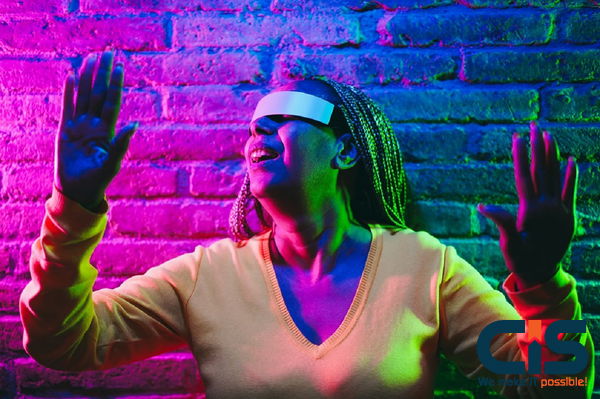
Ever wondered how businesses create and train AI and machine learning models without access to experts? Automated Machine Learning (AutoML) holds the answer. In order for businesses to fully take advantage of AI/ML technologies, autoML facilitates developing and optimizing machine learning models quickly and efficiently. Here in this blog, we delve into AutoML, exploring its advantages, drawbacks, techniques, and much more. Keep reading for an insightful discussion.
AutoML - The Future Of Artificial Intelligence
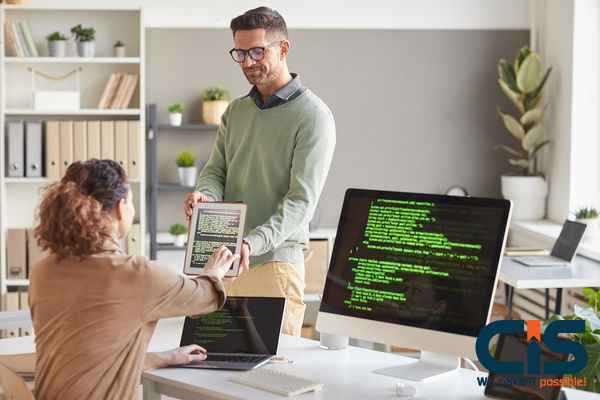
What is AutoML? AutoML is an innovative advancement in AI and machine learning. This technique involves training machine learning models automatically through automating the selection and tuning processes - from data preprocessing through model selection for any given task. Hyperparameter Tuning/Model Selection tools take care of this for you - providing users without prior machine learning knowledge the chance to train high-performing models quickly with minimum effort needed - be they business owners, researchers or data scientists alike.
Popular platforms offering AutoML services include Google Cloud AutoML, H2O.ai and DataRobot, among many more. Popular AutoML platforms include Google Cloud AutoML, H2O.ai, and DataRobot, among many more. AutoML provides explainable AI to increase the interpretability of models.
This enables data scientists to comprehend better how predictions made by AutoML models work - particularly helpful in healthcare, finance and autonomous systems environments where understanding how the model makes predictions is essential to providing correct forecasting of outcomes and bias detection and prevention of false positive predictions. AutoML's use can range from disease diagnosis using medical images through to fraud detection for finance sectors as well as product recommendations in retail outlets as well as self driving car transportation services.
Why Does AutoML Matter?
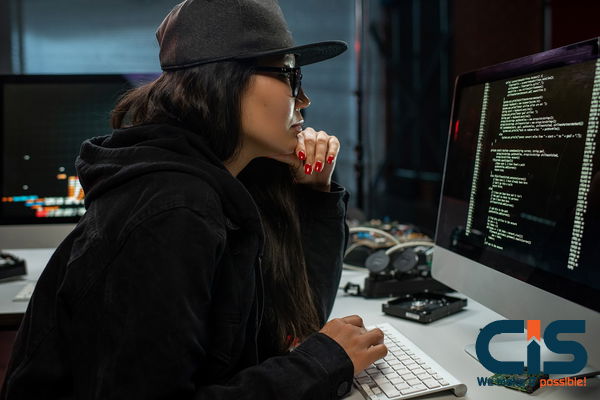
AutoML makes machine-learning models accessible to non-experts by simplifying the development of machine-learning models. This makes the technology even more valuable, given its potential use cases from image recognition and natural language processing, but developing machine learning models requires knowledge in algorithms, statistics and programming that may not come easily to them all - creating barriers for many who recognize valuable problems that could benefit from machine learning but lack the technical skill or domain expertise to develop models themselves.
AutoML offers an effective solution by automating the building of machine learning models, making it simpler and faster for anyone to jumpstart machine learning. It democratizes machine learning and makes it more widely accessible - providing significant potential advantages such as driving innovation or helping businesses address complex problems more efficiently.
How Does AutoML Operate?
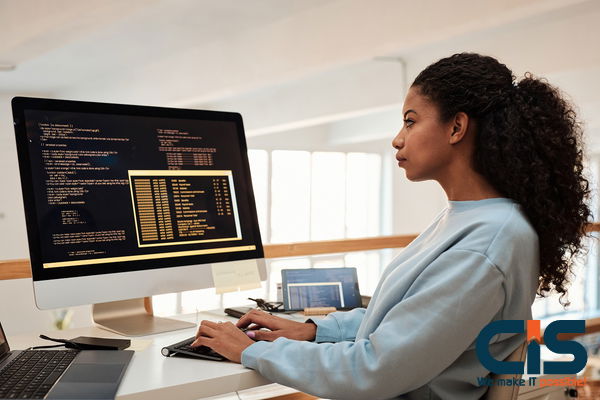
The typical process involved with AutoML involves several steps. These may include:
- AutoML systems rely on input provided by users, usually as large datasets that have been preprocessed and cleaned before being ready to train machine learning models.
- AutoML processes the data by performing tasks such as feature engineering and normalization to better prepare it for training machine learning models, potentially increasing accuracy while decreasing training times.
- AutoML works by training multiple machine learning models on preprocessed data using various algorithms and hyperparameters - this allows it to find which model performs best with regard to that dataset.
- AutoML system evaluates and selects one model that excels from among all those trained, to become its output.
- Users can then utilize their trained model to predict or act upon new, unseen data sets - typically through publishing it as a web service that other applications or users can access directly.
Outputs
Supervised machine learning models produce outputs by making predictions based on input data. Through training, this model learns to connect specific inputs to correct outcomes and then uses that knowledge to make future predictions based on similar sets.
Quality Input
Quality input data is vitally important to machine learning models because its quality has a direct bearing on the accuracy and performance of these algorithms. Poor-quality input could cause incorrect or misleading associations between inputs and outputs being learned by the model, and its predictions.
Hyperparameters
Hyperparameters are settings or parameters that determine the behavior of models, such as learning rate, number of hidden layers in neural networks or regularization strength. They should typically be set prior to training the model as their effects on its performance may have an immense effect. Unfortunately, optimizing hyperparameters is both a time-consuming and complex process that necessitates expertise and experience from professionals with expertise.
AutoML systems utilize machine learning models to automatically optimize hyperparameters by searching for the optimal combination of hyperparameters for any given machine learning model. This process works by training different combinations of hyperparameters on data samples before assessing each combination's effectiveness in training the model to analyze that particular data set.
Who Could Benefit From Automated Machine Learning?
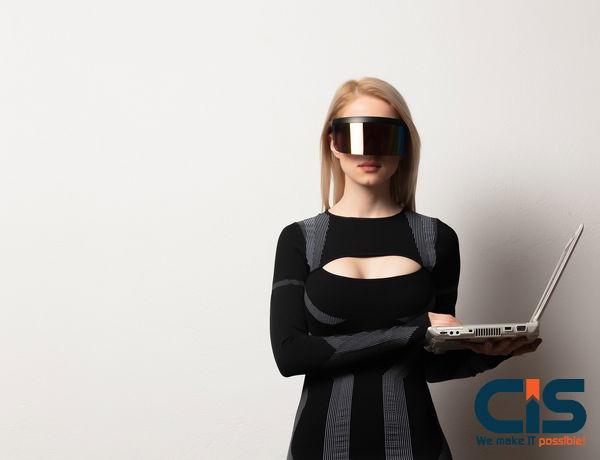
Applying machine learning algorithms to real-world problems takes both time and expertise from data scientists, with manual processes carried out by machine learning experts being required in many instances; novice users often struggle to develop models suitable for deployment - this is where Automated machine Learning (AutoML) comes into its own as it offers solutions.
Automated machine learning provides the ideal solution to companies on tight budgets or lacking machine learning expertise who want to save time with automated pipelines and faster results analysis and visualization - yet this functionality doesn't reduce demand on machine learning engineers or data scientists - it just opens new opportunities. However, interpretation and visualization still require considerable expertise from these individuals.
AutoML Vs Manual ML
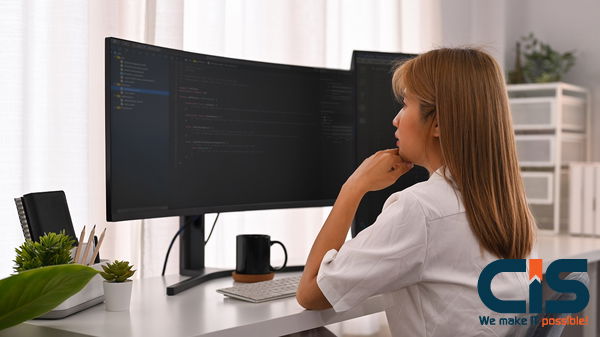
Automated Machine Learning (AutoML) and Manual Machine Learning (ManualML) offer two distinct approaches for building machine learning models, respectively. Let's take a look at their differences:
Automation Level
- AutoML: AutoML is an approach that utilizes automated processes for various stages of the machine learning pipeline, from data preprocessing, feature engineering and selection through hyperparameter tuning and evaluation of models. Various platforms and tools exist that utilize AutoML techniques in order to simplify model building for users of different skill levels.
- Manual Machine Learning: Engineers and data scientists using Manual ML must perform each step in the machine learning pipeline manually, including feature selection, model creation and hyperparameter tuning. This approach requires having an in-depth knowledge of machine learning algorithms' strengths and weaknesses as well as an awareness of any particular issue being solved with their solutions.
Experience
- AutoML: AutoML was created to lower the barrier of entry for machine learning and make developing effective models easier for newcomers to this field. However, advanced practitioners also find AutoML useful, as it allows them to explore various models and settings quickly and effectively.
- Manual Machine Learning: Manual machine learning requires an in-depth knowledge of machine learning concepts, techniques and algorithms; therefore data scientists and engineers need to have extensive expertise in this area so as to select appropriate algorithms, tune hyperparameters as necessary and build the optimal model possible.
Customization And Management Control Systems
- AutoML: It platforms typically provide less control and customization options compared to manual ML, as their focus lies more on automation and ease of use than providing advanced users with enough customization options for complex tasks. Advanced users may find AutoML's lack of options insufficient.
- Manual Machine Learning: Manual machine learning provides practitioners with control of every aspect of the modeling process, giving them complete freedom in creating highly tailored models tailored specifically to meet their own requirements and meet performance targets. While manual ML may lead to higher performance, results require higher expertise.
Save Time And Resources
- AutoML: It saves both time and resources by automating time-intensive aspects of machine learning pipeline development. This enables users to develop models quickly in fast-paced environments or when working with large datasets - an advantage especially beneficial when working in large-scale environments or dealing with massive datasets.
- Manual Machine Learning: Manual machine learning may take more time and resources, as practitioners need to perform each step manually. However, with skilled practitioners applying their models specifically to each problem area at hand, this approach could potentially result in improved performance.
Read More: 3 Forms of AI - What's the Cost, Gain, and Impact?
AutoML Techniques: An Extensive Overview
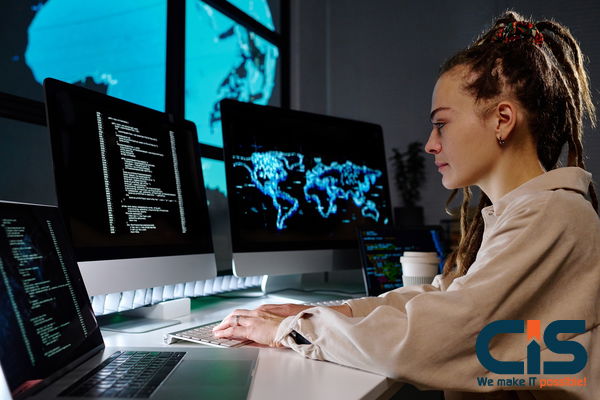
AutoML automates machine learning for real-world problems by performing tasks such as algorithm selection, hyperparameter optimization and feature engineering. Many different approaches exist for tackling various aspects of these complex issues - some popular examples can be seen below.
- Neural Architecture Search (NAS): This approach utilizes a search algorithm to automatically discover the optimal neural network architecture for any given task and dataset.
- Bayesian Optimization: Bayesian optimization utilizes a probabilistic model to guide its search for optimal hyperparameter values for any given model and dataset.
- Evolutionary Algorithms: This technique utilizes evolutionary algorithms such as genetic or particle swarm optimization in order to find an ideal set of model hyperparameters.
- Gradient-Based Methods: These approaches make use of gradient optimization techniques such as gradient descent to optimize model hyperparameters.
- Transfer Learning: Transfer learning uses an existing model trained on similar tasks or datasets as the starting point and fine-tunes it on target tasks and datasets to provide optimal solutions.
- Ensemble Methods: An ensemble approach uses multiple models to form one robust and accurate final model.
- Multi-Modal Methods: These approaches utilize data modalities from images, texts and audio streams in order to train models effectively and increase performance.
- Meta-Learning: Meta-learning utilizes a model to understand how best to interpret data for analysis, improving the efficiency of model selection.
- One Or Few-Shot Learning: With this method, new classes can be recognized from just a single or few examples.
AutoML: Exploring Its Drawbacks
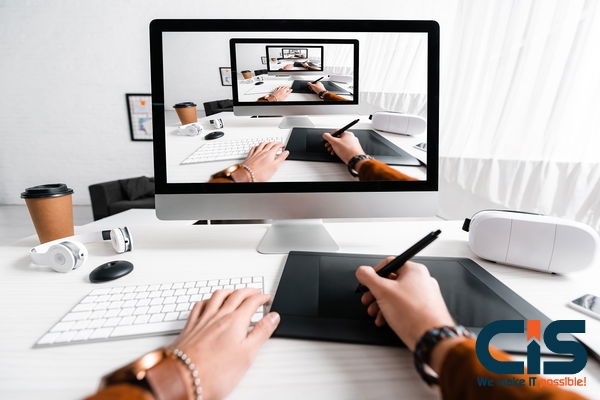
AutoML has quickly become one of the go-to tools for data scientists and analysts, but its implementation still poses certain limitations. Here are the drawbacks of AutoML:
- Limitations In Model Selection And Hyperparameter Tuning Processes: AutoML methods operate using predetermined algorithms and settings; as a result, users have little direct influence over what model will emerge as their final choice.
- AutoML Models: Often lack interpretability, making it hard for individuals to grasp exactly how the model makes predictions.
- Costlier Than Manual Model Design/Training: AutoML tools and infrastructure may incur greater expenses to implement and keep up to date.
- Difficulties Associated With Including Domain-Specific Knowledge Into AutoML Models: Since AutoML relies on predefined algorithms and data sets for its modeling techniques, it may not always provide effective means of including domain knowledge into its model.
- AutoML Methods: It could perform poorly when presented with data sets that differ significantly from their training data set due to edge cases or unusual distributions.
- AutoML Methods May Not Provide Adequate Support: For certain models or tasks, thus limiting their utility in these regards. AutoML methods rely heavily on large amounts of labeled data in order to train their models effectively.
- AutoML Methods May Struggle To Handle Data: With missing values or errors effectively, leading them to perform subpar. AutoML methods may make it hard to interpret their predictions and decisions, creating difficulties that make understanding how a model makes its predictions difficult for certain applications and industries.
- Overfitting: AutoML methods may lead to overfitting on training data if not closely managed, leading to subpar results when applied to unseen data sets.
AutoML's Advantages For Artificial Intelligence
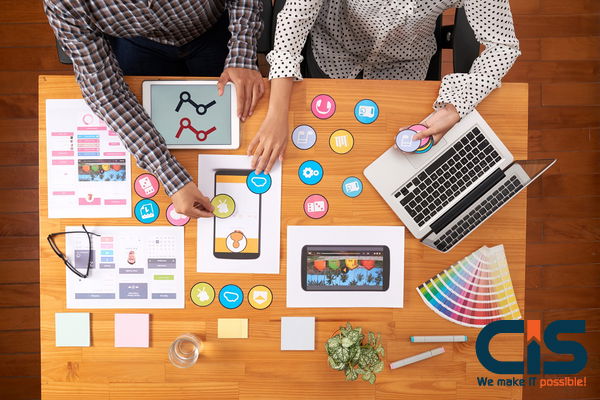
AutoML makes machine learning simpler and brings several advantages of AutoML, some of which can be seen below:
- Time-Saving: Automating model selection and hyperparameter tuning processes can save data scientists and machine learning engineers a considerable amount of time, as this step often falls under their purview.
- Accessibility: AutoML makes machine learning accessible, enabling even non-expert users to train high-performing models successfully.
- AutoML Methods: Often produce better model architectures and hyperparameter settings than manual ones, leading to greater model performance.
- AutoML Excels: At handling large volumes of data and finding models with more features that suit them all.
- Scalability: AutoML's capabilities of scaling to large datasets and complex models make it ideal for use in big data and high-performance computing environments.
- AutoML's Versatility: Extends across industries and applications such as healthcare, finance, retail and transportation.
- Cost-Effective: AutoML's long-term cost-effectiveness helps save resources and money by decreasing manual labor requirements and expertise needs.
- Reducing Human Error: Automating the model selection and hyperparameter tuning processes can significantly decrease human error while improving the reproducibility of results.
- Increased Efficiency: AutoML can be integrated with other tools and processes in order to increase data pipeline efficiency and thus save both time and resources.
- Handling Multiple Data Modalities: AutoML has the capability of handling different forms of data modalities like images, texts and audio for training models and improving performance.
Want More Information About Our Services? Talk to Our Consultants!
Conclusion
AutoML automates the machine-learning and Artificial Intelligence solutions model-building and tuning processes using algorithms rather than human expertise for search of optimal models and hyperparameters. As an added advantage, AutoML offers increased efficiency as it handles large data loads efficiently; making it ideal in situations when experienced machine learning practitioners are scarce - although AutoML may have its limitations too.
AutoML can be computationally intensive, and difficult to interpret the results of its automated search process, thus restricting its practical usage to data quality issues or availability of computational resources. As a result, this technology is typically employed within an industry setting to enhance productivity and model performance with images, speech samples, texts or any other form of data that requires processing.