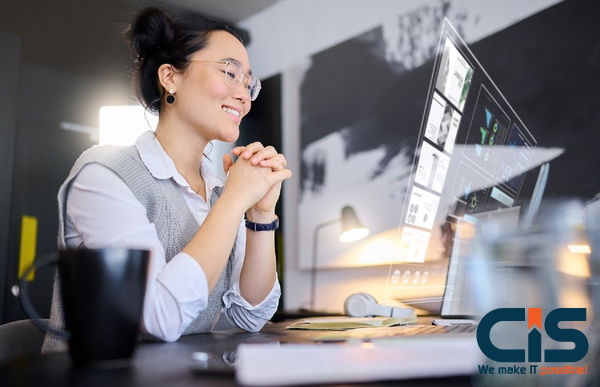
Before we explain these two technologies, it is essential to recall the origin of their technology: Artificial Intelligence. This is the machine's ability to process the information it gathers. These statistics are the outcome of prior experiences. It captures information and then transforms it into knowledge.
What is Machine Learning, and How Does It Work?
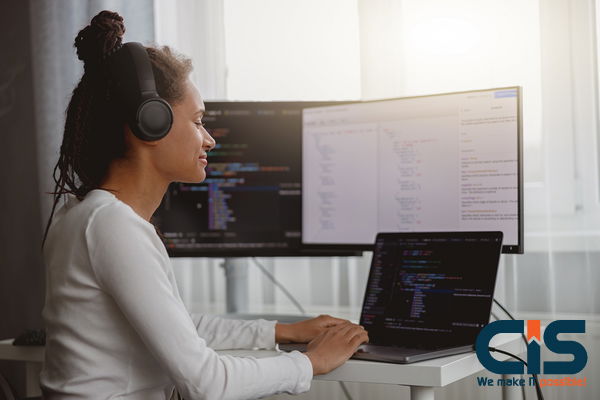
Machine learning, an innovative computer science technique, enables computers to automatically learn from experience and adapt over time without being explicitly programmed. Machine learning analyzes large datasets for patterns before exploiting these discoveries to build predictive models. Businesses can utilize machine learning to increase performance and operations efficiency.
AI and Machine Learning: What is the Difference?
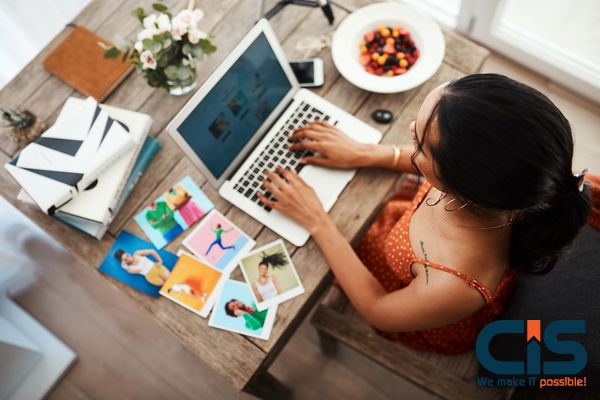
Machine learning (ML) and Artificial Intelligence (AI) are related yet distinct fields. AI concerns the creation of intelligent machines capable of performing tasks that typically require human Intelligence, including problem-solving, reasoning and making decisions, and processing language. In comparison, machine learning (ML) takes this concept further.
Machine learning, a form of artificial Intelligence (AI), allows computers to gradually improve at specific tasks by learning from data. Artificial Intelligence, commonly called AI, encompasses various techniques, including rule-based algorithms, genetic algorithms, decision trees, and other algorithms. AI comes in supervised and unsupervised forms and is utilized by various applications, including self-driving vehicles, chatbots and image recognition software.AI (Artificial Intelligence) refers to all techniques and methodologies for creating intelligent machines; machine learning (ML) is one specific AI technique.
Machine Learning in Business Software Examples
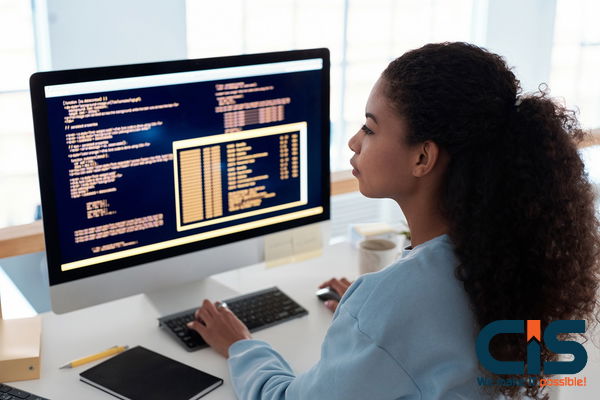
AI has transformed our world through products like ChatGPT and Notion AI. Document automation can be leveraged through machine learning as it eliminates human error.
Auto-Find is designed to quickly extract information from documents for approval or correction by an operator. At the same time, Advanced auto-find is more complex, categorizing fields by type and using regular expressions to detect irregularities within data structures while providing active AI training capabilities. Machine learning and AI will save time while freeing you to focus on tasks in your Excel.
Is Data Science and Machine Learning the Same Thing?
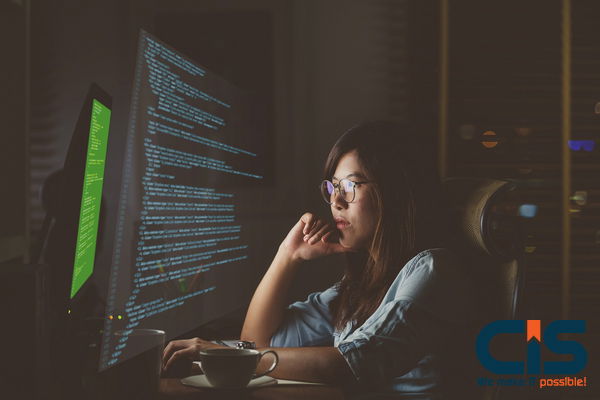
Machine learning and data science are complementary but distinct concepts.
Data science is an interdisciplinary field that utilizes algorithms, systems, scientific processes, and methods to gain insights from unstructured and structured data sources. Data scientists apply various tools from diverse fields like computer science, statistics, and domain knowledge in order to solve complex problems by making sense of data sets. On the other hand, machine learning is a field of AI that involves teaching machines how to improve over time at specific tasks by gathering and processing data. Machine learning uses models and statistical algorithms to help machines pattern recognition, classify information accurately, and foresee potential future outcomes.
In its broadest sense, data science refers to various techniques for extracting insights from data. The data science process includes many tasks like cleaning data, analyzing it and forecasting its outcomes - data cleaning can even include training machines with machine learning to understand data faster. Data cleaning techniques often used by data scientists can be seen as a subset of machine learning's predictive analytics techniques used with big data sets like Twitter.
What Are The Many Forms Of Machine Learning?
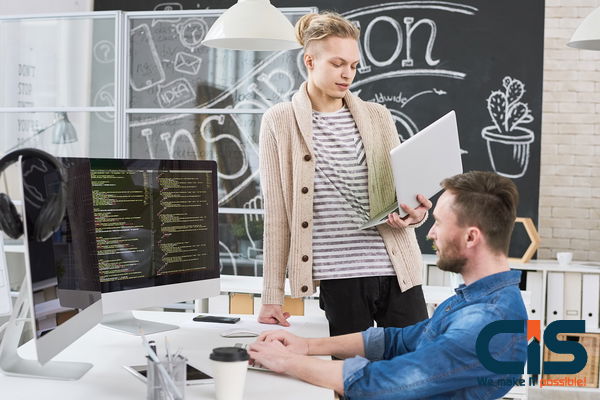
Machine learning can be divided into three different branches, with each type being best suited to certain tasks or applications. Let's find out!
Learners Are Supervised During Their Learning
Machine learning involves two forms. One form involves training an algorithm with data labeled with desired outputs and measuring their predictions against test data sets. Supervised learning can be used for image categorization, speech recognition, and spam filtering applications.
Unsupervised Learning
Unsupervised machine learning (UML) is an unsupervised training method in which an algorithm uses unlabeled input data without any labeled outputs; rather, its goal is for it to learn how to recognize patterns without guidance, producing clustered or grouping of data as output from its processing. Unsupervised learning can be used effectively for anomaly detection, market segmentation, and recommendation systems.
Reinforcement Learning
Machine-learning algorithms utilize feedback received from their environment to make decisions based on information acquired through learning algorithms that utilize actions that maximize rewards while decreasing negative results. Reinforcement learning has long been utilized in robotics and the control of autonomous vehicles. Other methods include semi-supervised machine learning, where data are labeled or unlabeled, and deep learning with artificial neural networks to extract relevant insights from data sets.
What Exactly Are Machine Learning Algorithms? (MLA)?
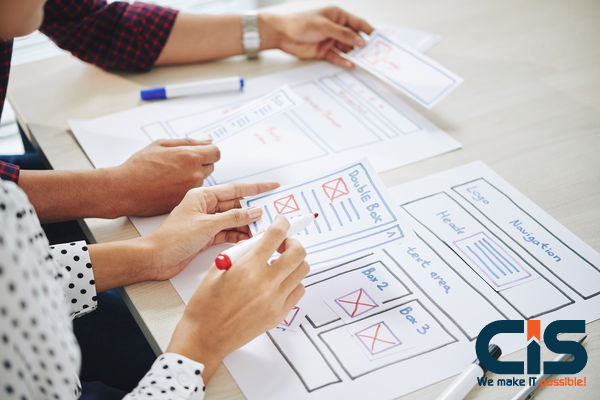
Computer programs called machine-learning algorithms allow machines to take the initiative in learning from experience without explicitly being programmed for it. By employing these algorithms, machines can improve their performance automatically through these machine-learning programs, learning data to make predictions or decisions based upon it. Let's look at some different kinds of machine-learning algorithms in more depth.
Learners Are Supervised During Their Learning.
Machine learning algorithms of this nature typically involve training a model using labeled data - that is, data that has already been labeled correctly - before applying what has been learned to predict new data that has yet to be labeled correctly. The machine learns by using data acquired via labeling to predict unlabeled records that come its way.
Unsupervised Learning
An unlabeled dataset machine-learning algorithm involves training a model with unlabeled datasets to recognize patterns and relationships within them; such an approach enables tasks like clustering and anomaly detection.
Reinforcement Learning
An adaptive machine-learning algorithm of this sort involves training its model through trial and error, using positive and negative feedback to maximize rewards. Machine learning (ML) algorithms have become an essential element of modern technologies and are increasingly popular as businesses look for competitive advantage through data use. Machine learning algorithms have applications in fraud detection, image recognition, natural-language processing, and predictive maintenance applications, among others.
Deep learning replicates how we acquire certain kinds of knowledge through human experience. Data science involves predictive models and statistics; hence, deep learning should be part of its practice. Deep learning offers data scientists an indispensable resource when collecting, analyzing, and interpreting large volumes of data. Deep learning provides an easy solution for automating predictive analytics. Deep learning algorithms gradually become more sophisticated as time progresses.
Imagine that a toddler's first word was a dog and, upon seeing objects with dogs on them, began pointing and saying the word - without parental intervention -- learning what constitutes or doesn't constitute a dog (such as parent statements like, "This puppy belongs here or no it doesn't.") by doing this they learn what type of puppy exists (or doesn't). As they point at various objects with this definition, the toddler becomes aware as each object pointed is answered positively or declined as a dog). Unknowingly this toddler clarifies complex abstract concepts related to dogs through an ascendant structure where knowledge gained at each layer is used in creating yet another level within this hierarchy -- without conscious knowledge gained at each layer being applied at this level as knowledge gained at each layer becomes used at creating yet another level within this hierarchy!).
Deep Learning: What is it?
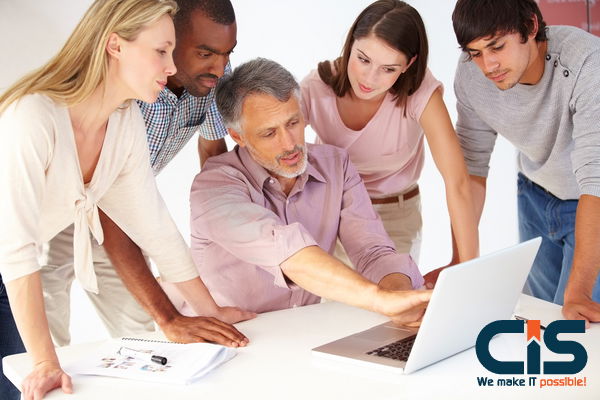
Artificial Intelligence's (AI) deep learning method instructs computers on handling information using mechanisms similar to the human brain. Deep learning models can recognize patterns and complex data in images, texts, sounds, and other types of data, producing precise insights and accurate predictions as a result. Deep learning models may also automate tasks that need human intelligence, such as describing a picture or converting audio recordings to text format.
What is the Importance of Deep Learning?
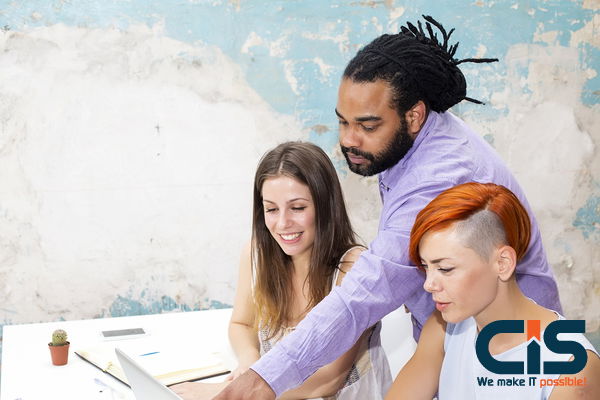
Artificial Intelligence (AI) refers to teaching computers how to learn and think like humans, using deep learning technologies as part of this process. Many AI products employ deep learning technologies for this purpose.
Digital Assistants with voice activation remotes, and fraud detection/face recognition software have quickly become key elements in emerging technologies, including virtual reality and self-driving vehicles. Data scientists use deep learning models, computer files they create using an algorithm, to train computers. Businesses then employ these models in various applications to analyze and predict results more efficiently.
Deep Learning: What is It Used for?
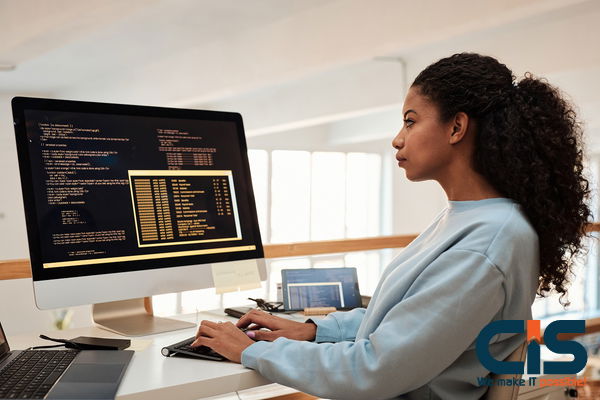
Deep learning finds many applications across industries, including automotive, aerospace and manufacturing, and medical research. To give some examples of deep learning applications:
Deep learning technology is being leveraged by autonomous vehicles to detect pedestrians and road signs automatically, defense systems to flag areas of interest in satellite imagery automatically, and medical image analysis to automatically spot cancerous cells. Deep learning algorithms are employed by factories to automatically detect when objects or people come within an unsafe distance from machines through four categories of applications: computer vision, speech processing, Natural Language Processing (NLP), and recommendation engines.
Computer Vision
Computer vision technology enables computers to analyze images and videos and extract useful information using techniques like deep learning. Computers are now capable of understanding images as quickly and clearly as humans do - this has many practical uses beyond image analysis, including those listed here: Image and video archives are automatically screened to detect inappropriate or unsafe material. Facial Recognition technology enables the automatic detection of open-eyed people, those wearing glasses, and those sporting facial hair.
Classifying images to recognize brand logos, clothing items, safety gear, and any additional details in them.
Speech Recognition
Deep learning models have enabled deep learning models to analyze speech accurately regardless of pitch, accent, language, and tone variations. Speech recognition software such as Amazon alexa uses deep learning algorithms for this function, while speech transcription systems use speech recognition software automatically transcribe audio files into text form.
Calls are automatically classified to aid call center agents. Real-time transcription of clinical discussions into document processing. Subtitling videos and recordings accurately to reach more viewers.
Natural Language Processing (NLP)
NLP-ML (Natural Language Processing Machine Learning) is an artificial intelligence subfield that aims to use computers as interpreters of human languages to simulate translation from language into code for processing by humans.
NLP Machine Learning involves developing algorithms and Statistical Models to process natural language data such as speech, images, or text for tasks like language translation, sentiment analysis, text classification, or information extraction. This data becomes useful in tasks like translation, sentiment analysis, or text classification, and extracting essential knowledge for translation or data extraction tasks. Machine Learning models trained for natural language processing typically utilize large volumes of text from articles, books, and social media posts for training purposes. Their models learn to detect patterns within this information before applying that knowledge to generate or predict new language generation or prediction forms.
NLP Machine Learning finds applications across industries. One example is customer service chatbots and voice assistants utilizing NLP; sentiment analysis provides another. Additionally, NLP can monitor social media for monitoring purposes.
Recommendation Engines
Deep learning technology can craft personalized recommendations and monitor user activity. Users may benefit from discovering new services and products by analyzing their behavior patterns; in fact, Netflix and Fox use deep learning video recommendation systems extensively as they serve their audiences with personalized video recommendations via deep learning frameworks.
What Exactly is the Distinction Between Deep Learning and Machine Learning?
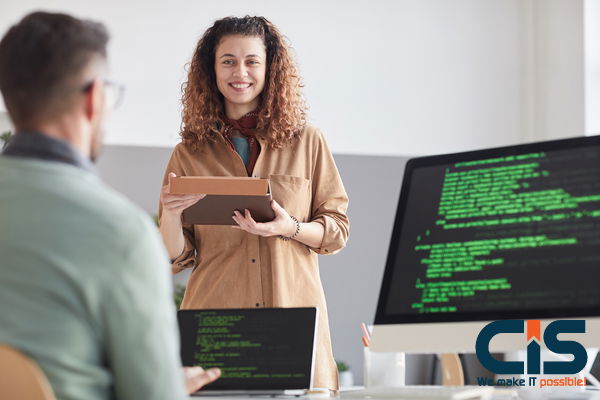
Deep learning, as part of Machine Learning, involves employing several layers of neural network. Both techniques use algorithms to train on data to predict or make informed decisions - however, there are notable distinctions.
Complexity: Deep Learning models are more complex than machine learning models; they consist of many layers and interlinked nodes, which can help extract abstract features from data sets. Traditional machine-learning models require feature engineering by hand to optimize model performance; deep learning models, on the other hand, have learned to recognize features automatically from raw data sets - saving both time and improving accuracy.
Data requirements: Deep learning model training requires large datasets in order to perform effectively; on the contrary, traditional machine learning models tend to perform better with smaller data.
Deep learning models: Deep learning models may prove more efficient for complex tasks like natural language processing or image recognition than machine learning models. However, more computing resources may be required for their training and deployment. Deep learning is a form of machine learning that uses advanced methods and data sets to perform complex tasks precisely. However, more computational and data resources may be needed for training than standard machine learning approaches.
How May Machine Learning and Deep Learning Be Applied in Our Organization?
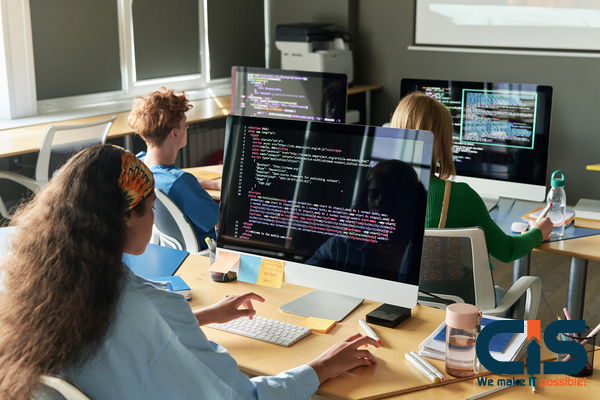
Deep learning and machine learning are key to our company's success in scaling, achieving higher performance, and cost savings. These technologies may also offer the following benefits:
- They personalize customer service: This allows for automatically identifying user preferences, allowing personalized products to be delivered. This improves the customer's perception of us and increases our loyalty. This technology is used by platforms like Spotify, YouTube, and Netflix to recommend other content based on what we have enjoyed.
- Process automation: Naturally, the automation of routine tasks is one of the most important contributions of both technologies. Automating routine tasks takes much time and effort and doesn't add value. Our systems are able to detect and deal with the required processes using machine learning.
- Reduce errors: Automatic Learning means that mistakes are not repeated because of the management systems used in the organization. It will become more resilient the longer it remains in the system.
- Preventive actions: Machine learning tools can be used to prevent errors and bugs. Artificial Intelligence can block any action that could compromise or risk the development of products and services.
Other Uses
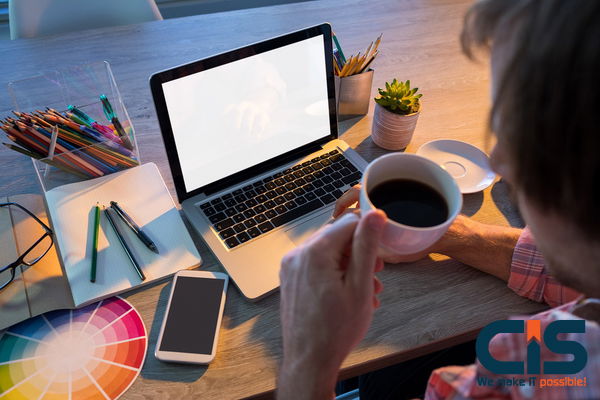
- Cybersecurity: This technology is a significant contributor to protecting neural networks, systems, and terminals from cyberattacks. Machine learning can prevent most malware from being injected by using similar code.
- Fraud detection: With the technology we have, it is possible for transactions to be identified as legitimate and those that are not. Even the mismanagement or misuse of resources can be revealed. This function can be achieved when financial movements are mapped.
- Medical diagnosis: These technologies, when integrated into the health system's technological tools, help insurers be more aware of possible health problems based on the frequency of medical consults. These technologies are also more affordable and can recommend different medications, among other things.
- Increases security and integrity of information: Cloud solutions are another service that supports these two aspects of AI.
What Function Does Machine Learning Play in Business?
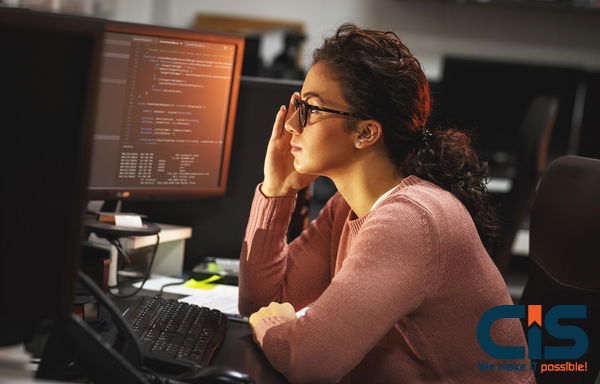
Machine learning has quickly become an invaluable asset to businesses - from marketing and customer service, risk assessment and forecasting, to risk mitigation and forecasting. Amazon uses machine learning to optimize prices, predict demand and recommend products, while Google uses machine learning for personalized emails and suggesting searches based on previous search activities.
Related:- Major differences between artificial intelligence and machine learning
Machine Learning Benefits for Business
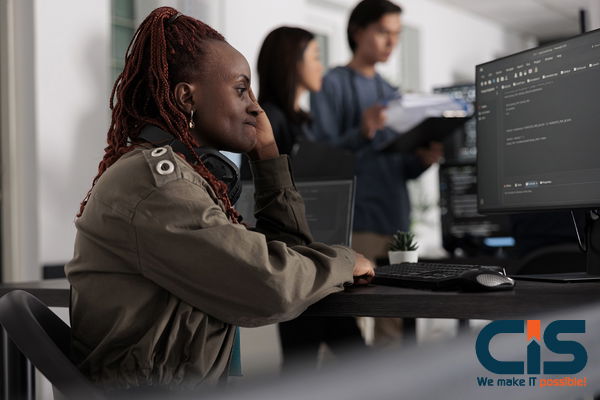
Machine learning offers businesses numerous advantages that cannot be overstated. Not only can machine learning increase business productivity and lower expenses, but it can also enhance customer service.
Machine learning offers numerous advantages for businesses. Below are just a few.
Efficiency and Productivity Improvement:
Machine learning algorithms automate time-consuming and repetitive tasks that keep employees from performing more important work; this frees them up for more productive duties that help boost efficiency and productivity across various business functions.
Cost Savings:
Increased efficiency is one of the easiest and simplest ways to cut costs for any business process, and machine learning can assist in this effort by automating processes and recognizing patterns within data, which is especially useful in marketing and operations.
Customer Experience:
Machine learning can help businesses personalize interactions with their customers for enhanced customer engagement. Machine learning algorithms may allow companies to make recommendations based on customers' past purchases and browsing histories.
Accuracy and Quality:
Machine learning helps increase accuracy and quality when entering data, performing analyses, or making decisions. It reduces mistakes while increasing overall performance.
Predictive Analytics:
Machine-learning algorithms use machine-learning techniques to accurately forecast future trends and outcomes and give businesses key insight into making more strategic business decisions.
Fraud Detection:
Businesses can use machine learning algorithms to detect patterns indicative of fraudulent activity to prevent and detect it. This aids both their prevention and their detection efforts.
Machine Learning can enhance a supply chain operation's effectiveness by optimizing levels of inventory, anticipating demand, and pinpointing improvement areas. Machine learning can be an incredible asset to businesses. It can improve operations, lower costs, and give them a competitive advantage in their fields - take a look below at some common applications of machine learning in business!
Customer Service Machine Learning
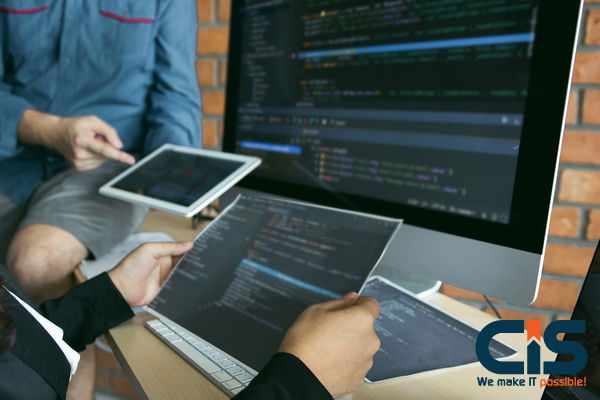
Machine learning can give customers a personalized service experience by increasing customer service efficiency. Machine learning can also help enhance customer service in various other ways.
Chatbots
Chatbots can be trained to respond instantly to customer queries using machine learning algorithms, offering businesses 24/7 customer service capabilities. Programmatically adapted chatbots may respond with personalized responses tailored to past interactions between themselves and customers.
Analyze of Sentiment
Machine learning-powered algorithms can be utilized to analyze customer feedback and gauge its sentiment analysis. Companies use this approach to comprehend customers' needs and preferences better while adapting their products or services.
Personalized Recommendations
Machine learning algorithms enable customers to get personalized suggestions based on past purchase history for products and services they might like, improving both satisfaction and loyalty among their customer base.
Predictive Analysis
Machine learning algorithms can identify customers at high risk for churning, giving businesses a proactive means of responding to them and responding with products or services likely to appeal to these individuals.
Voice Recognition
Machine learning algorithms designed to interpret customer voice commands can enhance accessibility and allow for hands-free interactions, making life simpler for the customer and improving accessibility for everyone involved. Machine learning enhances customer service by offering quicker, more tailored responses. Businesses can leverage machine learning to better comprehend customers' needs and deliver better service to meet them.
Machine Learning in Business
Machine learning can also help operations reduce costs and boost efficiency by cutting costs while improving efficiency. Machine learning offers another powerful solution for improving operations efficiency while simultaneously decreasing spending costs.
Fraud Detection
Machine learning algorithms can identify data patterns indicative of fraud. Businesses can leverage this capability to detect and prevent credit card and insurance claim scams.
Supply Chain Management
Machine learning algorithms can enhance supply chain operations by anticipating customer demand, optimizing stock levels, and pinpointing improvement areas- this decreases business costs while simultaneously improving customer satisfaction levels.
Predictive Maintenance
Machine learning algorithms offer businesses an effective means of planning preventive maintenance before equipment breaks down, thus decreasing maintenance and downtime costs while increasing operational efficiencies and, thus, business efficiency. Businesses can enhance customer satisfaction through machine learning to streamline efficiency and cut costs, providing insights and forecasts difficult to obtain through traditional means and helping make better business decisions.
Machine Learning Projects: Challenges for Business
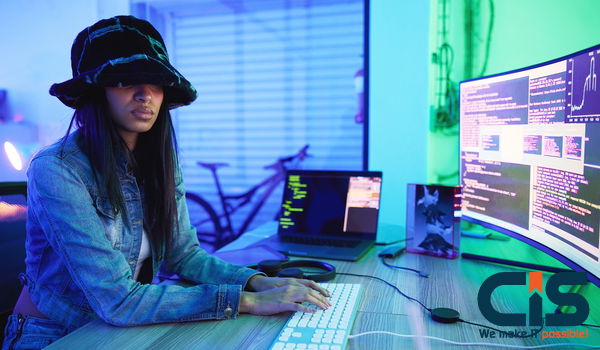
Machine learning offers numerous advantages to companies looking to implement it; however, there may also be challenges associated with its implementation for organizations looking at this method of data analysis. One key area where data privacy concerns could arise for businesses looking into this form of analysis. To avoid legal penalties related to data collection practices in their industry's regulations.
Data quality and volume present another challenge. In order to learn, machine learning algorithms require large volumes of information that must be collected, managed, and stored securely - many businesses require assistance in doing this work themselves - while the information used by machine learning projects may contain inaccurate or incomplete details that affect its accuracy. Businesses using machine learning should implement appropriate security measures to safeguard sensitive information against unapproved access or theft, including encryption, audits, and controls on access.
Machine learning projects entail data science, programming, and statistics expertise from multiple areas; unfortunately, many businesses find it hard to retain or find high-skill level individuals capable of creating and overseeing such endeavors - this often delays or fails their projects altogether. Understanding machine learning algorithms and integrating them with existing systems and processes can be challenging and intuitive, which makes the task all the more daunting for businesses with increasing data volumes and increasingly complex algorithms. Companies may need assistance scaling their machine-learning projects to meet demand.
Cost and return on investment remain major obstacles in machine learning projects, particularly those developed and maintained for businesses. Machine-learning initiatives typically incur high development and maintenance costs which require significant investments of hardware, software, and human talent; businesses must demonstrate a return on investment for these efforts to gain stakeholder support and justify investments made.
Machine Learning and Business: the Future
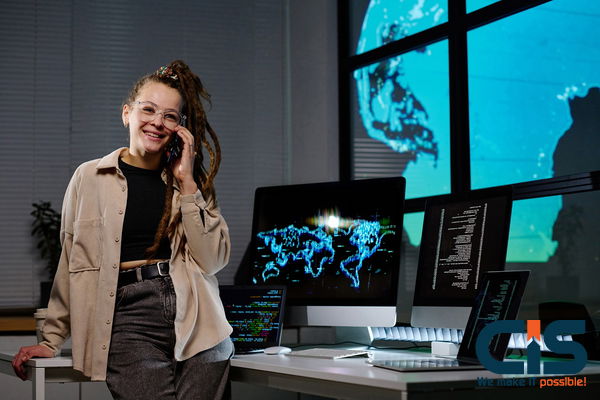
Gartner predicts that by 2022 over 40 percent of enterprise apps implemented by service providers will incorporate AI technology, shifting software creation from human beings to machines. Machine learning's future applications and uses are endless - helping businesses predict faster while making better decisions with machine learning.