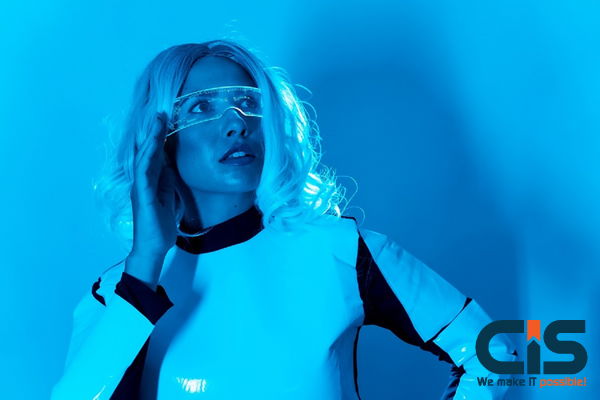
- Data quality
- Data Security
- Data Integrity
However, machine learning governance data is not a topic that is frequently searched on Google. As a result, business leaders are not aware of the latest developments (Figure 2). This article will examine the importance of machine learning data governance to inform business leaders about its:
- Key principles
- Benefits
- Use Cases
- Best Practices
- Future of Data Governance to Establish a Robust Data Governance Framework
What is Machine Learning Data Governance?
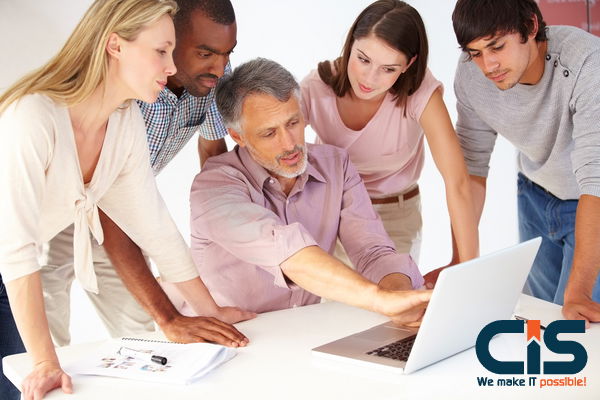
Machine learning data governance refers to the policies, processes, and technologies that are used to ensure the correct management and use of data in machine-learning applications. It involves:
- Data collection
- Data storage
- Data Processing
Data sharing is done in a controlled manner to:
- Protect data privacy
- Maintain data quality
- Support compliance with relevant regulations
Machine Learning Data Governance: Key Principles
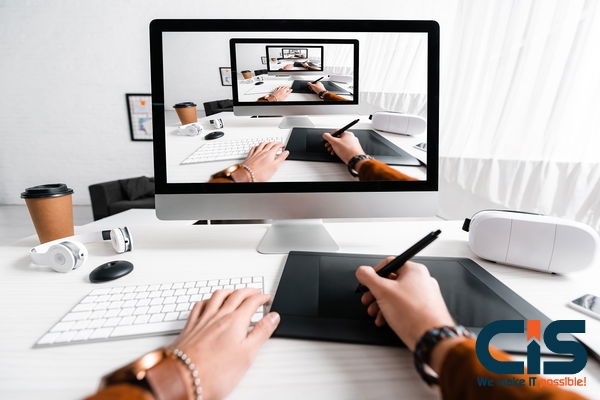
Data quality
For field of machine learning to produce meaningful and reliable results, it is essential that the data used is in wide range:
- Accurate
- Complete
- Consistent
The use of high-quality data in validation, data cleansing, and data enrichment can help maintain high standards of data quality.
Privacy and Security of Data
It is important to adhere to data protection laws such as GDPR or CCPA and to protect sensitive data. Data security and privacy can be protected by encryption, access control, and regular system audits.
Data Lineage
It is important to track the data's origins and transformations as they move through the ML pipeline. This will help you understand the impact that data has on the performance of the model and maintain the traceability of the data pipelines. Data lineage can be crucial in machine learning applications as it helps organizations identify the data sources and transformations that have contributed to model outcomes.
Data Accessibility
For the smooth operation of ML applications, it is essential that data be easily accessible by authorized system users. By implementing data storage solutions and efficient data models, data accessibility can be increased.
Data Compliance
It is important to comply with industry regulations and ethical guidelines, such as the Health Insurance Portability and Accountability Act. (HIPAA), in order to avoid legal and ethical issues relating to the use and misuse of data for ML applications.
Machine Learning Data Governance has 5 Benefit
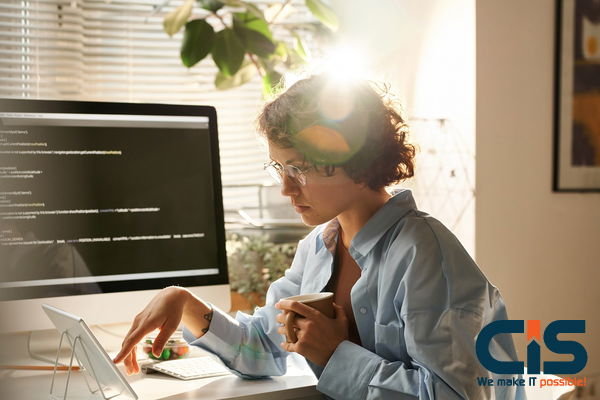
Improved Model Performance
Data that is high-quality and well-governed can be used to create machine learning models which are more accurate and reliable. This leads to better business decisions and outcomes.
Compliance With Regulatory Requirements
Robust data governance can help organizations comply with data protection regulations. This can help reduce the risk that non-compliance penalties or reputational damage will be imposed.
Transparency And Trust Are Enhanced
Data governance practices and policies demonstrate to customers, partners, and regulators that an organization is committed to ethical data use.
Data-Related Risks Reduced
By managing data definitions and security, as well as data privacy and quality, organizations can reduce the risk of data breaches, data misuse, and model bias.
Collaboration And Efficiency Are Improved
A data governance framework that is well-defined fosters collaboration between data scientists, engineers, and other stakeholders. This can accelerate the development and deployment process of machine-learning apps.
Machine Learning Data Governance: Use Cases
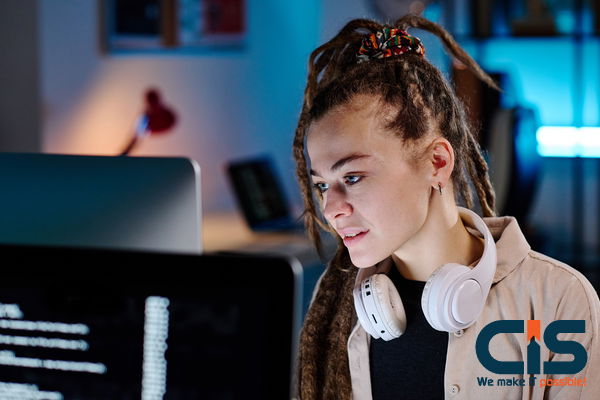
Fraud Detection
Machine learning is used by financial institutions to detect fraud. Data governance ensures that data fed to these algorithms are accurate, complete, and secure.
Personalized Marketing
Machine learning is used by retailers and ecommerce companies to create personalized marketing campaigns. Data governance ensures customer privacy and relevant content while maintaining data security.
Healthcare Diagnostics
Medical diagnostics increasingly uses machine learning algorithms. Data governance is crucial to maintaining data quality and privacy in healthcare applications.
Predictive Maintenance
Machine learning can be used by manufacturers to optimize maintenance schedules and predict equipment failures. Data governance is a way to ensure the accuracy of sensor data, IoT inputs, and other IoT applications.
Autonomous Vehicles
Data governance is essential to ensure the accuracy, quality, and security of massive amounts of data that are used in the development of self-driving vehicles.
Best Practices For Implementing Machine-Learning Data Governance
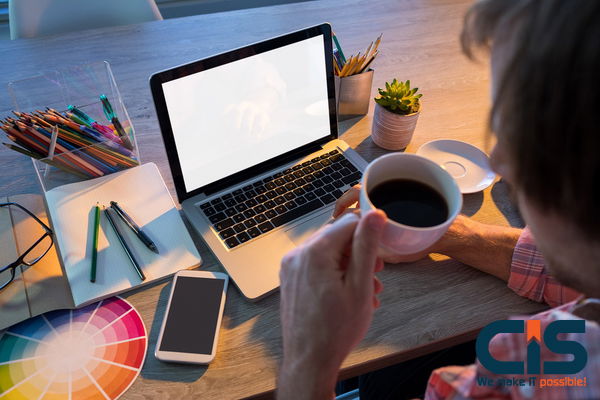
Create A Data Governance Plan
A data governance strategy defining your organization's and data stewards' goals, roles, and responsibilities can provide a roadmap for the effective management of data in machine learning applications.
Ownership And Accountability Of Data Should Be Established.
Data governance policies can be implemented more effectively by clearly defining ownership of data and assigning responsibility for quality, privacy, and compliance.
Implement Metadata And Data Catalogs
The creation of a data catalog and the maintenance of metadata for datasets that are used in machine-learning applications can help:
- Understanding data lineage
- Improving data discoverability
- Preserving data quality
Data Privacy Should Be Built Into The Design
Integrating data privacy considerations and security into the design and development of ML applications can help to ensure compliance with data protection laws and regulations.
Automate Data Governance Processes
Automating data governance tasks, such as cleansing, enrichment, and validation of data, can improve efficiency and maintain high standards for data quality.
Monitor And Audit
Regularly monitoring and auditing the data governance process can be helpful:
- Identifying potential issues
- Maintain data quality
- Check for compliance with all applicable regulations
Data fabric tools are particularly useful for monitoring and auditing data governance.
Want More Information About Our Services? Talk to Our Consultants!
Future Of Data Governance In Machine Learning
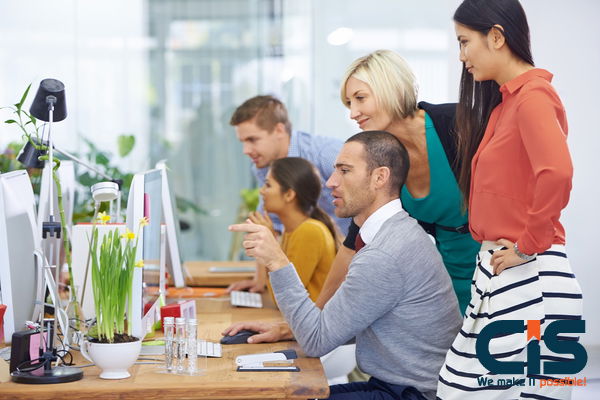
Ai-Driven Data Governance
We can expect AI-driven solutions for data governance to emerge as machine learning technology improves. These solutions will automate, optimize and streamline data governance processes. This will allow organizations to manage increasingly complex data ecologies more efficiently.
The Regulatory Landscape Is Changing
As more organizations adopt AI and machine learning, governments and regulators will continue to develop new guidelines and policies. Many organizations will have to adjust their data governance strategy in order to remain compliant with stakeholder expectations and maintain trust.
Privacy And Ethics Of Data
Data governance is important because of the increasing importance that data privacy and ethical issues have in machine learning. For organizations to maintain a competitive edge, they will need transparent, fair, and accountable data usage practices.
Data Democratization
Data governance is essential to maintaining data security and quality. When organizations democratize data and analytics tools, effective data governance empowers employees to take advantage of data-driven insights.
Integrating Data Governance With Model Governance
As machine learning models become increasingly complex and prevalent, the integration of data governance with model governance becomes more important. It is important to ensure that the data, as well as the models, are managed properly.
AI & ML can Transform Governance in 10 Ways
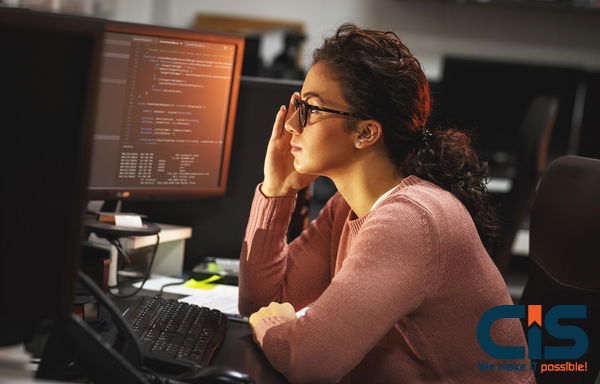
Artificial Intelligence (AI) and Machine Learning have dominated technology in the last five years. AI and Machine Learning are used by all industries, whether it is manufacturing, IT, or other. AI and ML are used by companies because they can do things humans cannot. AI and ML can have a positive effect on society and culture. Here are 10 ways that Artificial Intelligence (AI) and Machine Learning (ML) can improve governance.
Medicine and Healthcare
AI is a powerful tool in the healthcare sector. Precision medicine is one area in which AI has real potential. AI can be used to analyze millions of patient records at once. Data includes information such as past medical histories, gene variability, lifestyles, etc. These details can help doctors and medical practitioners accurately predict the best treatment options for each patient. IBM, for example, uses AI-based algorithms that accurately detect tumors in radiology scans. It's high time governments used AI to promote precision medicine.
Education
AI and ML, by analyzing a student's past data, can assist the student in making decisions about courses and electives in universities. AI chatbots are a great way to interact with students about admission queries. AI-based platforms for learning can provide personalized monitoring and attention to students. AI can be used to support personalized learning. Teachers can use AI to offer personalized advice to students. AI can help students by personalizing their class assignments and exams.
Agriculture
The world population is growing at an ever-increasing rate, so it's important to maintain a high food production level. AI can be a powerful tool for boosting efficiency in agriculture. It can gather and analyze data on various farming parameters, such as soil conditions or groundwater levels. AI can make recommendations based on data about what crops to grow, when to irrigate and fertilize them, etc. Precision farming is a type of AI-assisted agriculture. Precision farming is a way to increase food production and reduce waste without damaging the environment.
Read More: What Is Machine Learning? Different Fields Of Application For ML
Crime Prevention
AI and machine learning will have a huge impact on this sector. A platform based on AI can be used to collect all the data about crimes and criminals. Data such as the number and type of crimes, the criminal's behavior patterns, and their modus operandi or punishment status can be included. AI can detect patterns of crime and identify vulnerable areas through proper analysis. It can be used to track criminals and their likelihood of committing crimes. The Indian government launched a project in 2017 aimed at predictive policing. The project utilized AI and machine learning to facilitate criminal identity registration, search for missing persons, and tracking.
Advanced Robotics, Intelligence, and Surveillance
AI can be used for advanced surveillance and human intelligence gathering to monitor and control autonomous unmanned vehicles. Machine learning algorithms are able to evaluate border infiltration patterns and predict whether infiltrations will occur at specific times.
Humanoid robots soldiers have brought advanced robotics into the military. Robot soldiers are futuristic weaponry where a group of robots works together as a team to perform tasks, just like humans. The US government plans to deploy robots in combat roles by 2025.
Cybersecurity
In January 2018, the Indian government revealed that it had suffered a massive data breach. More than 1.1 billion Aadhaar numbers, along with the details of each user, were revealed. Massive breaches like this could be prevented if governments combined AI and machine learning with their current systems. AI's automation and self-learning features can increase security system effectiveness and reduce costs. They can use their prediction capabilities for fraud prevention and advanced threat detection.
Traffic Control
Traffic is a major problem for commuters today due to the increase in vehicles on the roads. Anyone who lives in a metropolis knows what it's like to be stuck in traffic. In most countries, traffic lights are set at a preset value in order to regulate the flow of traffic. This value does not change with changing traffic conditions. AI can be used to synchronize traffic data in real-time and control traffic accordingly. It allows for a smooth traffic flow without any inconveniences to commuters.
Disaster response
Earthquakes and tsunamis can cause immense destruction and leave countries utterly helpless. In these trying times, effective disaster management is a must. Machine learning and AI can be great help when managing disasters. AI can be combined with other modern technology for different applications, such as tracking people missing in real-time, identifying areas affected by disasters, conducting post-disaster studies, etc. Machine learning algorithms are useful for maximizing the use of limited resources.
Public Infrastructure
To avoid untoward incidents, it is essential to maintain public infrastructure regularly. The majority of infrastructure, such as roads and sewers, water systems, government-run hospitals, schools, colleges, etc., is maintained by the public sector. Regular inspections are required. AI can monitor machinery, infrastructure, equipment, etc. On a proactive basis. This allows for accurate maintenance predictions. Metrics and analytics are used to determine when and where maintenance will be required.
Citizen-Government Interfaces
AI can enhance eGovernment services by using citizen-government interfaces. The Andhra Pradesh Government in India, for example, partnered with Microsoft and created an AI-assisted application called Kaizala. Kaizala was a citizen-government portal that allowed citizen requests to be sent directly to the relevant government department. The app could be used by the government to send automated notifications to its citizens. Through these AI-assisted user interfaces, the government can better govern its citizens.
AI and Machine Learning in Data Governance
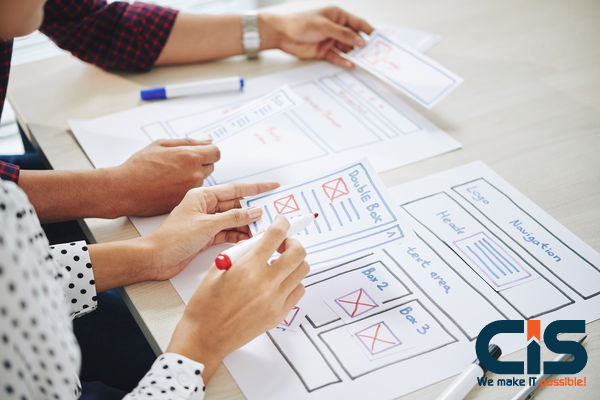
Data corruption can have a disastrous effect on enterprise Data Management. Data corruption is often caused by data silos, inconsistent data formats, divergent data views through different systems, and other factors.
Business leaders are becoming increasingly concerned with the reliability of this incoming data, as an increasing amount of data at high volumes and speeds choke the data pipelines of an organization. You cannot trust data if you can't trust anything else.
What does Data Governance have to do with all this?
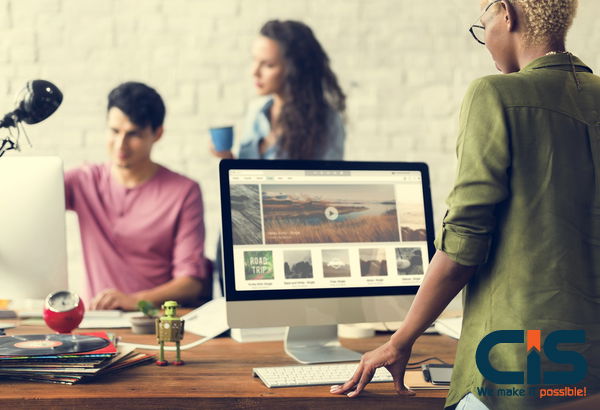
Data Governance (DG), also known as Data Asset Management, is a set of components that helps organizations gain control of their data assets.
The same customer data, for example, may be recorded differently in systems such as sales, logistics, and customer service. Data integrity issues will arise during the data integration stage, leading to mismatched data. This can also impact data analytics, reporting, and BI systems. These issues are a reflection of poor Data Governance and can lead to regulatory compliance issues.
Data consistency is a key objective for an enterprise Data Governance Program. Its primary goal is to standardize and create common data formats that can be used across the organization.
The World Economic Forum estimates that by 2025, 463 exabytes will be generated every day. This exponential growth of data volume will require enterprises to use automated Data Quality Measurement tools to support DG. A DG program that is AI or ML-assisted is a positive step.
Data of high quality is seen as a strategic advantage and a differentiator in the marketplace.
Many organizations are still using outdated practices, despite the availability of advanced technologies to enhance Data Governance initiatives. The AI and ML-assisted DG framework will help to "reduce risks and maximize the value of data and algorithms which increasingly drive competitive edge."
Recent technology trends, such as increased cloud adoption and omnichannel data, agile methodologies, self-service platforms, and the popularity and value of AI and ML, have made it necessary to modernize DG programs.
Data Governance without AI or ML: Challenges
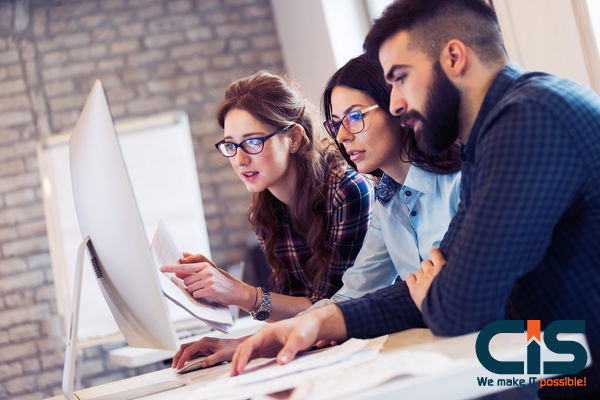
Here are some common challenges with traditional data governance:
- Data consistency across business functions is lacking
- Data available in different views across business functions
- Data definitions are not consistent
- Documentation of Data Governance Strategy
- Data misuse in self-service analytics and BI platforms
- Big Data Governance
DG author and coach Nicola Askham mentions, when describing six principles for a successful DG program, that executives want to know about the benefits from the beginning. Askham says, "If they don't feel that you're answering their question in a manner that is interesting to them and that benefits them, then they won't be interested."
A Data Governance program that is just getting started requires a kickoff meeting to demonstrate the benefits of the program. As the program grows, regular meetings with metrics and presentations will be required to convince business users of the importance of a DG program.
What kind of metrics should Data Governance program coordinators be using in their live presentations to explain the pros and cons of a DG program that is currently running?
Data accuracy and error rates, number of data corrections per quarter, and cost savings can all be used to measure Data Quality Improvement (DQ).
AI and ML Data Governance: A New Vision
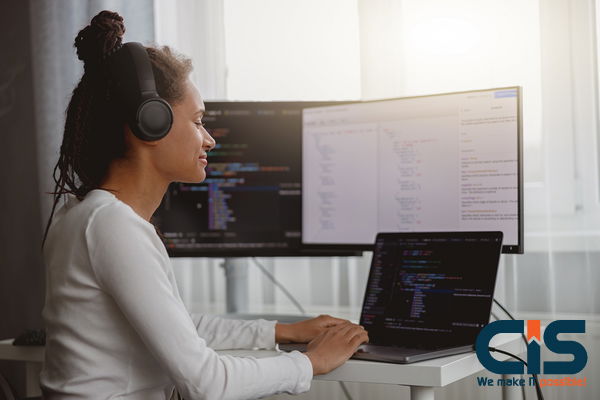
Today, in the business world, a company's competitive edge comes from its ability to utilize the best data analytics platform or BI for DM. Global businesses invest heavily in AI-and-ML-powered Data Management Solutions, including AI-and-ML Assisted DG Platforms. These advanced governance platforms provide maximum value and save unnecessary costs.
In the article Data Governance and Data Quality Challenges in an unsupervised Machine Learning Ecosystem, it is stated that organizations will be investing in advanced data technologies, such as AI and ML, to "achieve security, compliance, and quality at scale."
A modern AI and ML-assisted DG solution's primary goal is to improve Data Quality, Reliability, and Accuracy while preserving the data security and privacy for its customers. Well-governed Data Management practices imply responsible and accurate data usage within the limits of DG policy and procedures. Here are some of the implicit goals for an assisted DG platform.
- Source of data reliability
- Better Data Quality
- Data integration is seamless
- Compliance with regulatory requirements
- Data privacy and security are improved
Last but not least, the most important thing is to protect customer data.
The Modern DG Platform: Embedded AI & ML
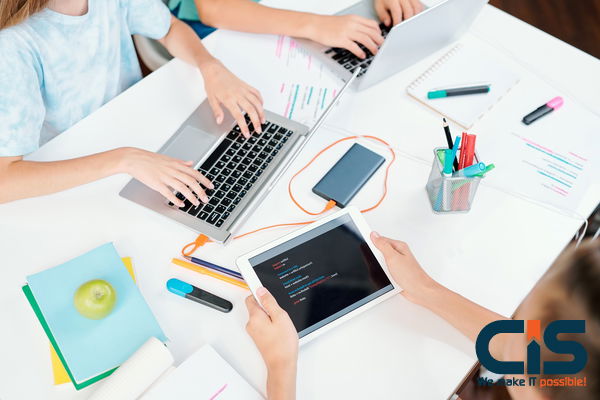
Data Governance challenges are not limited to the data deluge or cloud services. They also do not stop with strict data privacy laws. The problems only get worse when there is a lack of understanding of how people, processes, policies, tools, and technologies can be integrated within a machine learning Data Governance framework.
The current need is for assisted DG or to modernize the DG platforms by integrating AI and ML. All it takes is a simple recognition that AI and ML can automate many crucial DG processes, such as user access control, metadata management (MDM), or data security.
AI and ML: Role in Enterprise Data Governance
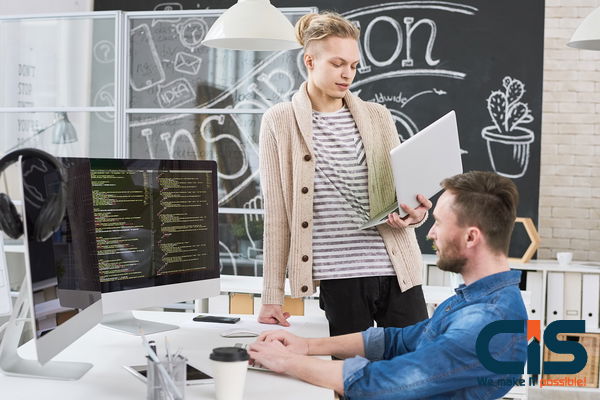
Ann Marie Smith, the author of the book Assisted DG, describes how DG helps ensure that ML models align with "policies and standards for Data Management and Usage" within an organization.
Here are a few examples of AI and ML-assisted DG within organizations.
- Data Stewards Complete Tasks Using ML: The ML algorithms help data stewards monitor the data and, specifically, metadata for millions of data elements within large organizations. Smart algorithms can complete tasks quickly, allowing data stewards the time to focus on more difficult but less laborious tasks.
- Reduced Data Cleaning Time: AI/ML tools reduce the data cleaning time by a significant amount while improving Data Quality. This step increases the organization's reliance on accurate, well-governed data.
- Faster implementation of DG Policies and Standards: Trained ML models implement approved policies and standards faster and with greater accuracy.
An author wrote an interesting article stating that "DG is interdependent with AI and ML." The author believes the business's actions in relation to "good data" are as important as their quality. AI and ML work together to enhance data analytics by automating tasks such as data preparation and cleansing, extracting insights in a matter of seconds from large amounts of raw data, and helping business staff make better and faster decisions.
Want More Information About Our Services? Talk to Our Consultants!
Conclusion
According to a blog post, European businesses have been fined $40.56 million for privacy violations in the first quarter of 2022.
The volume and complexity can overwhelm employees who are required to perform manual data-related tasks in a medium-sized company. Top business executives are not tolerant of outdated data processing, and IT departments are usually overwhelmed with requests for information. Who is responsible? Of course, it's the lack of infrastructure.
Manual processes are often labor-intensive and archaic in nature, which adds to the overhead costs of businesses. Automated data governance can solve these problems. This blog post explains why AI and ML can help DG.