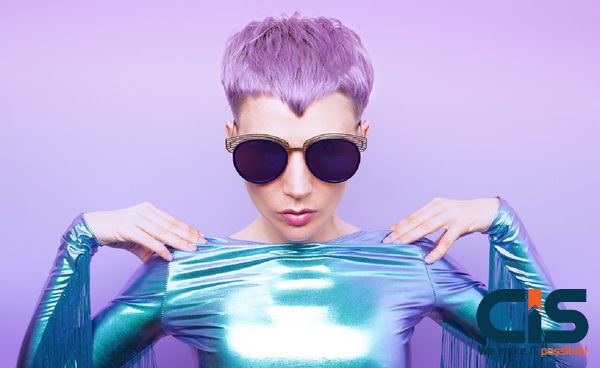
Data preparation for analysis and collection is the responsibility of data engineering. Data flow process automation is a specialty of data engineers. Let's investigate what data engineering is and examine how it could change the big-data ecosystem of a business.
When it comes to data, data engineering is a crucial area. But not everyone is able to explain what data engineers perform. Both large and small businesses depend on data to conduct their operations. Businesses employ data to analyze pertinent questions, such as the viability of a product and consumer interest. Data is crucial for growing your business and finding insightful information. Data Engineering is equally crucial.
In March 2016, over 6,500 LinkedIn members identified themselves as "data engineers." They provided a range of abilities, including expertise in Python, Java, and SQL. Data engineering: What is it? What precisely do data engineers perform? Find out by reading on!
What's Data Engineering?
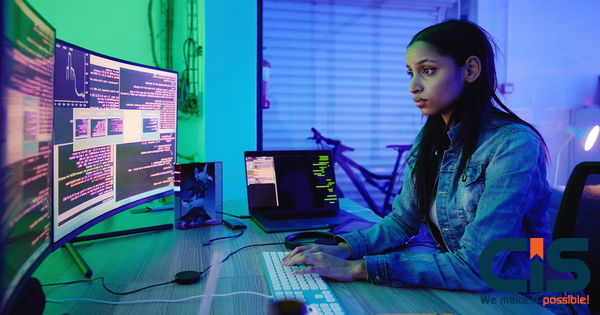
The construction of practical systems that store and gather data from many sources is the essence of data engineering. This might entail making a database more presentable or addressing flaws in it. The foundation of business process management is data engineering. Retail, healthcare, and the financial sectors are just a few of the businesses that might benefit from the adaptability of data engineering.
The "Engineering" part is the most important to comprehend in data engineering. Engineers create and construct things. Data engineers plan and build data pipelines that transform and move data so that Data Scientists and some other end users may use it. These pipelines are in charge of consolidating data from various sources into a single location that can be employed as a single source. Even though it seems straightforward, this job calls for far more data literacy expertise. Data engineers are hard to come by, and the job is poorly understood. An illustration of data engineering activities is shown below.
It consists of a series of operations that are aimed at developing interfaces and building algorithms. Data engineers are committed professionals who put the appropriate database strategies into practice in order to get the data ready for data scientists to analyze. Information engineering is another name for data engineering. It is a strategy for creating software-based information systems. The process of obtaining, modifying, and managing data from various systems is known as data engineering.
This ensures that data is both accessible and helpful. Data engineering places a focus on useful data gathering and processing. As you can see, the questions listed above call for sophisticated answers. Data integration tools and artificial intelligence are only a couple of the sophisticated techniques used in data engineering to gather and verify data. Data engineering also uses complex procedures to apply discovered data to actual circumstances. This includes creating and keeping track of sophisticated processing systems.
What is Data Engineering?
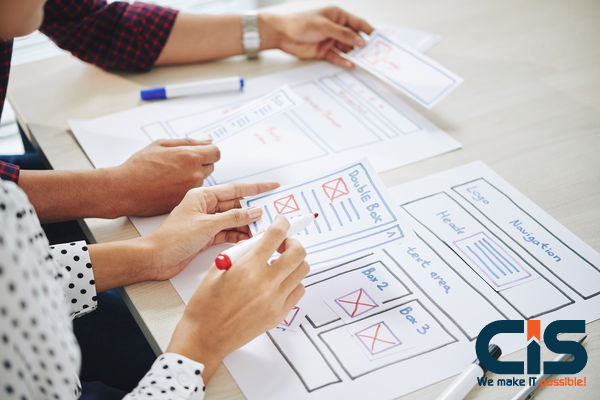
Many would contend that since the introduction of databases, Microsoft SQL Servers, as well as ETL, data engineering has existed for at least ten years. Many would contend that IBM made database management systems mainstream in the 1970s. Here is a quick summary.
Database design was first referred to as "information engineering" in the 1980s. Data analysis also includes software engineering. After the internet's development in the 2000s and 1990s, the term "big data" was developed. However, before then, people who worked in this industry were not known as "Data Engineers," such as DBAs, SQL Developers, and IT specialists.
Let us just sum it up by noting that numerous technology advancements have raised the volume, diversity, and velocity of big data. 2011 saw the debut of the phrase "Data Engineer" by newly data-driven businesses like Facebook as well as Airbnb. These businesses required software engineers to develop tools for processing mountains of real-time data fast and accurately.
The phrase "data engineering" refers to a position that makes use of ETL tools but has created its own tools to handle the expanding amounts of data. Because of big data, a branch of software engineering that concentrates on data infrastructure, data mining, and data processing is now referred to as "data engineering."
Why is Data Engineering So Important?
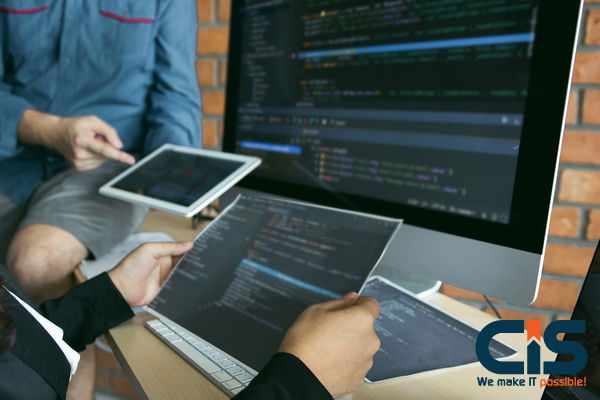
Data engineering is a vital component in the big data era. Companies have access to a variety of information in both the physical and digital worlds. Businesses may benefit greatly from this, but it may also result in information overload. As a result, there is a lack of consistency in the data, which makes it challenging for enterprises to understand their business operations and derive useful insights. In this process, data engineering is crucial.
Data is made more dependable and usable for data scientists by data engineering, which also simplifies the data. By building a data infrastructure, data engineering also enables businesses to profit from data analytics. Every organization needs data engineering and digital automation. For every business entity to last longer, data engineering is crucial. It covers both current operations and future analyses. Even if you might be able to monitor the daily inflow of data, if it isn't understandable, it won't be of any use. The most adept at making it coherent is data engineers. Data engineering is a built-in capability that enables data reading. You can make smarter decisions up to five times faster with the aid of accessible and usable business intelligence. Although data is a crucial component of a business, the idea is not new. However, a lot of individuals do not equate its importance with other roles. Let's examine these differences for a moment.
Data engineering is essential because it enables organizations to make data more usable. Data engineering is essential to the following tasks:
- To enhance your software development lifecycle, find the best practices.
- Boost information security to defend your company against attackers.
- gain more expertise in the business domain
- You can assemble data in one location using data integration technologies.
Whether corporate teams work with sales data or examine lead life cycles, data is present everywhere. Over time, the vitality of data has been significantly impacted by technological advancement. These developments include scalability of data expansion, open source initiatives, and cloud computing. The significance of engineering knowledge in the organization of massive volumes of data is highlighted in the last phrase. It is the responsibility of data engineers to make sure that data is both complete and coherent.
What is The Relationship & Difference Between Data Scientists & Data Engineers?
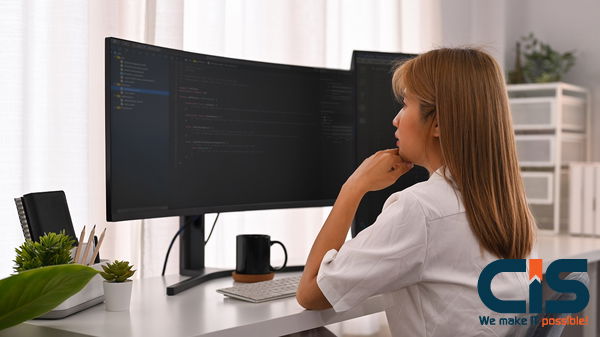
Despite covering a vast range of topics, data engineering, as well as data science, can be viewed as a single software engineering profession. Data engineering's primary component is large data optimization. Big data is a subset of data engineering. It alludes to methods used to handle huge or intricate data sets. A technology-based research company called Gartner discovered that in 2017, 60% to 85% of large projects failed.
The main cause is the use of unreliable data structures. Quality data engineering is more important than ever because of the digital transformation that many businesses believe is unavoidable in the present world. Data engineering was not well-known in the early days of massive data management. Product Teams of data scientists are now able to act as data engineers. This was ineffective. Only exploratory data analysis is taught to data scientists.
Data interpretation falls under the purview of data scientists. Data scientists lack the knowledge necessary to model data in a way that makes sense. Data scientists employ machine learning, statistics, and mathematics to accurately assess an analytics database. Data engineers ensure that the data is initially accessible to data scientists. To accomplish this, data engineers evaluate the quality of the data. If the data is of poor quality, it will be cleaned up to make it better. This is why a sizable amount of the job is database design.
Data engineers and data scientists can both be machine learning engineers. Sometimes, highly skilled data engineers can do the duties of machine learning engineers. We won't get into the specifics of how these jobs relate to one another. A lot has been written. Companies once thought that data scientists could take on the duties of data engineers. This explains the severe shortage as well as the "unicorn effect" in the hiring of data scientists for customers satisfied in software developers for business growth.
Read More:- 12 Key Technologies that Enable Big Data for Businesses
Data Engineers are frequently depicted as being able to handle the duties of a data scientist. Due to the volume and speed of data, data scientist, as well as data engineer are now separate positions, albeit there is some overlap. To enable advanced analytics teams, businesses require both data scientists and engineers. It's exceedingly challenging to conduct useful data science without Data Engineers. Although Data Scientists, as well as Data Engineers, frequently collaborate, their goals and tool knowledge are extremely different.
Advanced analyses of the data produced and kept in a company's database are a specialty of data scientists. Data engineers within an organization control, enhance, and plan the data flow between these databases. A strong foundation in arithmetic, statistics, R, algorithms and machine learning methods will be required of data scientists. SQL, NoSQL, MySQL, cloud technologies, architecture, and frameworks like agile and scrum, in addition to SQL, MySQL NoSQL, and NoSQL, will all be more familiar to data engineers. Both are likely to be knowledgeable in Python, Big Data Visualiazation methods, and other coding languages.
Responsibilities For Data Engineers
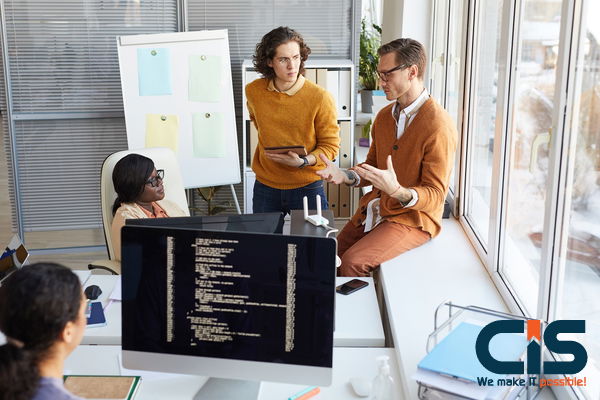
It is now evident that data engineers are in charge of organizing and preparing information for data scientists as a result of our improved understanding of data engineering. A data engineer is primarily responsible for two types of tasks.
Database Management
- Design of the data infrastructure for the generation, transmission, and storage of data
- Data accessibility and privacy
- Designing efficient pipelines
- Accurate reporting platforms and data warehouses
Data Insights
- Data analysis and development tools
- Algorithms for machine learning are being developed.
- Collaborating with engineers and data scientists to achieve business objectives
A skilled data engineer will ensure that your final data are accurate, relevant, reliable, and ready to be used.
What Data Engineering Jobs Are In Demand?
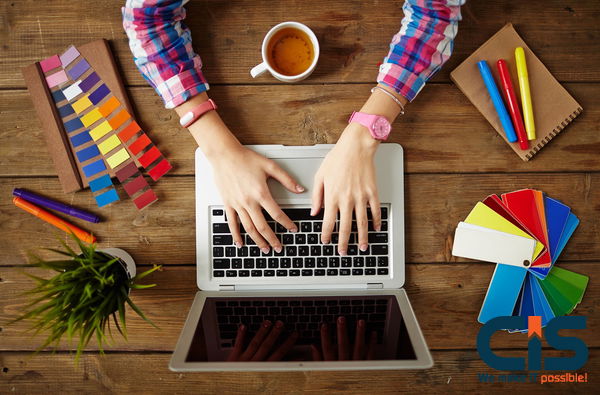
Data Engineering is not always an entry-level position. Before moving into managerial roles, data engineers frequently begin their careers as software engineers as well as business intelligence analysts. Some of the most popular job titles throughout the field of data engineering include data architect, big data, cloud data engineer, machine learning (ML), data warehouse manager, technical architect, data warehouse technician, solutions administrator, as well as extract, transform, load (ETL) developer. A highly specialized topic of study is data engineering. It is crucial that these IT workers have both real-world experience and academic knowledge because of this.
What Are Data Engineering Technologies?
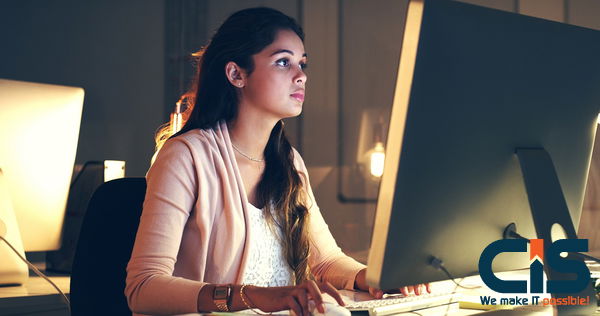
To gather, analyze, manage, analyze, and visualize massive data sets within the firm, data engineers employ a variety of data engineering tools and techniques. These consist of Engineering Lifecycle Management, Logilica Insights, as well as Data band, Logilica Insights, Stitch, Tableau, and Allstacks.
Data engineers must consider certain criteria before they decide to use a particular data engineering tool, such as.
- User interface
- Integrity and adaptability
- Usability
- worth the money
- Time to set up
- Compatibility of programming languages
Why is Data Engineering Critical For Digital Transformation?
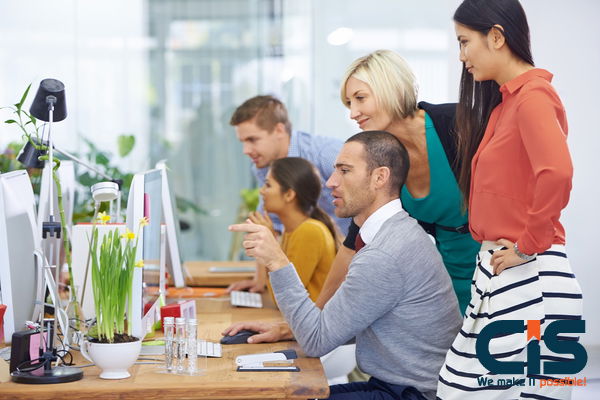
Due to the increasing evolution of data in contemporary industry, data engineering has emerged as a crucial growth instrument. When working with a lot of data, especially digital data, an organization needs automation that may truly be helpful. A fantastic tool for this and many other things is data engineering.
This is how it goes: A crucial element of the digital transition is data quality. Data engineering experts contribute to data separation and efficiency. It's likely that everything, from operations to analytics, will be improved with more emphasis. This necessitates upgrades to systems, infrastructure, and data architecture. Data engineering helps to facilitate these changes and meet business needs by creating efficient data pipelines.
What is A Data Engineer?
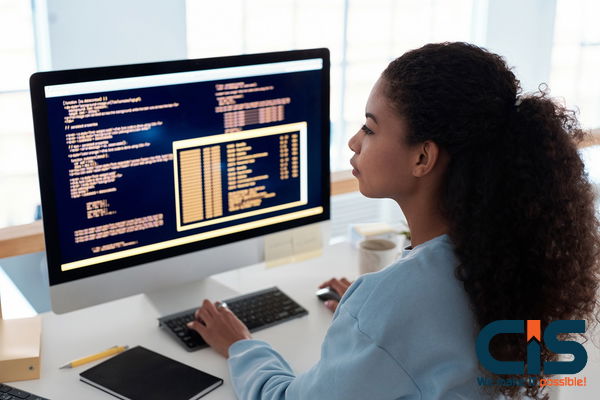
Experts with database architecture design include data engineers. For operational use, they develop analytics databases and data pipelines. They must prepare a lot of data and make sure the data flows are efficient as part of their job. Data scientists can execute queries for machine learning, prescriptive analytics, and data mining using the algorithms and datasets that data engineers have built. Data formatting for both organized and unstructured data is a part of the work. It is possible to include unstructured data in databases. Text, photos, music, and video can all be found in unstructured data, which traditional data models can not recognize. Data engineers need to be able to work with a variety of data assembling formats and techniques.
What is The Role of a Data Engineer?
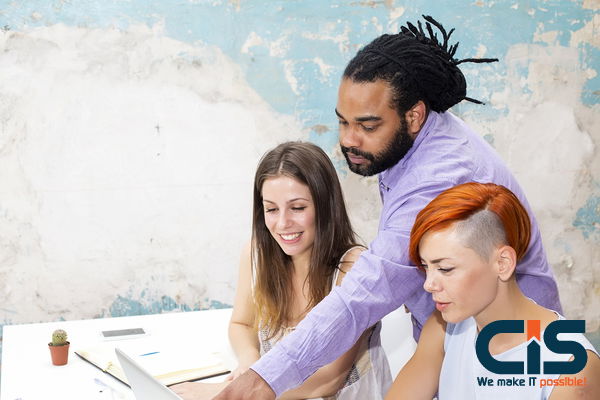
Data engineering encompasses many different roles. Here's a quick breakdown:
Generalist Data Engineers
A generalist data engineer does end-to-end data collection while working in small teams. Compared to other categories of engineers, generalists are more skilled but less familiar with system architecture. Generalists are less anxious about undertaking complicated jobs because small teams don't have very many users. They continue to play a wide range of roles.
Pipeline-Centric Data Engineers
Pipeline centric data analysts are more likely to be employed by larger and mid-sized businesses than by smaller ones. A data flow that combines data from various sources is called a data pipeline. To finish complicated data science tasks, pipeline-centric data engineering must collaborate with numerous systems.
Database-Centric Data Engineers
Large companies depend on database-centric data experts to manage data distributed across multiple databases. Analytics databases are the main focus of database-centric engineers. They collaborate across data warehouses to create table schemas and work closely with data scientists.
How Do Data Scientists And Data Engineers Co-Work?
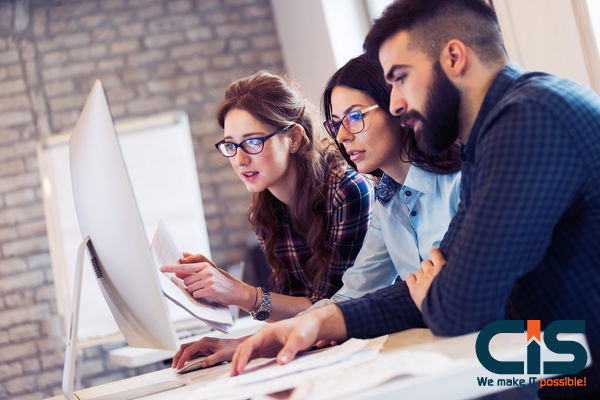
Using the comparison between civil engineers, architects, as well as civil engineers, it is simple to comprehend the functions of both civil engineers and architects. While civil engineers are in charge of implementing these designs, architects are in charge of developing the initial plans. Similar to the data world, data scientists are all in charge of creating the first blueprints, and civil engineers are in charge of putting them into action.
To deliver business solutions, both must collaborate to effectively integrate and simplify data. A data engineer will figure out how to include. Predictive Modelling that processes data that a data scientist has created into the broader data processing process. To consolidate ideas, both experts must be able to communicate effectively.
What Skills are Data Engineers Looking For?
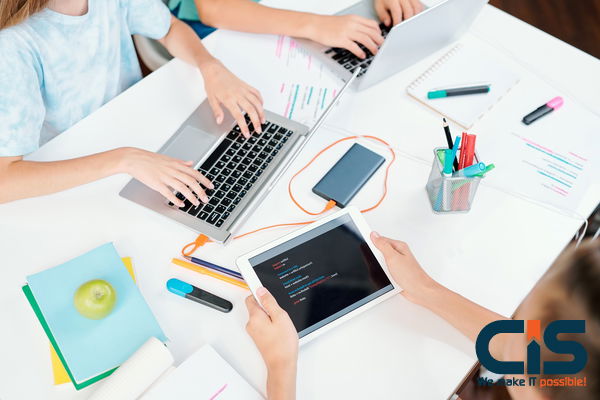
Let's examine data engineering in more detail now. Database analyses are carried out by data engineers using the software. This is why having a background in programming as well as software development is crucial. They should be competent in Python, Java, and Scala, among other programming languages. Additionally, they must be familiar with technological tools like Hadoop, Spark, SQL database architecture, and Hive. The most in-demand skills among data engineers are those related to designing, building, and managing data warehouses. Data architecture and pipelining are two topics that a competent data engineer has to be knowledgeable about, both conceptually and practically. Despite the fact that they are officially software engineers, data engineers are capable of far more than just programming.
In order to build software solutions around data, data engineers need to possess specialized expertise. In addition, it may be impractical to expect Data Engineers to be knowledgeable in more than a handful of tools and technologies-between 10 and 30. Additionally, these tools are always evolving. Additionally, it differs by industry. Some have existed forever, like SQL. Others, like Scala, are gradually losing popularity. Still others, like AWS, are seeing a sharp increase in demand. The most in-demand skills for data engineers on three employment platforms were recently analyzed by a published author and educator on data science and data engineering issues. His list of the top ten technological abilities needed is shown below.
It is quite tough to choose the correct person for the position because of the wide range of talents required and the intricacy of certain of them. Over the past few years, the qualifications needed to perform the duties of a data engineer have increased. For this reason, we advise that when it comes to data science, it's ideal to think of a "Data Engineer" as a group of individuals who have a variety of data engineering talents. Various factors will determine which ones you should give priority to.
The tools and duties that data engineers are required to be proficient in order to do their work are listed below.
ETL Tools
Extract, Transform, and Load is referred to as ETL. These technologies enable data integration through a set of tools. Traditional ETL tools have largely been supplanted in the modern era by platforms for low-code development. However, the ETL process continues to rule data engineering. Two of the most commonly used tools for doing this are Informatica as well as SAP Data Services.
Programming Languages
A variety of programming languages are needed for data engineering. Back-end languages, query languages, and particular languages for statistical computation are some of them. Some of the most popular programming languages regarding data engineering are Python, Ruby, and Java.
With its extensive library, Python is a general-purpose programming language. The language is adaptable and strong enough to be utilized for ETL jobs. Structured query language can also be used to carry out ETL activities (SQL). It is the most used language for executing queries against relational database tables, which is obviously a significant portion of data engineering. The most widely used software environment and programming language for statistical computation are R. Data miners and statisticians like it very much.
APIs
Any project involving the integration of data, including data engineering, must use app programming interfaces (APIs). APIs are necessary for any software engineering project. They are utilized for data transport and application connectivity. The use of REST APIs is crucial for data engineering. REST APIs commonly referred to as representational state transfer APIs, support HTTP communication. They become a priceless asset to just about any web-based tool as a result.
Data Warehouses & Data Lakes
Organizations store massive, complicated datasets in data lakes and warehouses to give business intelligence. Business analysts using computer clusters and information technology driven by businesses manage these datasets. You can use this computer network to aid you with more problems. Both of the most popular big data platforms, Spark and Hadoop, are accessible. Large data collections are prepared and processed using these frameworks. For tasks involving a lot of data, such as data mining and information analysis, each depends on computer clusters.
What Are Data Engineering Services?
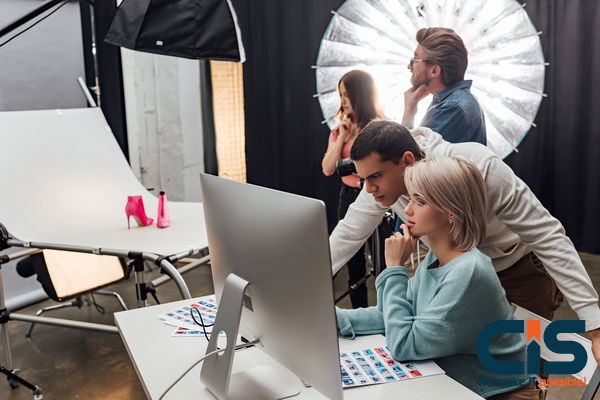
Your business can advance in data management, data use, and data automation with the aid of a company that provides data engineering services. Now that you are leveraging automated advanced data pipelines, you can concentrate on gaining insight.
Some of the services offered by data engineering include
- End-to-end complete data pipelines
- consuming data from many sources and sending it to desired locations
- Converting files in different formats
- Data Transformations
- Data Cleansing
- Data integrity:
- Create Data Models
- Carrying out ETL or ELT jobs
- Adding information to data for later analysis
- Data Analytics
- Performance tuning
Data Engineering: Is It a Good Career?
The research in 2022 discoverad that the need for data engineers increased year over year. It also outpaced data scientists as the tech industry's fastest-growing profession. As long as businesses need data to make strategic decisions and derive insights, there will always be a need for data engineers.
Rapid digital transformations, especially those following the epidemic, are to blame for the data explosion. Data engineers are now more in demand as a result of this. Data engineers are employed by a number of well-known tech organizations, including Capital One, Accenture, Amazon, and Accenture. Researchers have found that data engineers are well-paid, with annual salaries exceeding around $110,000.
Integrating data engineering techniques will probably be the solution if your objective is to address a complicated business problem. The future of business is digital automation as well as data-driven understanding. It won't be long before every organization understands how to use data wisely and realize its full business potential.
Data Engineering is Critical For Business
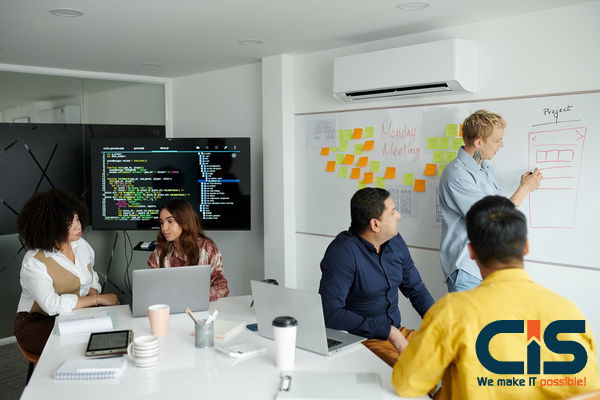
Data engineering is essential to achieving practically all corporate objectives. Data engineers can prepare and analyze data for upcoming studies using a range of techniques and equipment. If data cannot be read, it is of no use. Making data useful requires first performing data engineering. Data engineering is important for the scalability of businesses, as acknowledged by CISIN. We have highly skilled data engineers available to assist you in growing your business.
85% of big data projects fail, according to a researcher in a 2017 analysis, which you've certainly heard about or read. This was mostly brought on by the absence of trustworthy data infrastructures. Data could not be trusted enough to support important business choices. Since 2019, nothing has changed. The CTO estimates that 87% of data-science projects never reach production. Reiterating his earlier prediction that only 80% of programmes would fail, the researcher said. The New Vantage Report presented comparable statistics.
In the last ten years, the majority of businesses have undergone a digital transition. These changes have generated an enormous volume of data, as well as more sophisticated data more quickly. Although it was clear that Data Scientists were needed to interpret this data, it was not immediately apparent who would have to organize, secure, and make the data available so that Data Scientists could do their work.
In the early days of Big Data Analytics, data scientists were frequently needed to build the infrastructure as well as pipelines required to carry out their work. Their job description and skill set did not include this. It was improperly done to model the data. Data scientists would be required to perform duplicate tasks and make use of erroneous information. These challenges make it challenging for businesses to maximize the benefits of existing data projects. Companies eventually failed because they couldn't. This led to high turnover rates for Data Scientists, which still exist today.
It is evident that businesses need a lot of Data Engineers to ensure the success of their data science activities given the abundance of successful corporate digital transformations and the emergence of the Internet of Things. Data engineers will become more and more significant. To extract value from data, businesses require teams of individuals who are dedicated to doing so.
Bottom Line
You may swiftly find solutions to challenging business problems with data engineering. With the use of digital technology and artificial intelligence, CISIN Technologies can process massive datasets more quickly while preserving accuracy. This makes it possible for all interested parties to have a better grasp of how the company is doing and to come to wiser judgments that will ultimately result in success. Our data developers and engineers are experienced in assisting small businesses in making improvements to their operations and maximizing the use of their data management. We think it's only a matter of time before every company discovers how to make the most of its data to optimize business strategy and address issues.