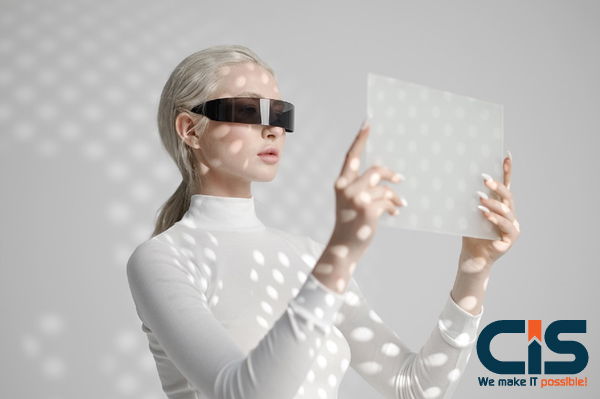
One area where AI shines is DevOps. This technology allows DevOps teams to automate their processes and increases innovation. Continue reading for more information about AI's impact and plans.
What's AI?
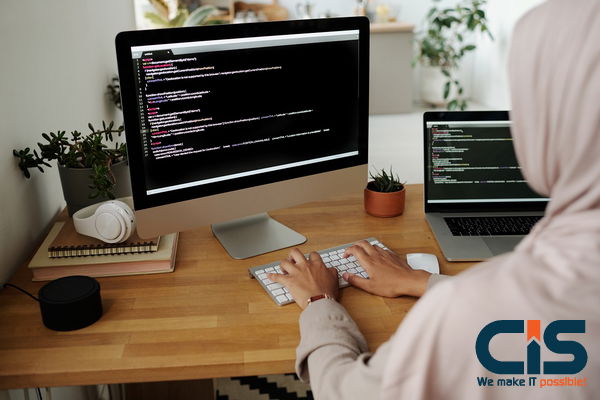
Artificial Intelligence is a term that refers to programs that can solve complex problems that humans can solve. This field is home to many popular programming languages and researchers. Their primary goal is to create programs that solve problems without supervision. They employ logic and methods that are different from the ones used by human intervention.
AI is revolutionizing the way many companies and organizations work. This is a great reason to use AI.
DevOps: Why?
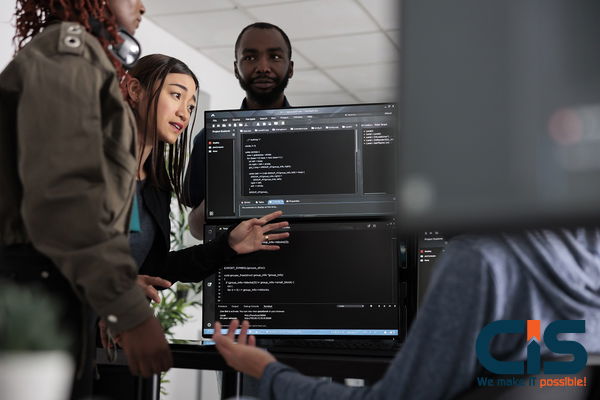
AI is already impacting DevOps, and it is expected to continue to make a significant impact. Together with AI, machine learning (ML) can improve DevOps. More organizations are using the model to improve efficiency in application development and other areas of business.
DevOps can have some positive effects on organizations.
- Collaboration and communication between teams have been improved.
- Automation
- Developer satisfaction is higher due to more incredible innovation and less time fixing bugs.
- Faster development and faster "go to market" times
- Problems can be solved quickly and efficiently.
- Lower operational complexity
- Continuous delivery fixes and features that have fewer flaws.
DevOps focuses mainly on load testing. Other tools and AI can also be used.
DevOps
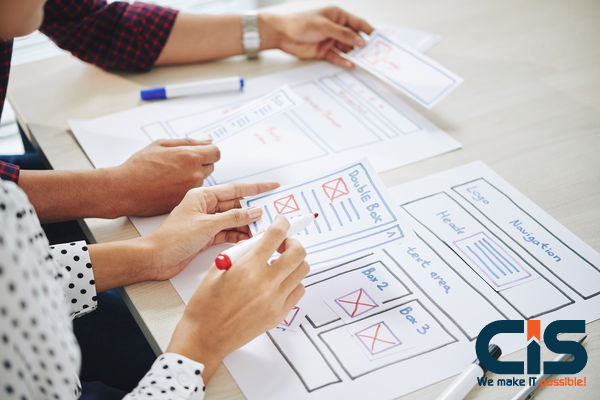
AI can help DevOps teams create, deliver, organize, and deploy applications. This will enable them to improve performance and run business operations. AI has many potential ways to impact, improve and change DevOps. These are the ones that we highlighted.
Enhanced Accessibility
DevOps teams are concerned about unregulated data access. AI can help address this problem by releasing data from its proper storage. This is crucial for large-scale data projects. AI allows teams to collect data from multiple sources and prepare them for reliable and robust evaluation.
Higher Implementation Efficiency
AI actively contributes to all types of self-governed systems. This allows DevOps teams to move from a rules-based, human-brain-managed system. This reduces the complexity of assessing human intelligence agents and increases efficiency.
Effectively
AI can automate routine and repetitive tasks. This could make it easier to manage multiple resources.
Companies Can Use AI to Optimize Their DevOps
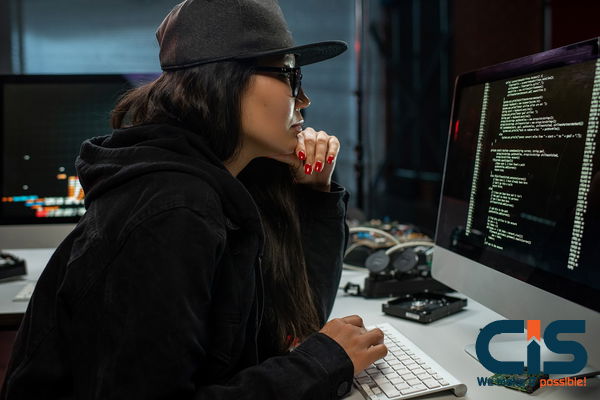
Organizations can now use AI, which can significantly enhance their DevOps environment. AI can manage complex data pipelines, which can be used to create models that let data enter apps. AI (Internet of Things) will dominate the IoT and digital revolution.
Implementing AI for DevOps presents many challenges to businesses and organizations of all sizes. Organizations need a customized DevOps platform to reap the benefits of AI technology.
Open-source projects like Fabrice for Deep learning and Model Asset eXchange can lower business entry barriers. They will be able to use machine learning and improve DevOps efficiency.
A significant ROI can be achieved by any company using AI to optimize DevOps operations and make IT more responsive. They can increase team productivity and efficiency, and bridge the gap between big and small data.
As a result of technology's global takeover, the impact of artificial intelligence (AI)on our lives is growing daily. DevOps is no different. Security is a critical aspect that stands out. DevOps Training and AI have made security one of the most critical components.
This brings us to the question, "How can a DevOps group take advantage of AI?" To answer this question, we will first focus on DevOps' definitions. Next, we'll discuss how DevOps can take advantage of AI. Then, we'll also address how AI is changing the former. Companies are willing to pay a lot for DevOps engineers with the combination of the right skills due to high demand. DevOps engineers are those who have completed certification in Best Online DevOps courses. They are often considered a vital member of any software development team.
How AI is Transforming DevOps
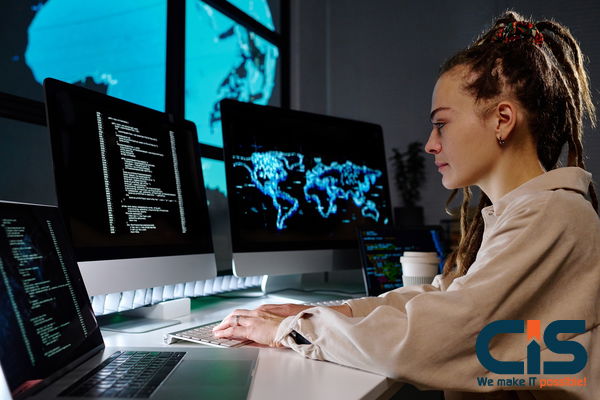
It is now time to understand the impact of AI on DevOps. Both can be used in combination to overcome many difficulties.
- Managing and acquiring input during each stage in the software development cycle is easier.
- Software testing increases productivity and the overall effectiveness of the development process.
- Increased deployment speed and greater capacity to execute security checks will lead to greater security.
- AI can collect data from many sources to create an integrated company. It can also be used to analyze data more efficiently.
Increased data accessibility via AI improves teamwork and efficiency in DevOps teams.
How to Implement AI in DevOps
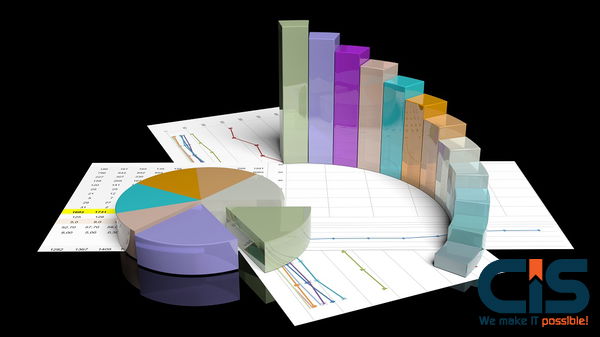
When integrating AI into DevOps, it is essential to consider the following:
- Data Quality: For AI to work effectively, high-quality data is essential. If the data used by AI is high-quality and correct, it can lead to better judgments.
- Data Management: Data management can be AI's most challenging and expensive aspect. DevOps teams need access to data to train AI models. This can be costly and time-consuming to collect and categorize the data. AI systems require constant maintenance and improvement as new data is collected and new issues are discovered.
- Ethical Issues: As AI systems become more sophisticated, they will make more decisions that can impact people's lives. AI systems could be used to determine who is eligible for a loan and who is a good candidate for a job. Because these decisions could significantly impact people's lives, it is essential to ensure that AI systems are ethically sound.
- A Disruption: As AI technology spreads, it may cause changes in corporate processes and paradigms. Suppose AI is used to automate customer service duties, for example. In that case, a corporation might need to reassess its personnel and business model. AI systems can also cause legal problems for businesses if they consider the impact of AI-generated judgements.
DevOps Problem Solving: Enhancing the Performance of Artificial Intelligence
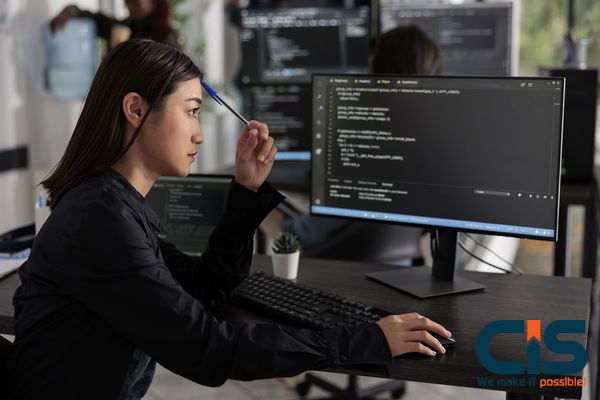
There are many ways to increase AI's effectiveness in DevOps problem-solving. One option is to use AI-enabled tools such as chatbots or virtual assistants. These technologies can be used for quicker problem-solving by developers.
Another technique to improve its performance is to use AI to automate repetitive tasks, such as testing code updates or checking log files. DevOps teams might have more time to dedicate to strategic priorities, which could make them more productive. Training and retraining AI models to keep them up-to-date with new DevOps trends and technologies is crucial.
The Potential Benefits of AI in DevOps
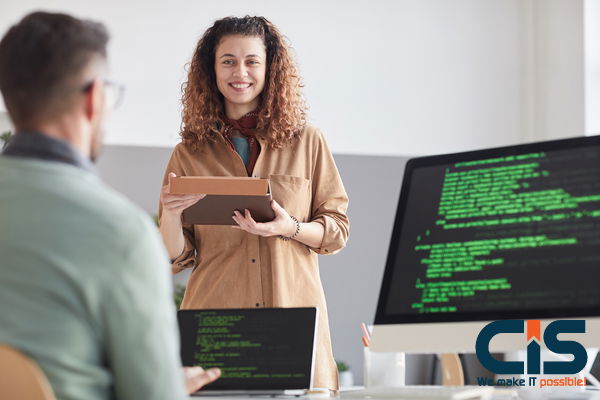
Here are some of the benefits of DevOps.
Automate Repeatable Processes
AI can automate routine tasks such as configuring resources and deploying applications. As a result, DevOps teams might have more time for strategic work.
Optimize your Workflow
AI can help DevOps teams optimize their workflow by identifying inefficiencies and bottlenecks. AI can analyze a process and suggest improvements to improve efficiency. For instance, AI might recommend a task that takes longer than usual to complete.
Monitoring the Performance of Your System
AI allows for real-time monitoring of the system's performance. This can be used to detect potential problems before they become serious. AI can be used to prevent problems from affecting customers.
Enhancing Customer Engagement
AI provides insight into customer usage patterns and can help DevOps teams improve customer satisfaction. For example, AI can pinpoint customer pain points and suggest changes to improve the customer experience.
Cost-Reduction
AI can help DevOps teams reduce costs by streamlining workflows and automating jobs. For example, AI can automate a task, which could result in cost savings.
A DevOps strong Foundation Certification Course is available, which can cover everything you need to know about coding and more advanced concepts like containerization and orchestration.
Limitations to AI in DevOps
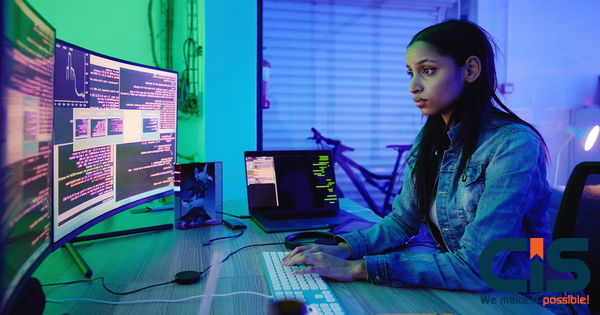
Although there are many benefits to DevOps with AI, some limitations remain.
AI is Imperfect and Prone to Error: An AI system that needs to be adequately trained or set up can make informed decisions that are not in the best interests of clients or businesses.
An AI system that isn't correctly set up can cause performance or outages, for example.
AI Implementation can be Expensive: DevOps teams need access to data, computing capacity, and qualified people to make AI work efficiently.
Data can sometimes be the most challenging and expensive part of the equation. Labeling and collecting data sets that will be used in training AI models can take time and money.
AI systems require constant maintenance and improvement as new data is collected and new issues are discovered.
AI could Raise Ethical Questions: AI systems may make more judgments that can impact people's lives as their technology improves.
AI systems could be used to determine who is eligible for a loan and who is a good candidate for a job.
Because these decisions could have a significant impact on the lives of people, it is essential to ensure that AI systems are ethically sound.
AI can cause Disruptions: As AI systems spread, they may alter existing corporate processes and paradigms.
Suppose AI is used to automate customer service duties, for example. In that case, a company may need to reevaluate their personnel and business model.
AI systems can also cause legal problems for businesses when they consider the impact of AI-generated judgements. These drawbacks aside, DevOps teams might find AI helpful. AI can automate repetitive tasks, streamline workflows and improve system efficiency. It is essential to consider the drawbacks of AI before using it to solve problems.
Read More: 3 Factors Accelerating The Growth of Artificial Intelligence (AI)
Case Studies of AI & ML Implementations in DevOps
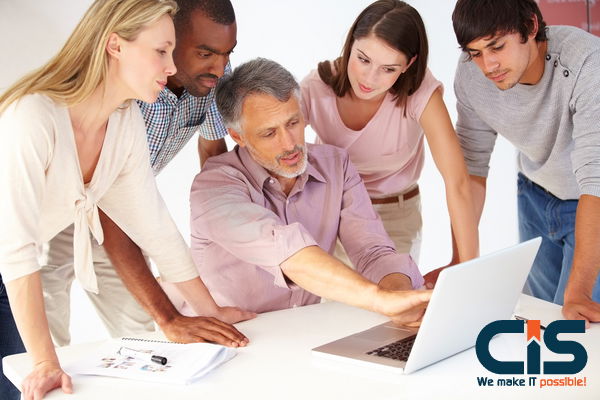
App Delivery: Insights
DevOps teams can use machine learning to identify anomalies in data collected from different DevOps technologies. This will help them find the "wastes" of software development. This can help teams streamline their delivery and processing. Activity data from tools such as Selenium and Jenkins, Puppets, Docker, Ansible and Nagios can provide valuable insights into the delivery process.
Failure Rate Predictions
Machine learning can predict the probability of failure by looking at past failures. This information can be used to identify problem areas and prevent or mitigate future problems.
Increase Efficiency of Resource use
Recognizing how resources are used can help you optimize resource consumption and reduce expenses. Machine learning can help you identify underutilized resources and make suggestions to improve their use.
Automation Efficiency Testing
Machine learning can be used for automated testing by identifying the test cases most likely to detect errors. These patterns will help you save time by focusing on the most critical test cases.
AI-Enhanced Collaboration in DevOps
Silos between the operations and development teams can lead to many problems. Machine learning can be used to understand the relationships between these teams better and improve collaboration.
One of the easiest ways to achieve this is to give all stakeholders of a project access to one source of truth, where relevant data can be retrieved. AI uses these touchpoints to improve its understanding of how these applications should work. These lessons can be used to improve routine workflows. They are sending notifications to alert you if there is an anomaly.
DevOps teams may use AI to automate tasks, improve productivity and optimize processes. As they get more familiar with AI, DevOps teams may use it in more ways to improve workflow. Online certification in DevOps Certification allows you to learn and even improve your skills.
The Best Tools to Support DevOps With Artificial Intelligence
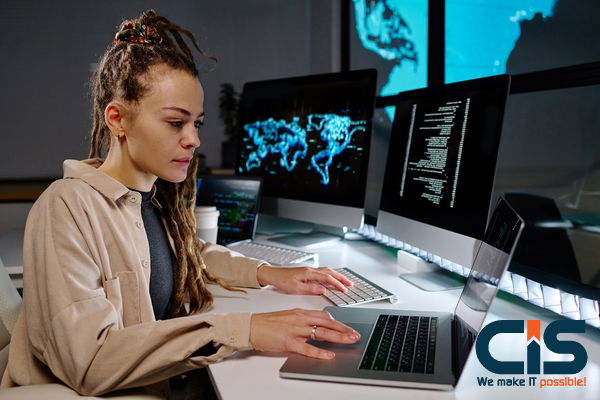
These are the best AI-enabled DevOps Tools:
- Chatbots: Developers can communicate with chatbots to solve problems faster.
- Virtual assistants can automate repetitive tasks like running code modifications or checking log files.
- AI-enabled monitoring software: These tools can spot faults and potential issues with code updates.
- AI-enabled tools for testing: These tools can be used automatically to test any modifications to the code and ensure that no bugs are introduced.
AI and Machine Learning for DevOps
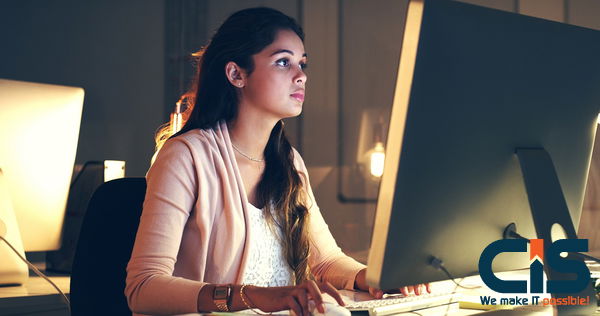
After being adopted into DevOps, AI and machine learning took many forms. These are just a few of the many ways these technologies are used in this space.
AI-Ops and ML-Ops
AI-Ops (or ML-Ops), which stand for "Artificial Intelligence Operations" and "Machine Learning Operations," respectively, use either machine learning or artificial intelligence to perform operational tasks. It is possible to train algorithms to run jobs during different stages of the software development cycle. AI-Ops employs machine learning and analytics to analyze large amounts of data gathered from DevOps pipelines. This allows them to learn which tasks are required and how to implement them.
Machine learning and artificial intelligence can be used to create virtual environments for testing source code in the build phase. They can also be used during the monitoring phase to monitor their resources. This ensures consistency between developers and operations in the application and infrastructure.
AI-Ops/ML-Ops allows computer systems to learn about the environment and package the code during the development phase so that it can run in production. This will allow teams to save time provisioning and managing resources. They can also monitor their performance passively.
Automation
DevOps teams have begun to use AI and machine learning for automating workflows. Machine learning, AI, and data science can quickly solve problems. Artificial intelligence, for example, can accelerate the development stages of the software development cycle. This can be achieved by using AI-powered code completion tools such as Tabnine or GitHub Copilot. These tools provide code suggestions to assist developers in the development phase. It can also automatically generate some tests required for quality assurance.
Testing teams will be able to spend less time testing and more time automating their environments to validate and push code into production faster. Machine learning and AI can automate more tasks than simply testing code. Machine learning algorithms can be used to automate code reviews. This will automate the process of optimizing code to improve app performance.
Quality
AI and machine learning can increase automation in software development and enhance the quality of enterprise software. AI-powered tools can predict deployment failures by looking at past code statistics.
Releases and saves application logs. Teams can improve their software releases by knowing which methods work best and implementing the changes before pushing them to production. Machine learning is used to identify potential issues in code, such as resource leaks, concurrency races, and wasted CPU cycles, which could impact the performance of the software in production.
This information will allow DevOps teams to gain more insight into performance bottlenecks and help them optimize their code and infrastructure to address those issues. Although there may be some doubt about the reliability of machine learning and AI in improving code quality, recent research has shown that they are reliable in managing software.
When it comes to discovering flaws and suggesting fixes in the source code at Facebook, machine-learning technologies like bug detection are correct around 80% of the time. Artificial intelligence and machine learning tools will grow more dependable in enhancing software quality.
Security
Despite the security threats they provide, AI and machine learning have shown to be incredibly helpful in keeping infrastructure and services secure. They can identify irregularities in services and systems. According to GitLab's DevSecOps Report, data mining, machine learning, and data integration powered by AI can all help accelerate anomaly detection until the device's boot time.
DevOps teams will be notified of system vulnerabilities as soon as the device boots up. Machine learning tools can also be trained to detect anomalies immediately and notify DevOps teams. They can prevent more vulnerabilities from being exploited in software and help keep end-users and enterprises safe.
Machine learning and AI are valuable tools for governing compliance in IT environments. DevOps teams can train AI and machine learning algorithms to ensure security compliance throughout the enterprise. They could also create a baseline to guide them. They can then take preventative actions and stop threats, such as informing users about vulnerabilities or closing down devices not complying with their security policies. Companies have been able to acquire or adopt new AI tools as a result of security advances.
JFrog recently purchased Video, an AI tool that detects and fixes vulnerabilities. It uses a survey to examine the behavior of the devices. The AI then compares the device's behavior to a baseline to determine when it isn't operating as intended. DevOps teams can then decide whether to close down the malicious device, isolate the device from critical resources, or conduct additional research into the device's behavior as indicated by the AI tool. Due to machine learning and AI's anomaly detection capabilities, DevOps teams will find it easier to maintain security and compliance.
No Code
The growing use of low-code or no-code tooling within the tech sector must be mentioned. Teams will require less manual management due to artificial human intelligence's capacity to learn about and handle technical skills resources. Teams only need to develop a little code to get their services and systems up and running. Future programming skills will be able to produce software with a minimum amount of manual labor and user-friendly interfaces while keeping the general caliber of their work.
Additionally, developers can create the necessary functionalities in an app with less code. This will allow them to work more efficiently and help with debugging issues. Low code/no code tools also reduce the complexity of programming languages. Developers and DevOps experts are increasingly embracing low-code and no-code tools as they seek to improve the quality of their software and build and deploy high-performance apps.
AI Tools
Many AI and machine-learning tools are already available in the industry. These are just a few of the tools that exist in this field.
Arize AI, a DevOps machine-learning tool, detects the root causes faster and solves model performance problems quicker. It can be used to monitor performance at a large scale and send real-time alerts directly to DevOps teams to resolve issues quickly. The tool will notify the DevOps team of potential infrastructure or application performance issues using patterns found in the metric data collected for training the models.
Dynatrace, an AIOps tool, provides observability, automation, and cloud-native app security, which DevOps teams can use in their CI/CD pipelines. Dynatrace's deterministic AI can be trained on operational data to provide actionable insights for heterogeneous cloud environments. This is an excellent tool for DevOps teams.
Applications may be safely deployed into cloud settings by detecting cloud-native anomalies before they negatively impact the organization. Its automated runtime vulnerability detection function covers the complete software development life cycle. Snyk's vulnerability database and Dynatrace analysis are combined to achieve this. Fixing security flaws in the code phase is the aim of the monitoring phase.
Another machine-learning tool worth mentioning is Diffblue. One illustration is Diffblue, an AI-powered platform that automates the testing stage of software development. It can be integrated with your Java code repository to produce unit tests that faithfully reproduce the code's behavior right now. This enhances continuous integration operations, enables automatic regression with no effort, and finds regression problems early.
The last tool on the list is Amazon CodeGuru. This AI program can automate code review and find security issues. The programme uses automatic learning, security best practices, and knowledge from code reviews. It automates code reviews and offers suggestions for resolving any problems. Adding machine learning models to this tool makes it the next evolution in static code analysis.
Want More Information About Our Services? Talk to Our Consultants!
Conclusion
AI and machine learning are already impacting how software and infrastructure are developed, deployed, and managed. Artificial intelligence, anomaly detection, and automated testing will all significantly improve the development cycle. DevOps teams can use automated, AI-powered solutions to replace some of their manual operations.
This will enable them to see all these capabilities and technologies and raise the caliber of their output. Also, it enables them to handle their systems more skillfully. We trust that you can now observe how a company uses artificial intelligence. DevOps teams can educate algorithms about the jobs and situations that require automation, making it easier for them to maintain the standards they need for their companies.