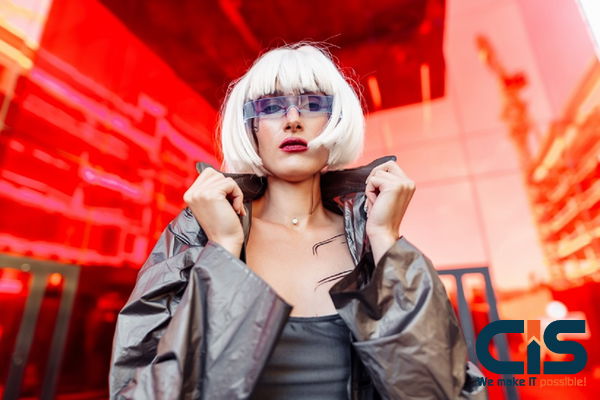
The term artificial intelligence (AI) refers to a very broad category of computer techniques. AI is frequently discussed in terms of machine learning and deep learning. Are they equivalent? No. Do they cross over? Indeed. While all machine learning (ML) techniques are AI techniques, not all AI techniques are ML techniques. ML is a subset of AI. In a similar vein, machine learning (ML) and deep learning (DL) are subfields of each other, not all ML and DL are equal to all machine learning.
How Does Artificial Intelligence Work?
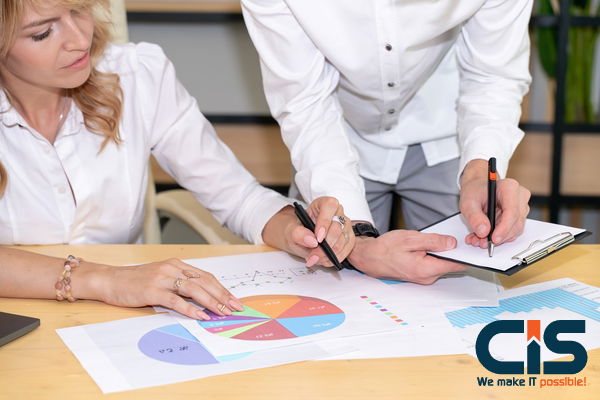
When it comes to some duties, AI helps people or, in certain situations, even takes their place. There are numerous instances in society, and the field of healthcare is one where there are many applications. Then, in this situation, how should we interpret AI? What does AI mean for healthcare?
Artificial Intelligence (AI) is the study of computers mimicking human behaviour, or at least trying to mimic human intelligence in activities. We may then define something like this in the context of healthcare: A subfield of computer science called artificial intelligence studies how to simulate intelligent human behavior in computers in order to address issues related to healthcare.
Applications can differ greatly from one another. Utilizing medical database AI trends, AI systems can efficiently explore patient databases, recognize patterns, predict disease trajectories, and autonomously perform surgical tasks like robots. However, these systems can also be used to support medical specialists' education by keeping them informed about the most recent advancements in the area of medicine, or they can be used to remind people who might otherwise forget about their appointments.
A Concrete AI Example: Rule-Based Engines
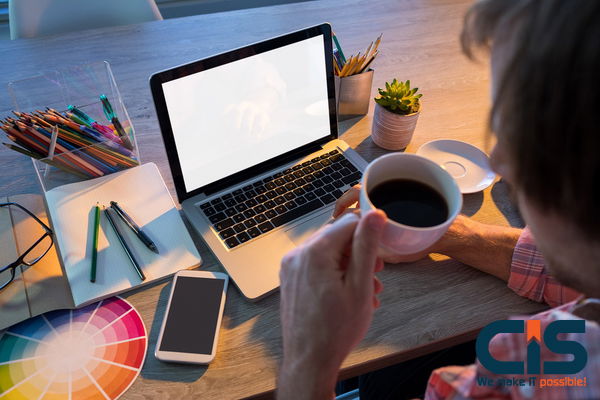
A rule-based engine is an example of an artificial intelligence-based technique that is not included in the ML and DL techniques. This method's idea is straightforward. All the algorithm does is provide a predetermined set of answers to a series of queries. For instance, the following process could be used to assess a patient's likelihood of having prostate cancer: Is the PSA value higher or lower than a particular amount? above.
Ask the second question in that scenario: did the MRI reveal any lesions, yes or no? Indeed. Then pose question three, and so forth. The algorithm is free to pose as many queries as it wants, with predetermined responses for each one. Upon completion of the entire query list, the computer will provide a conclusive response to the main query: does this patient have prostate cancer? It resembles an automated medical regimen rather closely.
What Is Machine Learning And How Does It Work?
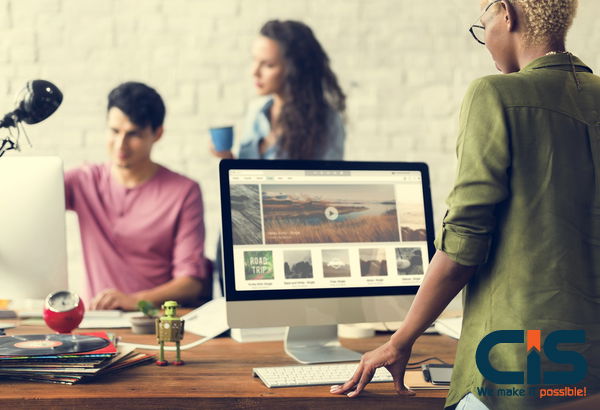
The topic of machine learning encompasses a vast array of techniques, some of which are related to deep learning, which we shall discuss in more detail in the following section. To gain an understanding of the various problems that machine learning can handle, let's first explore the several approaches that fall under the category of machine learning but do not fall within deep learning.
Differences Between Algorithms For Clustering, Regression And Classification
Algorithms for machine learning can help us in numerous ways. This section describes three categories of techniques: classification algorithms, regression algorithms, and clustering algorithms so that one can understand precisely how they accomplish this.
Classification methods assign classes to the inputs they use. To put it another way, a classification algorithm is one that determines a patient's status as having prostate cancer based on a variety of inputs, including age, estimated prostate volume, and other clinical characteristics, as well as PSA readings. Furthermore included in this category is an algorithm that offers a PI-RADS grading for a prostate MRI. A Support Vector Machine is a particular kind of such an approach.
Regression methods calculate a continuous value based on the input. In essence, it finds a function that, given an input value or values, outputs a value. An illustration would be a prostate cancer risk score determined by the PSA value (e.g., a PSA level of 6.2 ng/ml would indicate a 30% likelihood of prostate cancer). SVMs are frequently used for regression difficulties as well.
Clustering problems share similarities with classification problems. Additionally, a clustering algorithm arranges inputs into distinct categories. Nonetheless, classification algorithms are typically trained under supervision, that is, with access to ground truth. Conversely, clustering techniques are trained in an unsupervised manner, which means the algorithm itself has to figure out how to classify inputs into meaningful groups.
This approach is not frequently used in the healthcare industry as, in most cases, you already know exactly what you want your algorithm's output to be. The situation in which you wish the computer to classify things automatically is rare. Creating novel subtypes of prostate cancer through the use of an unsupervised algorithm trained on a genomic database is one example that comes to mind. Would you like to see a more thorough description of how a clustering method operates?
Read more: 4 Types of AI - How Much Will They Transform Our World?
Neural Networks - How Do These Fit In?
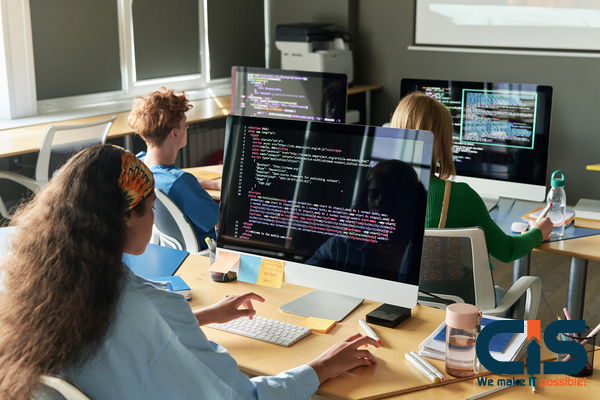
Artificial neural networks, or NNs as we will refer to them from now on, are a particular kind of technique with a wide range of applications. Not only are NNs excellent at solving classification issues, but they can also be used to calculate continuous values (i.e., solve regression problems) and carry out tasks that resemble clustering.
These networks are particularly effective at identifying patterns that are difficult for people to detect with the unaided eye or when there are no precise guidelines defining or measuring the patterns (such as minute variations in a lesion's size, form, or heterogeneity within a lesion). Therefore, you don't need to give the computer a lot of instructions-you can just give it a dataset and a ground truth and let it figure out the relationship between them on its own.
How are NNs operated upon? An image that resembles is frequently used to depict an NN. It is composed of two "layers," as you can see, an input layer and an output layer, both of which are composed of nodes. The input layer's nodes receive the input data, which in this example is an MRI of the prostate, and do calculations before sending the output layer the results. The solution to the issue that the neural network is meant to resolve is provided by the output layer. This would be the presence or absence of a lesion in the MRI prostate image in the case of the network.
How Does Deep Learning Work?
The next group of techniques we will talk about are all within the machine learning subject of deep learning. With the minor exception that deep neural networks have more than two layers between the input and output layers, their concept is quite similar to that of the neural networks we previously examined. These node-based hidden layers are also intended to identify particular aspects of the image. For instance, the first hidden layer usually examines higher-level elements like the image's boundaries, which can be useful in locating larger organs.
By combining this data, hidden layers below in the network and thus be able to concentrate on the finer points, including figuring out if suspicious tissue is truly BPH. Compared to a non-deep-learning NN, hidden layers can solve more complicated issues since they carry out more computations to evaluate all of these features. If you'd want to know more about how these computations are carried out inside a node and how neural networks function.
AI And The Prostate Cancer Pathway
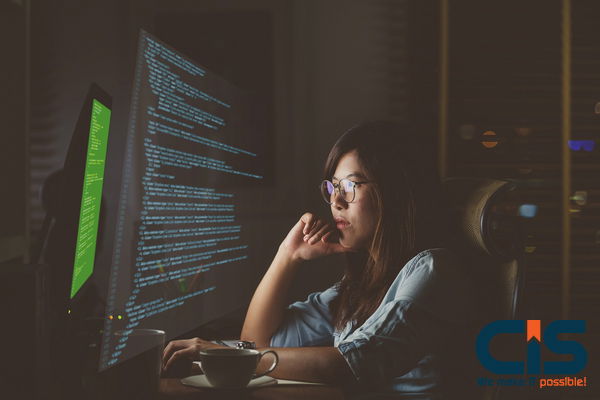
Prostate cancer affects millions of lives annually, making it a serious worldwide health concern. Advances in technology, especially Artificial Intelligence (AI), are bringing about a radical change in the detection and treatment of prostate cancer. We will examine how AI is changing the course of prostate cancer in this blog article, including everything from early detection to individualized prostate cancer AI treatment plans.
Early Detection With AI
Timely identification of prostate cancer is one of the most important management challenges. Conventional screening techniques, including the prostate-specific antigen (PSA) test, are not always accurate and frequently result in unneeded procedures. In this regard, artificial intelligence is changing the game.
Artificial intelligence algorithms examine extensive datasets, encompassing genetic data, imaging investigations, and medical records, to detect trends suggestive of prostate cancer. These algorithms continuously increase their accuracy by utilizing machine learning, which results in more dependable and early detection. This reduces the number of needless treatments for people who are at lesser risk in addition to helping to discover possible illnesses at an earlier, more treatable stage.
Advanced Imaging And Diagnostics
Artificial Intelligence is a key component in improving imaging methods for prostate cancer diagnosis. Artificial intelligence (AI) algorithms can help with the very accurate interpretation of Magnetic Resonance Imaging (MRI), which is a useful tool. Radiologists can diagnose patients more accurately by identifying tiny abnormalities that they might miss otherwise thanks to AI-based picture analysis.
Furthermore, AI aids in the creation of non-invasive diagnostic instruments like blood or urine testing, which can expedite the diagnosis procedure even further. By lessening the invasiveness of conventional biopsy techniques, these advancements provide a more patient-friendly method of verifying prostate cancer diagnoses.
Personalized Treatment Strategies
Developing an efficient treatment strategy is the next difficulty after a diagnosis has been made. Artificial Intelligence is highly proficient in examining a wide range of datasets, encompassing personal patient traits, genetic data, and therapeutic results. This abundance of data makes it possible to create individualized treatment plans that maximize results and reduce negative effects.
Oncologists can predict a patient's propensity to react to a certain treatment by using AI-driven predictive models. Medical professionals can use this information to make well-informed judgements about the best treatments for particular patients. Artificial intelligence (AI) is paving the way for more accurate and individualized prostate cancer treatments, including surgery, radiation therapy, and innovative targeted therapeutics.
Clinical Decision Support Systems
Clinical Decision Support Systems facilitate the integration of AI into clinical workflows (CDSS). By offering evidence-based suggestions for diagnosis, treatment planning, and monitoring, these tools support medical personnel. When it comes to prostate cancer, CDSS driven by AI can evaluate intricate datasets in real time and provide insights that help with decision-making throughout the patient's journey.
Enhancing Robotic Surgery
One of the mainstays of the treatment of prostate cancer is now robotic surgery. By offering real-time data analysis during surgeries, artificial intelligence (AI) improves the capabilities of robotic surgical systems. This helps surgeons perform more accurate motions and judgment calls, which eventually enhances the results of procedures performed with robotic assistance. The way that robotic surgery and artificial intelligence work together is a prime example of how technology is combining to change the way that prostate cancer treatments are provided.
Monitoring And Follow-Up
Monitoring after therapy is essential for evaluating the success of therapies and identifying any recurrence. Through the analysis of multiple parameters, such as biochemical indicators, imaging tests, and patient-reported data, AI-driven technologies enable continuous patient monitoring. Prompt intervention can be implemented upon early detection of indicators of illness recurrence, hence increasing overall survival rates.
Challenges And Ethical Considerations
Although the use of AI in the prostate cancer pathway has great potential, there are obstacles and moral issues to be considered. It is critical to protect patient privacy and security, correct biases in AI algorithms, and preserve decision-making openness. To fully utilize AI in prostate cancer treatment, a balance between technological innovation and ethical issues must be struck.
Future Of AI In Prostate Cancer Care
The use of AI in the treatment of prostate cancer is expected to grow in the future. AI algorithms will get better as long as machine learning, data analytics, and computing power continue to grow. The development of novel solutions will be fueled by the combined efforts of researchers, technology specialists, and healthcare professionals, which will ultimately improve outcomes for prostate cancer patients globally.
Conclusion
The prostate cancer route is being transformed by AI, from early detection to individualized treatment plans and continuous monitoring. The incorporation of AI-powered technology raises the standard of patient care overall, increases the precision of diagnostics, and offers insightful information for treatment choices.
Artificial intelligence (AI) has had a profoundly positive impact on the treatment of prostate cancer, despite ongoing obstacles and ethical issues. We are getting closer to a time when prostate cancer can be successfully treated with empathy, accuracy, and better patient outcomes as we adopt these technology developments.