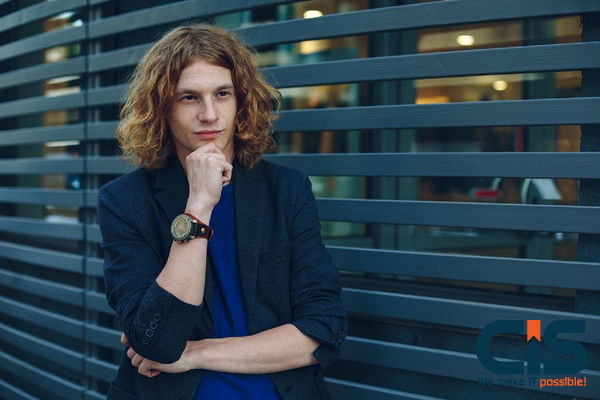
The COVID-19 epidemic has accelerated business digitization. More than two-thirds of top executives believe their organizations have advanced their implementation of digitization and automation since the crisis began. Many organizations (including banks, insurance companies, and other large corporations) deploy automation and artificial intelligence solutions to optimize end-to-end business processes, automating tactical activities and more complex prediction problems and decision-making.
By extracting new insights from complex data, intelligent automation solutions can help achieve efficiency and effectiveness advantages and support decision-making. However, as with other types of AI, they might raise business risks and lead to heightened regulatory scrutiny: these tools and technologies could eventually impair the delivery of vital business services to the surrounding ecosystem.
The dangers of AI come from a variety of places. Potential breaches of data-privacy rules in model development. A lack of openness about how these systems work, with the danger that defects in model design or training-data selection, could lead to errors, unfairness, or bias; and new cybersecurity concerns such as model extraction or deliberate "data poisoning" by hostile actors are among the most serious.
The majority of businesses lack the necessary structures and tools to adequately manage the risks and benefits of intelligent automation. Different components of system development and operation (such as implementation and system management, risk and resilience management, and business process optimization) are frequently managed in a fragmented manner by separate roles. Furthermore, most firms lack the necessary frameworks, processes, and technology to effectively assess the risks and benefits of AI and automation. As a result, it's becoming increasingly important for businesses to incorporate automation-specific issues into their larger AI and digital risk-management plans.
Towards a deeper grasp of the dangers of automation
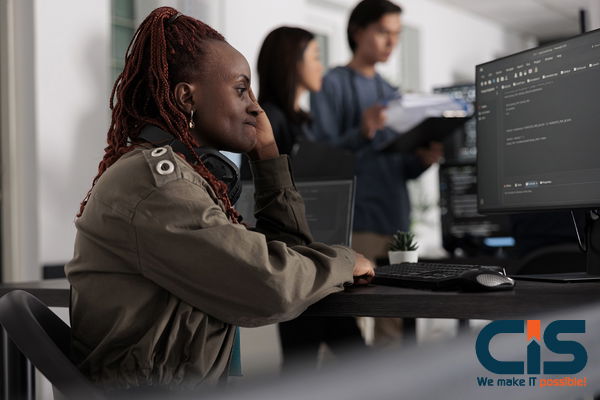
To drive strategic decisions across the company, institutions can build a holistic perspective of the benefits and hazards of intelligent automation, including where these tools touch essential operations and where vulnerabilities may exist. They must also comprehend how to simplify and automate processes, mitigate risk, and systematically strengthen institutional resilience. Five necessary tactical actions in the automation and AI life cycle can be considered for this aim.
Create a specialized risk-return center of excellence for intelligent automation
The first necessity is to centralize all essential information and decision-making authority. One way to do this is to create a dedicated center of excellence (CoE) for intelligent-automation risk-return management. This core position would ensure that AI and automation solutions improve performance and value across business processes while not exceeding the organization's risk tolerance.
The CoE will require a comprehensive view of three things to achieve this goal. First, it must comprehend the enterprise taxonomy of business services and processes across the organization; they will provide a single reference point for discussing and navigating the process landscape. Second, it must understand where AI and automation are now used in those processes and the opportunity to improve efficiency or business performance over time by deploying more use cases. Finally, it needs a clear picture of the flaws discovered in current and proposed AI and automation solutions.
This solution contrasts the risks associated with AI's complicated procedures, which are typically meant to deal with uncertainty and pose a broader variety of possible difficulties, such as explainability and bias. Because basic automation solutions are often based on deterministic methodologies, the dangers are more likely to revolve around implementation mistakes or incorrect configuration. As a result, automation risks are frequently thought of as a subset of AI concerns.
The intelligent-automation risk-return CoE can employ in-house expertise and skills from other company parts to create this image. In banking, for example, the model-risk-management team would generally provide insight on AI flaws like bias and fairness in decision-making or the "explainability" of the underlying models. The Center of Excellence would play a key role in coordinating actions already being carried out by the model-risk-management function and the legal, compliance, and IT teams and collecting important data through rigorous reporting processes.
Identify and prioritize business simplification and end-to-end optimization opportunities.
Businesses already use many AI and automation solutions, and many more are being considered or developed. A major responsibility for the intelligent-automation risk-return CoE is establishing and maintaining a complete inventory of these applications.
Banks' model-risk-management teams often keep a model inventory with a view on AI solutions utilized across the firm so that the process might build on existing intelligence. This inventory would include details on the methodology and techniques used in each case, the implementation platform, the business processes in question, the system's owner, and any technology vendors involved. It would also include information on all of the approach's possible flaws. For each AI and automation application they adopt, business units would be expected to report this information to the CoE, with frequent reviews and audits to guarantee that information is always up to current.
The AI and automation inventory support both sides of the risk-return equation. The inventory helps management uncover synergies and possibilities to replicate or expand established approaches into other areas by providing a comprehensive view of how and where AI is already being used in the enterprise. At the same time, the inventory allows the CoE to identify risks, determine who is responsible for them, and manage any necessary mitigation measures.
The risk-return CoE can make strategic decisions about where to deploy, enhance, retire, or consolidate solutions and technologies based on this cross-organizational view of intelligent automation. In this case, the company can create a framework for prioritizing procedures based on their organizational value and business criticality. When a global security and cash logistics company with operations in multiple geographies conducted an enterprise-level identification and prioritization effort, for example, leaders were able to identify more than 40 strategic existing AI solutions that other businesses could use.
Create a solid framework for integrating technology solutions throughout the entire value chain
The intelligent automation risk-return CoE can design a defined approach and set of principles for developing and implementing AI and automation technologies in collaboration with key analytics and technology teams. The CoE can then collaborate with business units to ensure that these principles are followed consistently throughout the company; This enhances transparency around AI and automation projects across the enterprise and highlights major risks and constraints early in the development cycle.
To create a uniform transformation methodology that could be deployed across every area and line of business, one multinational bank evaluated and reworked its automation and AI development playbook.
The playbook's guidelines emphasized the importance of keeping track of project risks-including the need for precise risk assessments and metrics-as well as the transformation's impact, returns, and other advantages. On the other hand, a strict prioritizing approach focused on the most crucial applications, processes, and services. The bank was able to build comprehensive mitigation controls after using this methodology to identify for the first time the limitations of the AI and analytics technologies employed across the enterprise.
Examine the dangers of AI and automation
Every AI system or automation tool should undergo a thorough testing procedure to discover any potential inaccuracy or bias in the input, processing components, or output. The evaluation would typically cover data quality (such as the risk of error or discrimination in the data sample), the correctness of implementation (including the deployment of the correct formulas and rules), system performance, sensitivity, and robustness (focusing on output accuracy), explain ability of the model (given its use cases and complexity), and any bias and unfairness in the results it generates, at a bare minimum. Some sorts of tests, such as those for security flaws or data poisoning vulnerability, may necessitate the utilization of specialized in-house or third-party teams.
Functions controlling analytics risk assessment (such as model-risk management) and the analyses they undertake can support decision-making on AI applications in industries such as banking. Chatbots, optical character recognition (OCR) technology, robotic process automation, and speech-to-text approaches were among the AI and automation technologies created by one large US bank as part of its digitalization journey. As part of the bank's routine oversight standards, an independent review and challenge process highlighted several possibilities to reduce risks and boost the efficiency advantages delivered by these systems.
In the case of OCR procedures, for example, instability difficulties developed. Characters in customer-account identifiers were frequently misinterpreted by the approach employed, such as the letter "O" being misinterpreted as the number "0," or the number "5" being twisted as an "S". These mistakes would cause consumer information to be erroneously mapped in relevant systems, necessitating several human interventions to manually repair the errors. Controls were put in place to guarantee that high-risk situations were recognized and processed in a controlled manner without harming customers.
Chatbots were scheduled for production in other places without a robust monitoring mechanism to guarantee that future changes (such as adding new languages or features, including access to sensitive banking information) were discovered and controlled before deployment. The bank can be safeguarded against potential financial and reputational problems by implementing such a plan. It also allowed the institution to track efficiency gains by determining how much human participation was required in basic client interactions.
Create a framework and infrastructure for tracking risks and returns
Finally, because decisions must be made continuously, businesses can establish systems to track the benefits and risks of AI and automation over time. A thorough monitoring system and infrastructure, with well-defined performance and risk indicators, must be created in this context. Such frameworks are already in use in the banking industry, though their focus has been on risk so far. Developing a viewpoint that considers both the dangers and rewards of AI and automation technologies is crucial in making strategic business decisions.
A dashboard with a consolidated picture of automation and AI across the organization and solution-specific data when needed would be excellent; This would eventually allow the intelligent-automation risk-return CoE to provide a real-time heat map and dashboard for action. Data science consulting services assist businesses in conducting experiments on their data to gain business insights.
This monitoring would be accompanied by a problem-solving approach for resolving faults and limitations found in AI tools and automation systems. It would also raise the stakes in circumstances where a system's efficiency degrades over time and needs to be improved or redeveloped.
Thanks to intelligent automation, many corporate procedures are becoming more efficient and productive. Companies are exposing themselves to ever-greater dangers as they strive to increase their usage of these technologies through broader applications and the adoption of more sophisticated methodologies. In the future years, balancing these hazards with the potential benefits of automation and AI will be a major task. Organizations that put the correct structures, systems, and governance mechanisms now will unlock considerable improvement potential in the future.
What is Intelligent Automation?
Intelligent automation (IA) is the application of automation technologies like artificial intelligence (AI), business process management (BPM), and robotic process automation (RPA) to streamline and scale decision-making across organizations. Numerous applications for intelligent automation simplify procedures, free up resources, and improve operational efficiency. An automotive factory, for example, might employ intelligent automation to speed up production or reduce the chance of human mistakes. At the same time, a pharmaceutical or life sciences company might use it to cut costs and obtain resource efficiencies when repetitive procedures exist. An insurance company can employ intelligent automation to calculate payments, create rate projections, and meet compliance requirements. Many AI mobile app development companies solve business problems with predictive analysis, robust applications, and deep learning.
There are 3 components of intelligent automation.
Three cognitive technologies make up intelligent automation. The combination of these elements into a solution drives business and technological transformation.
1. Artificial intelligence, or AI, is the most important component of intelligent automation. Businesses can establish a knowledge base and make predictions based on data by employing machine learning and complicated algorithms to evaluate structured and unstructured data; This is IA's decision-making engine.
2. Business process management (BPM), often known as business workflow automation, is the second component of intelligent automation. Corporate process management automates workflows to improve business processes' agility and consistency. Most industries utilize Automated business processing to streamline operations and enhance relationships and engagement.
3. Robotic process automation is the third component of IA (RPA). Robotic process automation (RPA) employs software robots, or bots, to perform back-office tasks such as data extraction and form filling. These bots complement AI because RPA can engage AI insights to tackle complex tasks and use cases.
The combination of these three components yields a game-changing solution that streamlines processes and streamlines workflows, eventually improving the customer experience.
Intelligent automation's advantages
As a result of massive amounts of data, the precision of calculations, analysis, and subsequent business application, intelligent automation platforms give numerous benefits across sectors. Some of the primary benefits are as follows:
Reduce expenses by increasing productivity and supplementing the workforce: Automating systems and processes and leveraging data and analysis to ensure correctness can help speed up production. IA also allows you to scale quickly without increasing risk, sacrificing quality, or putting a load on your current personnel. Business leaders reap higher production yields and greater ROI due to this capability.
Enhance quality by improving accuracy through consistent processes and approaches: The application of artificial intelligence to drive decision-making and bring a consistent approach to repetitive operations is the strength of intelligent automation.
Improve customer experience: Obtaining a higher-quality, more dependable product to market sooner (or instantly) or getting answers to customer questions faster (or immediately) gives the customer a richer, more positive experience, thus giving the company a competitive edge.
With confidence, deal with compliance and regulations: Many industries must follow strict regulatory guidelines. Intelligent automation's task automation characteristics can be used to demonstrate a more uniform approach to compliance.
IA aids in designing and developing end-to-end processes that enable current corporate operating models to be flexible, resilient, and adaptable. By freeing up employee time from activities that robots can handle, augmenting human experience with IA can unleash a fresh wave of innovation and motivate employees to boldly create new business value.
Effective IA necessitates collaboration between IT and business divisions to assess the efficacy of existing processes and then integrate systems to drive sustainable and scalable change into the process framework. Employees must be involved in the transformation journey for an IA program to succeed, so they can see firsthand the benefits of a new way of working and creating corporate value.
Intelligent Automation Applications
Intelligent automation automates previously manual procedures or relies on legacy systems, which were resource-intensive, expensive, and prone to human mistakes. IA is used in various sectors to improve efficiency in many organization sections.
Automotive
The benefits that intelligent automation can provide to manufacturers significantly impact the automotive industry. Manufacturers can better estimate and alter production in response to changes in supply and demand with IA. They can improve efficiency and reduce the risk of error in production, support, procurement, and other areas by streamlining operations. They can eliminate the need for manual labour and increase defect detection by using robots, resulting in a higher-quality product for customers at a reduced cost to the company. A Volkswagen engine manufacturing factory in Germany, for example, employs "collaborative robots" that work alongside the production team to complete a physically hard step in the engine assembly process. This aids in the prevention of harm, the speeding up of procedures, the assurance of accuracy, and the reduction of physical strain on staff.
Life Sciences
Drug manufacture is highly controlled and necessitates exact equipment calibration and product measurement. It also necessitates much data gathering, collation, processing, and analysis. A drug study cannot be effective without reliable research and outcomes, and a manual approach could result in calculation errors and need many resources and workforce to complete. The manufacturing of Covid-19 vaccines in record time, on the other hand, is an example of how intelligent automation may increase production speed and quality. It used to take 40-plus scientists with PhD degrees 25 years or more to identify a single commercial medicine before the adoption of artificial intelligence and the efficiencies that resulted.
Healthcare
The healthcare industry combines intelligent automation with natural language processing (NLP) to provide a uniform data collection, analysis, diagnosis, and treatment. Chatbots in remote healthcare sessions demand less human participation and, in many cases, result in faster diagnosis times.
Insurance
Using IA, the insurance business may practically remove the need for manual rate computations or payments and streamline paperwork such as claims and appraisals. Intelligent automation also makes it easier for insurance businesses to comply with all requirements. They can also calculate an individual's or an entity's risk and the appropriate insurance rate in this manner.
The Future of Intelligence Automation
Intelligent automation's value in today's environment, across industries, is undeniable. Businesses can save money and improve consistency in their workflows by automating repetitive tasks with IA. The COVID-19 epidemic has accelerated digital transformation initiatives, resulting in increased infrastructure expenditure to facilitate automation. Roles will continue to shift as the popularity of remote work grows. Individuals who were previously assigned to low-level duties will be reassigned to execute and scale these solutions, as well as other higher-level jobs. To keep employees motivated, middle managers will need to shift their focus to the more human aspects of their careers. Employees will need to adapt to their constantly changing work surroundings due to automation, which will expose skills shortages in the workforce.
Want to Knw More About Our Services? Talk to Our Consultant!
Middle management may also help employees stay resilient during these moments of change by supporting them in a way that reduces worry. Many Artificial Intelligence development companies offer a wide gamut of advanced AI development. Intelligence leaders recognize that AI can help them deal with the data flood, but they may be concerned about how AI will affect their work and workforce. According to surveys conducted in the private sector, there is a significant gap between the introduction of AI and the understanding of its impact. Even though nearly 20% of workers report a change in roles, tasks, or working methods resulting from AI implementation, nearly half of companies have not measured how AI implementation affects workers.