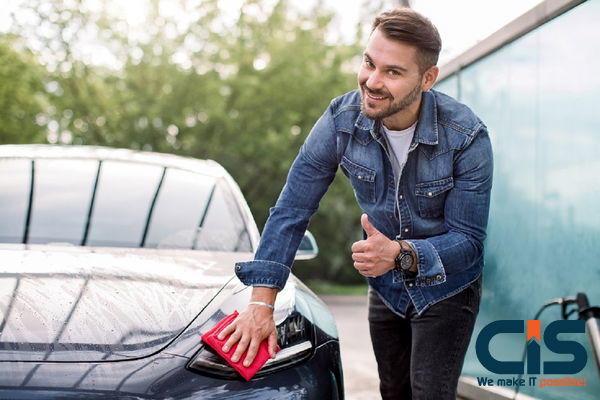
These three terms are often used interchangeably but do not refer to the exact same thing. Let's learn the difference between artificial intelligence, deep learning, and machine learning. You can see from the image above that Artificial Intelligence (AI) is a branch of computer science that helps us create intelligent, smart machines. ML is a branch of AI that helps machines to learn and create AI-driven apps. Deep learning, on the other hand, is a sub-branch within ML. It helps train ML models using complex algorithms with a large amount of data.
The three most debated commercial technologies today are artificial intelligence (AI), machine learning (ML), as well as deep learning. Companies use these innovations to create intelligent machines and apps. These terms dominate business discussions around the globe, but many people still have trouble distinguishing between them. This blog will give you a better understanding of AI and machine learning. Let's take a look at the opinions of tech influencers and industry figures before we get into the details. AI does not have to be bad to destroy humanity. If AI has a purpose and humanity is just in its way, then it will destroy mankind without thinking twice, with no hard feelings. Expert, Technology Entrepreneur, and Investor for artificial intelligence services.
Artificial Intelligence (AI), deep learning, machine learning, whatever you do, if you don't understand it, learn it. You'll be a dinosaur in 3 years if you don't. Expert: In deep learning, we are using algorithms that were developed in the 1980s and 1990s. Expert: People were optimistic, but they turned out to be a disappointment. These three terms are often used interchangeably but do not refer to the exact same thing. Artificial Intelligence refers to the idea of intelligent machines that are smart. Machine Learning is an artificial intelligence subset that allows you to build AI-driven apps. Deep learning is an advanced subset of machine learning that relies on large volumes of data to create a model. Let's now explore each of these technologies.
What Is Artificial Intelligence (AI)?
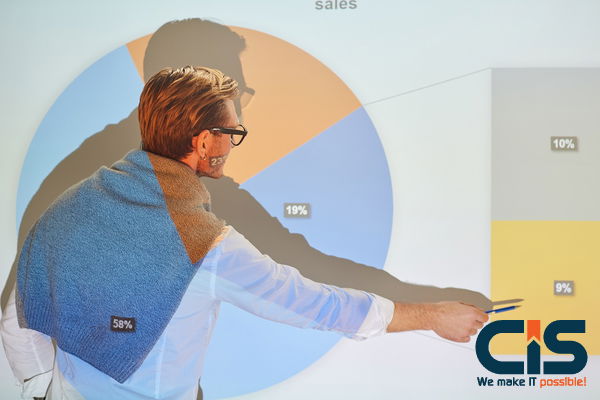
Artificial intelligence (AI) is a process that imparts data, information, and human intelligence to computers. Artificial Intelligence's main goal is to create machines that are self-sufficient and can act like humans. These machines can learn and solve problems to mimic human behavior. AI systems are designed to simulate intelligence in order to solve complex problems.
Amazon Echo is a good example of a product that uses AI. Amazon Echo is an intelligent speaker that utilizes Alexa, Amazon's AI virtual assistant technology. Amazon Alexa can be used to interact with voice, play music, set alarms, play audiobooks and provide real-time news, sports, traffic, and weather reports.
In the example below, you can see that the person is asking for the current Chicago temperature. First, the voice of the person is converted into machine-readable form. The data in the formatted form is fed to Amazon Alexa for analysis and processing. Alexa then returns the voice output desired via Amazon Echo.
Artificial Intelligence Types
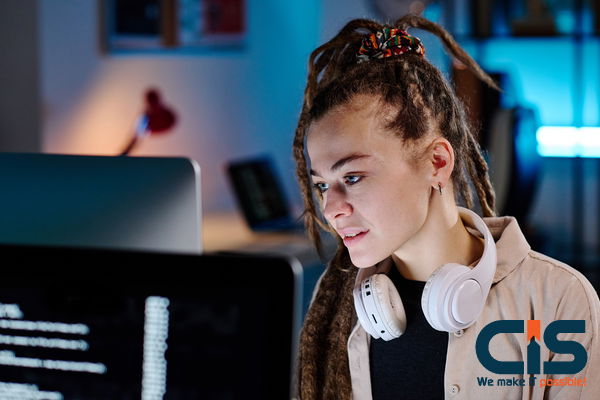
Reactive Machines are systems that only react. These systems do not have memories and don't draw on past experience to make new decisions.
Limited Memory: These systems refer to the past and add information over time. Referenced information has a short lifespan.
Theory of Mind: This is a system that can understand emotions in humans and their impact on decision-making. They are taught to adapt their behavior accordingly.
Self-Awareness: These systems were designed to be self-aware. They can predict the feelings of others and understand their internal state.
Artificial Intelligence Applications
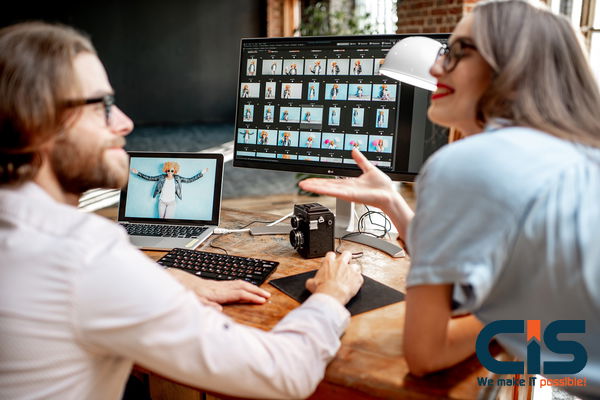
- Google Translate and other machine translation tools are available.
- Google Waymo and other self-driving vehicles.
- Sophia and Aibo are AI robots.
- Apple's Siri and Google's OK Google are both speech recognition applications. Translations of Languages.
- AI in the Healthcare industry.
- Speech recognition, facial recognition, text, and image recognition.
- AI in Astronomy.
- AI in gaming.
- AI in Finance.
- AI in data security.
- AI in Social Media.
- Artificial Intelligence in Travel and Transport.
- AI in the Automotive Industry.
- Artificial Intelligence in Robots.
- AI in Entertainment and agriculture, Ecommerce, education, etc.
We've taken a look at the basics of Artificial Intelligence and its AI Consultation. Let's now discuss the basics of Machine Learning for customer experiences. Let's now move on to the machine learning part of this article and learn how it works.
What Is Machine Learning?
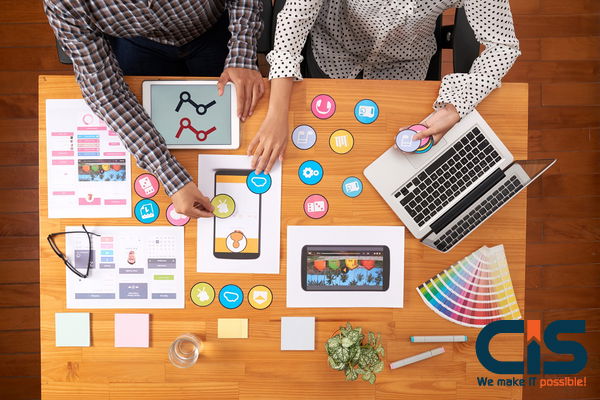
The discipline of machine learning in computer science uses algorithms and analytics from computers to create predictive models which can be used to solve business problems. According to experts, machine learning is built on algorithms that may acquire knowledge from data without the need for rules-based programmes. An expert book on machine learning says: "A computer program can be said to learn by experience E in relation to a class of tasks, T, and a performance measure, P, if it's a performance at tasks within T, as measured through P, improves over time." How does it work?
Machine Learning can be defined as a branch of Artificial Intelligence (AI) and Computer Science that uses algorithms to improve the performance of computers and machines by learning from past experiences. Machine learning is used to help machines and robots become intelligent.
How Does Machine Learning Work?
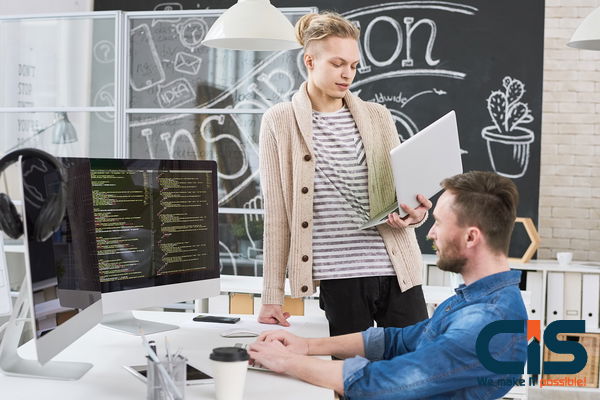
Machine learning uses data, both structured and unstructured, to learn and predict the future. The data is analyzed using several algorithms and techniques. Let's look at the different types of machine learning methods now that you know the basics.
Machine Learning is a set of algorithms and techniques which enable machines to learn and adapt from their past experiences and trends and then predict the outcome based on this data. Data preprocessing-processing allows machine learning to access large amounts of data. The data can be structured, semistructured, or unstructured. This data is then fed to machines and machine learning algorithms, which, based on past trends, predict the outputs. It's now time to learn about the different types of machine learning.
Machine Learning Involves A Number Of Steps
Machine learning involves seven easy steps:
- Data collection.
- Pre-processing-processing of data.
- Select model.
- Train model.
- Test model.
- Tune model.
- Prediction.
Now that we have covered the basics of machine learning and artificial intelligence, it is time to discuss deep learning.
Types Of Machine Learning
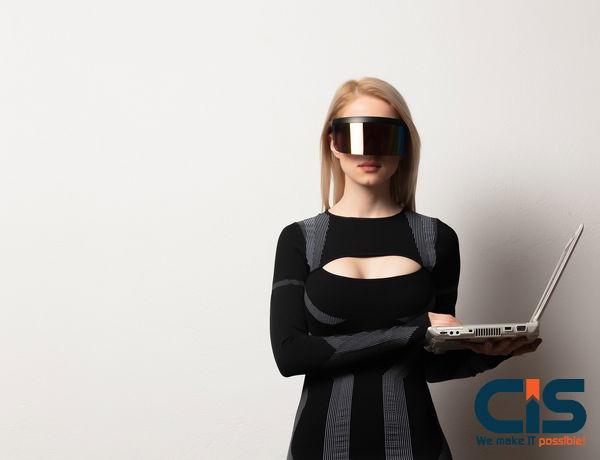
Three main categories of machine learning algorithms exist:
#1. Learning Supervised
The data in supervised learning is already labeled. This means that you already know what the target variable is. This method of learning allows systems to predict future outcomes using past data. For the model to be trained, it must have at least one input and one output variable. Examples of supervised learning are linear regression, logistic regression, support vector machine, Naive Bayes, and decision tree.
This type of machine learning method uses labeled data to train machines. Then, the machines can predict outputs based on this dataset. It requires supervision to train the models and predict outputs. Medical diagnosis, fraud detection, spam detection, speech recognition are all important applications of supervised learning.
- Classification.
- Regression.
Benefits Of Supervised Machine Learning
- Machine learning supervised helps predict outputs based on previous experience.
- This helps in providing a precise idea of the classes of objects.
Disadvantages Of Supervised Machine Learning
- This method does not solve complex problems.
- The output of this method is not guaranteed to be exact, as it includes both structured and unstructured data.
- To teach ML models, you need more computing time.
#2. Unsupervised Learning
Unsupervised algorithms use unlabeled information to find patterns on their own. The systems can identify hidden features using the data input. Patterns and similarities are more apparent once the data has been made more readable. Unsupervised learning is a method of training a model with unlabeled data. The data, in this case, consists of vehicles. The model's purpose is to classify different types of vehicles. Unsupervised learning can be done using k-means, hierarchical grouping, and anomaly detection.
Unsupervised machine learning is the opposite of supervised training. It does not require supervision and therefore does not use labeled datasets. In unsupervised machine learning, outputs are predicted without supervision. Unsupervised machine learning algorithms are designed to categorize or unsorted group data according to similarities, patterns, and differences. Unsupervised machine learning has many applications.
Unsupervised machine learning is divided into two forms.
- Clustering.
- Association.
Benefits Of Machine Learning Without Supervision
- This tool can be used for complex ML problems, as it uses unlabeled data.
- Comparatively, it is used for solving multiple tasks as opposed to supervised learning.
Disadvantages Of Unsupervised Machine Learning
- Unlabeled data can lead to inaccurate predictions.
- This is a fairly complex algorithm, as it works with datasets that are not labeled and do not map to output.
#3. Semi-Supervised Machine Learning
Semi-supervised learning is a hybrid of supervised or unsupervised machine learning techniques. It uses both unlabeled and labeled datasets to build models and predict outputs, but it mainly contains unlabeled datasets.
Benefits Of Semi-Supervised Machine Learning
- The algorithm is easy to understand.
- It is more efficient.
- This algorithm is used to overcome the shortcomings of both Supervised Learning and Unsupervised learning algorithms.
Disadvantages Of Semi-Supervised Machine Learning
- This does not include network-level data.
- This method is less accurate.
- The results of iterations may not be stable.
#4. Reinforcement Learning
Reinforcement learning aims to train an agent how to complete a task within an uncertain environment. The agent sends actions and receives feedback from the environment. The reward is a measure of how well the action has performed in achieving the goal. Deep Q-learning neural networks, and Q-learning are examples of reinforcement-learning algorithms.
Reinforcement learning can be defined as a feedback-based technique to improve models by learning from previous experience. This method involves an AI agent exploring its surroundings by hitting and experimenting with actions.
In addition, reinforcement learning algorithms do not use labeled information but instead learn from past experience. It can be used in a variety of real-world situations, including video games, resource allocation, robotics, and text mining.
The two types of reinforcement learning are:
- Learning by Positive Reinforcement.
- Learning by negative reinforcement.
Benefits Of Reinforcement Learning
- This technique is used for solving complex real-time situations where other techniques are ineffective.
- It is the most accurate because it learns like a human.
- This is important for long-term success.
The Disadvantages Of Reinforcement Learning
- Simple scenarios do not require a complex solution.
- This requires a large amount of data and computations.
Read More: How Artificial Intelligence Is Changing The Business World
Machine Learning Applications
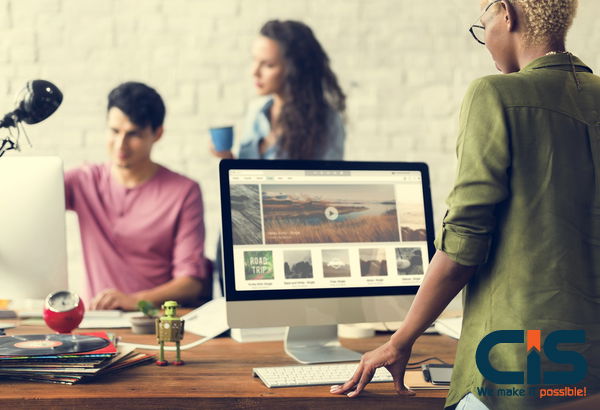
- Forecasting sales for different products.
- Fraud Analysis in Banking.
- Product Recommendations.
- Stock price prediction.
After exploring machine learning, we will now explore deep learning. What exactly is it, and how does it differ from AI?
Machine Learning Is Not New Technology
Machine learning can be thought of as a set of algorithms that analyzes data learned from it, and makes informed decisions on the basis of those insights. The use of machine learning can automate a wide range of tasks. This technology affects almost every industry, from IT security to forecasting the weather to stockbrokers searching for optimal trades. To achieve the desired results and functions, machine learning requires complex math and lots of coding. Machine learning incorporates classic algorithms for different types of tasks, such as clustering or regression. These algorithms must be trained on large data sets. The better your model becomes, the more data you give it.
Machine learning is an old field that incorporates algorithms and methods that have existed for decades, some even since the 1960s. The Naive Bayes Classifier and Support Vector Machines are two of the most common algorithms used for data classification. There are other algorithms for cluster analysis, such as K-Means or tree-based clustering. Machine learning methods like principal component analysis (PCA) and tSNE are used to reduce the dimension of data in order to gain greater insight into it.
A machine learning model's training component is the part of the model that optimizes along a particular dimension. Machine learning models aim to minimize the difference between the values they predict and the ground truth. We must define an error function, also called a loss function or objective function. This is because the model has a goal. This objective may be to classify the data into different categories.
What Is Deep Learning?
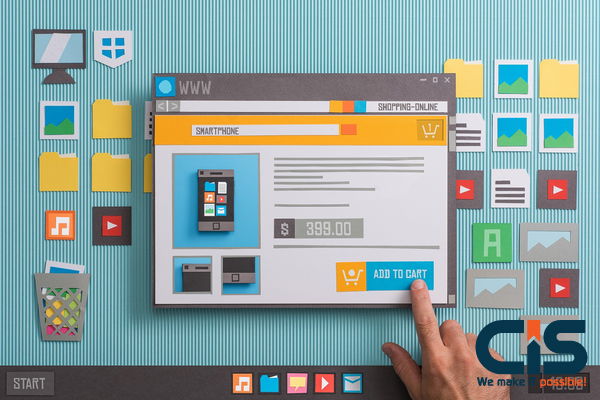
Deep learning is an area of machine learning which deals with algorithms that are inspired by the structure of the brain. Deep learning algorithms are able to handle a large amount of structured and unstructured information. Artificial neural networks are the core of deep learning, and they allow machines to make intelligent decisions. Machine learning and deep learning differ in the way that data is presented to machines. Machine learning algorithms require structured data, whereas deep neural networks use multiple layers.
Deep learning is the artificial neural network-based subset of machine intelligence and machine learning. The deep word in deep learning refers to how many layers are present in a network. Deep learning is an algorithmic set that's inspired by brain structure and function. It is able to predict accurate results by using a large amount of unstructured and structured data. Machine learning and deep-learning technologies differ primarily in the way they present data. Machine learning uses structured/unstructured data for learning, while deep learning uses neural networks for learning models.
If a machine-learning model predicts incorrect results, we must fix it manually. In deep learning, the problems are automatically fixed, so we don't need to do any explicit work. Self-driving vehicles are a great example of deep learning. Deep learning is useful for solving complex problems, such as image and voice recognition, product recommendation systems, natural language processing, etc.
A simple neural network is shown below: It has an input layer that accepts inputs taken from the data. The hidden layer can be used to identify hidden features in the data. The output layer provides the output. This is an example neural network using large unlabeled datasets of retinal images. This data is used to train the network model, which can then be used to determine whether a person suffers from diabetic retinopathy. Let's look at how deep learning works now that we know what it is.
How Does Deep Learning Work?
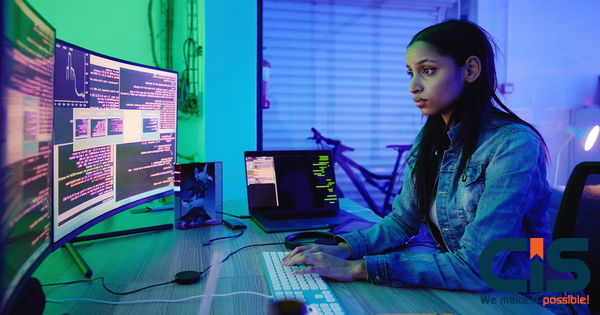
- Calculate the weighted amounts.
- The activation function receives the calculated weights as input.
- The activation function uses the "weighted input" to determine whether or not the neuron is fired.
- The output layer provides the predicted output.
- The output of the model is compared to the actual output. The model then uses backpropagation to improve performance after training the neural networks. The cost function reduces the error rate.
In the example below, neural networks and deep learning are used to recognize the number on the license plate. Many countries use this technique to identify speeding cars and vehicles that violate the rules.
Deep Neural Networks Types
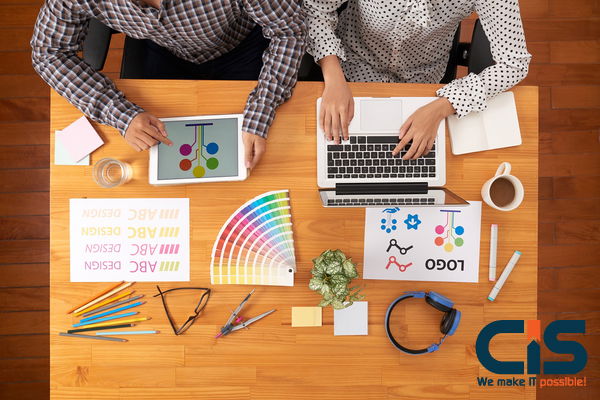
Convolutional Neural Network (CNN): CNN is the most common deep neural network used for image processing.
Recurrent Neural Networks (RNN): RNN builds a model using sequential information. This is often better for models which have to remember past data.
Generative Adversarial Networks (GAN): GANs are algorithmic architectures that use two neural networks in order to create new synthetic data instances that look like real data. A GAN trained with photographs can create new photos that appear at least superficially real to humans.
Deep Belief Network (DBN): DBN consists of multiple layers of latent variables called hidden units. The units and layers are not interconnected.
Deep Learning Applications
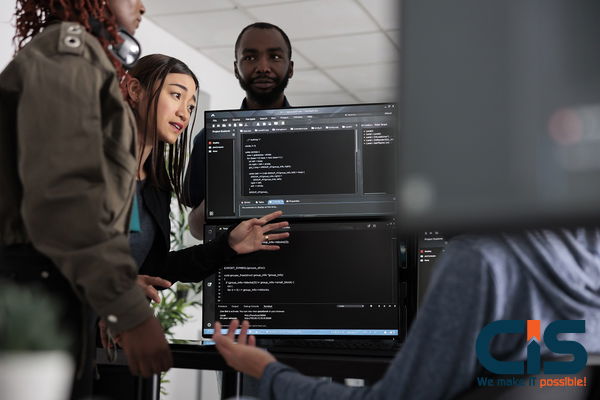
- Cancer tumor detection.
- Captionbot is a tool for captioning images.
- Music Generation.
- Image Coloring.
- Object detection.
- Self-driving cars.
- Fraud detection.
- Natural Language Processing.
- Virtual Personal Assistance.
- Text, speech, and image recognition.
- Healthcare, infrastructure, banking & finance, marketing.
- Enjoy the Entertainment.
- Education.
- Playing Automatic Games.
- Auto handwriting generation.
- Translation into automatic language.
- Pixel restoration, photo description & tagging.
- Predictions of demographic and electoral trends, etc.
Deep Learning Vs. Machine Learning, The Next Big Thing
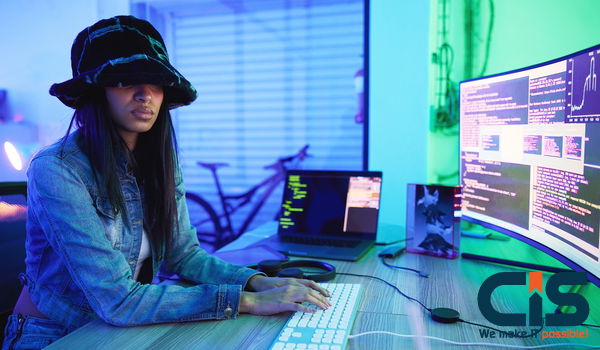
Deep learning is a relatively new subfield of artificial Intelligence based on artificial neural networks. We can call deep learning a subfield in machine learning since it also requires data to solve problems and learn. Machine learning vs deep learning are often used interchangeably. These systems are different. Deep-learning models can solve problems that machine-learning models cannot.
Deep learning is responsible for all recent advancements in intelligence. We wouldn't have chatbots, Alexa or Siri. Google Translate wouldn't be as advanced, and Netflix would not know what movies or TV shows to recommend. Artificial neural networks and deep learning are driving the new industrial revolution. Deep learning is the closest we've come to achieving true machine intelligence because it has two major advantages.
Why Is Deep Learning Better Than Machine Learning?
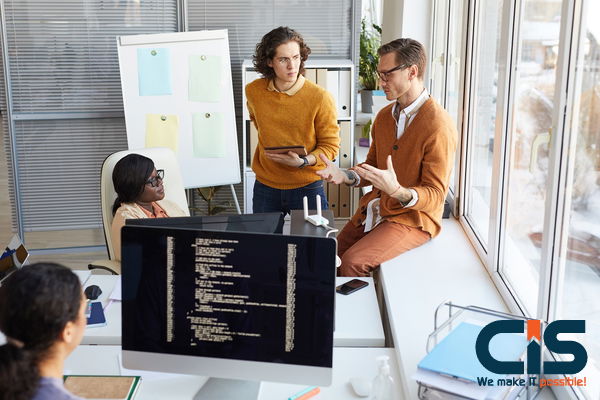
No Feature Removal
Deep learning has a number of advantages over machine learning. The most important is that it can extract features multiple times. Before deep learning became popular, we had traditional machine learning techniques (decision trees and SVM) as well as Naive Bayes classification, logistic regression, and Naive Bayes classifier. They are also known as flat algorithms. These algorithms are "flat," which means they cannot be directly applied to raw data, such as images, text, or.csv. We need to perform a step of preprocessing processing called feature extraction.
We provide an abstract representation of the raw data, which can be used by classic machine learning algorithms to accomplish a task. The classification of data into different categories or classes. The process of feature extraction can be quite complex and requires a thorough understanding of the domain. For optimal results, this step must be tested, adapted, and refined in several iterations. Deep learning models do not require feature extraction.
Artificial neural networks are the best deep learning models. They don't need feature extraction. Layers are able to learn implicit representations of raw data by themselves. A deep learning model creates an abstract and compressed representation of raw data using several layers of artificial neural networks. The result is then produced using a compressed version of the input data. The result could be the classification of input data into classes.
The neural network optimizes the feature extraction process during the training phase to get the best abstract representation possible of the input data. Deep learning models do not require much manual effort in order to optimize and perform feature extraction. In other words, feature extraction occurs automatically within the artificial neural network without any human input.
To use a machine-learning model to determine if a certain image is a picture of a vehicle or not, humans must first identify the unique characteristics of an automobile (shapes, sizes, windows, wheels, etc.). These features are extracted and given to the algorithm. The machine-learning algorithm would then classify the image. In machine learning, the programmer is required to intervene directly in the classification process. You can use neural networks for any other task. Let the model handle the data.
Deep Learning For Big Data
Deep learning is also powered by massive data sets. This is a major reason why it has become so popular. Deep learning will be able to benefit from the era of big data technology. Deep learning models increase in accuracy as the amount of data increases, while traditional machine learning models like SVM and Naive Bayes stop improving once they reach a saturation point. Data volume is a factor in the scaling of deep learning models. Andrew Ng is the co-founder and chief scientist at China's largest search engine Baidu. He is also the leader of the Google Brain Project.
Conclusion
Artificial intelligence, also known as machine learning and deep learning, is one of the five th-generation technologies that has changed the world. Artificial Intelligence Solutions allows us to create intelligent systems and give cognitive abilities to machines. Machine learning allows machines to learn from experience without human interaction and makes them capable of learning and predicting outcomes with given data. Deep learning, on the other hand, is the AI breakthrough that uses multiple layers of artificial neural networks to produce impressive results for various problems like image recognition and text identification. After reading this article, there should be no confusion between these terms. You should now be able to distinguish between the terms artificial intelligence (AI), deep learning (DL), and machine learning (ML) after reading this topic.