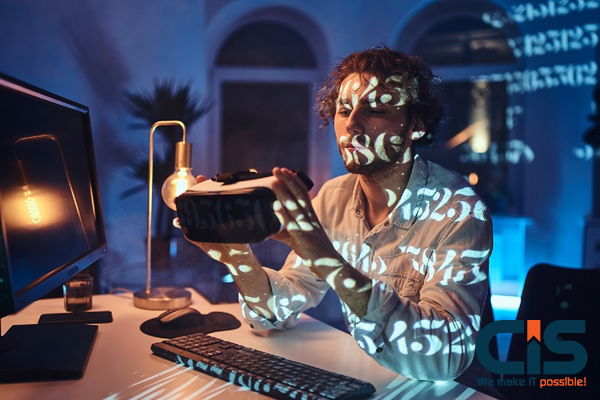
In the future, the push for cloud computing and the increasing number of machine-learning models that grew tremendously during the pandemic is expected to continue. While operationalizing Artificial Intelligence, it was found that only 27% of projects piloted in organizations made it to production.
Machine learning Operations: What are they?
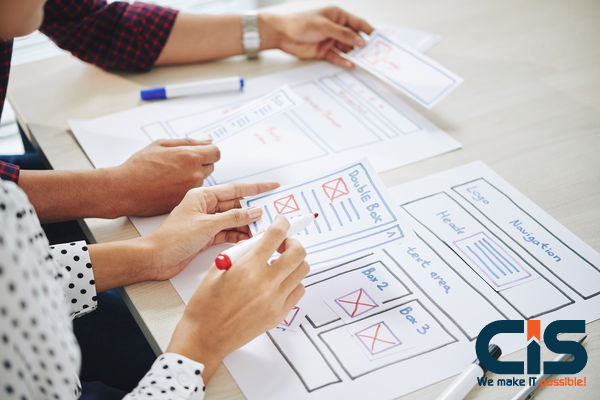
MLOps is the management of data scientists, operations, and other resources in order to develop, deploy, and monitor models successfully. MLOps help teams develop, deploy, monitor, and consistently scale AI and ML, thus reducing risks from not having an innovation framework. MLOps can be considered a formula for success.
Challenge
Several issues are responsible for the disparity in AI/ML's current and potential uses. The problems are mostly related to the model-building, iteration and deployment of AI/ML. These concerns must be addressed if AI/ML will change the corporate landscape globally. Suppose organizations have begun operationalizing AI/ML or are generating Proofs of Concepts (PoC). In that case, they can avoid these pitfalls by proactively incorporating MLOps best practices to ensure smooth model development.
Even worse, companies spend valuable time and money monitoring and retraining their models. Data scientists do not have the infrastructure to replicate successful machine-learning experiments.
Planning the implementation of
Machine Learning models are often developed with an objective in mind. This can be as simple as keeping fraudulent transactions below 0.1 per cent or even recognizing faces on social media platforms. Business objectives may also include technical requirements, financial constraints, and performance targets. These can all be expressed in key performance indicators (KPIs), which allow for the monitoring of ML models that are currently being used.
MLOps helps ML-based products get to production quicker through automating model training and retraining and using continuous integration and delivery strategies.
MLOps is a framework and set of practices that allows data engineers to create automated data platforms and pipelines. They can also automate data feedback loops to improve models. This helps to resolve more than fifty issues related to the absence of clean, monitored, controlled, and regulated data.
Machine Learning is a branch of Artificial Intelligence that uses algorithms to enable computers to gain knowledge from data without being explicitly programmed. "Machine learning" was first used in 1959, but the concept only took off recently. Machine learning is becoming more important in automating RPA and IPA processes and optimizing an organization's technology capabilities.
You'll see several short- and long-term benefits if your company can develop a solid machine-learning strategy. This can be a key component in your plan to go beyond the manual, time-consuming, and unreliable processes towards Intelligent Automation. This article will provide five tips on how your business can leverage machine learning and deeper learning.
What is Machine Learning?
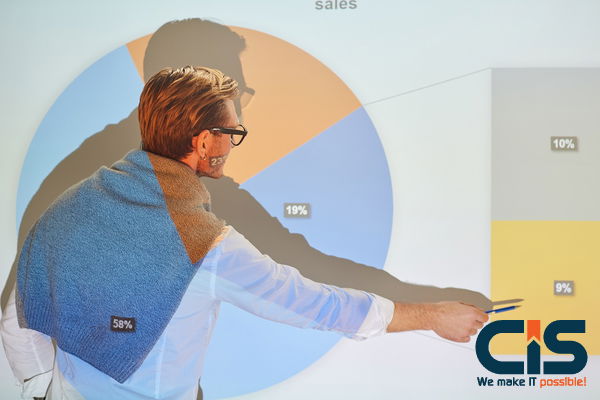
Machine Learning is a branch of Artificial Intelligence that allows machines to gain knowledge from previous data and experiences without being explicitly programmed.
Machine learning is divided into three basic types.
- Learning under supervision
- Reinforcement learning
- Unsupervised Learning
The choice of type will depend upon the expected results, the training data and the techniques used.
Machine learning programs allow companies to analyze and process data for improvement and better decision-making. It may sound abstract, but it is a way for software to learn to perform certain tasks without needing a person to guide them.
The use of Machine Learning is amazing. For example, it was used to build the neural networks supporting Sophia, a humanoid social robot. However, businesses commonly use machine learning to develop recommendation engines, workflows for customer experiences, fraud detection, scoring and modeling.
Want More Information About Our Services? Talk to Our Consultants!
Deep Learning: What Is it?
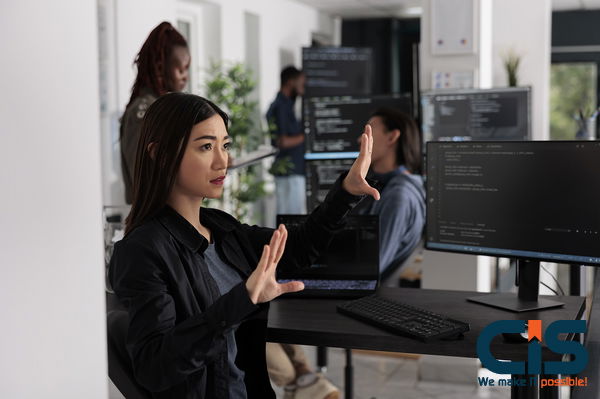
Deep learning, also known as Machine Learning (ML), is a technique that uses the same analytics lifecycle but neural networks to solve similar problems to those of a human brain.
Deep learning, the key technology in driverless cars, allows them to identify a stop sign or distinguish between pedestrians and lampposts. This technology is behind voice-activated devices such as phones, tablets and TVs.
What is Artificial Intelligence (AI)?
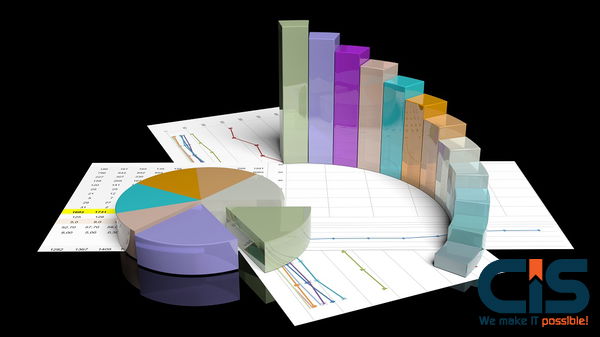
Artificial Intelligence is the concept of creating intelligent machines capable of simulating human behavior and thinking. Machine Learning, on the other hand, is an application that is part or subset of AI which allows machines to be taught from data.
Machine learning: Five uses for it
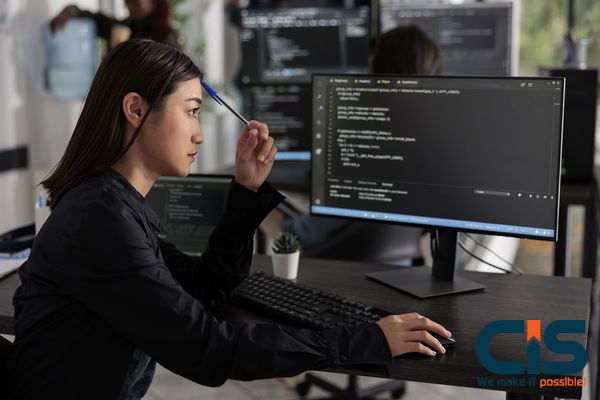
Machine Learning adds instantaneous, valuable data to your business. These are the top use cases for the technology that leaders in business leverage.
1: Smartly recommend products
Amazon and other online giants have become what they are because of machine learning and automation. Each time a consumer visits a website, searches for products, views product pages and purchases items, these actions become a data point used to create a detailed customer profile.
Machine learning allows you to analyze billions of data frames (data points) to begin understanding patterns in consumer behavior. Companies can use these insights to develop more personalized consumer experiences or product recommendation engines that offer the resonant and personal digital interaction consumers crave today.
2: Predicting demand
Machine learning is able to use different factors in a similar scenario to that described above to determine product demand. A sudden price increase, a change in competitors' pricing and changes to search volumes can all help identify changing demand. It has implications for the entire value chain.
Take the rapid change in demand for consumer products at the start of the pandemic. With machine learning algorithms, retailers were able to predict demand and identify an increase in toilet paper and cleaning products. They could then proactively replenish these items, order from suppliers and manage purchasing restrictions so that supply was even. You can use machine learning to identify decreasing demand and then adjust your stock plan or put specific products on sale. Machine learning can help you optimize planning and delivery dates and tell you when to promote and market products.
3: Dynamic Pricing
Typically, brick-and-mortar stores tag their items with a fixed price. Online retailers have more flexibility in pricing. Machine Learning allows you to monitor various factors, including changes in the demand for products, the competitive landscape and even searches to determine which services and products are popular.
If there is a spike in the demand for a product or category, it may be a sign to raise prices. However, if interest has dwindled on that particular item or category, then a drop in price could reignite sales. Machine Learning allows for automatic monitoring and timely adjustments driven by market changes.
Read More: Machine Learning And Deep Learning Are Becoming Increasingly Important For Businesses
4: Fraud detection
In all industries, fraud detection and prevention is of paramount importance. Human audits or manual processes can be prone to error. Machine Learning allows you to identify data anomalies that need to be investigated.
If a sudden increase in credit card spending is out of character for a customer, they could be making a big purchase. It could also mean that the cardholder is unaware of the fact. Fraud can be detected at all levels, including tracking customer and employee behaviors.
5: Video and image recognition
Data types such as images and videos are increasingly common in business. It doesn't matter if the data comes from social media or if it is data collected by field service technicians while they are working with clients. The ability to categorize and recognize what is happening in an image or a video is crucial.
Machine learning can automate this process, which also helps to quickly classify unstructured video or images for inclusion in your larger company data. This can be done to detect anomalies. Some companies use Machine Learning, for example, to check images and videos for security violations. Some companies use ML to analyze photos of the layout of stores to verify that retailers comply with CPG manufacturer marketing agreements.
Implementing Machine Learning
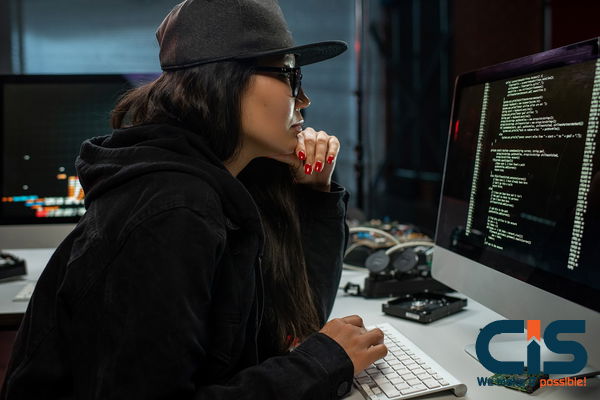
Leading organizations today use Machine Learning tools to automate their decision-making process, and they are now experimenting with Artificial Intelligence for Digital Transformation. Machine Learning is not without its challenges. Several factors can hinder the use of the algorithms. For example, the lack of familiarity with the technology or process flow may prevent implementation. Or the company might not be able to access the results for long enough so they could make the necessary decisions. It is also difficult to determine the right amount of money and time to spend to ensure it performs as intended.
You are fortunate to have experienced partners who can help in these situations. Cisin regularly works with customers who are implementing Machine Learning. Our experts have decades of experience in implementing Machine Learning programs. They assess the entire program, from the use cases to the model design.
Our team has experience in every aspect of Machine Learning from conception to implementation. Our team can provide ML programming in addition to its theoretical aspect. We can also help with data wrangling, testing, custom software development services implementation, hardware scaling, and training. Combining these elements will give your team the tools to make the most of Machine Learning.
Raw data and the Product Life Cycle
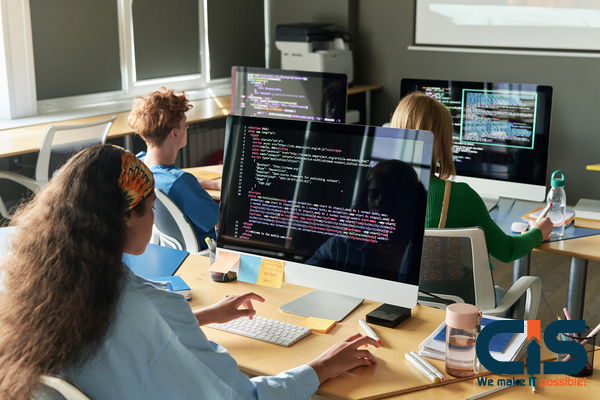
The product life cycle includes the planning stage, discovery, product definition, development and launch.
Data can be used to estimate the size of problems during the planning phase and help us understand possible solutions.
Data is a crucial component of the design phase for experiments to measure the impact of changes in a product. It can guide the decision-making process on whether or not to launch the product.
Data plays a key role during the launch in measuring the main improvement results based on experiment and OKR metrics. It also allows future data insights to be leveraged for future planning stages and experiments that will measure the product's long-term impact.
Big data collection includes metrics and data from different sources, enabling you to understand current and potential customers better. You can better understand your clients, their challenges, and their pains.
Data leveraging has many benefits
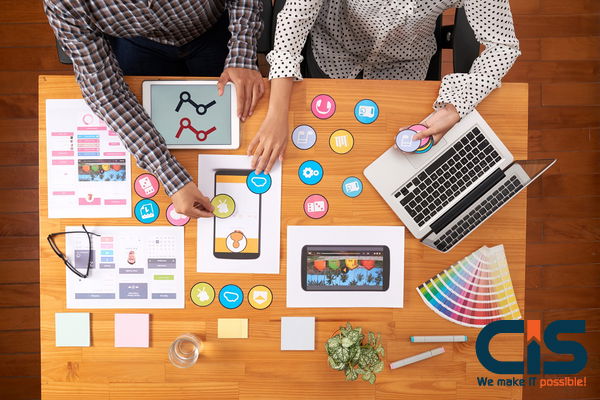
The research shows that data-driven companies are up to 5% and 1-3% more productive than their competitors.
We will quickly explore the main benefits that data insight can provide for your company.
Smooth product development
Before launching a product, there are many important steps to take, including conducting market research, analyzing feedback, developing a marketing strategy, and tweaking other aspects. The collection of data can assist businesses in identifying the products that their clients want. We can use data analysis to predict and measure future client outcomes. Businesses can use data to gauge customer feedback, create customer journeys and draft lead funnels.
Marketing cost reduction
Any business must focus on expanding its customer base and promoting new brands. Data analytics helps to focus only on strategies that have the desired outcomes. This allows you to launch customer-oriented campaigns, increasing the overall efficiency of your strategy and decreasing costs when persuading customers.
Core business processes can be streamlined to improve efficiency
Data analysis can identify areas needing improvement, reduce costs and identify new opportunities. The analytics also positively impact the overall customer experience, resulting in a faster improvement of product quality and sales.
Current market trends are important to be aware of
It can gain a significant competitive edge by collecting data from across industries and customer areas. This allows you to track current and future trends. When choosing a data-driven strategy, it is possible to learn from competitors' mistakes by leveraging data assets.
The longevity of the business increased
Having the right team of data analysts and collectors who can collect and analyze data is important, resulting in valuable and actionable business insights. This allows for better quality products, more informed decisions, and a longer business lifespan.
Security and risk reduction
The right data strategy is crucial in the resolution of fraud cases. It allows the identification of weaknesses, provides a view of all security breaches and opens up a single unified picture. Data management is crucial to settling instant threats.
Why do businesses overlook up to 75% of their data?
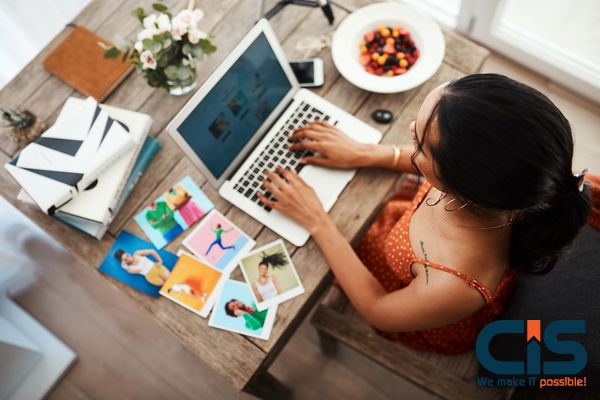
Estimates suggest that 2.5 billion GB of unstructured or semi-structured data are generated daily.
Even data-structured companies are only able to utilize a small fraction of the assets they have. There are many reasons for this, from low data quality to fragmentation and security concerns, then the data itself. Gartner's research shows that 80% of businesses use unstructured and semi-structured data.
There are many reasons. The first reason is that companies cannot cope with the huge amount of data. Another reason is that data comes from different sources, and you can't predict what format the next data will be in. The cost and time involved in handling multi-format databases and sources are prohibitive.
All organizations across industries strive to catch up with big data and gain more visibility. Data-focused companies can leverage only a small fraction of the assets they have. Although the reasons may vary, they are usually related to the quality and nature of the data collected - whether structured, semi-structured, or unstructured.
To manage and protect raw data, companies must choose the best data strategy, work with what they have, classify, and structure it.
How can you use data collecting to help your business grow?
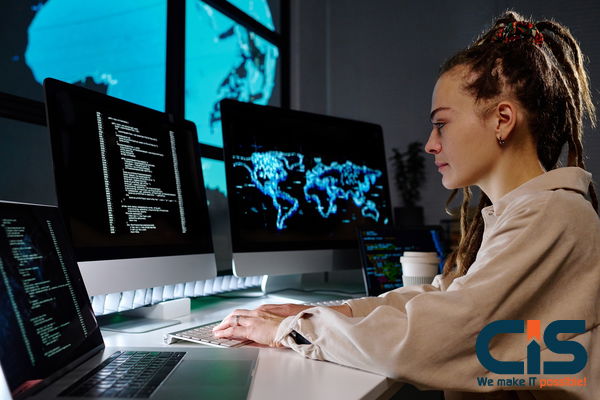
The data collection allows for the analysis of customer needs and pain points. You can then offer your customers exactly what they desire. You can provide the most effective solutions by using data to identify your customer's challenges.
You can also find new customers by studying the behavior patterns of your existing target market.
You can create custom-tailored marketing strategies by leveraging data and improving your conversion rate.
You need to take steps to maximize the efficiency of your data. This includes setting up a data collection system and assigning data owners. Also, you should create an analytics team and address security concerns.
Data strategy helps you to understand the quality of your data.
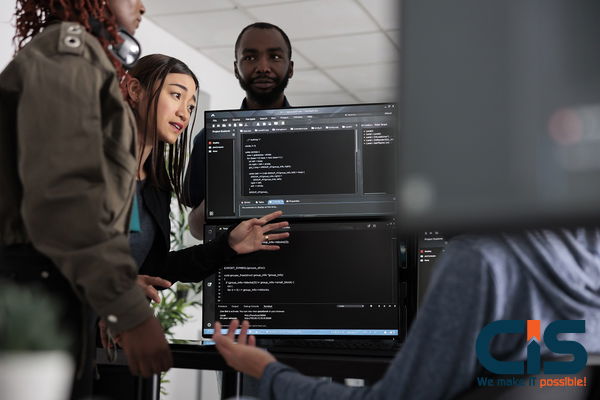
Data has certain characteristics that prove it's of high quality and can be transformed into useful business insight:
- A comprehensive database: Collecting data from different sources makes it possible to get a complete picture of the business.
- Reducing duplicates: Reducing repetitive data allows for a reduction in the number of repetitive analyses.
- Organized Data: The data from different resources must be analyzed using various algorithms. Data that is organized comprehensively will be more easily accessible to the user.
- Fast access to data in real-time: This enables quicker decision-making based on insights derived from data.
- Automation: Consolidates data, avoiding human error and enhancing workflow.
Data can be viewed as an asset and used to extract maximum value from the organization. This value depends on the integration capabilities of the organization.
A good strategy, a set of appropriate tools, a framework for overall integration, and an architecture with layers for optimum data consumption are all necessary.
How to leverage data effectively
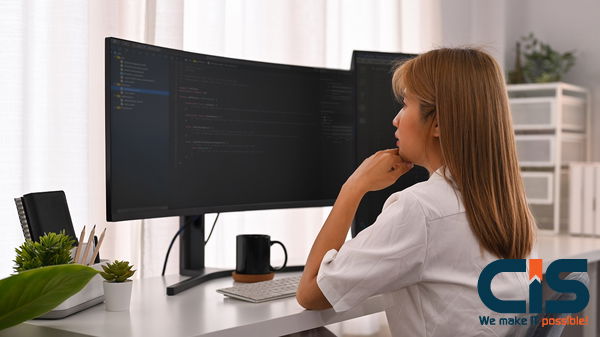
A study has found that organizations with rapid growth use analytics better than slower-growing companies. You need to know who controls your data, what systems are in charge, and how you can enrich and generate useful data by following an efficient overall strategy.
- Analyze your existing datasets and determine if you use them to their full potential. Schedule time to talk with your vendors about their latest platform updates.
- You can share your insights and resources with the other departments.
- Use your data to set specific goals. You can use it to learn about key markets and new initiatives or how to target your customers.
- Make sure you establish specific communication lines between the people who deal with data. It is important to define your goals. This will help you gather the correct data and produce a more effective work product.
- Establish a data governance framework to achieve the quality you expect. Consider data as an issue of business to create an effective governance model that gets more out of data management.
Data leveraging: The most common mistakes
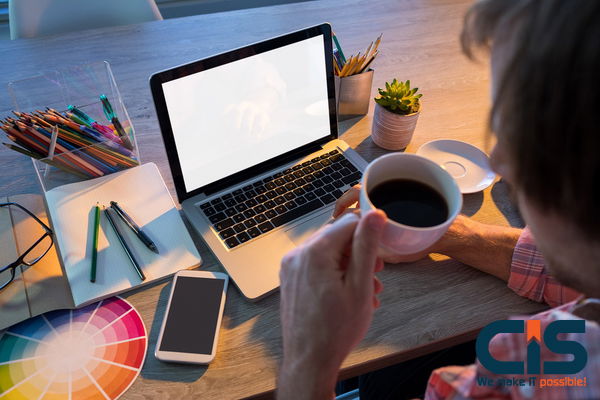
Fail to Address the Fundamentals
Data modes should be validated and aligned with the architecture, and a balance of data consistency and quality must be established. Ownership and access issues and data security and reliability also need to be considered.
It is essential to understand the fundamentals of data management to prevent security issues and costly operations.
Data collection can be overwhelming
Data overload can lead to paralysis in analysis and stalled projects. You must filter out data that produces unclear results or does not support your initiative.
There is no central data collection strategy
Data hygiene is a vital part of any organization. This will help you avoid data errors and low data quality.
Data is stored in silos
Most companies collect large amounts of data but do not use it to its full potential. They let it rot in silos.
Business Intelligence teams are not dedicated enough
It is easy to gather data, but extracting its value is not. A professional team of data managers responsible for managing data acquisition and usage will bring value to your business.
Data architecture is lacking
You need to ensure that you have the right data governance when working with large data sets. You also need to have a data architecture plan that is well-established for the storage of data.
What can Cisin do for you?
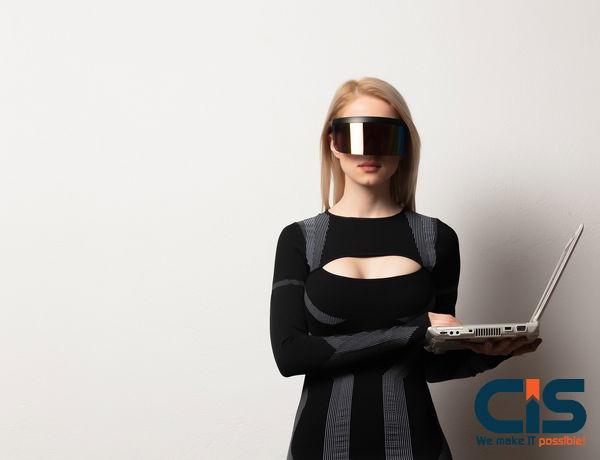
Cisin can help companies maximize the value of their data by leveraging it at every stage. Our experts can help you choose the best architecture, implement the tools, manage the operational side of the tool, and optimize the process.
Our data analysis tools and strategies help companies achieve their goals, improve intelligence and better understand business relationships.
We help you understand and analyze the database structure to generate business value from big data to scale and personalize customer service.
Want More Information About Our Services? Talk to Our Consultants!
The conclusion of the article is:
Few companies succeed in their quest to be more data-driven. There is no way out for the ones who find it. The biggest challenge in leveraging data is finding the best strategy for collecting, refining, segmenting, storing, monitoring and displaying excessive data.
Data can be used to improve business efficiency, predict customer needs, provide relevant products, increase the quality of various aspects, and measure performance.
Using the correct data when making crucial strategic business decisions is important. Expert data analytics teams are highly recommended to capture the relevant data, select the best data strategy, and extract insights.