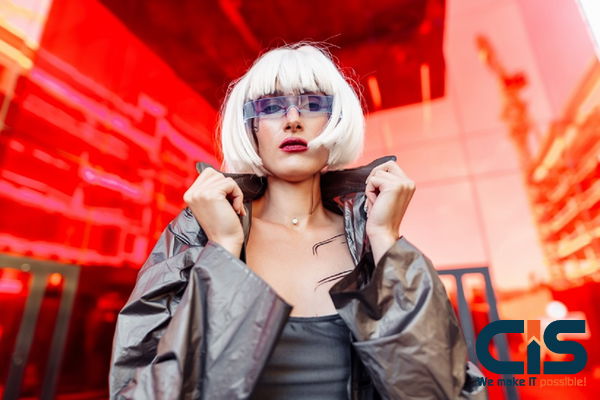
Retail faces problems such as a lack of data security or confidentiality. Further problems are the inability of companies to integrate analytics data into their businesses and the absence of a skilled team to make the necessary conclusions.
Data analytics can help improve customer retention and brand recognition. It provides customer satisfaction by providing the right skills and exact inferences from retailers. As new technologies emerge, data analytics investments may continue to be a critical component of the retail sector.
The retail industry is making significant progress compared to the past two decades.
Online retailers are gaining more customers than physical stores, and online shoppers are stealing their business. The retail industry had previously focused on customer service and marketing. The emphasis now is on data collection, analysis, and improvement of the marketing strategy based on the insights. Data-driven insights are essential for enabling companies to respond quickly.
Retailers can use data science and analytics to transform their data into actionable insights. Retailers can increase brand awareness and customer loyalty through data analytics.
Data Analytics in the Retail Industry
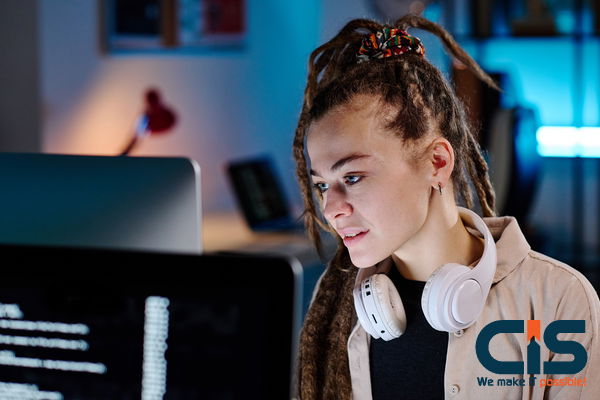
Data analytics has revolutionized brick-and-mortar retail. It has a new way of looking at the industry. It also aims to maximize revenues by optimizing brand strategy, discount coupons, and minimizing inventory loss.
Data analytics can also analyze and understand each store's sales trends and identify its customers' purchase habits. This pattern recognition will allow businesses to stock their stores with the most popular products and promote goods and services. Incentives and promos can be offered to clients to retain them.
Many businesses offer membership plans that allow customers to connect all their online or in-store transactions to one profile. This allows companies to better understand their customers and help them sell.
Retailers should care about Retail Analytics
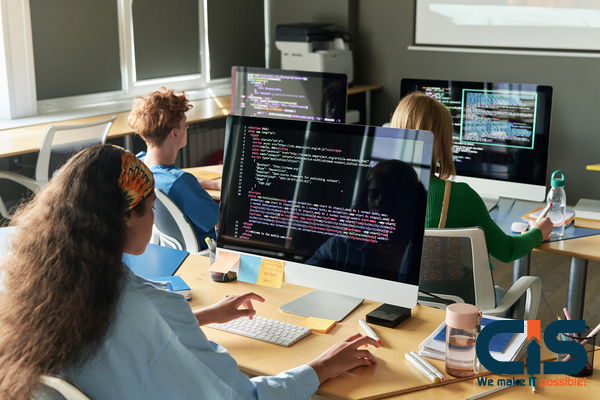
It is really easy. In the digital age, retailers must keep up with millions of things.
Retailers today face a host of new challenges. These include declining sales, fierce competition from online-only shops, and changing consumer preferences. Despite these challenges, many traditional retailers can still grow yearly, smashing previous sales records.
These winners do something different. They are using advanced retail analytics to help them survive and thrive in the rapidly changing retail landscape.
What advanced data analytics are transforming retail?
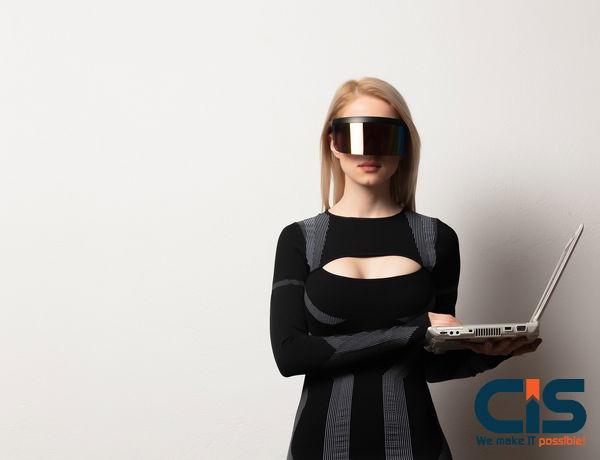
Data analytics is the modern accelerator that has helped business leaders achieve success. Retail analytics is expected to grow 19.1% between 2020 and 2027 to reach USD 23.8 billion.
One thing is certain: data analytics in the retail sector has a bright future. Data analytics plays a major role in the retail sector.
-
Data acquisition
Rewards cards are a popular way to collect big data in the retail industry. It can also be obtained through network connections, financial transactions, customer log-ins, or other methods. Retail companies can use more data to gain actionable insight and predict future purchases.
-
Forecasting spending
Amazon, for example, makes recommendations based on information about customers based on what you have done in the past. Their recommendation algorithm analyzes over 150 million profiles to generate 35% of their sales. This has resulted in significant revenue for the online company.
-
Personalizing the customer experience
Retail data science and advanced analytics offer the opportunity to improve customer relationships. Walmart keeps track of transaction details to keep customers satisfied.
-
Retail demand prediction
Many algorithms consider social media and web browsing habits to forecast future retail market developments. One of the most interesting sample points for forecasting sales is the atmosphere. Pantene, a company that uses the Weather Report to modify product suggestions for customers, took into account climate patterns and used them to make these recommendations. Retailers use retail estimates and commercial predictions to ensure that they are properly deploying their resources throughout the year.
-
Analyzing customer experiences
A consumer's journey is not linear. The cycle of a consumer's journey is not continuous. It spans all channels, from research to purchase. Big data is the only way to improve customer experiences and understand customer behavior. Analytics solutions enable retailers to answer questions such as: Where can shoppers find product pages? What are you doing wrong? How can you approach them to encourage them to purchase?
Want To Know More About Our Services? Talk To Our Consultants!
Why should your Retail Company invest in advanced data analysis?
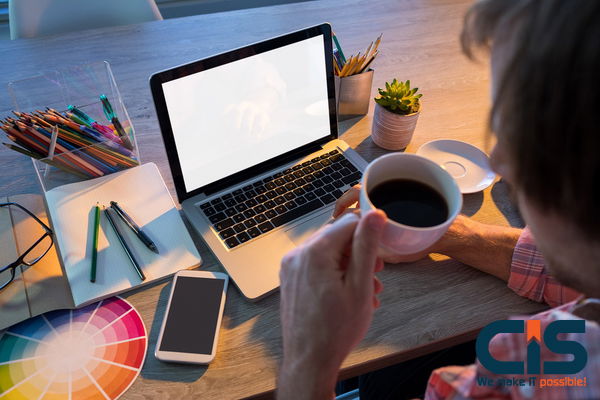
Data analytics in the retail sector offers more than just customer insight. It also provides data about the company's operations and potential improvement opportunities. These are the top reasons retail companies should increase their investment in advanced data analysis.
-
Customer interactions personalized
Personalization can help businesses stand out from their competitors by allowing them to tailor their services.
Data analytics can monitor retail businesses' data at all stages of the buying process. They also track previous transactions. This data can be used to create customized conversations tailored to customers. They are more efficient than traditional marketing methods.
-
Optimizing the price
Pattern recognition can help predict the growth and fall in demand. Predictive research has shown that businesses can increase demand by gradually decreasing the price of a product.
Data visualization solutions can increase customer operational efficiency by up to 30%.
-
Client experience enhanced
Data analytics aims to offer each customer personalized service, from product recommendations to transactional support. Customers stay longer with the company as a result.
Data analytics can also increase customer satisfaction by evaluating what consumers buy together and making suggestions so that customers buy a combination of products at a discount price.
Data analytics algorithms can generate cross-selling sales, which help merchants increase their revenue and improve user satisfaction.
-
Forecasting market trends
Because of their profitability, most brands offer end-of-season or festive deals. Marketers use sentiment analysis to analyze the market's attitude. Data from sophisticated machine learning algorithms can even predict the top-selling products.
-
User loyalty
Data analytics can be used to find customers who aren't engaged with your business but could become long-term or regular customers.
It is easier for retailers to offer customers special rewards and deals to retain and attract customers.
-
Increased ROI
Data analytics investments can help businesses find opportunities with a high investment return. Predictive analysis is a way to assess customer response to marketing campaigns and to determine their likelihood to purchase.
-
Inventory control and forecasting of demand
Retailers that employ data analytics to better understand their customers' needs and highlight high-demand product categories can benefit from this. Businesses can use data-driven conclusions to estimate demand and keep inventories in line.
-
Successful retail spaces
Data visualization can help businesses identify where customers spend most of their attention.
Analytics also provides data about demographics, market conditions, and people's standards of living. This data is very useful in helping clients decide where they want to open a retail store so that they attract the best customers.
-
Data-driven and strategic decision-making
Businesses can rely on data to make informed decisions about their clients and products using a single reliable source of information.
-
Retailers: Data Analytics Strategic Areas
Retail players can identify strategic areas in which they have ready access to data analytics. These are just a few examples of such areas.
1.) Price Optimization
Data analytics is a key component of price determination. Algorithms can perform many functions, such as tracking demand, inventory levels, and competitor activities. They also respond in real-time to market challenges, making it possible to take actions based on the insights. Price optimization is a way to decide when prices should be reduced. This is commonly known as "markdown optimization." Analytics shows that revenue growth can be achieved by reducing prices gradually from the point where demand begins to decline. The US retail Stage Stores proved this. They used experiments to determine the rise and fall in demand for certain products. It beats the traditional end-of-season sale.
Walmart and other retail giants spend millions on merchandising systems in real-time. The goal is to build the world's largest private cloud so millions of transactions can be tracked daily. Algorithms, as well as others, perform this function.
2.) Future performance prediction
This is an important aspect of data analytics in the retail industry. Every customer interaction has a huge impact on both existing and potential relationships. It is risky to share the whole idea with the sales team as a mistake could lead to a loss of income or delay. Top business leaders have found the best way to manage cause and effect between key performance and strategic shift indicators through a test-and-learn approach. Customers or representatives will compare the test group results with those of a closely matched control group. This is data science.
3.) To accommodate small-scale retailers
Small-scale retailers can benefit from the assistance of data platform development that offers data analytics. Some start-ups offer social analytics, which helps increase awareness of products via social media. Small-scale businesses can benefit from data analytics retail without spending too much to not hurt their finances.
4.) Demand prediction
Once retailers clearly understand customer buying patterns, they can focus their efforts on high-demand areas. This involves collecting demographic, occasion-led, and seasonal data to help create an accurate picture of the purchase behavior across target markets. This is very useful for inventory management.
5.) Choose the best Return on Investment (ROI), Opportunities
After understanding potential and current customers deeply, retailers use data-driven insight and predictive risk filters to model expected responses for marketing campaigns. This is based on their propensity or likely buying behavior.
6.) Forecasting trends
Today, retailers have many data visualization tools to help them understand current trends. Forecast trends algorithms use buying data to determine what marketing departments should promote and shouldn't.
7.) Identifying customers
This is important for data analytics retail as it allows you to identify customers who would most likely purchase a product. This is why most retailers rely on online recommendation engine technology and data gathered via transactional records online and loyalty programs offline. Amazon, for example, might not be able to ship products directly to customers' homes before they place orders. However, they are actively looking into this direction. The demand forecasts for each geographic area are based on the demographic information they have about their customers. It means that they can fulfill orders more efficiently and faster. Data gathered about how customers contact retailers are used to decide which path would work best in getting their attention for a product or promotion.
Read More: How Technology Has Led To The Rise Of The Delivery Business
What types of Retail Data Analytics are there?
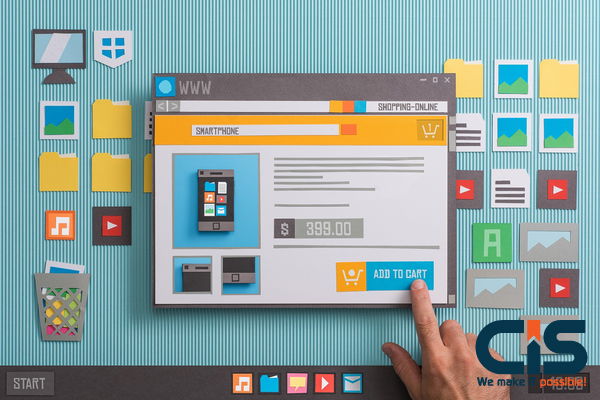
There are four types today of retail data analytics. Each plays an important role in providing retailers with valuable insights into their business operations. These are the four types of retail data analytics.
-
Descriptive Analytics
-
Diagnostic Analytics
-
Predictive Analytics
-
Prescriptive Analytics
Descriptive analytics Definition
This is the most popular type of data analytics. It allows retailers to organize their data in a way that tells a story.
It uses raw data from multiple sources, including POS terminals, inventory systems, OMS, ERPs, and OMS. It can provide valuable insight into past and future performance.
Analysts used Excel to gather data from various sources, format it, chart it, and so on. Many of these data-gathering and reporting tasks can now be automated using power BI migration tools and integrations.
Descriptive analytics simply uses data to show "what" in your business. However, it can't answer the "why" unless combined with other data analytics types that can show patterns or correlations.
Diagnostic Analytics Definition
Diagnostic analytics is the simplest form of "advanced analytics" -- it allows retailers to use data to answer specific business problems.
Diagnostic analytics is based on the same data as descriptive analytics. Still, it uses algorithms and statistical analysis to dig deeper into the data and discover correlations between data points.
Suppose results don't match pre-programmed business rules or benchmarks. In that case, diagnostic analytics can identify anomalies and flag problems as they occur.
The most successful analysts have done all of this manual work for years. They would analyze the data, create statistical models, search for patterns and look for correlations.
This is almost impossible in today's data-rich world. Larger retailers cannot use machine learning and artificial intelligence to effectively analyze diagnostic data because of its sheer volume and complexity.
There are no "diagnostics" tools that retailers on their own can use. Because diagnostic analytics is a fundamental science that identifies hidden relationships between variables within a business, it is better to use this information to predict the future and automate complex analysis.
Predictive Analytics Definition
Predictive analytics is what you do next. While descriptive analytics can show you the "what" and diagnostic analytics the "how" of what's going on in your business, predictive analytics will tell you "what's next."
This is the second-most advanced type of analytics.
Predictive analytics is a combination of descriptive and diagnostic analytics. It uses both to predict the future. To accurately predict the future, it is necessary to understand what has happened and why.
Predictive analytics detects clusters of exceptions automatically and uses complex algorithms to predict future trends.
Many retailers do this manually, just like other types of analytics. Analysts compile data in Excel and apply statistical models to predict future trends.
Retail businesses can be very complicated. Many factors influence each other (demand, price, inventory, product assortments, competitor behavior, etc.). It is impossible for anyone to manually account for them all. Simple sales forecasts are less accurate than demand predictions.
Retail predictive analytics combines AI, advanced mathematics, and intelligent automation. It can accurately predict the future and account for the most important correlations.
Prescriptive Analytics Definition
Prescriptive analytics is the last frontier in analytics and the most advanced.
These analytics tell retailers "what's happening," "why" it happened, and "what's next." Prescriptive analytics tells retailers "what to do next" to achieve the best results.
Prescriptive analytics systems need to predict the future and determine the actions that will result in the best outcome.
This is difficult because there are almost infinite actions that a business can take to make a difference.
There are many ways to do it:
-
Simulations can run on a finite number of initial conditions (different pricing, assortment, etc.). Choosing the best conditions to maximize profit
-
Algorithmic AI is a method that allows retail customers to make recommendations that result in the highest possible mathematical outcome (profit, GMROI, etc.).
-
A machine learning program is used to teach patterns and clusters of actions to produce the best results.
The exact method analytics companies use to achieve this feat is still a secret. The process must generate recommendations that retailers can trust to be followed 99% of the time.
Read More: How the Retail industry is going all in on artificial intelligence
Role of data analytics in the Retail Industry
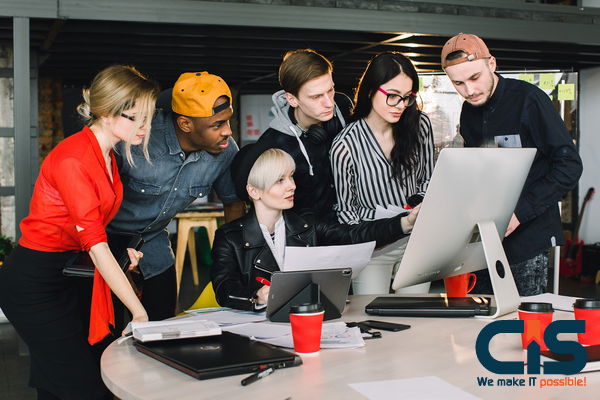
Data analytics also plays a crucial role in other key areas:
a.) Discount Efficiency
Nearly 95% of shoppers admit that they use coupon codes when shopping. To maximize the benefits of offers for retailers, they must first consider how valuable this deal would be to their business. While promotional offers will certainly attract customers, they may not be a good strategy for maintaining long-term customer loyalty. Instead, retailers can analyze historical data and use it to predict the long-term impact of such offers. A team of data scientists and analysts can create a history of events that could have happened without discounts. To better understand how effective each discount is, they compare this to real events that occurred when there were discounts. Once they have this information, the retailer can adjust its discount strategy to increase the number of discounts in different categories or eliminate less profitable deals. This will increase the average monthly revenue.
b.) Reduction in Churn Rate
Brands must create customer loyalty as their number one priority. Attracting new customers is six times more expensive than keeping existing ones. You can calculate churn rates in many ways, including the percentage of customers who have lost their customers, their recurring value, and the value of their recurring business. Big data analytics can provide insights that help retailers determine the best way to modify their subscriptions to avoid churn. A retailer might analyze customer data following a monthly subscription box to find new customers. This would reduce monthly churn and allow brands to calculate lifetime value, which would help them to recover high marketing costs.
c.) Product sell-through rate
Retailers can analyze data-related products to determine what pricing, visuals, and terminology will appeal to potential and current customers. Retailers will increase sales if they alter the product showcase based on the analyzed data sets. Uber's business model relies on big analytics to source crowds and sells through products. Uber uses customers' data to match customers with the best drivers based on their location and ratings. Because of this personalized experience, customers will prefer Uber's offers to regular taxis or competitors.
A US department store giant discovered that it is crucial to attract the right customers to their stores. Their analytics revealed a lack of vital "millennial" demographic groups. The One Below basement was built at their flagship New York store. Promos like "selfie walls" or while-you-wait custom 3D-printed smartphone cases were available. These were all just ideas to attract young customers to the store to provide an amazing experience.
Opportunities in Retail Analytics
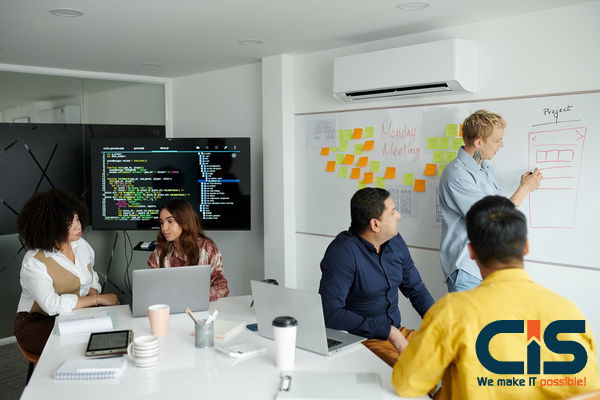
There are also many opportunities for retail analytics.
1.) Big data promises
Retail data grows exponentially yearly in volume, value, and velocity. Smart retailers know that every interaction has the potential to bring in profit. Profit.
According to a 2011 report, big data analytics can help retailers increase their operating margins by up to 60%. The data scientist is responsible for making big data (structured and unstructured, internal or external) clear. This is done to assist retailers in taking actions that increase sales and reduce costs.
2.) Marketing
-
You can create targeted offers by using online behavioral analysis and web analytics.
-
Mobile devices can receive personalized and location-based offers.
-
Targeted campaigns that use analytics to segment consumers identify the best channels and ultimately achieve the highest return on investment.
-
Real-time pricing uses "Second by Second" metrics.
3.) Customer Experience
-
Multi-level reward programs and personalized recommendations based on online data purchases preference, smartphone apps, etc.
-
Sentiment analysis of customer records, social media streams, and product reviews for market insights and customer feedback.
-
Predictive analytics enhances customer experience on all channels and devices, online and offline.
4.) Merchandizing
-
A detailed market basket analysis yields a faster growth rate in revenue.
-
Video data analysis can be used to identify shopping trends and cross-selling possibilities.
-
Daily profits rise through external and internal data like seasonal and holiday trends, economic forecasts, traffic, and weather reports.
5.) Omni-Experience
Everyone involved wants a seamless and streamlined experience. The retailer strives for maximum efficiency in every department, from when the product leaves the manufacturer to the point purchased at the store or warehouse.
Data analytics in the retail industry have made it clear that there have been many operational changes in the retail sector over the years. Big data analytics solutions in the retail industry have made these changes possible. These analytics solutions are rapidly becoming more popular, allowing retailers to work hard for their supply chains to improve, market campaigns, and increase customer satisfaction. This also helps them achieve a high success rate in retailing.
Challenges
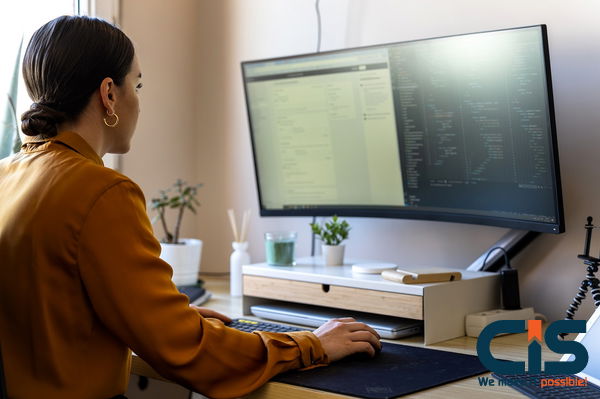
Many issues must be addressed to maximize data analytics in the retail industry. Analytics must be considered in terms of security, privacy, liability policies, and intellectual property. Analytics and big data are interrelated, so professionals with special training would be needed to join the team to use big data analytics.
Companies would also find it useful to include information from different sources, mainly third-party data, and help create an environment conducive to efficient data.
Companies often fall into shortsightedness and fail to implement the insights gained from analytics. This could be corrected by constantly changing retail styles to allow for the implementation of insights.
Want To Know More About Our Services? Talk To Our Consultants!
Conclusion
Retailing is now a platform for more disruption. Data quality has been gathered from multiple sources, including social media conversations and internet purchases. Location-specific smartphone interactions have made it possible to create a new entity for digital-based transactions.
Data analytics can bring organizations many benefits, including improved risk management, performance improvement, and the ability to uncover hidden insights.
Retailers will continue to use data analytics solutions to improve customer loyalty, promoter scores, and brand perception. Retailers and companies can now use data analytics to gain information about their customers and determine how they can reach them. One thing is certain: data analytics will not be left behind as technology continues to dominate the retail industry.