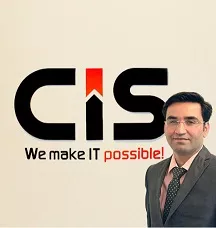
Contact us anytime to know more - Abhishek P., Founder & CFO CISIN
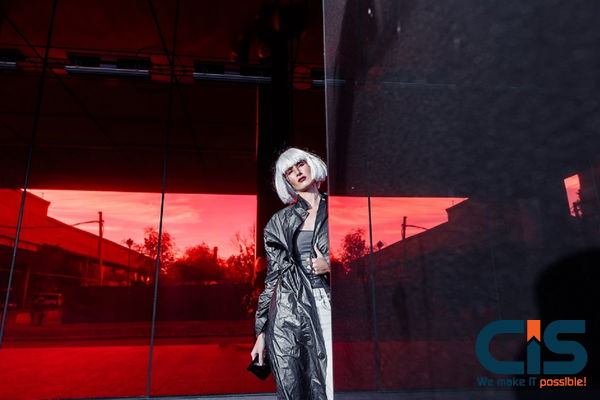
Retail industry digitalization has heralded an exciting era where data-driven technologies like machine learning are revolutionizing customer shopping experiences. E-commerce platforms now use machine learning algorithms to offer highly tailored interactions tailored to each person's preferences and interests, from product recommendations to customer support chatbots.
Understanding the Power of Personalization
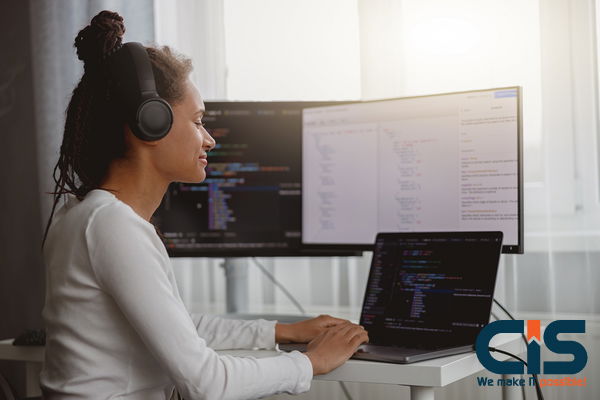
Personalization is at the center of modern e-commerce. Customers expect customized experiences that save them time, anticipate their needs and offer relevant product selections. Machine learning drives this revolution of personalization, its function being:
Recommendation Systems
Recommender algorithms form the cornerstone of e-commerce personalization. Utilizing large volumes of client information like browsing history, purchase trends and preferences as input into machine learning algorithms in order to provide personalized product suggestions, these systems can be divided further into the following groups.
Collaborative Filtering
Collaborative filtering provides products based on your similarity to others who share similar preferences and behaviors by analyzing user trends and patterns to suggest products people with shared interests have shown an affinity towards. It's an effective strategy for discovering new items while increasing cross-selling/upselling prospects.
Content-Based Filtering
Product suggestions are generated via content-based filtering by looking at the traits and attributes of goods that customers have either bought or used in the past. For example, if a user indicates interest in smartphones, the algorithm can recommend other devices with similar specifications. Content-based filtering does wonders for gaining a more comprehensive picture of user preferences.
Hybrid Models
Numerous recommendation systems employ a hybrid strategy that blends content-based and collaborative filtering. The advantages of both approaches are used in this hybridization to produce recommendations that are more varied and accurate. Hybrid models frequently outperform single-method recommendation systems.
Dynamic Pricing
Machine learning-driven dynamic pricing is a real-time method that modifies product prices according to multiple criteria. These elements may consist of:
Demand and Supply
The best pricing for a product can be found by using machine learning algorithms to examine supply and demand data in the market. In situations where there is a surplus of demand relative to supply, it may be profitable to raise prices.
Competitive Pricing
Retailers are able to keep an eye on what their rivals are charging and modify their own rates accordingly. This guarantees that their prices will always be reasonable in the marketplace.
Customer Behavior
Additionally, dynamic pricing can accommodate the unique behaviors of each consumer. A consumer who often purchases high-end equipment, for example, might be shown different pricing possibilities than someone who is shopping for the first time.
Personalized Marketing
Personalized marketing extends beyond suggested products. It includes a variety of approaches and techniques that use machine learning to give clients individualized experiences:
Email Personalization
Through the analysis of consumer data, machine learning algorithms create highly customized marketing emails. These emails may contain content that corresponds with the recipient's interests, product recommendations, and special offers.
Behavioral Targeting
Machine learning can be used to target customers with relevant adverts and content by monitoring user behavior on an e-commerce platform. For instance, a consumer who often browses the sports section may see advertisements for clothing and equipment for sports.
Customer Segmentation
Customers can be divided into discrete categories using machine learning on the basis of their behavior, demographics, and preferences. Retailers are able to develop marketing efforts that appeal to each category because of this segmentation.
Chatbots and Customer Service
Chatbots and AI-driven virtual assistants are revolutionizing e-commerce customer service:
24/7 Availability
Customers can get immediate service from chatbots day or night. Being available 24/7 improves the client experience and guarantees that questions are answered right away.
Natural Language Processing (NLP)
Chatbots can comprehend and reply to natural language inquiries thanks to NLP technology. Through chat, users may get product recommendations, ask questions, and even get help resolving problems without speaking to a live representative.
Order Tracking and Returns
Chatbots can help clients with order tracking and return processing. They make it easier for customers to return or exchange items, give shipment updates, and walk them through the process.
Personalized Assistance
Through the analysis of client data, chatbots may now offer individualized support thanks to machine learning. Based on a user's browsing and purchasing history, they can provide product recommendations, provide information, and offer discounts.
Also Read: Is Machine Learning Revolutionizing IT? Costing Billions in Industry Impact!
The Benefits of Machine Learning in E-commerce
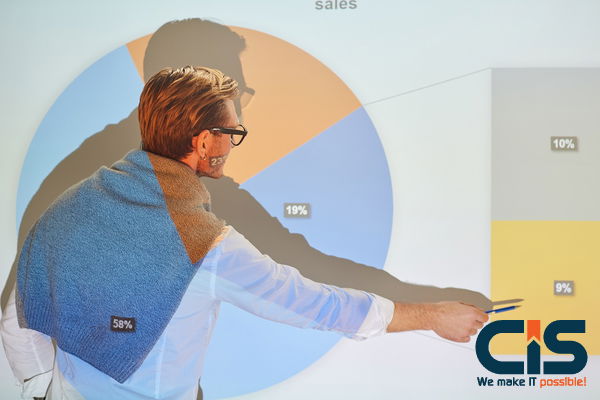
There are many benefits to e-commerce businesses and consumers alike when machine learning is integrated:
Improved Customer Engagement
Customization fosters a closer relationship between online retailers and their clientele. Users are more inclined to interact, spend more time on the platform, and make purchases when they believe that a website is aware of their preferences.
Increased Sales
Systems that provide recommendations greatly increase sales. A McKinsey analysis claims that product suggestions can account for up to 35% of online sales. Customers are more likely to convert when they are shown products in which they truly have an interest.
Enhanced Customer Loyalty
Personalized interactions increase client loyalty. Customers are more inclined to come back and make more purchases when they feel appreciated and understood. One-time transactions are not nearly as valuable as this ongoing relationship.
Efficient Inventory Management
Retailers may maximize their inventory management with the use of machine learning-powered demand forecasts and dynamic pricing. This lowers the problems of overstocking and understocking, which saves money and improves customer satisfaction.
Time Savings
Customers save time by using personalized experiences instead of sifting through large product catalogs. Rather, the selections that match their tastes are shown to them, making the buying experience more efficient.
Challenges and Ethical Considerations
Even though machine learning has many advantages for e-commerce, it's important to recognize the difficulties and moral issues raised by this technology:
Data Privacy
User data is necessary for personalization. Therefore, it is important to handle this data carefully. Retailers who want to secure consumer information must prioritize data privacy and abide by laws like GDPR.
Bias in Algorithms
If machine learning algorithms are not properly taught and controlled, they may unintentionally reinforce bias. Prejudiced pricing or suggestions might cause discrimination and harm a company's image.
User Consent
Before gathering and utilizing user data for personalization, retailers are required to acquire explicit and informed consent from consumers. To gain the trust of customers, data utilization must be transparent.
Algorithm Transparency
Consumers may want to know how suggestions are made or why particular rates are displayed. Maintaining algorithm openness promotes confidence and always fears.
Future Trends In Machine Learning And E-commerce
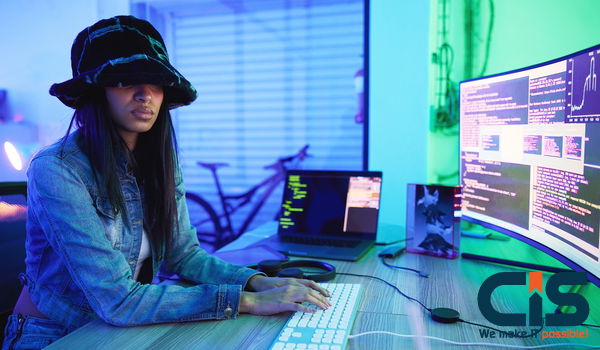
The relationship between e-commerce machine learning is still developing, and the following developments are expected to emerge:
Visual Search
Instead of using text to search for products, customers may now utilize images with visual search. As this technology advances, consumers will find it easier to locate products that resemble what they see in real life.
Augmented Reality (AR) Shopping
Customers will be able to virtually try on clothes, view furniture in their houses, and see how makeup looks on their skin thanks to augmented reality. By emulating the tactile sensation of physical stores, augmented reality (AR) improves the online buying experience.
Voice Commerce
The usage of voice assistants for shopping, such as Google Assistant and Alexa from Amazon, is growing. Voice commerce will proliferate as NLP and voice recognition technology advance.
Sustainability
Additionally, machine learning can support e-commerce sustainability initiatives. Sustainable products can be promoted to environmentally conscious consumers through individualized suggestions and algorithmic supply chain optimization.
Want More Information About Our Services? Talk to Our Consultants!
Conclusion
E-commerce is changing, and machine learning is one of the key factors. It gives retailers the ability to offer incredibly entertaining, effective, and individualized shopping experiences. It does, however, come with obligations for ethical use, bias prevention, and data privacy. In order to secure a better, more customized future for e-commerce, merchants must find a balance between innovation and responsibility as machine learning continues to progress. Contact us as we are the best Ecommerce Application Development Company.