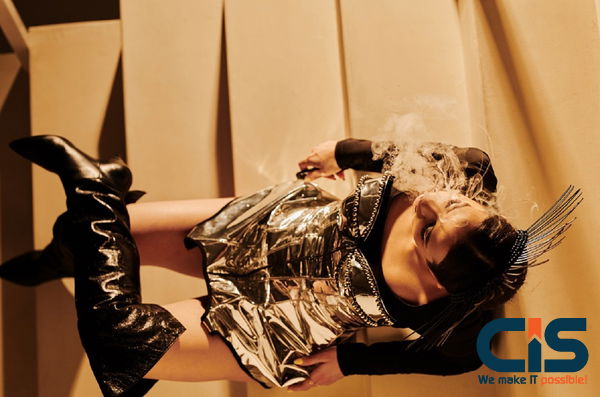
Today's machine learning market is rapidly democratizing, with solutions accessible to any business, regardless of the level of data science experience. Solutions from AWS and AWS Partner Network (APN) Partners can help you take advantage of the power of machine learning technology, regardless of whether you have a dedicated data science team or are brand-new to machine learning and data science.
This blog post describes in detail how, in comparison to earlier timescales, AWS and its Machine Learning Competency Partners use the cloud's elasticity, scalability, and dependability to accelerate the adoption of AWS Machine Learning Solutions for companies of all sizes. Regardless of your organization's level of data science expertise, you'll learn how AWS and AWS Partner Network (APN) Partners can help you get more out of machine learning.
You'll also learn how companies realize the benefits of machine learning for their particular use cases thanks to a combination of massive computing power, data lakes, security, analytics capabilities, and seamless integration with AWS environments.
How AWS Looks At Machine Learning
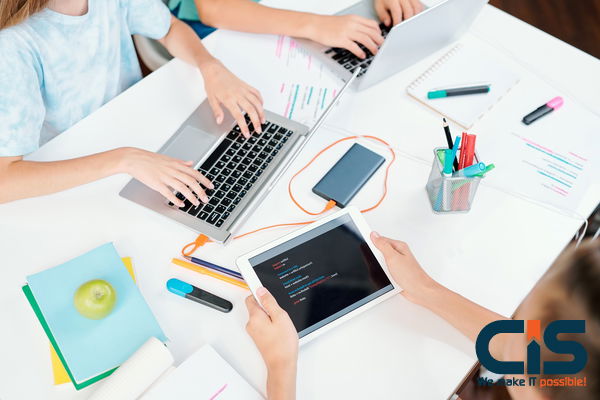
The term machine learning (ML) describes the application of learning algorithms to create a model of knowledge about the connections between already-existing data to forecast new data. Although the phrases are sometimes used synonymously, artificial intelligence (AI) and this term actually refer to different but related concepts.
Without explicit programming, artificial intelligence (AI) is the capacity to perceive, learn, reason, act, and adapt to the real environment. The goal of artificial intelligence (AI) is to design systems that enable computers to learn and make decisions without explicit human guidance. Machine learning (ML) is the process by which these capabilities are developed.
The third phrase that's frequently used while talking about machine learning is deep learning (DL). DL uses systems known as neural networks to simulate how the brain functions and learns, as opposed to using explicit mathematical procedures. When the answer is difficult to express, DL is a useful tool to have. For instance, it is very challenging to briefly describe how we distinguish a dog from all other objects and animals. But thanks to DL, we can use assessment and feedback to let the neural network educate itself on what a dog is just like a kid learns to recognize a dog.
Read More: Maximizing ROI: How to Design Effective Tech Solutions with AWS?
Advantages Of AWS For Machine Learning
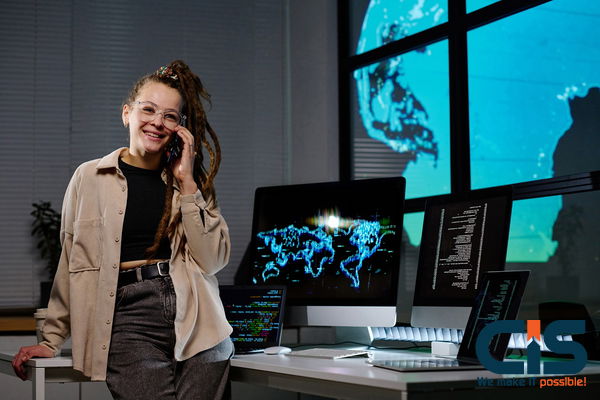
AWS provides a flexible, scalable, and cost-effective platform for building, training, and hosting machine learning and artificial intelligence applications.
Advantages of AWS include:
- Powerful computing resources, including the most powerful GPU-based instances in the cloud, make model training and inference fast and efficient.
- All popular frameworks are supported, including Torch, PyTorch, Chainer, TensorFlow, Microsoft Cognitive Toolkit, Caffe, Caffe2, Theano, and Apache MXNet and Gluon.
- Flexibility to deploy ML models in Amazon EC2 instances, Amazon Lambda, on FPGA (on F1 instance types), or even on an edge using AWS Greengrass.
- Deep machine learning knowledge from thousands of Amazon engineers who have created ML solutions for many different departments within the firm, including Amazon Go, Amazon Alexa, and the retail website Amazon.com.
- Security, encompassing a wide range of identity management, encryption, and access control features.
- Platform integrations, such as those for database and data lake technologies required to execute machine learning workloads.
- All-inclusive analytics services to support machine learning applications, such as batch, stream, and data warehousing, business intelligence, and data workflow orchestration.
Explore AWS Machine Learning Services
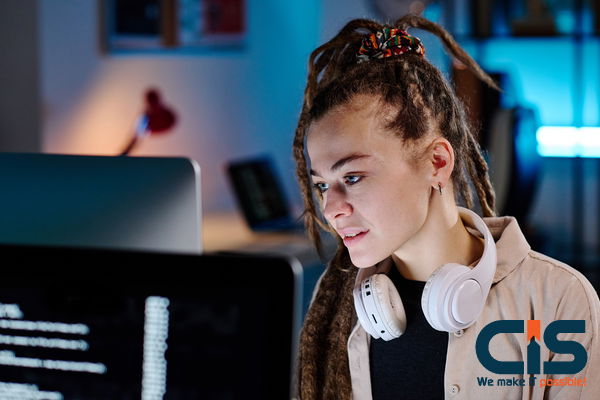
Using AWS for deployment enables businesses to develop and adapt more quickly. With AWS, users can store and examine data sets on a petabyte scale while only using the resources they really use and paying for what they use. With the help of AWS services, businesses may develop and implement cloud-based machine learning solutions that include:
- Prepare data sets so that machine learning algorithms can analyze them.
- Create, evaluate, implement, and enhance machine learning models.
- Provide end-user machine learning solutions tailored to particular use cases.
Amazon SageMaker- Build, train, and deploy machine learning models at scale
Amazon Rekognition- Deep learning-based image and video analysis
Amazon Transcribe- Automatic, grammatically correct transcription of speech
Amazon Polly- Generate realistic speech from text in a variety of languages
Amazon DeepLens- An entirely programmable video camera with pre-trained models, code, tutorials, and an aim to enhance deep learning capabilities.
Amazon Comprehend- Uncover connections and insights throughout the text
Amazon Lex- Integrate conversational chat and voice bots into your apps
Amazon Translate- Provides natural and fluid language translation.
Amazon Deep Learning AMIs- Ready-made settings for developing deep learning apps fast
The AWS Machine Learning Competency
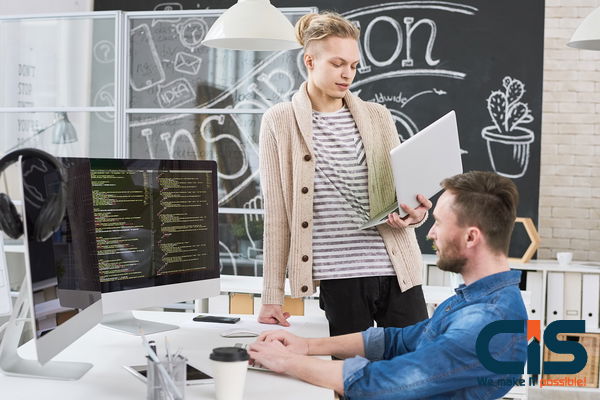
AWS Machine Learning Competency Partners offer SaaS-based capabilities that improve end applications with machine intelligence, as well as solutions that assist enterprises in overcoming their data difficulties and enabling machine learning and data science workflows.
AWS Machine Learning Competency admission is extremely competitive, and potential Partners must pass a demanding auditing procedure in order to be accepted into the program. These Partners have proven to have extensive knowledge of machine learning on AWS and the capacity to offer their company's solutions on the platform with ease.
Featured Partners increase the advantages of AWS by providing technological solutions that make cloud-based machine learning accessible to enterprises of all sizes.
Anodot- Automated time series analysis for anomaly detection
DataRobot- Automated machine learning platform
FigureEight- Human-in-the-loop automated data annotation
NarrativeScience- Natural Language Generation (NLG) for interpreting large data sets in context
Trifacta- Data wrangling (formatting and preparation) for use as training data for machine learning models
AWS Machine Learning Success Stories
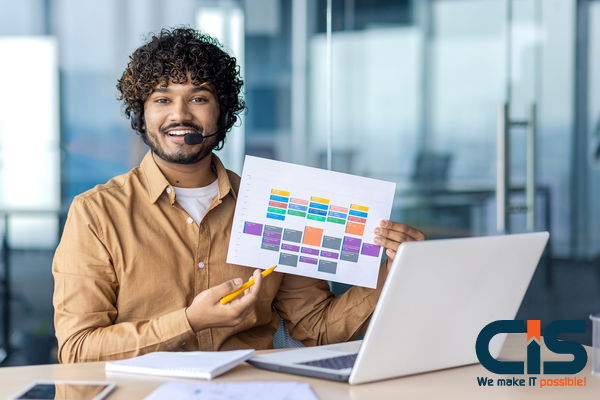
Real-world applications of AWS machine learning are demonstrated by the case studies that follow:
Case Study 1: Lyft
Automated Study Of Time Series To Discover Anomalies
Lyft is a prominent ride-sharing company with a market capitalization of over $11 billion. Unreported issues, such as delays in matching passengers with rides, can cause the business to lose money, clients, and market share. Given the vast volume of data collected by its mobile app usage, Lyft recognized the need for an automated method to identify significant deviations that might indicate more serious issues.
Lyft decided to use Anodot, an AWS-based platform. With the help of sophisticated machine-learning algorithms, Anodot's AI-powered analytics can spot any issues in real-time without requiring manual inspection of numerous dashboards, thereby overcoming the limits that come with human data analysis. Through time series analysis, Anodot automatically learns the normal behavior of the data. It then recognizes any deviations in real-time, groups and correlates various anomalies, and sends out alerts for noteworthy events.
Lyft can track a lot of KPIs using Anodot and AWS without setting up thresholds. Customers may detect problems in real-time and swiftly comprehend the extent and the underlying cause of the problems thanks to the simplicity of generating real-time alerts and the connections between abnormalities.
Case Study 2: Trupanion
AWS-Based Automated Machine Learning Platform
Trupanion, a Seattle-based company, was established in 2000 and offers comprehensive medical insurance plans for pet owners. Merely 1% of pet owners have insurance plans in the comparatively limited field of medical insurance for dogs and cats. The majority of veterinarians demand that pet owners pay the entire cost of their pet's care, which makes filing insurance claims a laborious and manual procedure.
In order to cut down on the amount of time and physical labor required to approve or reject insurance claims, Trupanion set out to develop an accurate predictive data model. The business saw DataRobot, an AWS-based machine learning platform, as a solution that could quickly ingest data and produce trainable algorithms for result prediction.
Trupanion reduced time-to-insight by a significant amount by building highly accurate predictive models more quickly with the help of AWS and DataRobot. In comparison to slower, more manual ways of claims processing, Trupanion's claims procedure has been truly optimized for both claims processors and policy owners, who now know whether a claim will be granted before leaving the veterinarian's office. This represents a considerable increase in customer service.
Case Study 3: Deloitte
Aws-Based Natural Language Generation (NLG) Platform
Over 84,000 people work for the sizable professional services company Deloitte US globally. Deloitte frequently deals with data overload having access to numerous, enormous data sets without having the time or means to examine them in order to make insightful recommendations thoroughly. The demands of Deloitte's clients, who frequently approach the firm with a desire to develop their businesses, add to the requirement for rapid data analysis.
Natural language generation (NLG) is a tool that Deloitte uses to assist in interpreting data meaningfully. Narrative Science is an AWS Machine Learning Competency Partner. Quill, an NLG platform from Narrative Science that is hosted on AWS, assists users in contextually and meaningfully interpreting big data volumes.
Deloitte uses Quill to automate internal manual analysis and reporting processes that are currently in place. Additionally, Quill aids in the company's growth and enhances client connection for a range of customers. In order to provide its clients with a complete end-to-end solution utilizing NLG, Deloitte has contributed its own industry experience as well as an extensive understanding of system and workflow integration. Deloitte can swiftly spin up and deploy secure NLG solutions to its clients thanks to AWS's cloud infrastructure.
The internal operations of Deloitte have already saved more than half a million dollars because of the company's usage of Narrative Science's NLG platform. As of this writing, three more NLG solutions will be internally implemented by Deloitte in the upcoming months.
Conclusion
The partnership between machine learning and Amazon Web Services (AWS) is transforming how businesses function in the rapidly evolving field of technology. By utilizing Amazon's twenty years of machine learning experience, AWS has been instrumental in the democratization of machine learning, making the field affordable and available to companies in a variety of industries.
The method used by AWS is transparent and demystifies the differences between AI, machine learning, and deep learning. It presents itself as an enabler, allowing businesses to apply machine learning for certain use cases independent of their experience with data science.
AWS is distinguished by its scalable, cost-effective, and adaptable platform that supports popular frameworks and provides strong computational resources. The real-world applications of AWS machine learning services, such as automated insurance claim processing, natural language generation, and anomaly detection, are exemplified by the triumphs of Lyft, Trupanion, and Deloitte.