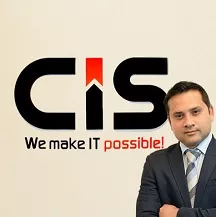
Contact us anytime to know more - Kuldeep K., Founder & CEO CISIN
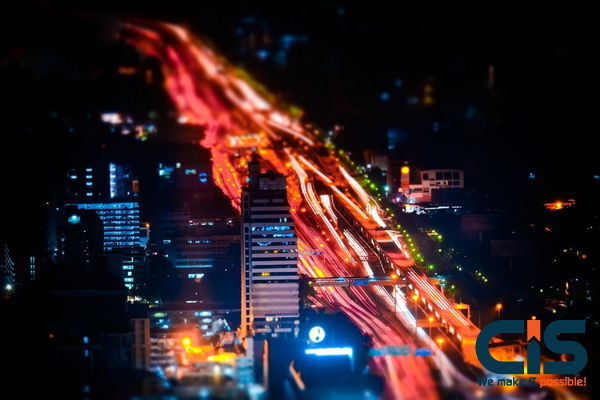
A well-liked trend in AI and machine learning projects is self-driving automobiles. Many advantages of autonomous driving include simplicity, effectiveness, and safety. For instance, self-driving automobiles could lower traffic jams, congestion, and pollutants. Many advantages of autonomous driving include improved convenience, effectiveness, and safety. For instance, self-driving automobiles could lower pollutants, traffic jams, and congestion.
High-impact research issues in autonomous cars can be found in machine learning (ML), an abundant source (AVs). They include user-machine communication, multiagent planning, intelligent infrastructure, perception, state estimation and probabilistic modeling, time series prediction, gesture recognition, robustness guarantee, and real-time restrictions. This workshop intends to promote inter-field conversations within ML subfields to accomplish the shared objective of an autonomous vehicle.
By lowering traffic accidents, promoting independence, and motivating younger generations with concrete instances of ML-based technologies that are readily apparent on neighborhood streets, autonomous driving as an application of ML has the potential to improve society significantly.
What Is Machine Learning?
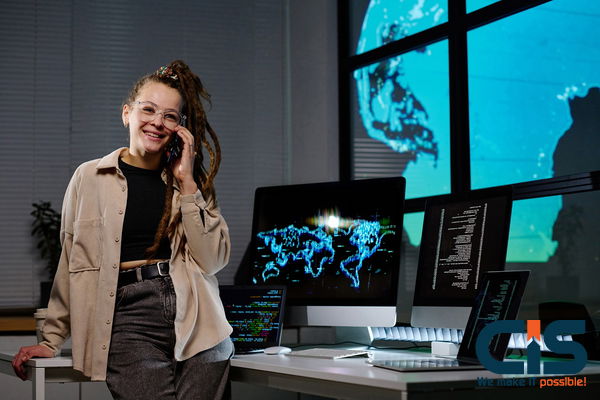
Even though AI is not involved, it is a crucial stage in the process. Programming is not necessary because this is a machine-learning process. You can learn more quickly as a result of the ease of usage. When developing dot-net websites, you can use this to combine input and output effectively.
This skill comes through experience and can help with task performance. It offers a trustworthy plan and upgrades the system's knowledge. The use of AI is in machine learning. Machine learning is the process of using data-driven mathematical models to help a computer learn without explicit instructions. This enables a computer system to grow and learn from its errors.
The next phase in developing and utilizing AI is machine learning (ML). Neural networks, computer programs modeled after the human mind, are the foundation of machine learning. These networks can categorize data based on the elements they include (such as photos of dogs or heavy metal songs). With a reasonable degree of accuracy, ML employs probabilities to make predictions and judgments about data. It is also advantageous to hear opinions on its accuracy. To increase its odds of making wise decisions in the future, ML can alter how it analyzes data and what data it deems significant. The use of ML to find shapes is helpful. It can recognize the letters and objects needed for transliteration.
A machine can learn from past errors and advance by using machine learning and artificial intelligence. Explicit programming is not necessary for machine learning. It uses algorithms and extensive data analysis to make sensible decisions. Artificial intelligence can be attained through machine learning. This area of artificial intelligence uses algorithms to find patterns and draw information from data automatically. They then apply the knowledge to decide more wisely.
To enhance machine learning's perception, cognition, and behavior, programmers can examine and evaluate it. Deep learning, a more complex variety of machine learning, advances the process. Deep learning models use large neural networks to comprehend data like the human brain. Without human assistance, they can understand complex patterns and make predictions.
The Importance Of Machine Learning
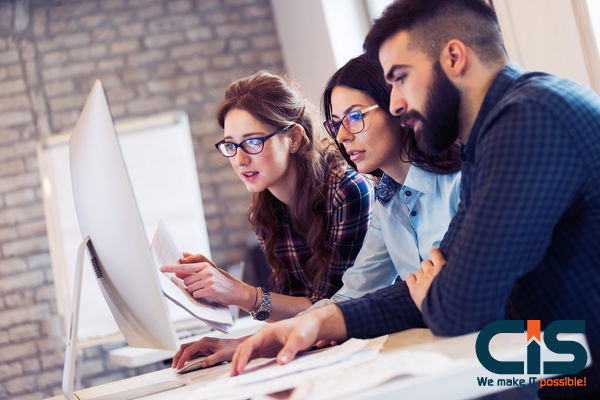
Why is machine learning used? Due to rising data volume and complexity, convenient and affordable access to computing power, and high-speed Internet, machine learning is becoming increasingly crucial. This element of digital transformation enables the development of straightforward models that evaluate huge, complicated data sets quickly and precisely.
There are various methods to apply machine learning to lower expenses, enhance quality of life, and control risk. In addition to enabling self-driving cars and detecting cybersecurity breaches, it may also be used to advertise products and services. Machine learning is becoming more widespread as we have more access to data and processing capacity.
It is expected to be incorporated into many facets of our daily life. Since the beginning, machine learning has existed. The term "machine learning" was initially used by an IBM computer scientist who significantly contributed to AI and video games. Checkers-playing computer software was made by a scientist. The program employed algorithms and played games to gather more data to forecast future events.
Use Of Machine Learning
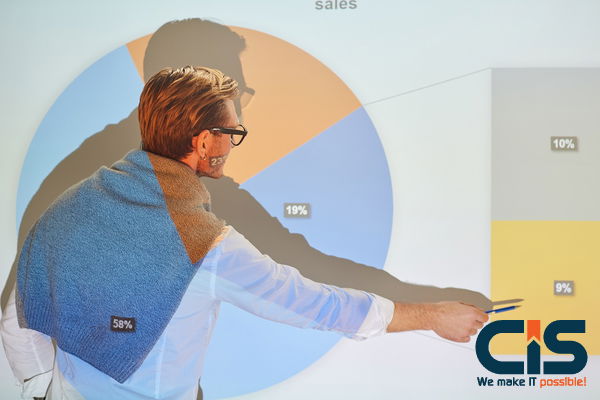
For years, we have been using ML to filter email spam. The Multinomial Naive Bayes Classifier is an easy spam filter. With machine learning, you can discriminate between spam and non-spam phrases. So, by comparing the frequency with which spam terms appear in penalties with the frequency with which spam terms you have not regarded spam, you may determine whether any new sentences you get via email satisfy your spam criteria. Online marketers use similar ML techniques to serve adverts depending on user activity. Automation of routine operations, rule-based procedures, and predictive analysis is facilitated by machine learning (ML).
Machine learning is essential because it enables organizations to identify trends in customer behavior and patterns in their operations. Also, it makes it easier for new product creation. Machine learning is a significant part of many profitable business process outsourcing today, like Uber, Google, and Facebook. Machine learning has become popular among companies looking for a competitive edge.
What Is Autonomous Driving?
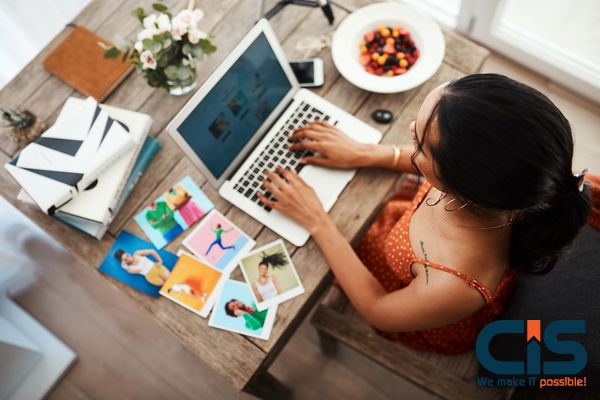
A vehicle that is self-driving or capable of being driven without human intervention is called an autonomous vehicle. The degree of automation varies among the many types of self-driving cars. Six of these levels have been adopted by The Society of Automotive Engineers. The terms "self-driving" and "driverless" also describe autonomous vehicles. The phrase "self-driving vehicle" is becoming popular as these technologies develop.
Autonomous vehicles use sophisticated artificial intelligence (AI), machine learning (ML), and machine vision (ML) systems to "understand" the environment and respond to commands. Sophistic computer vision functions and intricate sensors and actuators are coupled to build a constantly changing picture of their environment, recognize adjacent persons and cars, calculate distances, and detect uneven surfaces on roads and sidewalks. Autonomous vehicles might be connected to external devices like intelligent traffic lights or highways, even if many of these concepts are still merely theories.
Algorithms For Machine Learning
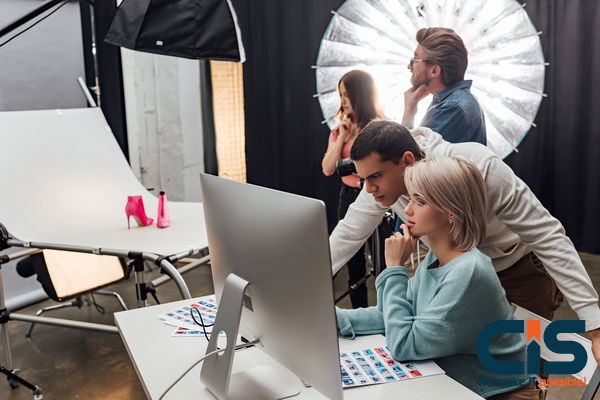
Fully autonomous cars will soon be on the roads, although semi-autonomous vehicles with ADAS are unavailable. Radar, lidar, and sonar sensors provide data to the program that controls the autonomous vehicle's infotainment system. Cameras, too. The vehicle then recognizes barriers and anticipates its next step. Driver motions and speech recognition can be tracked by machine learning algorithms, which can then translate them into the vehicle's system. It is capable of four things in an autonomous vehicle.
Supervised Algorithm
Keep learning until you make the fewest errors possible.
Unsupervised Algorithms
Decoding data helps you learn. Based on the data's similarity, they use it to find patterns and subgroups of data.
Reinforcement Algorithm
They fall between the first and second categories. They analyze all potential outcomes before deciding on the best one. They then take something away from it.
Want More Information About Our Services? Talk to Our Consultants!
Machine Learning Algorithms For Autonomous Driving
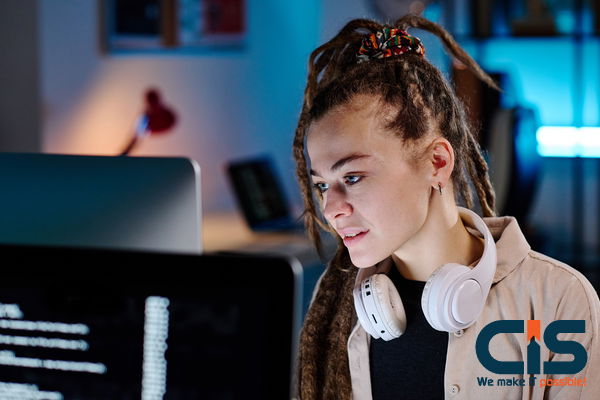
Regression Algorithm
The findings can be evaluated after calculating the association between two or more variables. A statistical model of the relationship between an object and its position is built using repetitive surroundings elements. This enables picture sampling-based online detection.
Categorization Algorithm For Pattern Recognition
Recognition of patterns is essential for classifying objects. By identifying edges and fitting line segments or circular arcs around them, ADAS data can then be filtered. The final components necessary for object recognition are created by combining these parts.
The Majority Of Clustering Algorithms Are
This is helpful when finding or detecting an object in low-resolution photographs is challenging. In these situations, clustering can extract structure from the data points.
Decision Matrix
This algorithm controls how self-driving automobiles move (make left- or right-hand turns or apply brakes). It evaluates and rates information and values performance relationships.
Autonomous Driving: The Benefits
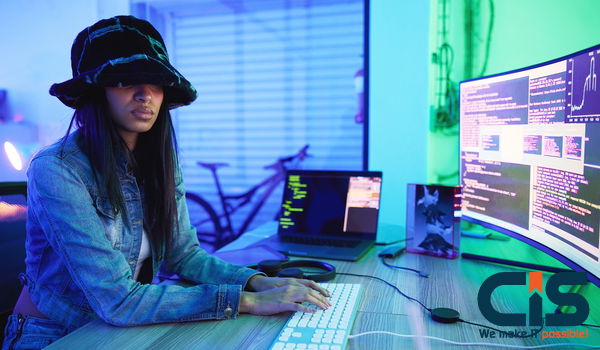
Depending on how autonomous the car is, driving has different advantages. Still, even simple maneuvers like staying in your lane can improve your enjoyment of the experience. These are the benefits of higher autonomy:
Safety
Autonomous vehicles have a lot of advantages. One of the biggest benefits is safety. Autonomous vehicles could significantly reduce accidents and fatalities; there were around 6 million auto accidents in the US. This caused 2.3 million injuries and about 42 915 fatalities. The majority of these accidents were the result of human mistakes. Autonomous vehicles, which are carefully constructed and can reduce the number of fatalities and accidents on the road each year, do not have these same hazards.
Efficiency Increases
Traffic pollution and congestion can be decreased with autonomous vehicles. Because they are more effective, autonomous vehicles can use less fuel when commuting. Autonomous vehicles may assist in lessening or eliminating traffic bottlenecks by coordinating their movements and exchanging information with one another. Because of their lower accident risk, autonomous vehicles are lightweight and can lessen their environmental effect.
Greater Accessibility
Moreover, autonomous vehicles provide improved accessibility. Autonomous vehicles can also give elderly or disabled individuals greater independence.
Improved Standard Of Living
Autonomous vehicles have the potential to improve the quality of our lives greatly. Imagine viewing a movie while driving for nine to ten hours on an entire project. Imagine being able to use the rowing machine to get fast exercise while you're driving to work. Autonomous vehicles make all of this possible.
Autonomous Driving: The Disadvantages
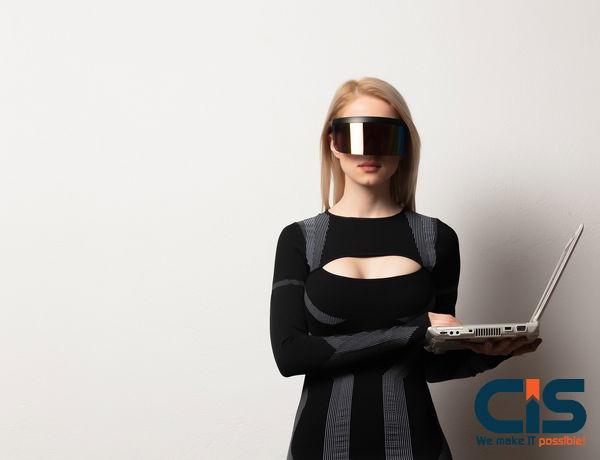
Let's examine the main drawbacks of autonomous vehicles.
Difficulties With Data Protection
It might first generate a cyber security issue because it is always connected to the outside world. Even managing the road network can be affected.
High Implementation Cost
Around 5G network coverage, autonomous vehicle infrastructure is built. Because of this, it may take a while for governments to make the necessary infrastructure investments to operate at their best.
Vehicles At A High Price
Although the cost of producing their implements has significantly decreased, these savings are insufficient to make them a financially viable alternative for the average family. It will take time for them to become a regular occurrence for the middle class.
Types Of Machine Learning
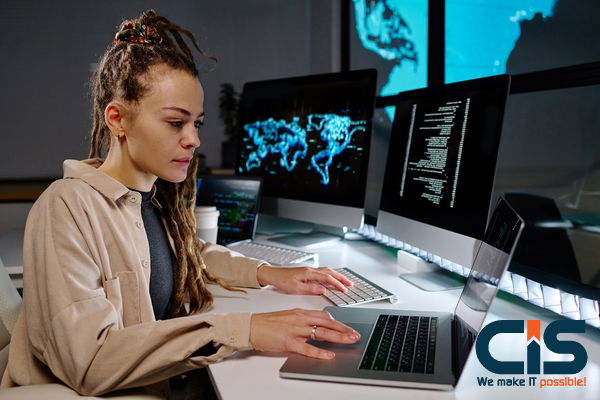
Machine learning algorithms can be trained in various ways, each with specific benefits and drawbacks. Machine learning may be divided into these main categories with the aid of these tools and learning strategies:
Monitoring Machine Learning
Supervised machine learning is one of the most used methods in machine learning. Because the algorithm learns from a collection of training datasets, this is sometimes called supervised learning. This is comparable to a teacher instructing his class. When the algorithm reaches a particular degree of dependability, it stops learning. With tagged data, data scientists train algorithms. These give the algorithm the variables it should look for correlations between. The algorithm's input and output are specified.
The monitoring of this kind of machine learning (ML) is necessary. Machines can anticipate outputs using the training they receive from labeled data after training. Several input and output parameters have been mapped using a dataset that has been labeled. The machine is given the parameters for the input and output. The device is introduced using the test data to forecast the outcome.
Read More: Machine Learning And Deep Learning Are Becoming Increasingly Important For Businesses
Automatic Machine Learning
Unsupervised learning, a less popular type of machine learning, only takes input data and has no output variables. Contrary to supervised learning, unsupervised learning is a type of machine learning that yields erroneous results. This kind of machine learning looks at the underlying structure of the data. Automated methods can determine the data's past, present, and future designs. These methods are employed in machine learning and are based on unlabeled data.
The algorithm searches for connections between various data sets. To train algorithms, predetermined data is used. To create forecasts and offer recommendations, predetermined data is used. Unsupervised learning is a type of training where supervision is not necessary. The system can guess the result after receiving an unlabeled dataset and proceed carelessly. Unsorted data is categorized using unsupervised learning techniques based on similarities and contrasts.
Semi-Supervised Learning
Any of the techniques above can be integrated with machine learning. An algorithm may be fed largely with training data by data scientists. The model training can still learn from and explore the data.
The best aspects of supervised and unsupervised machine learning are combined in semi-supervised knowledge. Semi-supervised learning trains its algorithms using both labeled and unlabeled data. Both datasets are used in semi-supervised learning. The majority of the major issues are addressed here. Consider a college student as an illustration. Under the supervision of an instructor, a student can study a subject through supervised learning. When students learn a subject at their own pace without teacher supervision, this is known as unsupervised learning. When a college instructor mentors a student through the course, this is called semi-supervised learning.
Reinforcement Learning
Data scientists frequently utilize reinforcement learning to teach robots to adhere to a certain set of rules. Labeling tasks completing algorithms can be developed by data scientists, who can then provide it with cues that can be positively or negatively conditioned. Very likely, the algorithm will decide what to do next. To reinforce learning, feedback is essential. Thanks to the hit-and-test technique, the AI component can assess its environment before acting. It gains knowledge from its prior errors and performs better. Every accomplishment is celebrated, and every mistake is penalized. The business goal of the reinforcement learning component is to reinforce good behavior as frequently as possible.
Reward learning does not need labeled data, in contrast to supervised learning. Instead of deliberate learning, agents learn through their experiences. There are video games to consider. Reinforcement actors' actions influence the environment. As feedback, the reinforcement agent may get rewards and penalties. The final score of the game will be affected by this. The rating for entire team agents will be excellent.
Machine Learning: The Benefits
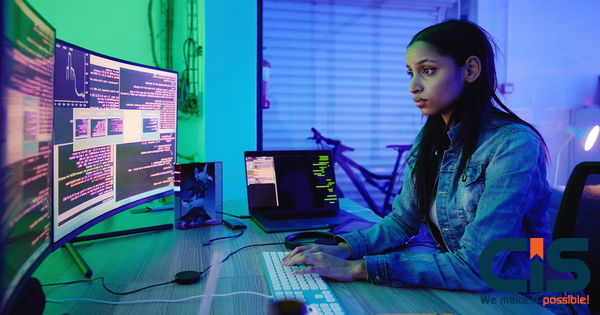
ML provides lots of advantages. Let's examine a few of the most beneficial. The advantages of machine learning demonstrate how they might be useful to human data annotators.
Automation
Automation includes a significant amount of machine learning. Time is being saved, and workers' workloads are being lessened, thanks to machine learning. Today, automation is prevalent everywhere. This difficult algorithm takes care of everything. Automation is more dependable, effective, efficient, and quick. Modern computers are built with machine learning as a significant component. This computer can handle advanced algorithms and machine learning models. Industry automation is expanding quickly, which calls for more innovation and research.
A type of machine learning is the process of using computers to comprehend and analyze data. Machine learning uses computers to examine data and generate predictions without human assistance. The process of choosing the software or algorithm that will result in the best results is known as machine learning. Voice recognition technology is used by Google Home to identify users' voices and choose the best response. The malware is found and eliminated by the antivirus application.
Opportunities For Improvement
Machine learning is a dynamic field. There is much to be learned about machine learning. In the upcoming years, this technology might represent the biggest technical advance. Many breakthroughs and fields of study are built around this technology.
It Has Many Applications
Machine learning can be helpful in many facets of real life, including medicine, education, engineering, and other professions. Simple applications of machine learning are possible, as well as its usage as a well-organized tool for predictive analytics. It also provides specialized services in addition to healthcare.
Ability To Advance
People can pick up knowledge and skills through experience. Machine learning is a tool that improves productivity and accuracy. Better decisions were made as a result. The weather report makes this clear. If the machine has more data, it will forecast more accurately.
Improve Your Online Shopping And Educational Opportunities
Education will include a substantial amount of machine learning. The quality of instruction will increase, and education will become more innovative thanks to machine learning. The students are more attentive. Your search results are analyzed by machine learning, which then produces recommendations. Based on surfing and search history, it creates customized notifications and ads.
Limitations On Machine Learning
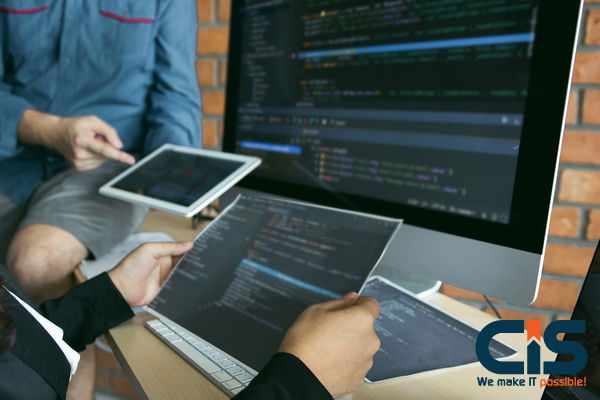
For autonomous driving, machine learning algorithms can be an effective tool. There might be some restrictions, though. These potential weaknesses could keep a machine-learning system from being flawless. Machine learning techniques offer several advantages. Understanding its limitations is crucial, though. If you don't study the advantages of machine learning, you won't know the risks. Let's examine machine learning's drawbacks.
Data Acquisition
Finding useful data is the foundation upon which machine learning is based. The outcome won't be correct if the data source is unreliable. High-quality data is crucial. If a user or institution asks for the information, wait. The output will therefore be delivered later as a result. How well the data is collected has an impact on machine learning.
The more data a system can process, the more exact and effective it will become. As a result, forecasting and decision-making become more challenging. Yet occasionally, it might not be achievable. The accuracy and objectivity of the data are crucial. It can be challenging to meet data requirements at times.
Time And Resources
Machines can detect discrepancies in data because they process vast amounts of data. Machines need time to adjust to their surroundings and absorb new information. Trials are performed to validate the machine's precision and dependability. It costs a lot of money to construct infrastructure of superior quality. Trial runs could be as expensive as the money and effort they need. The learning process can occasionally take a very long period. This is why it sometimes takes a long time because efficiency and effectiveness can only be acquired via experience. You might require more computers to expand your resources.
High Error Rate Probabilities
It was a terrible error to make at first. It can wreak havoc if it is not fixed. It's critical to address bias and error independently. They do not need to be connected. The use of machine learning requires both data and algorithms. Both variables cause all errors. Each inaccuracy in any variable has the potential to alter the result drastically.
Because machine learning is significantly more precise than conventional methods, it is also incredibly open to intrusion. The machine can be given the wrong set of instructions. There could be several errors if the same training progress program is used to create more than one forecast or prediction. Finding the error's cause could take some time because it might not be clear.
Data Interpretation That Is Incorrect
We are aware that manipulations and biases can result in long-term mistakes. Also, there is always the chance of misunderstanding. Even the most fundamental or unaltered facts can occasionally be misinterpreted by computers. It can be the case because the data doesn't adhere to specific standards.
Want More Information About Our Services? Talk to Our Consultants!
Conclusion
Machine learning algorithms have made it possible to create self-driving cars. These algorithms enable vehicles to collect information from cameras and other sensors, evaluate it, and decide what to do. With machine learning, both cars and people can carry out these jobs. A machine learning solution is a comprehensive combination of tools, software, and intellectual property for creating AI on a wide range of hardware. Future machine learning technologies will likely include driverless vehicles.
Autonomous vehicles use machine learning to gather data, analyze it, and draw conclusions about the environment. The detection of pedestrians and other objects on the road and the behavior of both are predicted using machine learning algorithms. When to brake, turn, accelerate, and how to steer the car generally can all be determined using these data.