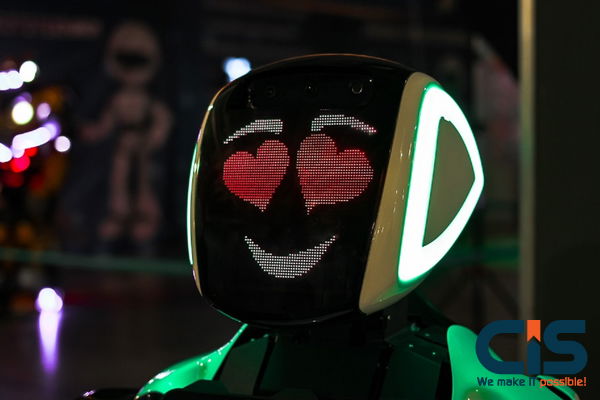
Recently, a lot has been written on the distinctions between the various data science roles, particularly those between data engineers and data scientists. Maybe the shift in viewpoint is what's causing the increase in interest. Unlike earlier times, when the focus was on deriving useful insights from data, data management has slowly started to gain industry acceptance. You can build the most sophisticated models, but your data will not be qualitative. This is exactly the same as the old adage, and it still holds true.
Data engineers have gradually been relegated to the background.The main distinctions between data scientists and data engineers will be covered in this blog article. It will concentrate on duties, instruments and languages, educational background, pay, hiring, employment prospects, and tools you may utilize to get started in either engineering or data science.The infographic "Data Engineering or Data Science" contains references and a visual presentation.
What Is The Difference Between A Data Engineer And A Data Scientist?
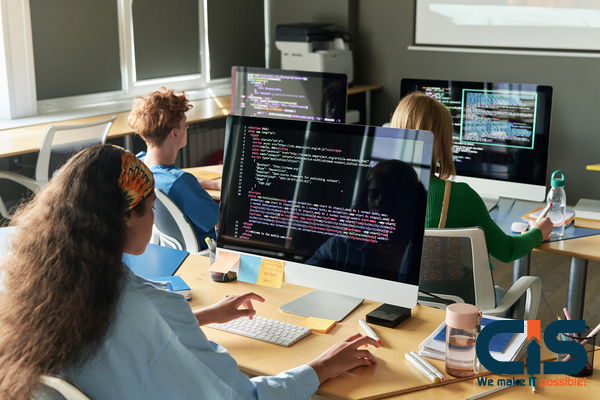
Data scientists used to be expected to perform the duties of data engineers. As data management and data collecting have become more challenging, the work of a data scientist has grown more challenging. The data that organizations gather should provide them with additional information and solutions.
Data engineering creates and maintains the systems and structures used to store and retrieve data, which is now the fundamental distinction between the two data specialists. Data scientists examine the information to find trends and offer commercial advice.
Data Engineer vs. Data Scientist
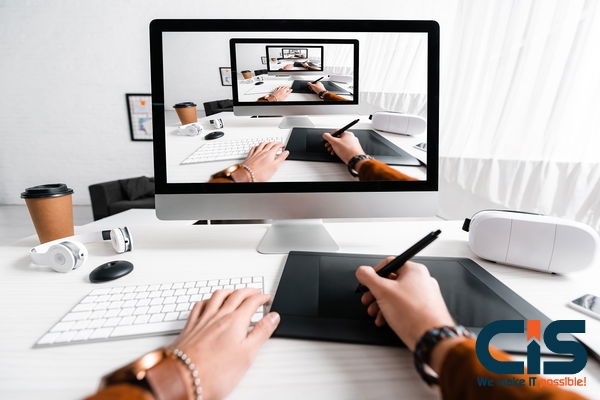
Despite the fact that data scientists and data engineers share some abilities and that, in the past, data scientists were expected to carry out some of the same tasks as data engineers, these professions are separate from one another.
What Does A Data Engineer Do?
Data engineers are experts who build the framework required for data analysis. They emphasize production readiness as well as additional factors, including formats, scalability and resilience, data storage, security, and security. Data engineers are in charge of creating, testing, and managing data from several sources. Moreover, they develop the systems and infrastructure required for data creation.
Its primary goal is to combine diverse big data service technologies to build free-flowing data pipes that enable real-time analysis. To make data accessible, data engineers can also write sophisticated queries.
Data engineers provide data to data scientists, who then use it to get fresh insights. They create hypotheses and perform online experiments. They interact with corporate executives to comprehend their needs and then deliver complex results in a form that is understandable to a broad audience.
What Does A Data Scientist Do?
Since the early 2000s, data science has experienced tremendous growth. A data scientist, for instance, has to be knowledgeable in SQL query writing as well as R, Python, or both. Also, they must be knowledgeable with machine learning frameworks like TensorFlow and PyTorch.
Nevertheless, not all businesses define this position in the same way. The most well-known example is probably ETL.
Extract, Transform, and Load is referred to as ETL. It describes the procedure of extracting unorganized data from a source, cleaning, aggregating, and massaging the previously raw data, and then entering the newly converted, significantly more presentable data into a new target location, typically a data warehouse. Because of Stitch, the T and L can occasionally be inverted to speed up the operation.
Even if ETL is more automated than ever, oversight is still necessary. Data engineers have often been in charge of this. In this regard, an expert is a traditionalist. According to experts, having the data engineering team handle the ETL process results in better results, especially if it's not a one-off.
According to an expert, "Unless a Data Scientist has considerable expertise or time committed to it, you definitely don't want them managing or managing a continual data pipeline that's going to be to continuously run processes and constantly update data in a warehouse."
This isn't always the case, though. For example, Shopify's data scientists are in charge of ETL. Shopify's senior data product manager is Miqdad Jaffer. Data scientists, according to him, are more used to the tasks they will perform and the data sets they will use.
It is worth reading his argument:
This shouldn't be a highly skilled or specialized position. It is done out of love for all that the profession holds to be pure and holy. Writing, maintaining, and altering ETL data to produce data you never use or consume is the most taxing activity there is.
Give everyone control over the work they produce as an alternative (autonomy). When it comes to data scientists, this would also involve ownership of the ETL. Ownership over the analysis and outcomes of data science is also included here.
Role and Responsibilities
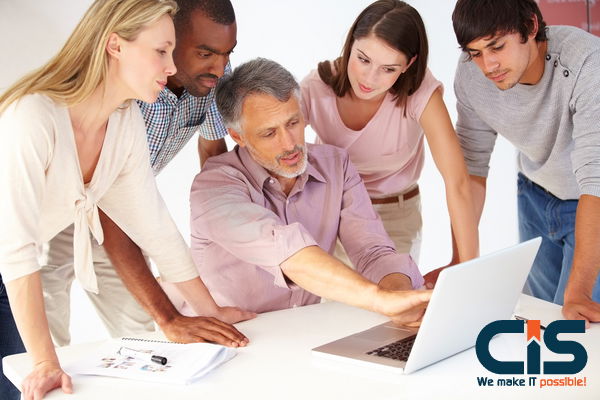
Understanding data scientists and data engineers as complementary professions are useful. The platforms that help data scientists do their work are subject to optimization. These systems are created and built by data engineers. The massive volumes of data that engineers handle provide meaning to data scientists.
Data Engineers' Responsibilities
Large-scale processing and database systems are built, tested, and maintained by data engineers. The data scientist, on the other hand, is a person who organizes and purifies large amounts of data.
Although using the word "massage" may seem like a strange choice, it only serves as an illustration of the distinctions between data engineers and data scientists.
Both sides will put in various amounts of effort to get the data in a manner that can be used.
Data engineers work with unprocessed data that might be contaminated by instruments, machines, or human mistakes. Data may not have been verified and may include questionable records. Moreover, it might not be formatted and might have system-specific codes.
It will be up to data engineers to recommend and occasionally put into practice improvements to data efficiency, quality, and dependability. They will need to merge systems or look for possibilities to obtain data from other systems, which will require them to use a range of languages and technologies. This is done so that data scientists may utilize system-specific codes to guide subsequent processing.
These two problems are interconnected. The infrastructure in place must assist data scientists, stakeholders, and the business, according to data engineers.
To enable data mining, modeling, and production to be given to the data science group, the data engineering team must also design data set procedures.
In our post, you can read more about the duties of a data engineer.
Data Scientists' Responsibilities
Frequently, data used by data scientists have already undergone some initial cleansing and processing. To prepare the data for predictive and prescriptive modeling, this data may be put into advanced analytics programs, machine learning, statistical approaches, and other statistical methods.
Data scientists will need to investigate the market and provide answers to business queries in order to develop models. To meet business objectives, they will also require extensive access to data from both internal and external sources. This occasionally entails going through and studying data to find hidden patterns.
Key stakeholders will be informed of the study's findings and a compelling narrative when it has been finished by the data scientists. Automation will be required when the results are approved to make sure that company stakeholders can access the insights on a daily, monthly, or annual basis.
To handle the data and make decisions that are essential to the business, both sides must cooperate. Although the two's skill sets are somewhat similar, they are diverging more and more in the business. The data scientist will need to be knowledgeable in statistics, arithmetic, and machine learning in order to construct predictive models, while the data engineer will deal with databases, APIs, and tools for ETL.
Distributed computing must be understood by data scientists. They will require access to the data that the data engineering team has processed. They must also report to corporate stakeholders, though. It's crucial to prioritize narration and imagery.
Read More: What Are The Different Types Of Data Analysis?
Languages, Tools & Software
The languages, tools, and software they both utilize might vary according to their different skill sets. Both open-source and for-profit solutions are included in this overview.
Data engineers frequently deal with tools like SAP, Oracle, and MySQL. However, the specifics of the position will determine which ones are utilized by each party.
Models will be created by data scientists using languages like SPSS, R, and Python. The two most popular tools are R and Python. You will use R to make stunning data visualizations using programs like ggplot2 and Pandas. While working on data science projects, numerous additional programs, like Scikit-Learn and NumPy, can be employed.
There are commercials. In the sector, SAS and SPSS are widely used. Data scientists will also benefit from additional programs like Tableau, Rapidminer Matlab, Excel, and Gephi.
The emphasis on narrative and data visualization, which distinguishes data scientists from data engineers, is plainly reflected in the technologies described. You may have already guessed that both sides share the same software, languages, or tools: Scala, Java, and C#.
These languages are not popular among data scientists or engineers. Scala could be more popular among data engineers, as Spark's integration consulting with Scala makes it easy to create large ETL flows.
The popularity of the Java programming language has decreased. Yet data scientists use it more frequently than experts do. Both job advertisements will include these languages. This holds true for any technologies that may be used by both parties, including Spark, Storm, Hadoop, and Storm.
A context-sensitive approach is required when comparing tools, languages, and software. In some circumstances, data science and engineering can coexist. The distinction between the two can, however, be a bit hazy at times. This is an excellent idea, but it is still worth a discussion.
Education Background
Both data scientists and data engineers may have a background in computer science in common. Both of these professions place a high value on this field of study. It is also common knowledge that data scientists have degrees in statistics, econometrics, and operations research.
Compared to data engineers, data scientists frequently have more business sense. Engineering backgrounds are common among data engineers. They frequently have some background in computer engineering. This does not exclude data engineers from learning from prior research in operations or business savvy.
It's critical to realize that individuals from a variety of backgrounds work in the data science sector. Physicists, biologists, and meteorologists frequently transition into the field of data science. Some people work in database management, web development, or both.
Outlook for the Job
Roles and titles are necessary to represent shifting requirements, as was previously indicated. Sometimes they are designed to set you apart from competing hiring agencies.
In order to store and manage data, businesses are also seeking methods that are affordable, adaptable, and scalable. Companies must develop "data lakes" to replace the Operational Data Store or supplement current data warehouses in order to shift their data to the cloud. Data flows will need to be updated or rerouted during the next few years. Data engineers are now being sought after for more jobs as a result.
Since the beginning of the buzz, data scientists have been in great demand. Today, however, businesses choose to build data science teams over recruiting unicorn data scientists. These data scientists need to be intelligent, creative, and good at communicating. It's challenging for recruiters to discover candidates with all the traits that businesses want.
It may be argued that the "data scientist bubble" has burst. It could rupture once more in the future. Through it all, one thing will never change: there will always be a need for data scientists who are enthusiastic about what they do. According to McKinsey, the US may have a deficit of 140,000 to 190,000. Moreover, they want 1.5 million analysts and managers who can utilize big data analysis to make wise judgments.
Is It Possible For A Data Scientist To Become A Data Engineer?
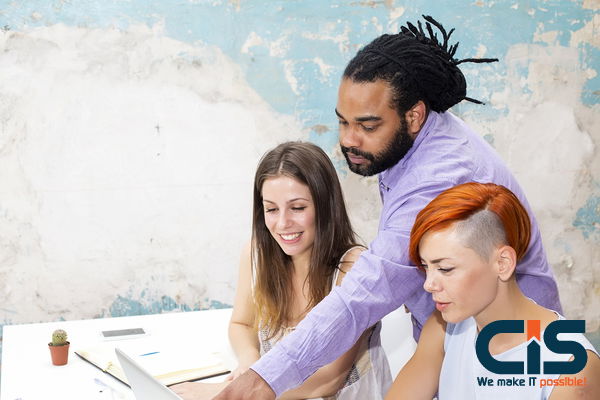
Despite the fact that data scientist and data engineer professions are distinct from one another, there is some work overlap, making it easy to move between the two. For convergence, Python and SQL are both necessary. The most used language for storing, retrieving, and altering data is SQL.
On the other hand, Python is a well-liked programming language. Candidates with experience in the conventional fields of data engineering are preferred by organizations with significant data science teams (big Data Tools, data modeling, and data warehousing).
The organization's size and personnel skill levels have an impact on ETL and model generation as well. Smaller teams could find it difficult to replicate this procedure and would need to combine duties into each function. Not many businesses, according to experts, are able to distinguish clearly between these roles.
Frequently, there is overlap. There are many similarities between data scientists and engineers, but there are some things you need to remember when transitioning from one to the other.
A network of data science bootcamps and extensive online courses has expanded along with the growth of data science. Another important lesson: On-ramping into an analytics position is an alternative. There are various prospects in data engineering.
Although there are certain courses, the bootcamp movement has not significantly touched data engineering. The classic software engineering approach is the more common one. This sector frequently attracts engineers that are passionate about data structures and distributed system architecture. This position may be characterized as a general software engineering challenge.
Data engineers who are interested should also practice their analytics abilities, just as aspirant data scientists are urged to be proficient in certain areas of data engineering. Although they may be skilled programmers, according to experts, they will want to know how their outputs will be used.
The millions of data models that companies use today were created with the help of both data scientists and data engineers.
Data Scientist Vs. Data Engineer: Which Is Best For You?
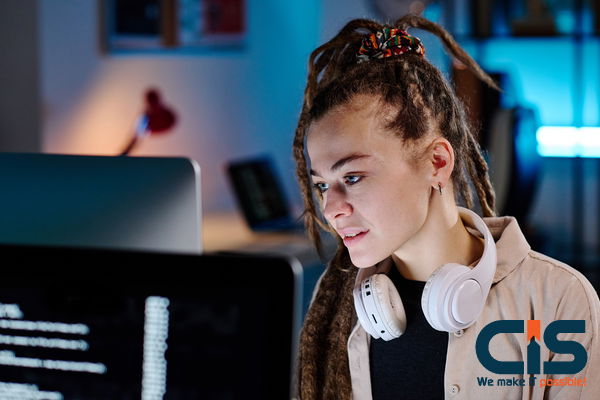
Despite having similar skills, data engineers and data scientists have distinct responsibilities. Some roles might be more suited for certain personality types.
If You Are Interested In Becoming A Data Engineer
The architecture and infrastructure that stores and organizes data are the responsibility of data engineers. These engineers are proficient coders who take pleasure in discovering new technologies and improving the functionality of software and systems.
They also like saving money and time. If you enjoy creating tools that help others and are constantly seeking ways to do better at what you do, a career in data engineering may be right for you.
If You Are Interested In Becoming A Data Scientist
Data scientists are analytical thinkers who are receptive to new concepts, curious by nature, and eager to examine their assumptions to the hilt. Data scientists utilize data to predict future trends and events as well as to make sense of previous occurrences. If you enjoy doing intricate statistical analyses, creating machine learning algorithms, and coming up with original solutions to issues, a job as a data scientist may be right for you.
Want More Information About Our Services? Talk to Our Consultants!
Conclusion
There are many reasons to start using data. This is not a problem. Many organizations offers courses that will help you start learning data engineering. Of course, there are other courses like Exploratory Data Analysis, Introduction to R for Data Science, Machine Learning Toolbox, and Introduction to Python for Data Science for people who wish to start learning about data science.