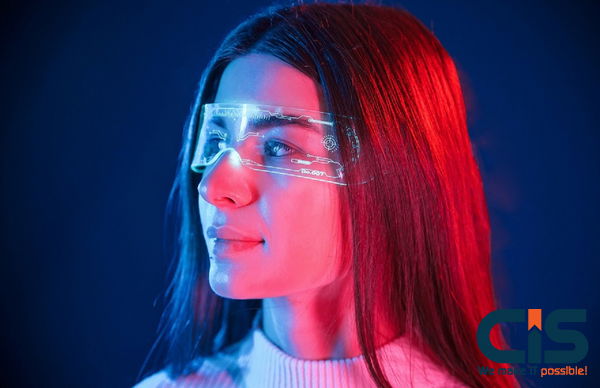
Big data was initially associated with three concepts: volume and variety. Big data analytics presents unique challenges when it comes to sampling, with limited opportunities for observation or sampling. Veracity refers to data quality or insight. If insufficient investments are made into experts who ensure its integrity, costs, and risks could outstrip an organization's capacity for creating value from big data.
Big data refers to data with greater variety and volume arriving faster, also known as the three Vs of big data.
Big data refers to large, complex data sets derived from new sources that exceed traditional data processing software's capacity to handle them. With access to such an abundance of information, business problems that had seemed impossible can now be tackled more efficiently.
What is Data?
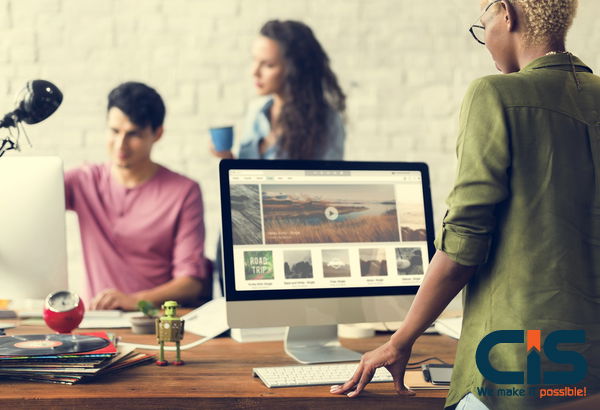
Computers use quantities, characters, or symbols stored as electrical signals on magnetic, optical, and mechanical media to perform operations. These signals may be stored, transmitted, and recorded for future reference.
What is Big Data?
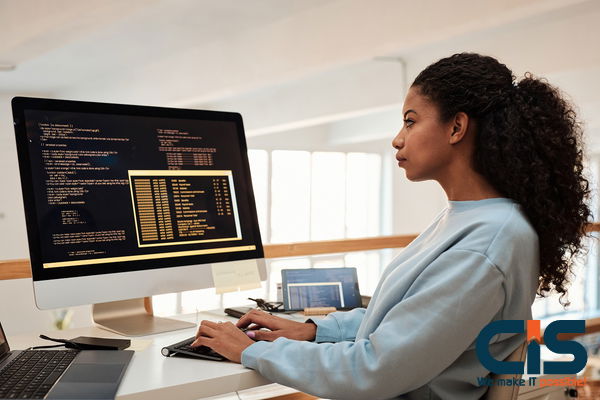
Big data refers to an accumulation of enormous volumes and growing complexity that traditional data management software systems cannot store or process efficiently. Big data encompasses everything from medical records and emails to geodata, tweets, and phone records - it all falls under this definition of huge quantities of information that's hard to store efficiently or process efficiently.
"Big data" refers to large and diverse information sets that continue to expand at an ever-increasing rate, often through data mining. Big data comprises three components - volume, velocity, and diversity of collected points - with mining being one source providing big data in various forms.
Big Data: A Brief History
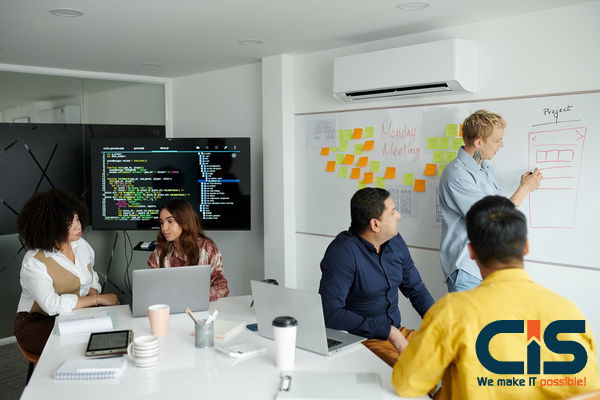
Large datasets date back to the 1960s when data centers and relational databases emerged from their infancy.
That same year saw Hadoop (an open-source framework designed specifically to store and analyze large data sets) come into existence; NoSQL also began gaining traction at this point.
Hadoop and Spark (and, more recently, Spark) have been essential in driving big data growth because they simplified working with it and made storage cheaper. Since their creation, the amount of big data created by users has ballooned dramatically, though not solely as humans produce vast amounts of information.
IoT (Internet of Things) technology has enabled more devices and objects to connect to the internet, collecting customer behavior data and product performance measurements. Machine learning has further expanded this pool of information.
Big data is still in its infancy. Cloud computing has expanded the potential for big data analysis, offering developers an elastic scale through which they can create ad hoc clusters to test out small subsets of information. Furthermore, graph databases are becoming increasingly prominent as they can display vast quantities of information quickly and comprehensively.
Characteristics
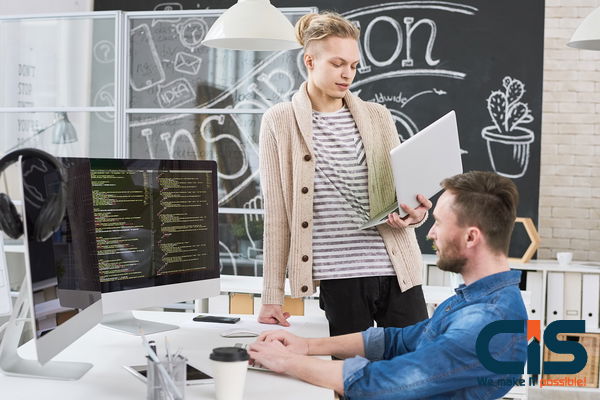
The following characteristics of big data can help define it:
Volume
Quantity and storage size of data. Size plays an integral part in determining its value, potential insights, and whether or not it falls under the umbrella of "big data," typically larger than terabytes or petabytes sizes.
Variety
RDBMSs were capable of handling structured data efficiently and effectively while transitioning from structured to semistructured or unstructured presented a new set of challenges to tools and technologies already in use. Big data technologies were initially developed to store and process semistructured and unstructured data produced at high speeds and large volumes, known as varieties. While structured data has also been stored using these tools and technologies. Processing structured information has always been left as an optional step, whether using traditional RDBMSs or big data solutions. By analyzing collected information through social media, logs, sensors, or any other source - such as log files or sensors - data analysis becomes much simpler to exploit any hidden insights it uncovers. Big data solutions combine text, images, and audio/video streams into one complete picture by filling gaps by merging missing pieces with related datasets.
Velocity
Big data's fast generation and processing are essential to meeting the challenges and demands associated with growth and development. Real-time access is possible to big data; its production occurs more frequently than small data. Frequency of generation, handling, recording, and publishing, are two forms of velocity related to big data.
Veracity
Big data analysis is only worth its weight in gold if its results can be trusted, as data quality may differ drastically, which impacts its accuracy and precision.
Value can also be assessed by looking at other qualities and attributes of big data and its profitability.
Variability
Big data can be defined by its changing format, structure, or source. Big data may be structured, unstructured, or both - and its analysis may involve raw data originating from multiple sources and transformation from unstructured to structured.
Big Data: More Characteristics
Here are some details regarding the new Vs. Associated with big data.
- Veracity refers to how accurate and trustworthy data sets are. Data quality issues may arise due to raw data from different sources that are hard to pinpoint; such issues could lead to analysis errors if left uncleaned through cleansing processes. Data scientists and consultants may add their input as well. Before undertaking big data analytics, organizations verify if the collected information relates to real business issues before sets often come with multiple meanings or formats from various data sources, making big data analytics and management increasingly complex.
Types of Big Data
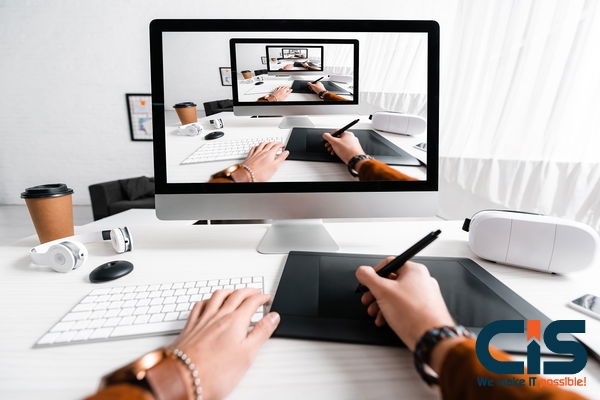
These are some instances of big data:
- Structured
- Unstructured
- Semistructured
Structured
Structured data refers to information easily accessed, stored, and processed using a standard format. Computer scientists have developed techniques for handling this kind of information where its format has already been established and ways of extracting value from it. Computer scientists anticipate potential issues due to increased access and storage requirements as its size expands exponentially.
Unstructured
Unstructured data refers to information with an unknown structure or form, posing various processing and extraction challenges for organizations today. Unstructured data typically comprise text files, images, and videos and poses significant difficulties for processing and extraction; many organizations today possess large quantities of unstructured data but lack an effective strategy for extracting value from it.
Semistructured
Semistructured information encompasses both structured and unstructured forms of data. Semistructured data may appear structured, yet it's not defined by something like relational database management system tables. An XML data file is one example of semi-structured information.
Big Data: How It Works?
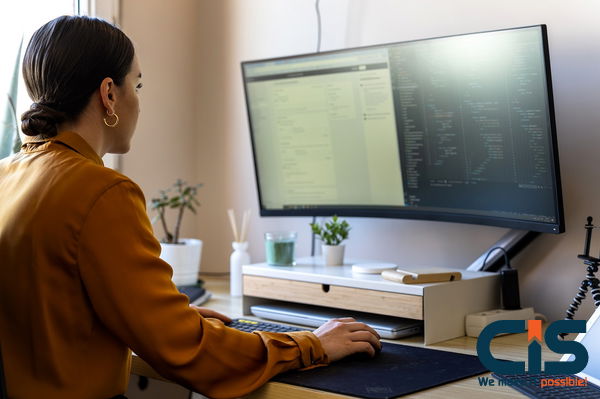
Big data can be divided into two distinct categories, unstructured and structured. Structured data refers to information managed by an organization, such as spreadsheets or databases, and is usually numeric. On the other hand, unstructured data refers to any form of unorganized information that does not fit a particular model or format; examples of unstructured data include social media data that helps organizations collect customer needs information.
Collecting big data is possible through various sources, including public comments posted to social networks, apps, and personal electronics devices with sensors that enable collection in diverse situations, questionnaires and purchases made online or electronically check-ins at retail establishments, purchases made electronically via check-in devices as well as purchases of goods with bar codes allowing collection.
Big data is often stored in databases and analyzed using software for large, complex datasets. Many software-as-a-service (SaaS) companies specialize in handling this kind of complex information.
Related:- Which Tools Are Involved In The Data Analysis In 2022?
Big data can provide you with new insights and open new business opportunities. Three key steps are required to get started:
- IntegrateBig data combines data from multiple sources and applications
Data integration methods such as extract-transform-load (ETL) cannot handle large datasets effectively. New technologies and strategies must be utilized to analyze them efficiently. You must integrate and process your data before providing it in formats your analysts can utilize.
- You can also manage
Big data needs storage solutions that support it locally and in the cloud, allowing it to be easily accessible when processing engines are required for analysis. People typically choose storage solutions based on where their data resides; cloud computing has grown increasingly popular as it meets your current computing requirements while offering additional resources when needed.
- Analyze
Reaping the benefits from your big data investments requires taking an active approach to acting and analyzing it. Visual analysis can give you fresh insights into your data. Explore your data to uncover hidden mysteries. Share what you discover with others. Create data models using machine learning or artificial Intelligence technologies. Put it all to good use!
Big Data: Its Uses
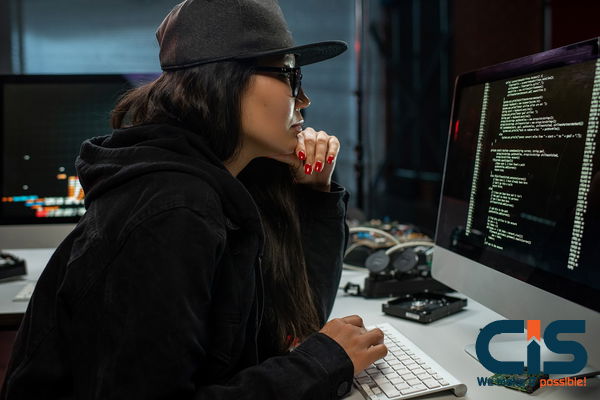
Data analysts use correlation analyses to examine relationships among various data types, such as demographics and purchase histories. Assessments may be performed internally by data analysts or externally by third-party specialists who specialize in converting big data into digestible formats - these experts are often hired by businesses for this task.
Data analysis can be applied across almost every department in an organization, from marketing to human resources. Big data is intended to speed the introduction of products onto the market faster while decreasing the time and resource requirements needed for reaching target audiences and gaining acceptance within markets - ultimately leaving customers satisfied.
Big Data Challenges
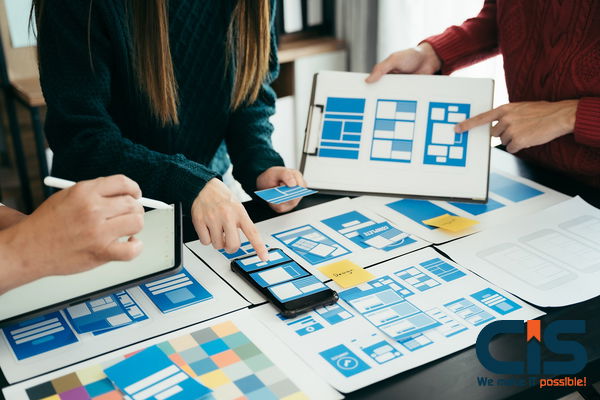
Big data is full of promise, but it also has its challenges.
First and foremost, big data is simply big. Data volumes continue to double every two years despite new storage technologies being developed; organizations often struggle to effectively store this massive volume of information and keep pace with its growth.
Data storage alone isn't enough; data must also be organized effectively to be useful. Curating involves much work in creating clean data for clients that enables meaningful analysis; data scientists typically spend up to 80 percent of their workday curating, organizing, and preparing information before being able to use it themselves.
Finally, big data technology is changing at an astonishingly rapid pace. Apache Hadoop became popular a few years ago. Later that same year, Apache Spark came onto the scene; today, most organizations use both frameworks together for optimal results. Staying up-to-date with emerging big data technologies remains an ongoing challenge.
Why is Big Data Important?
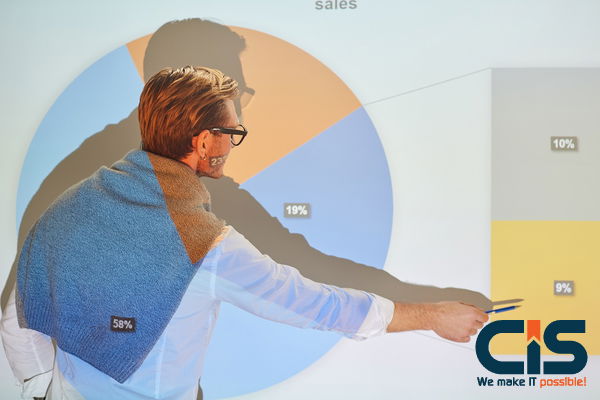
Big data definition allows businesses to enhance their operations, offer better customer service, customize marketing campaigns, and take other measures that increase revenues and profits. Businesses that utilize big data effectively enjoy an edge as they can make more effective and quicker business decisions than their competition.
Big data offers businesses invaluable insights about their customers that they can use to optimize marketing, advertising, and promotions, thus increasing engagement and conversion rates. Historical and current data can also help determine preferences among corporate or consumer buyers and better serve customer needs and wants.
Medical researchers and doctors use big data to diagnose diseases and medical conditions. Information obtained from social media platforms, electronic health records, and websites provides healthcare agencies and government entities with accurate updates about potential outbreaks or threats of infectious disease outbreaks or threats.
- Organizations use big data in numerous ways:
- Energy firms employ big data to identify drilling sites and track pipeline activities. At the same time, utilities utilize it to monitor their electrical grids.
- Financial services firms utilize big data systems for real-time market analysis and risk management. At the same time, they're used by manufacturers and transportation companies to optimize delivery routes and manage supply chains.
- The government uses emergency response, crime prevention, and smart city initiatives.
What Are Some Examples of Big Data?
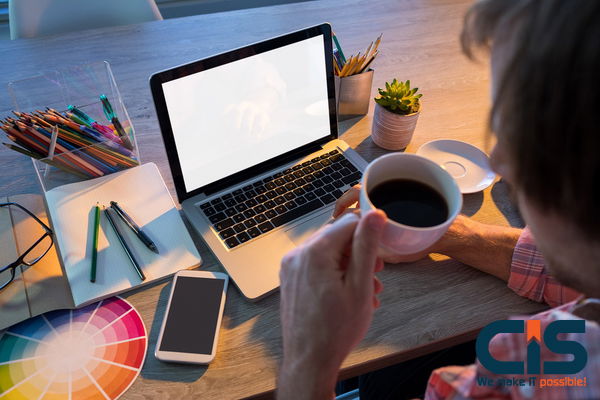
Big data comes from multiple sources: transaction processing systems (TPSs), customer databases (DBs), documents, emails, medical records, and clickstream logs from websites like clickstream logs on mobile apps and social networks, as well as log files generated by servers and networks as well as sensor-collected data from manufacturing equipment, industrial equipment or internet-connected devices.
Big data environments include external information on consumers, financial markets, and weather, as well as internal data such as images, videos, and audio files that they collect internally and streaming data which is collected and processed continuously by numerous applications that leverage big data environments.
Big Data: the V's and the A's
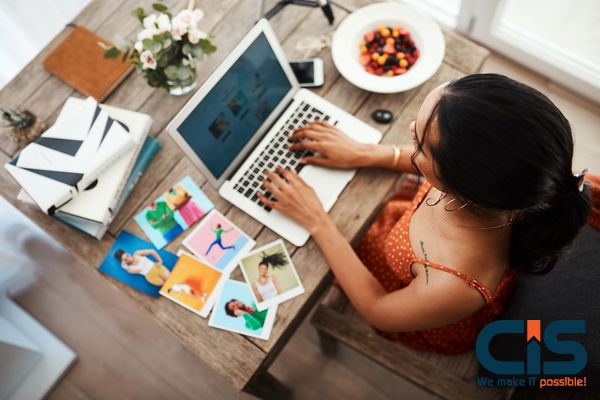
Big data's most distinctive trait is its volume. Although data collected and stored within a big data environment may contain large amounts, clickstreams, log files from systems, and stream processing are all sources of massive amounts of data that make up big data environments.
Big data encompasses an array of data types. Structured records such as financial transactions are examples of structured data. Unstructured documents, text files, and multimedia files also fall under this umbrella term. And finally, semistructured logs such as those generated from web servers or sensor data should not be forgotten either.
Big data systems must store and manage multiple types of data simultaneously. Applications utilizing these databases may contain multiple sets that may or may not be integrated; an analytics project, for instance, might try to predict sales by correlating past purchases with returns and online reviews with customer service calls.
Velocity refers to the speed at which data must be created, processed, and analyzed. Big data sets often need to be updated in real-time or near real-time instead of the daily, weekly, or monthly updates commonly seen with data warehouses. Proper velocity management becomes even more essential as big data analysis expands, including machine learning and AI analysis processes automatically detecting patterns that generate insights.
Advantages of Big Data Processing
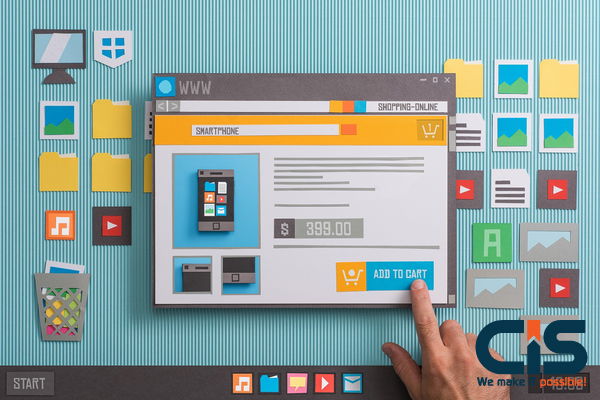
Big data processing with DBMS offers many advantages to businesses. Companies can utilize external intelligence from sources like Facebook and Twitter to make better business decisions; furthermore, social data from sites like these can help organizations to adapt their business strategies more precisely.
Improved Customer Service
Advancements in Big data technology are rapidly replacing traditional customer feedback systems. These new systems utilize big data and natural language processing techniques to analyze customer responses.
Early identification of any potential risks related to the product/services
Improved Operational Efficiency
Big data technologies increase operational efficiency by creating a landing area for new data before determining which should be transferred into a data warehouse. Integrating big data and data warehouse also allows organizations to offload seldom accessed files.
How Are Big Data Processed and Stored?
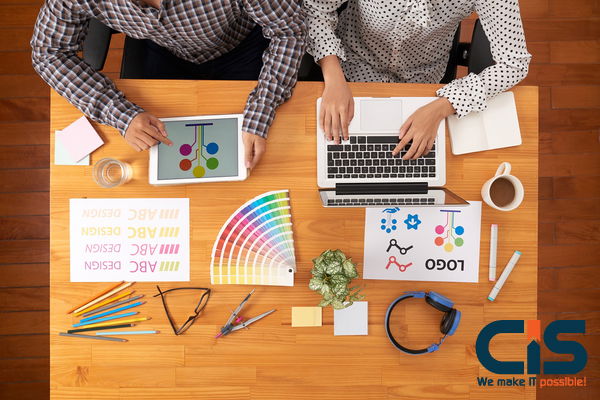
Data lakes are frequently used for storing large volumes of data. Common platforms for data lake storage include Hadoop, cloud object storage, NoSQL databases, or other similar platforms. While data warehouses typically utilize relational databases to organize structured information into databases for easy storage purposes, data lanes can accommodate various data types.
Big data environments often involve multiple systems integrated with a distributed architecture. For instance, a central database lake may be linked with relational databases and a data warehouse for analytics needs. Big data systems often store their raw data before being organized for analytics; sometimes, data preparation software preprocesses it before storage in analytics tools.
Big data processing places immense strain on computing infrastructure. Clustered systems utilizing technologies like Hadoop and Spark to distribute workloads among hundreds or thousands of commodity servers provide the computing power to process big data sets of virtual machines.
Accessing processing power at an affordable price can be challenging. Cloud-based big data systems have become an increasingly popular solution; organizations can either deploy their own or subscribe to managed big-data-as-a-service offerings from cloud providers, with users increasing the number of servers required for analytics projects as necessary - instances being turned on and off as required; thus businesses only pay for computing and storage time consumed cloud user.
What is Big Data Analytics? How Does It Work?
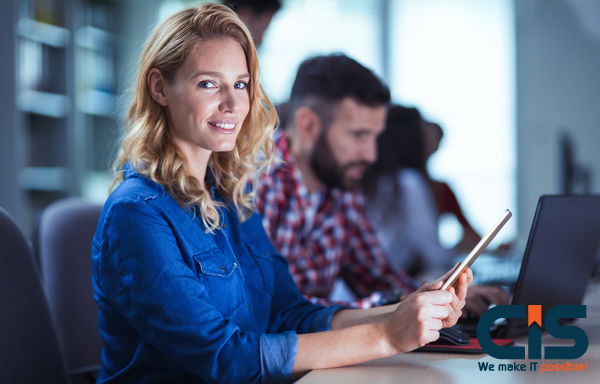
Data scientists and analysts must have an in-depth knowledge of available data to produce meaningful and valid results from applications that utilize big data while understanding their goals as they conduct analytics processes on it. Data preparation, therefore, plays a key part in analytics processes by profiling, cleaning, validating, and transforming sets of private clouds.
Once data has been gathered and ready for analysis, various data science and advanced analytical disciplines may run various applications using tools with big data analytics capabilities and features. These may include machine-learning and deep-learning applications, predictive modeling and statistical analysis, streaming analytics, text mining, and many other cloud vendors of private cloud or virtual machine users.
Analyzing customer data sets as an example; a wide range of big data analyses has many branches to explore:
- Analysis of comparative data. This technique compares companies' products, services, and brands against their rivals by analyzing customer behavior metrics hybrid cloud.
- Social media listening. Social media listening involves monitoring what people say about a product or business to detect marketing challenges and target audiences more accurately cloud providers analytics tools.
- Marketing analytics. Marketing analytics provides data that can be used to enhance marketing campaigns, promotional offers, and business initiatives.
- Sentiment analysis. Customer data can provide insight into customers' attitudes toward brands or companies, their satisfaction levels with services provided, potential issues they might encounter, and ways to enhance customer service google cloud platform.
Big Data Management Technologies
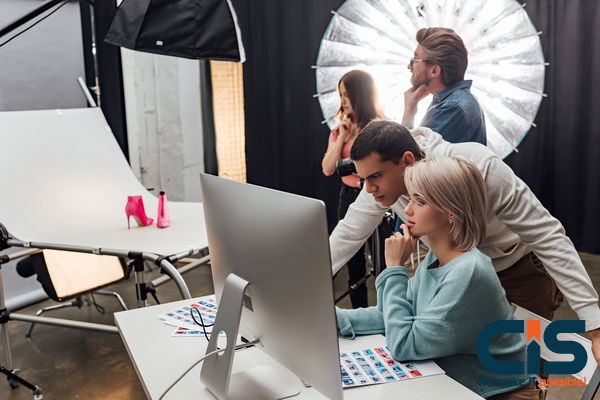
At first, Hadoop was the go-to big data architecture, an open-source distributed processing framework first released in 2006. But as processing engines such as Spark have come online since 2006, MapReduce has seen less use. Now there is an ecosystem of big data technologies which often coexist but may also be deployed independently for different uses of google cloud or google cloud platforms.
IT vendors offer big data platforms that combine various technologies into one package, typically used for cloud applications. Below is a listing alphabetically of such products available today:
- Amazon Elastic MapReduce (EMR), Cloudera data platform, and Google Cloud
- Dataproc can all provide services similar to the MapR data platform
- Both organizations currently use Amazon EMR as an EMR alternative for
- Amazon Web Services and Microsoft Azure HDInsight services for real-time analysis of large datasets.
Organizations looking to deploy big data systems themselves on-premise or via the cloud have access to tools, including Hadoop and Spark, that can assist them with google cloud or cloud users.
- Storage repositories such as Hadoop Distributed File System, Google Cloud Storage, and Azure Blob Storage; cloud management frameworks like Kubernetes (also known as Mesos), YARN (Yet Another Resource Negotiator) or Hadoop's integrated resource manager and scheduler may all be utilized for data storage needs.
- Flink (also called Hudi), Kafka, Samza, and Storm are stream-processing engines that leverage streams. Spark has its own streaming and structured streaming modules built in; NoSQL technologies such as Cassandra (also Couchbase), CouchDB, HBase, and MarkLogic Data Hub provide NoSQL database options, as do MongoDB Neo4j Redis Redis and other similar technologies.
- Amazon Redshift and Google BigQuery are popular data lake and warehouse platforms; Drill Hive Impala Presto Trino are some SQL query engines to consider for managing data lake environments and warehouses cloud computing services cloud users.
Conclusion
Big Data, low-cost hardware, and modern information management and analysis software have contributed significantly to a radical transformation in data analytics history and practice. Together these trends have come together, enabling us to quickly and efficiently analyze previously unimaginable datasets rapidly and efficiently - not as theoretical or trivial capabilities; rather, they represent real gains in efficiency, productivity, and revenue for organizations that embrace cloud computing services.