; more advanced techniques like deep learning or its less sophisticated version of machine learning have become popular AI technologies and powerful marketing tools; however, machine-learning apps still require training with large amounts of data before becoming helpful marketing tools. Let's now consider standalone versus integrated AI.
Stand-Alone Applications
AI programs are distinct entities that exist independently from the channels customers and employees use to learn about, purchase, and get support when using company offerings or the channels employees employ to market, sell, and service these offerings. Put, employees or customers need to access AI beyond these channels.
Behr Paint Company has developed an application to assist with color discovery. Utilizing IBM Watson's natural-language processing and tone analyzer capabilities, this app provides customized Behr paint color recommendations based on the mood preferences of consumers in any given room. Users may select two or three hues before selling it directly through Home Depot through this connection.
Applications That Are Integrated
These AI applications, when embedded within existing systems, are less noticeable to customers, salespeople, and marketers than standalone applications. Platforms used for managing the buying and placement of ads use machine learning technology to make split-second decisions on which digital ads users should see. Netflix uses integrated machine learning technology for video recommendations on its menu when viewers visit its website; otherwise, users must use an independent app to request recommendations.
Machine-learning capabilities are now being embedded into CRM products. Salesforce's Sales Cloud Einstein suite incorporates several AI features, such as ranking B2B leads based on their likelihood to purchase. Cogito's AI coach for call center sales people integrates their app with Salesforce CRM as well.
Our four-quadrant framework combines two forms of intelligence with two structures, specifically stand-alone applications for machine learning, integrated apps that support machine learning, and task automation apps that operate stand-alone but integrate. Understanding which quadrant applications fall within can assist marketers with planning the introduction and introduction of new uses.
Step-by-Step Approach
Marketers will likely gain more by exploring machine-learning applications than task automation and rule-based systems alone. Still, simple task automation and rule-based systems may improve highly structured processes while providing viable commercial potential. Furthermore, machine learning and task automation are increasingly combined to extract data from messages, make complex decisions, personalize communications, etc.--thereby creating a hybrid that bridges all four quadrants.
Stand-alone applications remain helpful in situations where integration is either impossible or challenging. However, we advise marketers to gradually incorporate AI into their current marketing systems rather than continuing with standalone apps; AI Survey, 74% of global AI executives indicated this goal in three years.
Getting Started
If your firm lacks AI experience, purchasing or building simple rule-based apps could be beneficial. Many companies employ a "crawl, walk, run" strategy in which they initially deploy task automation apps that do not directly impact customers - perhaps, for example, one to guide human service agents when engaging with customers - which is usually best practice.
After developing basic artificial intelligence skills and amassing sufficient customer and market data, companies can transition from task automation to machine learning. Stitch Fix uses AI to help their stylists make offers based on customer feedback, style preferences, and the items kept or returned - becoming even more powerful when customers were invited to select images from its Style Shuffle tool - further enriching its models' data outputs.
Marketers must constantly look for new data sources, including internal transactions, external suppliers, or potential acquisitions; AI applications and machine learning require large quantities of high-quality information. One machine-learning-based pricing model used by the charter jet firm XO to increase EBITDA by 5% was utilizing external sources for supply data regarding private jet supply along with factors influencing demand such as significant events, macroeconomic factors, seasonal activity patterns, or weather. Although their model used publicly available data, it would be wiser for marketers, whenever possible, to seek proprietary sources rather than public data models, which could easily be copied by competitors.
Challenges And Risks
Even the most straightforward AI applications can be challenging to implement; less advanced task-automation AI may still prove challenging to configure to specific workflows. Companies must acquire the necessary AI skills. Any integration requires careful integration between machine and human tasks, not hindering individual skills or creating problems. At the same time, many organizations rely on chatbots with rules-based automation for customer service automation; more limited bots may only serve to frustrate customers instead of aid human agents and advisers more directly.
Companies adopting sophisticated and integrated applications will face additional considerations as they adopt advanced AI applications, including difficulty in incorporating them with third-party platforms like Procter & Gamble's Olay Skin Advisor, for example. Olay Skin Advisor uses deep learning technology to analyze customer selfies, assess age and skin types, and recommend products. It is integrated into Olay.com as an ecommerce and loyalty platform. Olay Skin Advisor has seen increased conversion and bounce rates, as well as average basket sizes, across some regions. Unfortunately, its implementation with Amazon and retail stores, which account for most Olay sales, proved more challenging; furthermore, Olay does not feature its vast store on Amazon and, therefore, cannot provide customers with a seamless AI-assisted experience.
7-Step Ai Project Management Guide
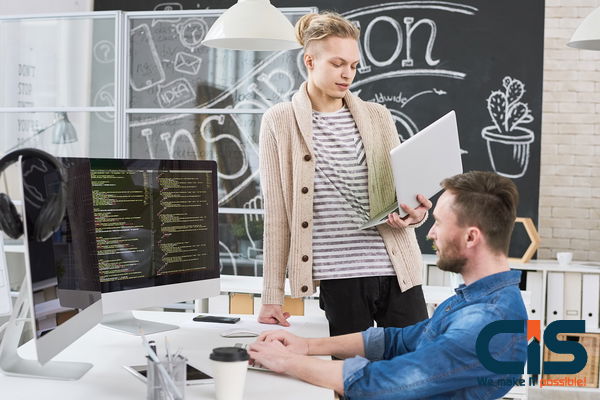
Most AI projects today use waterfall and agile processes for their creation and execution. Waterfowl projects begin with waterfall planning before switching to agile once data flows and model architecture become stable.
As the industry matures and advances, tools, and processes will emerge to support an Agile approach. However, in the interim, you should employ both waterfall and agile processes in your project management processes. Here is an outline of the AI project development cycle:
Requirements Gathering
Documenting and gathering requirements is the starting point for an AI project. Requirements are the cornerstone of any successful initiative and ensure that projects meet user and stakeholder expectations. Project managers should include these aspects in their requirements-gathering process:
- Project Overview: Provide an overview of your project's purpose, desired outcomes, goals, and objectives.
- Roles and Responsibilities: Outline all team member roles. Include stakeholders, those providing input for project requirements definition, and management levels. Utilize task management software to assign tasks to team members.
- Constraints: It is essential to document any potential restrictions on the project that might impede its planning, including time, budget, and technology constraints. Tech Stack: Once you have selected a tech stack, it is important to document it now; leave the decision of AI tools up to your technical team.
- Functional Requirements: Document the requirements that outline how the system is expected to function from an end-user's viewpoint. Nonfunctional Requirements: Include a list of nonfunctional requirements that outline expected performance criteria, such as reliability, scalability, and security of your system.
- Testing Plan and Validation: Describe the testing process, such as test cases and specifications.
Solution Planning
Once your project requirements have been clarified, it's time to give them to your development team for evaluation and solution planning. They should follow roughly these steps for planning solutions: Before beginning work on the solution plan, it is crucial to conduct a feasibility study to ascertain its technical, economic, and operational feasibility. A technical team should evaluate any constraints or risks related to your project before considering the scope and requirements.
- Determine an AI Approach: After conducting a feasibility analysis, teams must choose an approach for AI usage that will best help achieve their project's goals. They may opt for supervised or unsupervised learning, reinforcement learning, or some combination thereof.
- Selecting the Technology Stack: Under project constraints and requirements, as well as the AI approach chosen, a team must select an appropriate technology stack. This may consist of one or more AI models as well as cloud platforms, data analysis tools, and development platforms.
- Design of Architecture: When developing an AI architecture for their project, teams should collaborate to design it. They must identify all models, frameworks, algorithms, infrastructure tools, and infrastructure necessary. Furthermore, their design must take into account project constraints as well as technology stack requirements.
Create the Solution Plan. Your team should draft a comprehensive project implementation plan. It should include information on AI approaches, tech stacks, requirements for development, and an evaluation strategy - which could later serve as the basis for creating data strategies.
Also Read: All About the History of Artificial Intelligence (AI)
Data planning
Now that you have a solution, the next step should be forming the data plan. This work could be performed within your team that developed it or delegated to an independent data team. Your data plan should outline the scale and sources required to reach the accuracy level you require.
Consider where your data will come from - scraping data from publicly accessible websites or internal sources requires a license, so be aware of any possible licensing requirements before beginning. Your options might include:
- Past Data: AI capabilities can often be built onto existing products, meaning you could already have data collected by them that needs cleaning up and organizing for machine learning purposes.
- Manual Data Collection: Hiring data labelers to label datasets manually can be a time-consuming and costly process.
- Automated Data Collection: This involves using automated tools and scripts to gather information from websites or other sources. While automated collection may be cheaper, its quality may suffer.
- Purchased datasets: An efficient and economical approach Purchasing datasets through third-party providers is an efficient and cost-effective way to gather large volumes of data quickly. However, additional work may be needed to identify inaccurate or unnecessary details.
- Generic Models: Generative models are artificial intelligence algorithms that can learn to interpret an existing dataset and then produce new data using what has been learned - with that data potentially contributing to enhancing an AI project's dataset.
- Preprocessing: may vary depending on your final solution and data type, as it involves converting and cleaning raw data so it can be utilized by an AI solution - doing this reduces noise while increasing the accuracy of models.
Implementation
Implementation is the act of turning a solution into reality. Teams involved in AI projects should follow these key steps when undertaking implementation:
- Accumulate Data: Gather all the necessary data for your project from sources listed in your data plan, collecting enough for an adequate level of accuracy.
- Cleanse Data: Remove all irrelevant or redundant information to make the data suitable for the project, making it essential.
- Build Your AI Model: If you are developing an artificial intelligence (AI) model, use data collected to train AI models so they can identify patterns.
- Develop Models: This involves selecting and optimizing parameters and hyperparameters to ensure the model runs as expected, producing desired results.
- Validate models: While more testing and evaluation work will take place later in this step, validating models using real-world data to ensure accuracy and reliability will also play a part in iterative validity work. Modify real-time to enhance its performance.
- Integrate components: It is essential that all the parts of a project, such as data pipelines, are correctly connected for it to function efficiently.
Testing And Evaluation
Testing should continue following implementation to ensure models operate efficiently, within time limits, and meet accuracy expectations. AI systems can be tested using traditional black-box and white-box testing methods and more specialized ones such as load testing or simulation testing.
Deployment
An AI project deployment is one of the critical steps of AI project management, enabling internal users and customers access to your software. Deployment varies depending on factors like its type, environment of use, and required infrastructure. However, project leaders should always keep these aspects in mind when deploying tools:
Monitoring and Maintenance
AI systems, like any software application, require periodic monitoring and maintenance to continue providing value to their users. A project manager can use original deliverables and objectives as measures of long-term performance evaluation; product teams should systematically perform such checks.
Want More Information About Our Services? Talk to Our Consultants!
The Conclusion Of The Article Is:
Management of an AI project is no simple task. For successful AI implementation, organizations require not only technical know-how but also an influential data culture to help accelerate adoption.