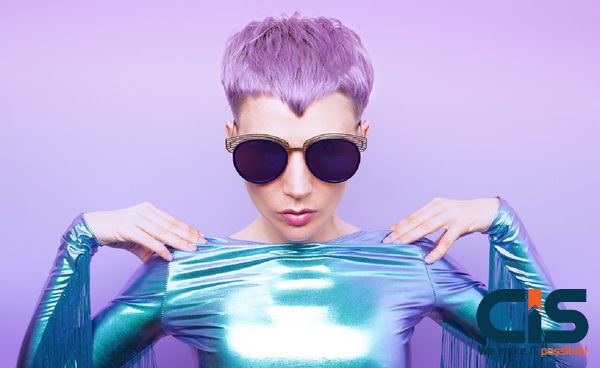
Artificial Intelligence (AI) is revolutionizing supply chains as quickly as other industries. Supply chain managers rely on artificial Intelligence (AI) to manage vast volumes of data, make predictions, connect disparate sources in an increasingly complicated supply chain environment, and quickly meet industry-specific challenges. AI may help address many major obstacles facing supply chain management as well.
According to research, early adopters of AI outperformed slower-moving competitors in terms of inventory levels by 35%, service levels by 66% and transportation costs by 15%. Artificial Intelligence offers several advantages for supply chain management that will only become stronger over time. In this article, we delve into the role of AI in the supply chain, exploring its benefits, challenges, and much more. So, keep reading to gain deeper insights.
What Role Does AI Play In The Supply Chain?
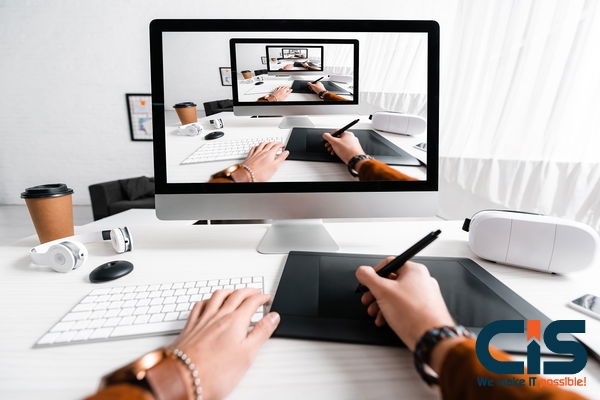
Complex supply chains have grown increasingly complex due to environmental sustainability requirements, broad product portfolios, multiple partners, volatile markets and rising costs. Below we have outlined the important role of AI in supply chain management:
- Demand Forecasting: AI models can create complex forecasts using almost endless data sources to support business decisions, considering multiple market groups, relationships and factors like holiday celebrations or consumer trends.
- Real-Time Inventory Management: AI allows for closer monitoring of stock. By automatically adapting to current conditions and making necessary adjustments, AI helps prevent stock outs or overstocking situations. AI also helps predict when new orders should be placed based on demand and availability.
- Robotics And Automation: Artificial Intelligence can offer great potential if automation is one of your goals. It may dynamically adjust to new issues and demands, including problems surrounding problem-solving needs and improve system efficiencies by rapidly adapting.
- Real-Time Monitoring: AI's real-time monitoring creates end-to-end transparency. For instance, its responsive system can monitor perishable goods to take appropriate actions when its best-by-date approach is used.
The Benefits Of AI In Supply Chain
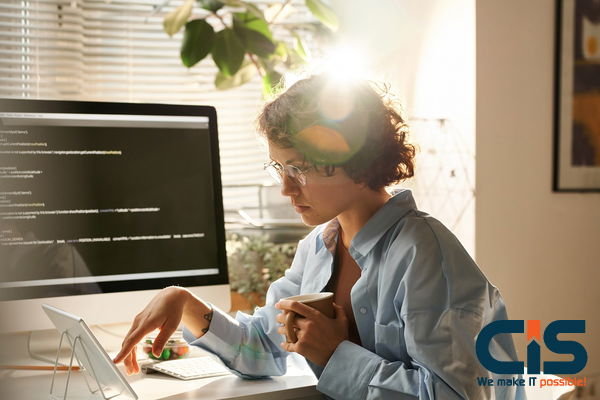
Here we have outlined the important benefits of AI in supply chain:
Enhance Warehouse Productivity
Artificial Intelligence (AI) can assist warehouse management in optimizing productivity by aiding with rack arrangement and layout design. Machine learning (ML) models can provide floor plans that maximize material movement through warehouse aisles, decreasing access time from receiving racks to packing and shipping locations.
AI-enabled forecasting tools assist firms with optimizing warehouse capacities further by assessing demand signals from marketing, production line and point-of-sale systems. Furthermore, employees and robots will find faster pathways for moving inventory around, boosting fulfillment and fulfillment rates even further. AI forecasting tools also aid firms by helping balance carrying costs against inventory carrying costs while optimizing warehouse capacities further by balancing demand signals from marketing, production line and point-of-sale systems.
Lower Operational Costs
Repetitive operations like counting, tracking and documenting inventories can now be carried out more accurately and with reduced labor thanks to AI's capacity for understanding complex behaviors in unpredictable environments and mitigating bottlenecks in supply chains. Artificial Intelligence may save costs by uncovering inefficiencies while learning from repeated actions. AI can save distribution managers and manufacturers costs by minimizing equipment downtime. Intelligent systems that process IoT device data within smart factories can detect faults early or anticipate breakdowns before they occur to prevent financial disruptions and losses.
AI Can Reduce Waste And Errors
AI programs typically perform much quicker in identifying odd behavior in both machines and humans than humans can. Therefore, producers, warehouse managers, and shipping firms have implemented algorithms in their processes and products to detect employee inefficiency, mistakes and weaknesses in products. Cameras installed in delivery vans, assembly lines, and logistics centers feed data into computer vision systems that use artificial intelligence (AI) to inspect work and reduce returns, recalls, and rework.
Time and material waste can be reduced as intelligent systems identify errors made by employees and machines before items are reassembled or shipped to incorrect destinations. Furthermore, intelligent systems can analyze root causes, which examines vast amounts of data to find patterns that explain malfunctions more quickly, helping teams address them more swiftly.
Enhance Inventory Control
Manufacturers are harnessing AI's capacity for more effective and precise inventory control by gathering customer inventory information that will then be fed back through AI forecasting systems to estimate customer demand projections if customer demand changes over time. In such an instance, manufacturing demand projections might need to be adjusted downward if customer interest drops significantly - leading to improved inventory control overall.
Simulation-Based Optimization
Supply chain managers can utilize AI models to gain an in-depth knowledge of complex international logistics networks and identify areas for potential improvements. Artificial Intelligence is increasingly being employed alongside digital twins - visual 3D representations of real-world processes or items, like assembly lines or manufactured commodities.
By operations planners for testing different strategies and tactics (e.g. how much output would increase with capacity added at point A versus point B), without impacting actual operations themselves. Furthermore, simulations made more precise with AI-provided models and control workflows can produce even more excellent precision simulation results than their traditional computer counterparts.
Faster Deliveries
Producers who assemble goods through complex supplier networks depend heavily on quick, well-organized deliveries of components; any delays could wreak havoc with production schedules, and AI is helping producers overcome them to ensure timely deliveries of components. Machine learning can help logistics organizations develop models to optimize and manage delivery routes used by components as they travel along the supply chain.
Shipment priorities may be set based on factors like order volume, contractual deadlines, delivery guarantees, customer importance or product availability; additionally, these models provide more accurate arrival time estimates at each node within their distribution networks while flagging those that could cause more severe complications if delayed further.
Improved Supply Chain Sustainability
Artificial Intelligence (AI) can significantly decrease environmental effects associated with supply chains while simultaneously improving operational efficiencies, thus increasing sustainability. AI may play a part in helping companies reduce energy usage during deliveries by optimizing truckloads and routes for efficiency; using machine learning models trained on fuel savings could assist companies with cutting consumption significantly.
Furthermore, AI also holds promise of cutting product waste along the supply chain: for instance, by considering production planning tools that take into account historical inventory levels, current demand estimates, as well as real-time machine maintenance status updates to avoid overproduction by manufacturing plants using production planning technology from AI production planning solutions like these.
AI can also assist the circular economy - one in which resources are recycled and reused-- by providing insights that support life cycle analysis of completed goods. AI-enabled supply chain planning and sourcing tools may assist suppliers in becoming more transparent while adhering to social and environmental sustainability guidelines, such as paying workers fair wages.
More Accurate Demand Forecasting
Artificial Intelligence (AI) has quickly become the industry standard in forecasting demand based on various external signals such as macroeconomic outlooks, seasonal sales patterns and sales pipeline/marketing lead data as well as internal signals like these. Demand planning software with built-in AI allows supply chain planners to accurately estimate demand while considering any effects from events like recessions.
Read More: AI: A Game-Changing Technology? Cost, Gain, and Impact Revealed!
AI's Challenges In Supply Chains
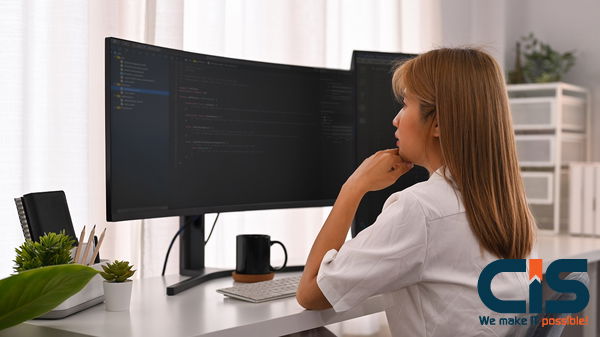
Here we have outlined the important challenges of AI in supply chain:
Training Costs
When it comes to AI implementation and integration into production environments, training the individuals who will interact with these unfamiliar and sometimes alarming systems is crucial - just like with any new technology. Scheduling time off work may require staff members to adjust to change without resistance - this requires downtime as an expense. Partners across supply chains should work collaboratively with AI suppliers or integrators to develop cost-effective and beneficial training programs before any outages; it should also be noted that any such strategy likely comes at some cost associated with doing just this one thing alone.
Initial And Ongoing Expenses
When adopting AI into practice, expenses tend to go beyond buying equipment and software. You don't always have to build machine learning algorithms from scratch - prebuilt models may suffice. They can even be modified for specific supply chain use cases. Businesses should train these models using their data to reap maximum advantages - doing this may take considerable work due to gathering, validating, converting and cleaning enormous volumes of high-quality information that must be stored somewhere before being fed into AI models if proper preparation of high-quality data sets isn't adhered to.
Operating and managing AI systems at scale across global logistics networks takes ongoing work. Even though maintenance does not need as much processing power as training, robust platforms like edge servers or virtual machines are still required for their upkeep and administration. Thanks to cloud infrastructure providers offering managed data science platforms that streamline machine learning model creation processes while automating their progression and supervising artificial intelligence activities can make AI technology increasingly affordable and accessible.
Complex Systems
Artificial Intelligence (AI) systems involve many moving parts. For instance, sensors and devices stream real-time data streams directly into servers powered by GPUs for training machine learning models both initially and over time; edge and cloud servers host production models; applications take action based on patterns or recommendations found; all this must be integrated by organizations through multiple links in global supply chains while continuously reviewing them to adjust functionality, find any bugs and address them immediately.
Conclusion
AI will play an increasingly pivotal role in supply chain management as technology progresses and solutions become ever more sophisticated, making it easier for the average person to use and adapt quickly to any new obstacles. No matter where your artificial Intelligence (AI) journey starts or stops, one essential aspect will always remain consolidating all data sources into one place. Cisin uses electronic data interchange (EDI) as an effective Artificial intelligence solution. For more information about how Cisin's AI services could fit seamlessly into your supply chain to deliver future-ready solutions, explore our options or reach out directly.