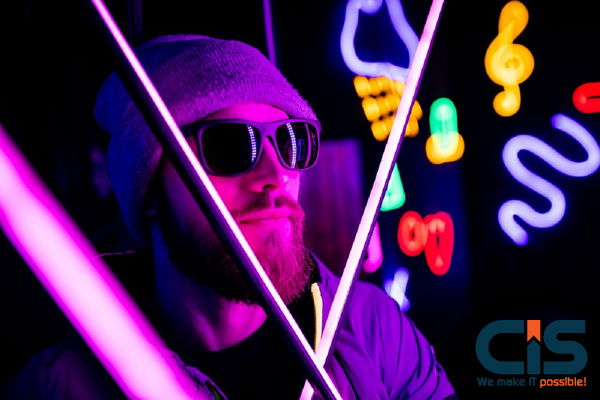
What Is Big Data?
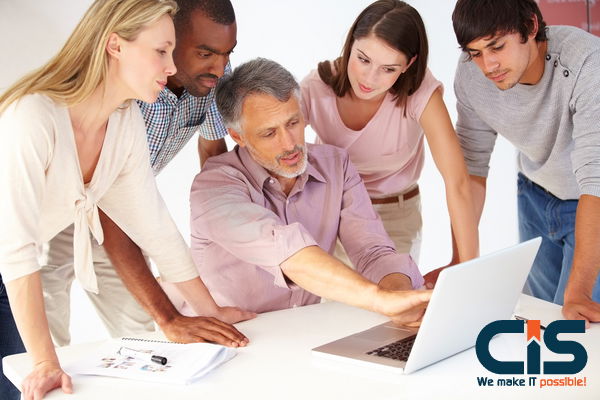
Big data refers to any mass of unstructured, complex information that exceeds traditional database management systems' handling capability. Data of this magnitude cannot be managed or processed using traditional database management applications or data processing software.
What sources are this data? What contribution have we made towards such vast collections of data?
Sources Of Big Data
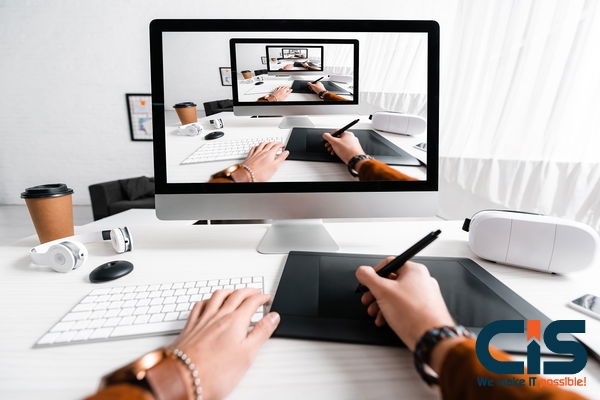
Data available on Earth continues to expand exponentially as humans generate it through daily activities and various sources. Internet, mobile phones, and IoT have connected us worldwide; every decision leaves its digital trail behind.
Whenever you access an app on your mobile phone, sign up on a website, visit a webpage, or type keywords into search engines, some data is collected about you. Imagine this scenario. In our browser, we typed in "Big Data". After clicking on this article's link, we see its sheer volume of information. Imagine people spending their free time online: visiting various websites, uploading images, and more - this vast amounts to an immense amount of data that requires storage space for processing and analysis.
Primary data sources are mobile phones, social media platforms and websites, digital images, videos, and sensor networks; purchase transaction records, medical files, eCommerce systems, military surveillance systems, medical records, and scientific research are also important sources.
It would take approximately Quintillion Bytes of data storage space for all this to exist, and 40 Zettabytes would be generated each year, which equates to multiplying every grain of sand on Earth by 75 times!
Big Data Types
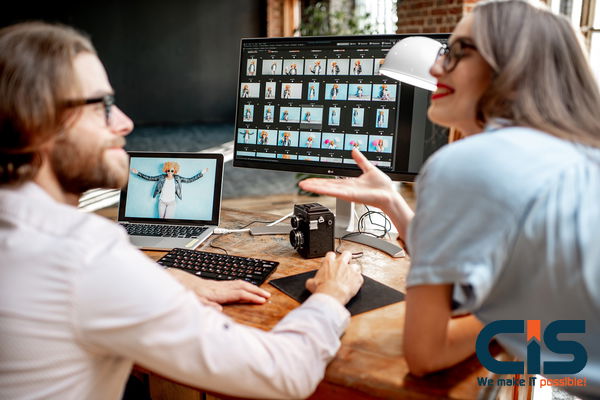
Big data comes in three distinct forms: Structured, Semistructured, and Unstructured. Let us now examine these forms and their examples in more depth.
1. Semistructured
Semistructured data refers to information that does not follow an ordered list like RDBMS tables; instead, it uses organizational properties like tags or markers for the separability of semantic elements, thereby making analysis more straightforward.
2. Unstructured
RDBMS cannot store unstructured data as it lacks form or structure; such unstructured information can only be adequately analyzed once converted to a structured format; over 80% of organizationally generated information falls into this category.
3. Structures
Structured data refers to information that is quickly processed, stored, and accessed using standard formats for processing, storage, and accessing purposes. Structured data has an easily manageable structure with a predetermined schema that makes processing straightforward; SQL can help manage structured data effectively.
5 V's Of Big Data
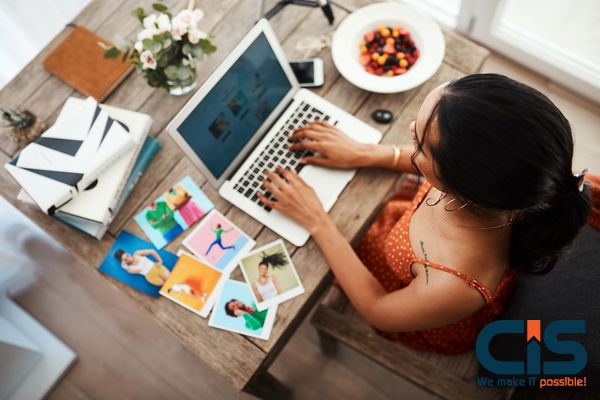
The 5 Vs. are Volume, Variety, Velocity and Value. These are the Big Data Characteristics .
1. Volume
Volume is defined as the daily accumulation of data. Volume helps define whether we classify certain sets as big data; "Volume" ranks highly when dealing with Big Data.
2. Velocity
Velocity is the rate at which massive volumes of data are created from various sources daily and constantly flowing into society.
Facebook DAU Mobile now has approximately 1.03 billion Daily Active Users, an increase of 22% year over year and evidence that social media use and data collection continues at an incredible pace. This shows how fast its growth is taking hold across platforms like mobile.
Real-time data allows us to gain insights and make decisions in moments, provided we can handle its speed.
3. Variety
Variety refers to data generated from diverse sources in its various forms; semistructured, unstructured, and structured forms all fall under this definition.
Data used to be contained solely within Excel or databases; now, it may come in forms such as pictures, audio/videos/PDFs, etc, and create issues when storing, mining, and extracting it for insight.
4. Veracity
Data inconsistency, incompleteness, and integrity contribute to data uncertainty; organizations must consider this when dealing with Big Data.
5. You Can Also Value
Data without analysis lacks value and must be converted to something usable for its full benefits to become apparent. Simply gathering and storing vast volumes of raw information has no meaning or use; until its analyses occur, it's simply gathering dust.
Want More Information About Our Services? Talk to Our Consultants!
Big Data Challenges
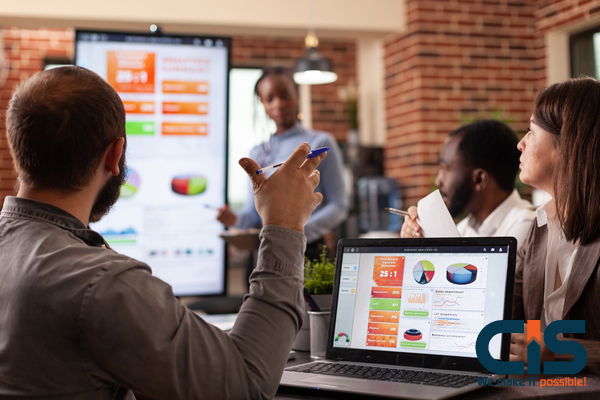
1. Data Growth
Finding insights within rapidly increasing data can be challenging; finding relevant data amidst vast quantities produced every second can be impossible.
2. Data Storage
Organizations need help managing and storing vast volumes of data. Reliable storage solutions must be in place to do so effectively and flexibly.
3. Data Quality
Data created by organizations often needs to be more coherent, complete, and organized - making its management even more complicated than expected. Companies in the US alone lose about $600 billion every year because of flawed or inconsistent information.
4. You Can Also Read About The Advantages Of Using It.
Organizations often need more awareness of what data exists within their system, making data analysis challenging.
5. The Security Of Your Own Home
Organizations face the daunting challenge of safeguarding all the information within their possession from advanced persistent threats that seek to compromise it through authentication, authorization, encryption, etc. To keep data safe, they enlist authentication services such as OATH to authenticate users before authorizing access and encryption of data, etc.
6. Miscellaneous Challenges
Big data presents numerous difficulties that must be navigated successfully, from talent availability and integration issues, cost concerns, and accuracy issues, all the way up to timely processing requirements and timely processing requirements.
Big Data Examples
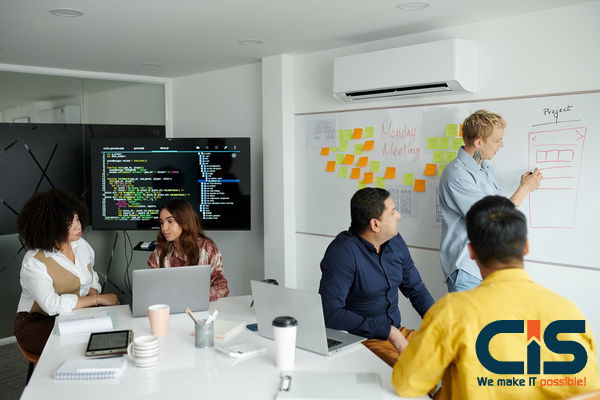
- The New York Stock Exchange produces one terabyte worth of new trading data daily.
- Every day, 500 million tweets get sent.
- Amazon handles 15+ million clickstreams of customers per day on average to recommend products.
- Walmart, an American Multinational Retail Corporation, handles about 1,000,000+ transactions per hour.
- Every day, Whatsapp sends 65 billion messages.
- In 30 minutes, a single jet engine can generate more than 10 Terabytes of data.
- Every day, 294 billion emails are sent.
- Nearly 100 sensors are used in modern cars to monitor tire pressure, fuel levels, etc. Sensor data is generated by the car.
- Facebook analyzes and stores more than 30 Petabytes per day of data generated by its users.
- Every minute, YouTube users upload 48 hours of videos.
Big Data Analytics: Advantages
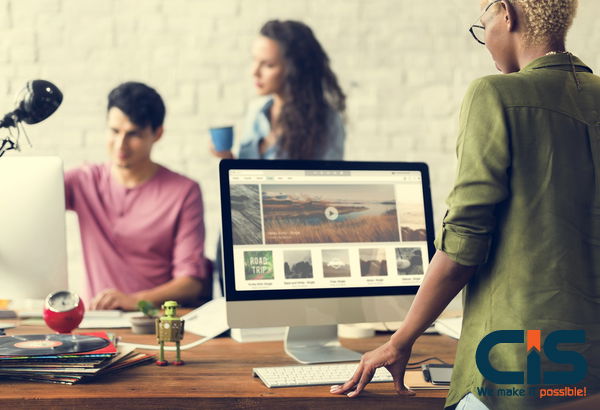
Without analysis, all that growing Big Data would be useless to anyone. Analyzing data has many benefits that must be considered.
- Data analysis allows businesses to draw upon external intelligence when making decisions, drawing data from social media websites like Facebook and Twitter to strengthen their business strategies.
- Big Data Analysis can assist organizations in improving customer service. New systems utilizing big data technology are replacing traditional customer feedback systems; these read and evaluate responses using Big Data and Natural Language Processing technologies to analyze them properly.
Big Data Job Opportunities
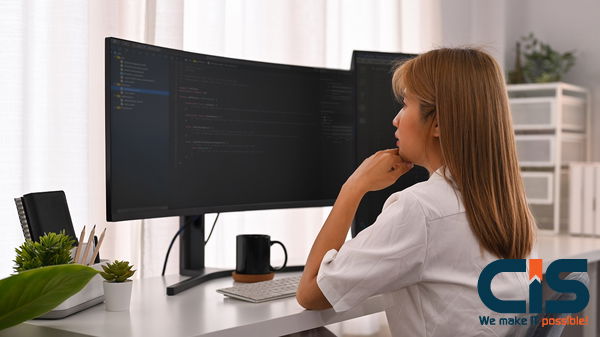
By 2025, the global big data market is projected to reach USD 229.4 Billion with compound annual compounded annual growth at 10.6% compound annual compound annual. This rise is primarily attributed to IoT devices' growing adoption, organizations' more readily accessible data for insights, and government investments across regions.
These factors offer ample job opportunities in this domain for individuals in this profession - analysts, data scientists, architects, database managers, and prominent data engineers, among many more vital roles that are sought out to fill this space.
Big Data Technologies Stack
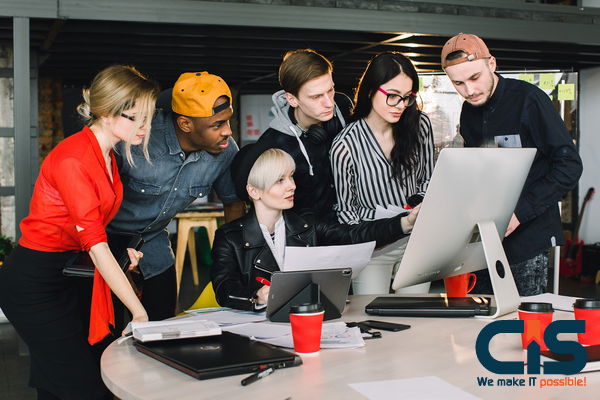
Big data technologies and tools exist to handle such an abundance of information. You should master these technologies if you wish to excel in the big data market:
- Apache Hadoop is a distributed processing framework that is open-source. This is the best option for batch processing.
- Apache Spark Apache Spark is a real-time processing framework that is open-source. It is a real-time processing framework.
- Apache Kafka : This platform is for streaming.
- Apache Hive Apache Hive is an open-source data warehouse tool that allows you to query a large amount of data in Hadoop HDFS.
- Apache Cassandra This is a distributed NoSQL open-source database that stores and processes Big Data.
Big Data Applications
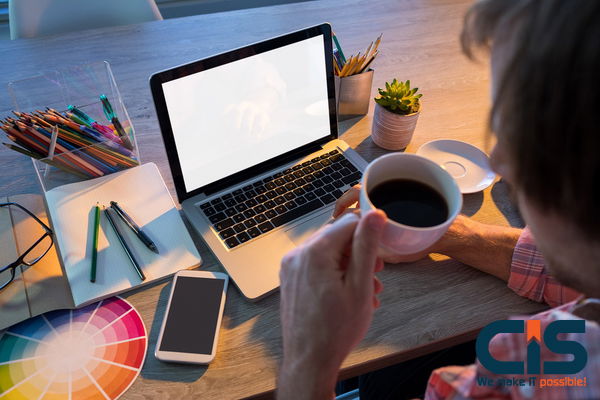
Big data applications span many industries and domains; this article details some of them briefly. Bank and Finance: Utilizing Big Data helps detect frauds, manage risks, and analyze abnormal trading in banking and finance institutions.
- Agriculture: Agriculture uses data collection techniques to increase crop productivity. One such practice involves planting test plants that record and store data regarding how plants react to environmental fluctuations; later, planners can use this information when planting crop fields.
- Marketing and Advertising: Advertising agencies gather customer data about customer preferences and behaviors to gain a fuller picture of their audience members.
- Entertainment and Media: Entertainment and media industries utilize big data analytics to reach their audiences effectively, knowing exactly which ads draw customers in and when to broadcast them.
- Education Sector Big Data Analysis has made waves in education. Many applications use Big Data Analytics to create an inclusive platform and understand each student's learning capabilities.
Prerequisites For Learning Big Data
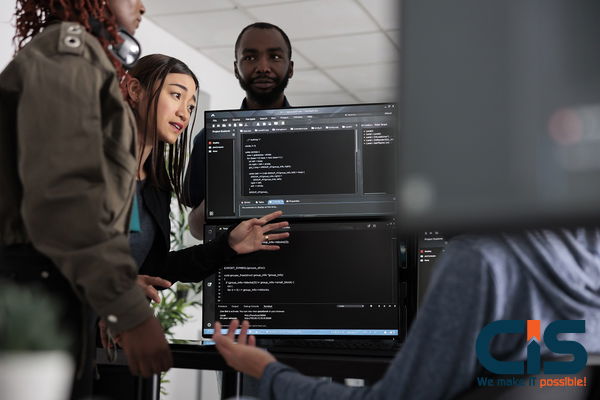
Here are the prerequisites you should master to learn about big data.
- SQL, Data Warehousing/Data Processing, and Database Knowledge: This area involves SQL to query databases and manipulate information within them; data mining/warehousing to extract key insights; building/training models using Machine Learning algorithms on those insights derived.
- Data analytics languages include Java, Scala, and Python; therefore, to gain customer experience with big data, you should know at least one programming language.
- Mathematics and Statistics: Formulae and concepts from mathematics and statistics play a central major role in most Big Data and Machine Learning algorithms.
- Any enormous data enthusiast must possess analytical and problem-solving abilities in order to successfully use data. These include being able to solve issues by asking pertinent questions and examining them effectively to gain meaningful insights from them.
Also Read: Big Data Analytics Benefits - How To Analyse Big Data
Get Started With Big Data Fundamentals
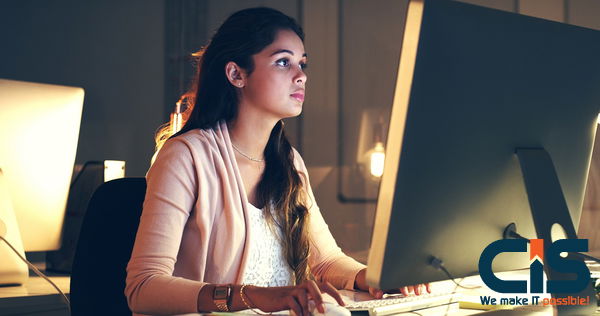
Your journey with big data should begin by learning its fundamentals before transitioning into its rapidly developing field of big-data analytics. Big data is more than simply storing lots of information; instead, it involves finding insights to inform decisions by analyzing large volumes of structured and unstructured data sources. By starting right, you should quickly become adept in solving real-world big data problems using these approaches and methods.
You can start by following the steps below:
1. Learn The Fundamentals Of Data Analytics And How To Build A Foundation For Big Data
At its core, these skills encompass complex abilities such as Structured Query Language (SQL), Python programming language, and mathematical concepts such as Probability and Statistics; furthermore, they cover soft abilities like communication, presentation skills, and business domain knowledge.
2. Get Cloud And Big Data Certifications
Take online courses and gain knowledge on Big Data analytics theory; this article provides an introduction.
Learning big data at your own pace has always been challenging with YouTube tutorials. These online courses let you set the pace, along with informational blogs and newsletters from top writers and YouTube tutorials.
Add certificates to your portfolio to expand its scope. Depending on your level and interest of expertise, there is an array of big data cloud certificates available; we suggest getting hands-on experience working with various cloud service providers to learn how they might solve real business issues.
3. Work On Real-World, End-To-End, Hands-On Big Data Projects
Integrating theory learning, project implementation, and practical applications of your knowledge are equally important in real-world situations. Projects will allow you to identify appropriate methods when responding to particular use cases or business problems.
Practical experience is vital when starting in Big Data, enabling you to develop new ideas and enhance your analytical abilities. Prominent data experts are in demand among organizations looking for natural solutions; projects where their outcomes help make appropriate decisions, require Big Data experts. You can gain a better grasp of various paradigms within this discipline through working on various projects involving Data Analytics, Cloud Computing, and Data Processing as you gain experience working on various paradigms such as Predictive Modelling or Exploratory Analysis with Algorithms as starting points.
4. Doing Big Data Internships
Internships provide the ideal way to develop skills. They combine theoretical knowledge with real-world experience.
Internships in Data Science, Analytics and Statistics, Deep Learning, Machine Learning, Cloud Computing, and Python Development can be an excellent way to learn about big data. At Forage, we offer hands-on experiences from projects at well-recognized companies leveraging Data Analytics - giving an excellent virtual impression of the work culture within these organizations.
Some of the top internships to consider as starting points include:
- The Google Data Scientist Internship
- The IBM Data Science Internship
- The Microsoft Data Sciences and Applied AI Internship
You can also learn a lot by interning at a startup. They will assign you tasks that you have to complete from scratch.
5. Keep Learning, Researching, And Building Projects
Becoming an effective programmer involves skills such as problem-solving, analytical thinking, object-oriented coding, debugging, and object-oriented programming. Computer Science and Mathematical Concepts may also be necessary. Big Data professionals require additional specialized expertise.
Functional programming offers an approach to learning popular programming languages such as Python, R SQL, Java, or Scala, which help solve business issues efficiently and quickly process large volumes of data.
As part of applying your knowledge, the last step should be building prototypes. This allows for deploying working models into production by working on technologies like Hadoop Framework, Apache Spark/Spark SQL, Docker, or Kubernetes.
Top Three Reasons To Learn Big Data In 2023 And Beyond
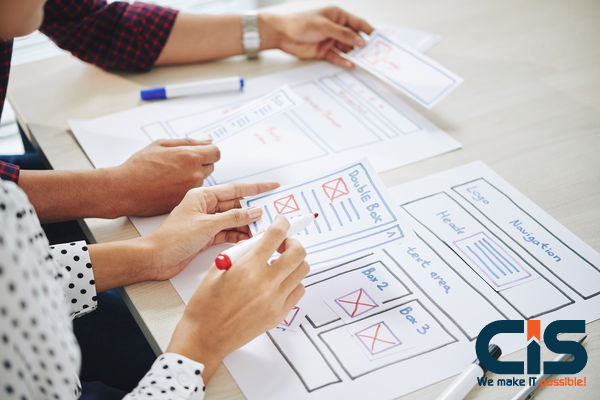
Big Data offers an untapped source of extra income. As more data becomes available, analysts, scientists, and architects become in high demand.
Glassdoor reports that the average salary of prominent data engineers in India massive amounts to Rs 729 359 annually, making this field increasingly relevant as 2023 progresses and beyond. Learning considerable data skills should be for more than just financial gains. Other reasons make learning this field important, too!
The Exponential Growth Of The Big Data Market
Big data is anticipated to experience exponential global expansion without showing signs of rapid decline over the long term. According to projections by NASSCOM, India's significant analytics data sector will grow from $2 billion in 2025 to an anticipated total value of $16 billion by 2025.
Big Data Learning Is Full Of Career Opportunities And Job Prospects In The Industry.
Data professionals contribute to all industries. You could work anywhere - finance, manufacturing, or information technology are just three. Every sector uses Big Data today as a competitive advantage and to make data-driven decisions; now is an opportune moment to explore this exciting profession further!
Big data salaries depend heavily on education, domain knowledge, and acquired skills. Now is an opportune time to add significant data skills to your resume and take advantage of job market demand; Big Data careers span multiple domains, including Engineering, Science, and Analytics.
Learning Big Data Provides Competitive Advantage
Data-driven decision-making is one of the core capabilities of big data, providing businesses with a competitive advantage in the job market through data analysis rather than instinct. Gaining proficiency with big data requires understanding cutting-edge tools and technologies for greater profits from companies using it, including competitive job searches that leverage it effectively.
An effective investment is learning about big data. Extensive data analysis offers many mysteries to be resolved and will sharpen both analytical skills and enhance thinking ability. To be truly successful on one's career journey, one must have both hard and soft skills at their disposal.
Want More Information About Our Services? Talk to Our Consultants!
Summary
Big Data refers to any vast accumulation of information from various sources - websites, mobile phones, weblogs, IoT devices, etc - created using no traditional database management system.
Big data can be divided into semistructured or unstructured categories. Unstructured data refers to information with no defined structure, while structured data has predetermined rules attached. Big Data is defined by five Vs: Volume, Velocity, and Variety of Veracity and Value that describe its formation and analysis by companies like Facebook, Whatsapp, Twitter, Amazon, etc, daily.
To establish yourself in Big Data, it is crucial that you become acquainted with different tools like Apache, Hadoop, Spark, Kafka, etc.