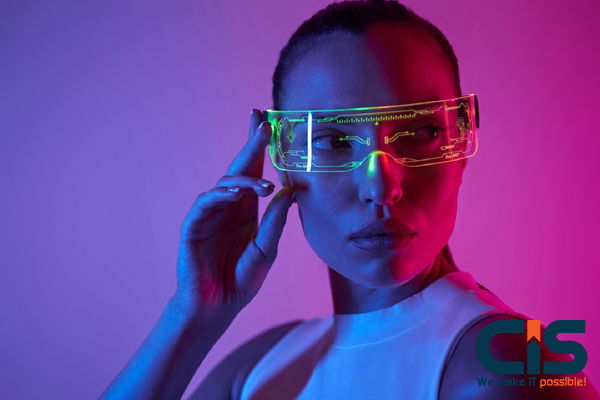
The fundamental principles of AI are similar to how humans constantly change and learn. Machine intelligence technologies will advance due to human intelligence, creativity, knowledge, experience, and innovation.
This is a standard definition that modern AI-powered systems such as virtual assistants would have to meet to demonstrate 'narrow AI.' It means they can use their training to perform restricted tasks such as speech recognition and computer vision. AI systems typically exhibit at least one of the following human intelligence behaviors: learning, reasoning, and problem-solving. Knowledge representation, motion, and manipulation are also common codes.
What are the Various Types of AI?
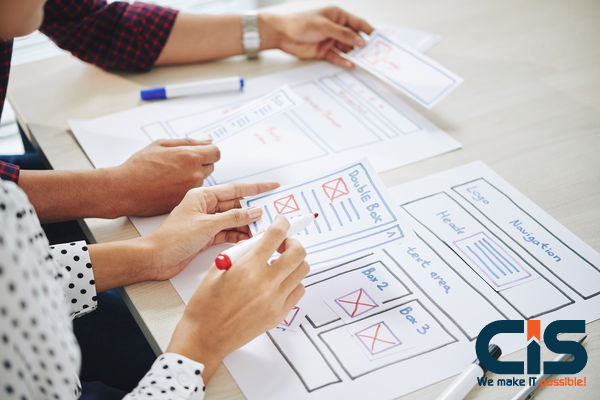
Artificial intelligence can be divided into two types at a very high level:
NarrowAI
Narrow AI refers to what you see in computers today. It is an intelligent system that has been trained or learned how to perform specific tasks without being programmed.
This type of machine intelligence software can be seen in Siri's speech and language recognition on the Apple iPhone and in self-driving car vision-recognition systems. Or in recommendation engines that suggest products based on past purchases. Unlike humans, these systems cannot learn or teach themselves how to perform a specific task. This is why they are called narrow artificial intelligence.
General AI
General AI is very distinct. It is the type of intelligent being found in humans. It is a flexible intelligence that can learn to do vastly different tasks. This includes everything from cutting hair, creating spreadsheets, and reasoning about many topics.
This is the AI you see in movies like Skynet in The Terminator or HAL 2001. However, it has yet to exist, and AI experts are divided on when it will be a reality.
What is Narrow AI Capable of?
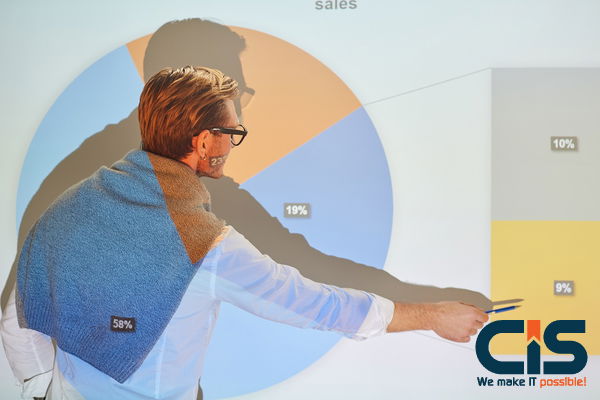
There are many emerging applications for narrow AI:
- Interpreting video feeds taken by drones for visually inspecting infrastructure, such as oil pipelines.
- Organizing your personal and professional calendars.
- Simple customer service queries can be answered.
- Coordination with other intelligent systems to complete tasks such as booking a hotel at the right time and place.
- Radiologists can spot potential tumors on X-rays.
- Online flagging of inappropriate content and detecting wear and tear in elevators using data gathered from IoT devices.
- The ability to create a 3D model from satellite imagery.
These learning systems are constantly being used in new ways. Nvidia, a graphic card manufacturer, recently introduced Maxine. This AI-based system allows people to make high-quality video calls almost regardless of their internet speed. This system decreases bandwidth by transmitting only a portion of the video stream over the internet. Instead, it animates a few static images of the caller to recreate the facial expressions and movements of the caller in real-time. It is virtually indistinguishable from the video.
However, these systems still have a lot of potential, and sometimes the technology's ambitions outweigh reality. Self-driving cars are an example of this. AI-powered systems like computer vision power these vehicles. Tesla, an electric car company, is not on track with CEO Elon Musk's timeline for the Autopilot system to be upgraded to "full-self-driving." This was a result of the system's limited assisted-driving capabilities. The Full Self-Driving option was only recently available to a few experienced drivers in a beta program.
What is General AI Capable of?
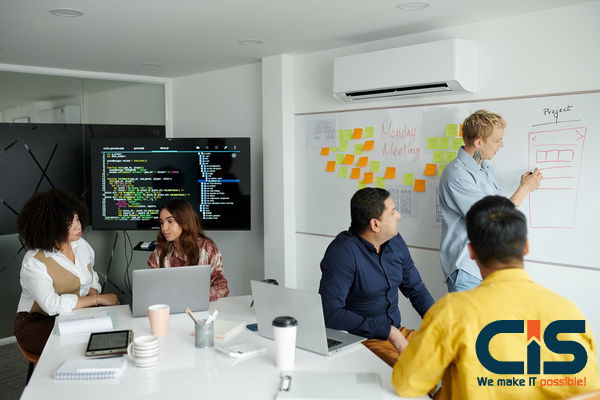
It found that 50% of those surveyed believed that Artificial General Intelligence would be developed by 2050 and rise to 90% by 2075. They predicted that "superintelligence" - defined by Bostrom as "any intellect that significantly exceeds the cognitive performance of human beings in virtually all domains and interests"- would be developed within 30 years of attaining AGI.
Recent assessments by AI experts have been more cautious. Given the skepticism of AI pioneers and the distinct nature of modern narrow AI systems, there is no reason to fear that general artificial intelligence solutions will soon disrupt society.
What is Machine Learning?
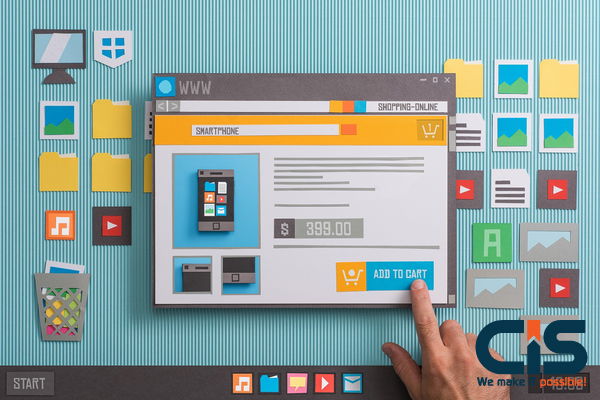
Machine learning is the subset of AI responsible for the most recent achievements. Machine learning is the most common form of AI.
Machine learning is currently enjoying a revival. Simply put, it's when a computer system learns to perform a task instead of being programmed. Arthur Samuel, who was a pioneer in the field and the inventor of the Samuel Checkers-playing Program, coined the term machine learning in 1959.
These systems receive huge amounts of data to help them learn. It is important to have a dataset that is both large and quality for a system to complete its task correctly. If you are building a machine learning system to predict house prices, your training data should include more information than the property size. It should also include other important factors like the number of bedrooms and the size of the backyard.
What is a Neural Network?
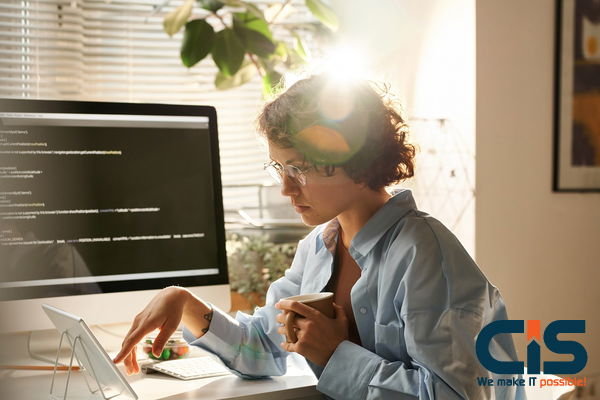
Neural networks are the key to machine-learning success. These mathematical models can be modified to produce different outputs. A neural network receives data that teaches it what to output when presented with specific data. The network may be given greyscale images of numbers between zero to nine, along with a string of binary digits (zeroes and ones) that indicates which number is in each greyscale picture. The network would then be trained and adjusted to classify each image's number accurately.
The brain's connections are the basis of the structure and function of neural networks. Neural networks are composed of layers of algorithms that feed data into one another. You can train them to perform specific tasks by changing the data's importance as it passes through these layers.
The weights attached to data when it passes between layers of the neural network will be changed until the output is close to the desired result. The network will then " learn" how to perform a specific task. This output could range from accurately labeling fruit in an image to predicting when an elevator will fail based on sensor data.
Deep learning is a subset of machine learning. This involves expanding neural networks into large networks with many layers of size and is trained with huge amounts of data. These deep neural networks are responsible for the recent leap in computer technology's ability to perform speech recognition and computer vision tasks.
There are many types of neural networks, each with strengths and weaknesses. Recurrent neural networks (RNNs) are an ideal type of neural network that is well-suited for Natural Language Processing (NLP), which involves understanding text meaning and speech recognition. Convolutional neural nets, on the other hand, have their roots in image recognition. They can be used as widely as NLP and recommender systems.
Researchers are refining a better form of deep neural networks called long-term memory (or LSTM) -- a type of RNN architecture used for tasks like NLP or stock market predictions -- which allows it to operate quickly enough to be used in online systems such as Google Translate.
What Other Types of AI are There?
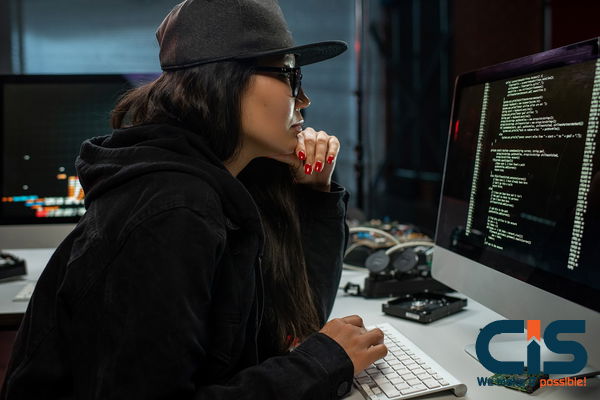
Evolutionary computation is another area of AI research. It draws inspiration from Darwin's theory of natural selection. In order to find the best solution to a problem, genetic algorithms are subject to random mutations and combinations of generations.
This method has been used to design AI models. AI can be used to build AI. Neuroevolution is the use of evolutionary algorithms for optimizing neural networks. This could be useful in designing efficient AI systems as intelligent systems become more common, especially as demand for data scientists is often higher than supply. Uber AI Labs published papers about using genetic algorithms to train deep neural networks for reinforcement learning.
Expert systems are computers that are programmed with rules to allow them to make a series of decisions based on a large number of inputs. This allows the machine to imitate human experts in a particular domain. One example of a knowledge-based system is an autopilot system that pilots a plane.
What are the Factors Driving the Revival of AI?
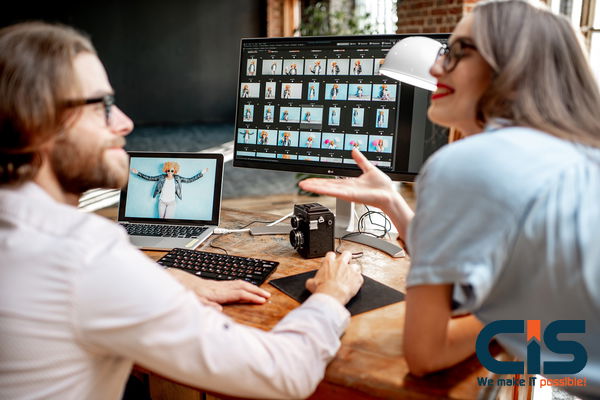
As we have seen, the most significant breakthroughs in AI research in recent decades have been machine learning and deep learning. This is partly due to the ease of accessing data and an explosion of parallel computing power. During this time, using clusters (GPUs) to train machine-learning systems has become more common.
These clusters can train machine-learning models much more efficiently than traditional systems. They can also be accessed as cloud services via the internet. The major tech companies, such as Google, Microsoft, Tesla, and Tesla, have shifted to using specialized chips that can both run and train machine-learning models.
Google's Tensor Processing unit (TPU) is an example of such a custom chip. The latest version accelerates the speed at which machine-learning models created using Google's TensorFlow library can infer data and the speed at which they are trained.
These chips are used for training DeepMind, Google Brain, and models underpinning Google Translate. They also provide models that enable image recognition in Google Photos. These chips were unveiled at Google I/O 2018 in May 2018. They have been packaged into pods, which can perform over 100 million trillion floating-point operations per second (100 petaflops).
Google has been able to enhance its services by continuing TPU upgrades. For instance, Google Translate's machine-learning models have been halved in time.
Want More Information About Our Services? Talk to Our Consultants!
What are the Components of Machine Learning?
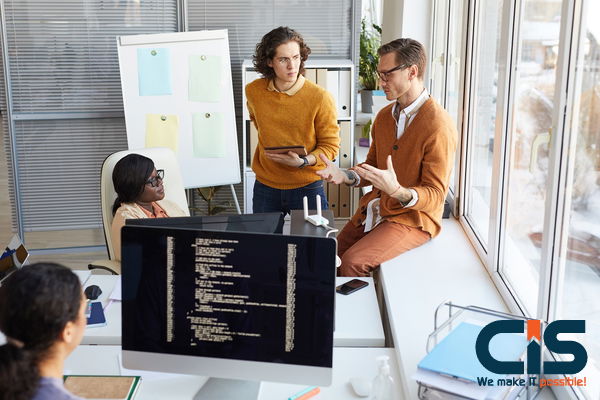
As a subset of AI, machine learning is mentioned above. It can be divided into two main categories: unsupervised and supervised learning.
Supervised Learning
One common method of teaching AI systems is to train them by using labeled examples. Machine-learning systems receive huge amounts of data annotated to highlight key features. These could be photos that are labeled to show if they include a dog or sentences with footnotes to indicate if the word "bass" is related to music or fish. After being trained, the system can apply these labels to any new data, such as a photo of a dog that has just been uploaded.
Supervised learning is the process of teaching a machine through an example. These examples are often labeled by online workers who work through platforms such as Amazon Mechanical Turk.
These systems require large amounts of data. Some systems may need to search millions of examples to understand how to perform a task efficiently. However, this is becoming more possible with the advent of big data and widespread data mining. The size of training datasets is increasing. Google's Open Images Dataset contains about 9 million images. YouTube-8M links to 7 million labeled videos.
This database, one of the first to be created, contains more than 14,000,000 categorized images. It was compiled over two years by almost 50 000 people, most of whom were recruited via Amazon Mechanical Turk. They checked, sorted, and labeled nearly one billion candidate images.
Access to massive labeled datasets might be less important than having access to high amounts of computing power over the long term. Generative Adversarial Networks have been used in machine learning systems for years. They only require small amounts of labeled data and a lot of unlabelled data. This, as the name implies, requires less manual labor to prepare.
This could lead to semi-supervised learning being used more often. Systems can be trained to perform tasks with much less labeled information than supervised learning systems.
Unsupervised Learning
Unsupervised learning, on the other hand, uses a different approach. It is based on algorithms that attempt to find patterns in data and look for commonalities that can be used to classify that data. One example is clustering fruits of similar weight or cars with similar engines. The algorithm doesn't pick specific data types in advance; it just looks for data similar enough to group them, such as Google News, which groups stories on similar topics daily.
Reinforcement Learning
Reinforcement learning can be described as rewarding your pet for performing a trick. Reinforcement learning is a system that tries to maximize a reward based on its input data. It goes through a process of making mistakes until it achieves the best outcome.
Google DeepMind's Deep Q network, a form of reinforcement learning, has been proven to be the best in human performance in many classic video games. The system receives pixels from every game and calculates various information, such as the distance between objects.
The system also looks at each game's score to determine which action will maximize the score under different circumstances. For example, Breakout shows where the paddle should be moved to catch the ball.
This approach can also be used in robotics research, where reinforcement learning can be used to teach autonomous robots how to behave in real-world situations. Many AI-related technologies are nearing or have already reached the "peak of inflated expectations" in Gartner's Hype Cycle. The backlash-driven "trough of despair" lies in wait.
Which AI-Focused Firms are the Most Successful?
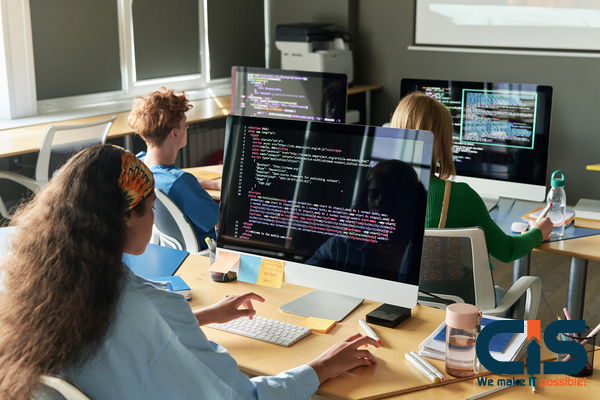
Each tech company strives to create machine-learning technology that can be used in-house or sold to the public through cloud services. AI plays an increasing role in modern software and services. Each makes headlines regularly for their groundbreaking AI research. However, Google and its DeepMind AI AlphaFold/AlphaGo systems have made the most impact on public awareness of AI.
What are AI Services Currently Available?
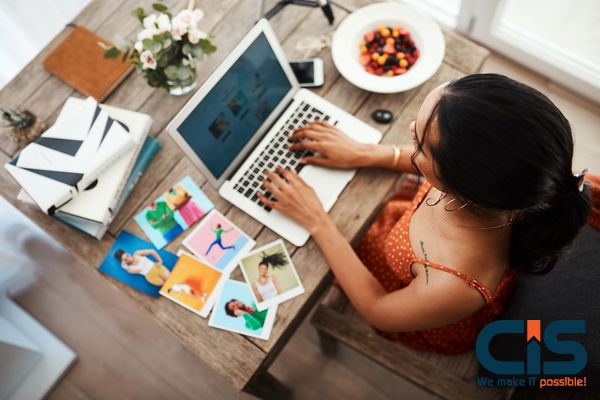
All major cloud platforms, including Amazon Web Services, Microsoft Azure, and Google Cloud Platform, offer access to GPU arrays to train and run machine-learning algorithms. Google is also preparing to allow users to use its Tensor Processing Units, custom chips that are optimized for running and training machine-learning models.
The cloud-based data storages, which can store the huge amount of data required to train machine-learning algorithms and services to transform the data and display it clearly, are all available to the big three.
Cloud platforms make it easier to create custom machine-learning models. Google offers a Cloud AutoML service, which automates the creation of AI models. The drag-and-drop service creates custom image recognition models and does not require any machine-learning knowledge.
Machine-learning services that are cloud-based are always evolving. Amazon offers many AWS services that can be used to speed up the process of creating machine-learning models. Recently, Amazon SageMaker Clarify was launched to assist organizations in removing biases or imbalances from training data that could cause skewed predictions.
Microsoft Azure is the best choice for firms who wish to avoid creating their machine learning algorithms but want to use AI-powered on-demand services such as voice, vision, and language recognition. It closely follows Google Cloud Platform and AWS. IBM is trying to sell sector-specific AI services to complement its on-demand offerings. It has invested $2bn to buy The Weather Channel, allowing it to access a wealth of data.
Which tech company is leading the AI race?
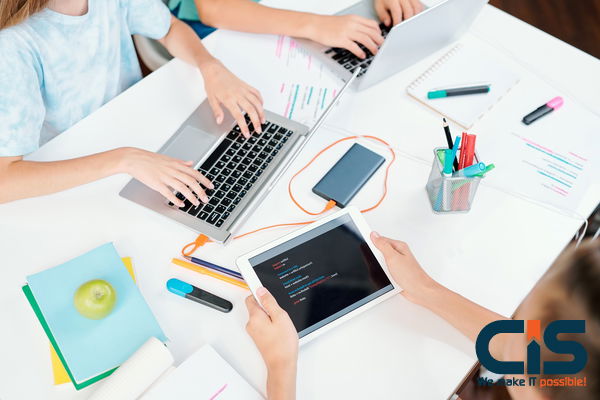
Each tech company, including Facebook, uses AI internally to drive various public services. These include offering recommendations, serving search results, providing recommendations, recognizing people in photos, and on-demand translation.
One of the most prominent manifestations of the AI war is the rise of virtual assistants such as Apple's Siri, Amazon's Alexa, Google Assistant, and Microsoft Cortana. These assistants rely heavily on natural language processing and voice recognition. They require a large corpus of information to answer questions. This is why a lot of tech goes into their development.
While Siri was Apple's first assistant, Amazon and Google have since surpassed Apple in AI. Google Assistant can answer any query, while Amazon's Alexa has a vast array of skills that third-party developers have added.
These assistants have been improving over time and are better equipped to answer the kinds of questions that people ask them in their regular conversations. Google Assistant offers Continued Conversation. This allows users to ask questions that follow up on their initial query. Then, follow that with 'What about tomorrow.' The system also understands that the question in the next step relates to the weather.
These assistants can handle more than speech. The latest version of Google Lens can convert text into images and allows you to search for furniture or clothes using photos.
Cortana is not a feature of Windows 10. Amazon's Alexa is now free for Windows 10 PCs. Microsoft also revamped Cortana's role in Windows 10 to make it more productive, such as managing users' schedules, than the more consumer-focused features of other assistants like playing music.
How do we get started with AI?
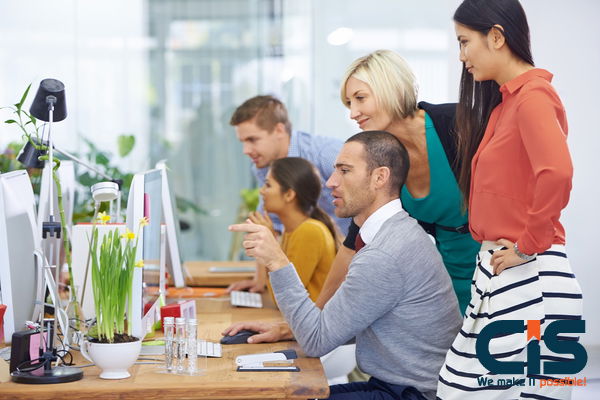
You could purchase an Nvidia GeForce GTX 2060 GPU or a faster Nvidia GPU for your computer and then start training a machine learning model. However, the best way to experiment with AI-related services is through the cloud.
Each of the top tech companies offers a variety of AI services. These include the infrastructure to create and train your machine learning models, as well as web services that let you access AI-powered tools like speech, language, and sentiment recognition.
Various Sectors Adopting AI
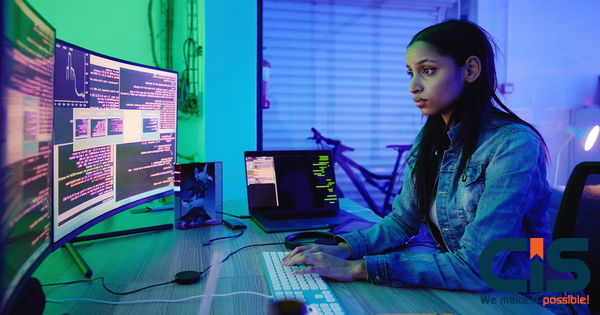
A business can be small to benefit from AI. However, it doesn't necessarily have to offer clients superior solutions to reap AI's benefits. AI can help small and medium-sized businesses better anticipate client product needs and provide faster solutions.
AI: The Outcomes
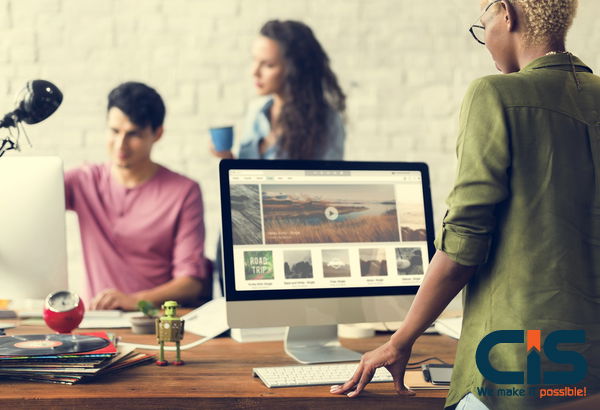
There are many discussions about the effect of AI on companies' ability, strategy and willingness to move to it. The goal of the transition will decide if it happens. Companies should not be transiting to replace labor but to improve human capabilities. Artificial Intelligence Service won't lead to unemployment. Instead, it will redefine the job market and the organizational structure. To add new jobs and create new roles for intelligent technologies, businesses must restructure their work.
Research
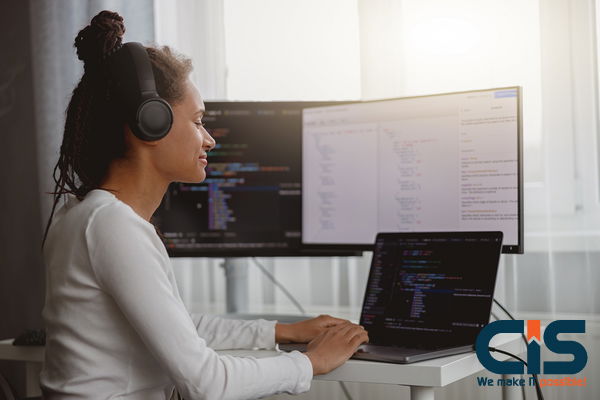
Both Forbes and Gartner published studies showing how AI can be used to create autonomous vehicles. They can interact naturally with humans and the environment. Gartner defines "augmented intelligence" as machine learning that alters how analytics create articles. This will enable businesses to get the best out of their employees, not just information scientists and analysts.
The study also discusses the increasing demand for easy-to-use tools more accessible to programmers than information scientists. This is also known as "expansion in bright places," which refers to a physical or digital environment that allows people and technology-enabled system interaction to create more open, connected, and coordinated intelligent ecosystems.
AI is not just a buzzword. The rapid rise in AI development and the fact that individuals and businesses are more adept at it show that it's no longer a trendy term. It is important to understand AI and what we can expect fully.
Want More Information About Our Services? Talk to Our Consultants!
Conclusion
Every day, artificial intelligence improves the effectiveness of our lives. Several applications and services that assist us in performing routine tasks, including contacting friends, utilizing email, or using ride-sharing services, are powered by AI.
Knowing that most of us use AI on a daily basis and have for many years may give you some peace of mind if you have concerns about its use.