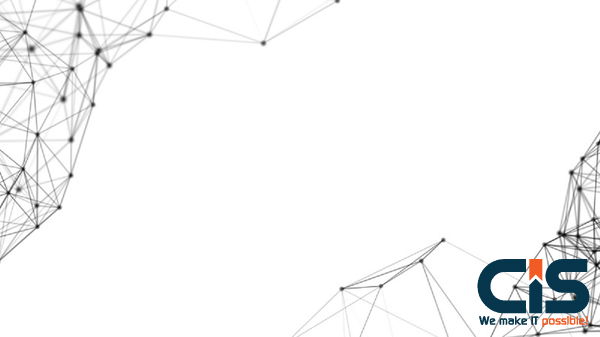
The current market dynamics do not allow for such a slowdown. Amazon and Google are using AI technology to disrupt traditional business models. Laggards must reimagine their business to stay competitive. Cloud providers are launching cutting-edge products, like serverless Best Big data analytics platforms, that can be instantly deployed, giving adopters a quicker time to market. Analytics users demand more seamless tools, such as automated platform deployment, to use new models. Many organizations have adopted APIs to integrate data from disparate sources into their data lakes and quickly integrate insights. As companies prepare for the new normal and navigate the COVID-19 pandemic, they must be flexible and fast.
To maintain or build a competitive advantage, companies must adopt a new way of defining, implementing and integrating data stacks. They can leverage cloud computing (beyond Infrastructure as a Service) and other new concepts and components.
What Is Enterprise Data Architecture?
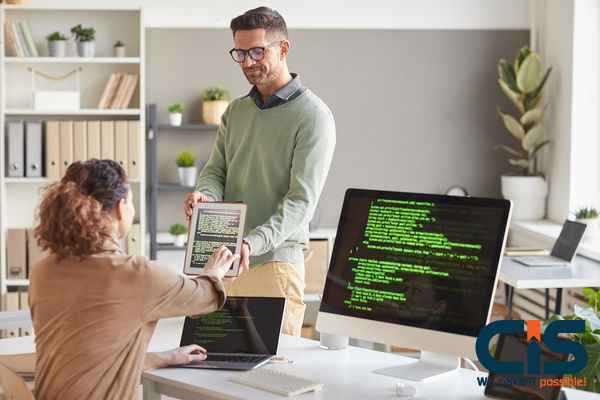
Here is a brief definition of enterprise data architecture:
- Enterprise Data Architecture (EDA) is a set of policies that defines how your organization collects, integrates and uses data.
- Dataversity says that enterprise data architectures aim to keep the supporting data framework clean, auditable, and consistent.
- It's not just a list of rules; it's discipline.
Data architecture, designed and managed by your enterprise's data teams, standardized data management processes. It helps maintain high availability, quality and governance. This ensures a constant stream of organized, reliable and consistent data available at all times for business insights. Solid architecture helps bridge the gap between technical teams and business strategists, allowing them to work together toward long-term organizational goals.
Enterprise data architecture is a familiar idea. It is used by many organizations for their internal infrastructures and on-premise systems. However, These outdated systems must be more flexible and adapt to changing needs. These systems require high maintenance costs and financial investments, resulting in low returns.
Data Standards
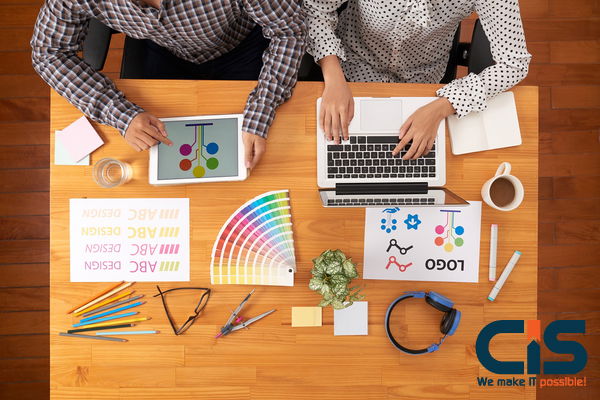
Data standards are the standards that govern a data architecture. They apply to data schemas, security and other areas.
Data Schemas
The architecture sets data standards and defines what data types will be passed through. A data schema can help achieve these standards. The data schema is defined as:
- Each entity should be collected. Contact information should include name, email address, phone number and workplace.
- Each piece of data should have a different type. Name is text; email is text, and phone number is an integer.
- Relationships between the entity and other entities in the database. For example, where it came from and where it is going.
Data schema will be updated by most companies. Companies will use relational databases instead of traditional SQL databases as data becomes more prevalent. NoSQL (relational) databases let you easily add data and piece it together like a network rather than a hierarchy. These relational databases can grow larger and handle dynamic data additions, which traditional SQL databases cannot (or were strongly advised against).
Versioning is vital. Versioning data schemas helps standardize:
- Where to look for what.
- Ability to ask where data is.
Data Security
The data standards help to set security rules in the architecture. The architecture and schema can show how data is protected when traveling from A to B.
The following are examples of security protocols:
- Encrypting data during travel.
- Restricted access to individuals.
- The anonymization of data reduces the value received by the receiving party.
- Additional Actions.
New Architecture
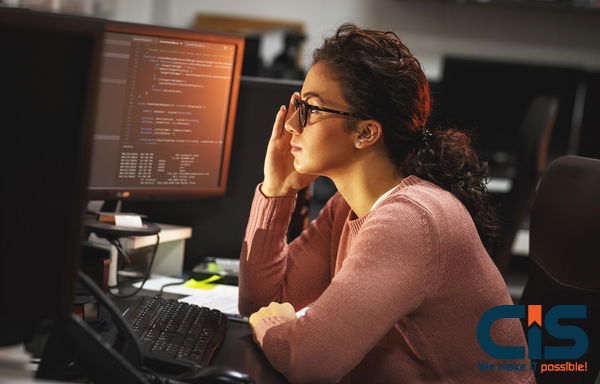
This is the six changes:
- Cloud-based platforms replace on-premise data platforms.
- Data processing from batch to real-time.
- Commercial solutions pre-integrated to modular platforms of the best-in-class.
- Decoupled data access from point-to-point.
- Domain-based architecture from an enterprise warehouse.
- Flexible data schemas are the future of rigid data models.
It would be best if you always thought about the data architecture when thinking of anything related to data, which is probably everything.
Modern Approach
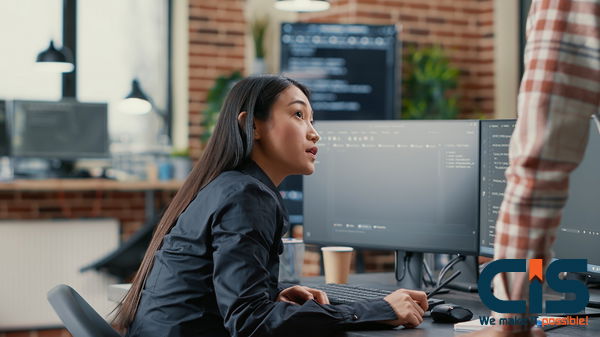
Modern enterprise data architecture (MEDA) takes the principles of traditional architectures and applies them to complex big data analytics requirements. MEDA focuses on flexibility and scalability to help you overcome the limitations of traditional systems.
You can use these principles to design your MEDA:
- Centralize Your Data Management: Data silos cause problems. MEDA replaces your silos with a central system. This allows you to increase your data visibility throughout your organization and correlate data across different business functions.
- Restrict Your Data Movement: Data movement in traditional architecture is time-consuming, costly and can lead to errors. MEDA limits data movement by supporting parallel processing across multiple workloads. It optimizes costs and reduces errors.
- Curate Your Data: Data curation can have a variety of meanings. But, it is about managing data within your organization and connecting stakeholders from different departments. Data curation includes a variety of tasks, including cleaning raw data and transforming it, as well as setting data dimensions. By designing your MEDA in this way, you can unlock the full potential of your shared information and improve the user experience.
- Create A Common Vocabulary: Regarding data, it's useless if people speak different languages. MEDA ensures that definitions for data are consistent across the enterprise and are understandable by all users. This reduces disputes and ensures that your teams are on the same page.
By following these principles, you can create a solid architecture that will benefit everyone in your company, not just the data teams. It would be best to keep a few things in mind to get the most from MEDA.
Want More Information About Our Services? Talk to Our Consultants!
Six Steps To Create A Data Architecture That Will Change The Game
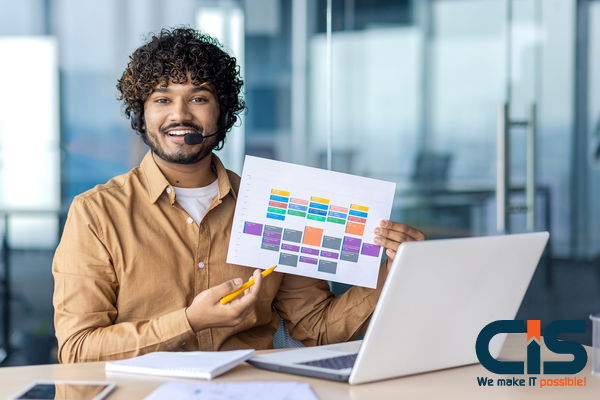
We have identified six fundamental shifts in data architecture blueprints that companies are making. These enable them to deliver new capabilities faster and simplify their existing architectural approaches. These changes affect almost all data-related activities, including acquisition, processing and storage, analysis and exposure. While some organizations may be able to implement certain shifts without affecting their core technology stack, others require a careful re-architecture of the existing infrastructure and data platform, which includes both legacy and newer technologies that were previously added.
These efforts are significant. The investments can range from tens to hundreds of million dollars, depending on the capabilities needed to meet basic needs, like automated reporting. It is, therefore, important for organizations to develop a strategic plan. Data and technology leaders must make bold decisions to prioritize changes that impact business goals and invest in the appropriate level of sophistication. Data-architecture blueprints can look different in each company.
If done correctly, the return on investments can be substantial (more than 500 million dollars annually for one US bank and 12 to 15% profit growth for one oil and Gas company). These benefits can be derived from various sources: IT cost reductions, productivity gains, reduced operational and regulatory risk, or the creation of entirely new services, capabilities, and businesses.
What are the key changes that organizations should consider?
Cloud-Based Platforms Replace On-Premise Data Platforms
Cloud computing is the main driver for a new approach to data architecture because it allows companies to scale AI capabilities and tools quickly. This gives them a competitive edge. Cloud providers like Amazon (with Amazon Web Services), Google Cloud Platform, or Microsoft Azure have revolutionized how organizations of all sizes procure, deploy and operate data infrastructure, platforms and applications.
For example, a utility services company combined a cloud data platform with container technologies, which hold microservices, such as searching for billing data or adding properties to an account, in order to modularize the application capabilities. The company delivered new self-service features to around 100,000 business clients in days rather than months. It also delivered large amounts of real-time inventory and transactional data to users for analytics.
Enabling concepts, components and elements:
- Serverless data platforms such as Amazon S3 or Google BigQuery allow organizations to create and run data-centric apps with unlimited scale without installing and configuring solutions or managing workloads. These offerings reduce the expertise needed, can speed up deployments from weeks to minutes and require almost no operational overhead.
- Containerized data solutions based on Kubernetes, available from cloud providers and open source and that can be quickly integrated and deployed, allow companies to automate the deployment of additional compute and storage systems. This is especially useful for data platforms that require complex setups, such as those that need to retain data between application sessions and those requiring backup and recovery.
Data Processing: From Batch To Real-Time
Real-time messaging and streaming costs have dropped significantly, opening the door to mainstream adoption. These technologies enable new business applications: transportation companies, for instance, can inform customers as their taxi approaches with accurate-to-the-second arrival predictions; insurance companies can analyze real-time behavioral data from smart devices to individualize rates; and manufacturers can predict infrastructure issues based on real-time sensor data.
Data consumers, such as data marts or data-driven staff, can subscribe to real-time streaming features, like a subscription mechanism. This allows them to receive a constant stream of transactions that they require. Common data lakes are often used as the "brains" of such services. They retain all transactions.
Concepts and components:
- Apache Kafka, for example, provides a publish/ subscribe service that is scalable, fault-tolerant, and durable. It can store and process millions of messages per second, either immediately or at a later date. This allows real-time applications to be supported while bypassing batch-based solutions. It also has a smaller footprint (and lower cost) than traditional enterprise message queues.
- Streaming analytics and processing solutions, such as Apache Kafka Streaming, Apache Flume, Apache Storm and Apache Spark Streaming, allow direct analysis of messages. The analysis can be based on rules or use advanced analytics to extract signals or events from the data. Analysis often integrates historical data to compare patterns. This is particularly important in recommendation engines and prediction engines.
- Alerting platforms like Graphite and Splunk allow users to take business actions, such as letting sales reps know if they are not meeting daily sales targets. They can also integrate these actions with existing processes, which may be run by enterprise resource planning systems (ERPs) or CRMs.
Read More:
How Much Does Developing An Augmented Reality App Cost?
Commercial Solutions Pre-Integrated To Modular Platforms Of The Best-Of-Breed
To scale their applications, many companies need to go beyond the limits of the legacy data ecosystems provided by large solution providers. Many companies are moving towards a modular data architecture using open-source and best-of-breed components.
This utility services company is implementing this new approach to deliver data-intensive digital services at scale to millions of customers and to connect cloud applications. It offers, for example, accurate daily views of customer energy consumption and real-time insights into comparing individual consumption to peer groups. The company created an independent data layer, including commercial databases and open-source components. Data is synchronized with the back-end system via proprietary enterprise service buses, and microservices in containers run the business logic using data.
Enabling concepts, components and elements:
- API interfaces and data pipelines are designed to simplify the integration of disparate platforms and tools. They shield the data teams from complexity and speed up time-to-market while reducing the risk of creating new problems within existing applications. These interfaces allow components to be replaced more easily as the requirements change.
- Workbenches for analytics, such as Amazon Sagemaker or Kubeflow, make end-to-end building applications in a modular architecture easy. These tools can connect with many databases and services and can be designed highly modularly.
Hire big data developers from CISIN to get dedicated employees for your projects.
Decoupled Data Access From Point-To-Point
Data can be exposed via APIs to ensure limited access and security and to provide faster and more current access to data sets. Data can be reused easily among teams. This speeds up access and allows seamless collaboration between analytics teams to develop AI use cases more efficiently.
A pharmaceutical company is, for instance, creating an internal "data market" via APIs for all employees to standardize and simplify access to core data assets rather than relying upon proprietary interfaces. For 18 months, the company will gradually migrate its most valuable data feeds into an API-based system and deploy an API management platform to expose these APIs to users.
Enabling concepts, components and elements:
- It is essential to have an API management platform, also known as an API gateway, to publish data-centric interfaces, control access and measure usage. The platform allows users and developers to find and reuse existing data interfaces instead of building new ones and searching for them. A data hub often includes an API gateway as a separate area. Still, it can be created as a stand-alone capability.
- A data platform often needs to "buffer transactions" outside core systems. These buffers can be provided by central platforms, such as data lakes or distributed data meshes. A distributed data mesh is an ecosystem of platforms best suited to each business domain, including data lakes, warehouses and so forth. One bank, for example, built a columnar database to deliver customer information, such as the most recent financial transactions, directly to its online and mobile banking apps and reduce expensive workloads on their mainframe.
Domain-Based Architecture: From An Enterprise Warehouse
Data-architecture leaders are moving away from central enterprise data lakes to "domain-driven designs" that can be tailored and "fit for purpose, " allowing them to reduce the time it takes to launch new data products and services. While the data may reside on the same platform, the "product owners" of each business domain are responsible for organizing the data in a way that is easily consumable for both users in their domain and downstream data consumers from other business domains. It is important to maintain a balance to avoid fragmentation and inefficiency. However, this approach can save time by reducing the time needed to build new data models in the lake.
A European telecoms provider implemented a distributed domain architecture to allow sales and operations staff to expose customer, billing, and order data to data scientists to be used in AI models or to communicate directly with customers via digital channels. Instead of building a central data platform, this organization used logical platforms managed by product owners in the company's operations and sales teams. Product owners are rewarded for promoting the use of data analytics and using digital channels, hackathons, and forums to encourage adoption.
Enabling concepts, components and elements:
- Data infrastructure is a platform that provides tools and capabilities to store and manage data assets. This helps speed up implementation and relieves data producers of the burden of creating their platform.
- Data virtualization techniques are being used by enterprises to integrate and organize distributed data assets. They began in niche areas, such as customer data.
- Data cataloging software allows for enterprise-wide data search and exploration without the need to prepare or access all of the data. A catalog will also provide metadata definitions and an end-to-end interface for data assets.
Flexible Data Schemas Are The Future Of Rigid Data Models
Predefined data models from software vendors and proprietary data models that serve specific business intelligence needs are often built in highly normalized schemas with rigid database tables and data elements to minimize redundancy. This approach is the most common for regulatory and reporting use cases. However, organizations must have a strong understanding of their systems and undergo long development cycles to include new data sources or elements.
Companies are adopting "schema light" approaches to gain greater flexibility when exploring data and supporting advanced analytics. They use a denormalized data model with fewer physical tables to organize the data for maximum performance. This approach has several advantages: it allows for agile data exploration and greater flexibility when storing unstructured and structured data. It also reduces complexity because data leaders don't need additional abstraction layers, such as multiple "joins" between highly normalized tables to query relational information.
Enabling concepts, components and elements:
- Data vault 2.0 techniques, such as data point modeling, can ensure data models can be extended in the future so that data elements can easily be added or removed.
- In recent years, graph databases, a form of NoSQL, have attracted much attention. NoSQL databases are ideal for digital applications requiring massive scalability, real-time capabilities and data layers for AI applications. Many companies use graph databases to build master data repositories that accommodate ever-changing information models.
- Azure Synapse analytics, for example, allows users to query file-based data similarly to relational databases. This is done by applying dynamic table structures on the files. Users can continue using common interfaces, such as SQL while gaining access to file data.
- Using JavaScript Object Notation to store information allows organizations to change database structures without changing business information models.
How To Get Started
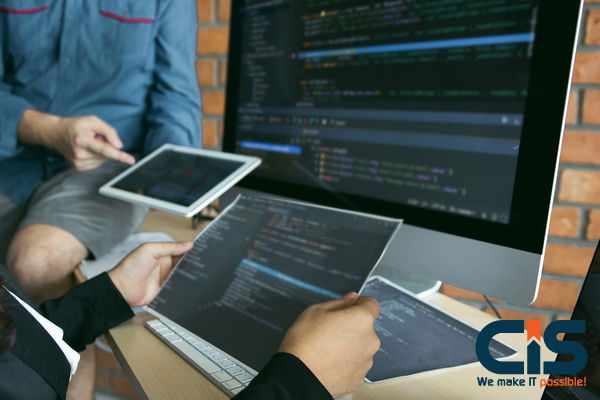
Data technologies are changing rapidly, which makes traditional efforts to define and build towards three-to-five-year architectural target states risky and inefficient. Leaders in data and technology will benefit from adopting practices that allow them to quickly evaluate and deploy new technological solutions so they can adapt. Here, four practices are essential:
- When building architectures, Use a test-and-learn mindset, and experiment with various components and concepts. Agile practices have been used in the application development space for a long time and recently have moved to data. Instead of engaging in lengthy discussions about the optimal designs, products and vendors to identify the "perfect choice", followed by lengthy budget approvals. Leaders can start with smaller funds and create minimal viable products or string existing open-source software to create an interim solution before releasing it into production.
- Create data "tribes" where teams of data stewards, engineers, and data modelers work together to build the data architecture. These tribes work on standardizing and repeating data-and-feature-engineering procedures to support the big data development of highly-curated data sets that are ready for modeling. These agile data practices accelerate the time-to-market of new data services.
- Investing in DataOps, or enhanced DevOps, can help teams implement solutions quickly and update them frequently based on feedback.
- Create a culture of data where employees are excited to apply and use new data services in their roles. To achieve this, it is important to ensure that the data strategy is tied to the business objectives and reflected in C-suite messages to the organization. This can reinforce the importance of the work done by business teams.
Want More Information About Our Services? Talk to Our Consultants!
The Conclusion Of The Article Is:
Data, analytics and AI are becoming increasingly integrated into most organizations' daily operations. A new approach to data architecture will be needed to grow and create a data-centric organization. Data and technology leaders that embrace this new approach are better positioned to make their organizations more agile, resilient and competitive.