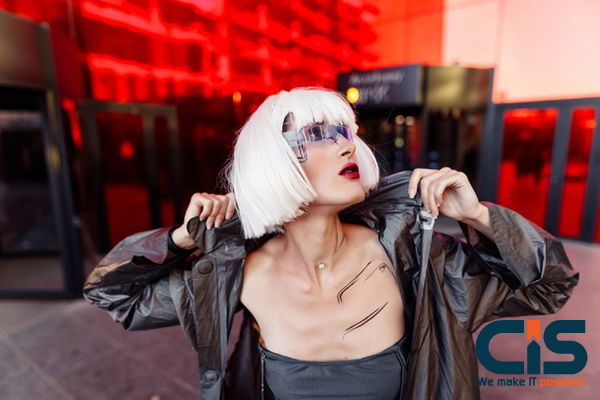
Three concepts discussed in connection with this futuristic future include big data, data science, IoT, or the Internet of Things IoT, and it's difficult to talk about either without the third. What's the difference between Data Science, the Internet of Things solutions, and Big Data? This isn't the most appropriate to ask because the three technologies are more similar than different and complementary. This is why we have to think about the connection between these three incredible technologies.
What is IoT?
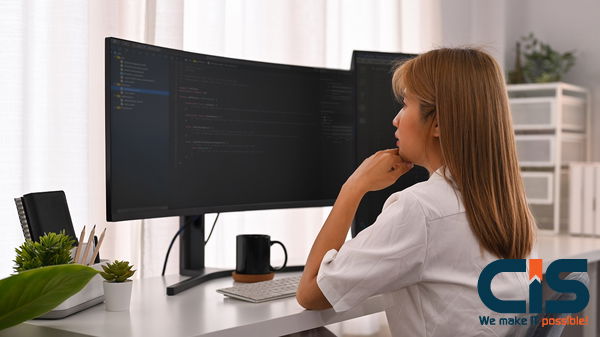
The term "generally speaking" refers to broadly speaking, the Internet of Things (IoT) covers any physical object, i.e., "things" connected to the internet as well as other devices.
Definitions of IoT are changing, and the term is increasingly used to refer to objects that interact with and "speak" to each other. We can improve the way we conduct our business.
IoT devices are characterized by their capacity to collect data about their surroundings, exchange this information with electronic gadgets, and finally, help the user gains knowledge, resolving an issue, or finishing a task.
Imagine a moment when you've gone to the bathroom in a hotel, and the light turned on all by itself to grasp the concept. Have you ever wondered how it was possible? There is likely a motion sensor in the area that detects movement. It automates and then connects with the light to switch it on.
It's not the only basic type that can be used as an IoT solution since IoT technology is being used to build larger ecosystems, such as smart homes and intelligent cities. Suppose you're reading your emails using a voice-controlled virtual assistant or tracking your steps and heart rate via a smartwatch, or managing your security system via your phone. In that case, You're getting the benefits of IoT applications regularly.
The Future of IoT Technology
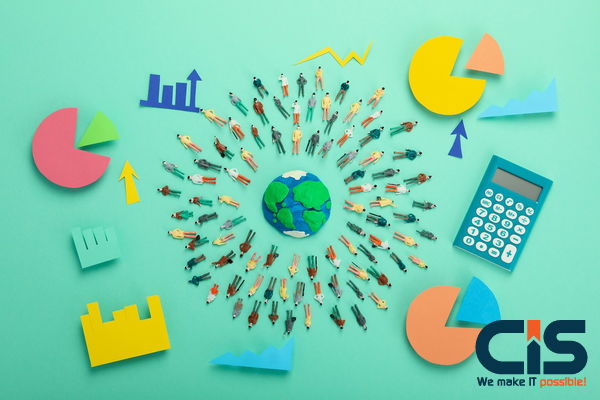
Based on Mordor Intelligence, the IoT technology market is predicted to grow to $1.39 trillion in 2026. This phenomenal growth could be due to several reasons:
-
The COVID-19 epidemic has accelerated the development of remote monitoring and smart home devices, and data analysis software.
-
Businesses are racing to come up with superior Artificial Intelligence solutions. This usually requires an array of sensors and edge computing within the realm of IoT.
-
IoT networks can complete certain tasks faster than central solutions.
A Network of Data
Because artificial intelligence technologies are heavily based on data, IoT sensors can be an incredible contribution to the machine learning data pipeline. Research and Markets report that AI is a key component of IoT technology and is expected to reach $14,799 million in 2026. The quality of data you collect is crucial to the success of machine learning methods. For example, data from IoT sensors that monitor factories can help machine learning algorithms to decide what equipment should be repaired in the future. This is referred to as predictive maintenance. It is among the most crucial applications to use AI in manufacturing.
5G: Advanced Mobile Networks
In most IoT technologies, the connectivity infrastructure has to be established before various sensors, edge devices, or other devices can be kept up to date. Mobile networks such as LTE could be an alternative in certain circumstances like outdoor settings. The downside is that 4G LTE can be limited due to bandwidth. 5G networks are quicker and can handle the data engineering required for IoT networks more effectively.
Edge Computing Grows
As IoT networks grow and grow, they'll open the way for a new unavoidable technology called edge computing. Edge computing could be a way to make self-driving vehicles more feasible while also solving some of the security, bandwidth, and cloud reliability problems. Even with these benefits, it's still a niche technology. However, that is likely to alter as it expands into part of the Internet of Things.
Edge computing is expected to become reliable and expandable when IoT security and processing capabilities increase. As smart urban infrastructure grows more widespread and reliable, the technology could impact consumer-facing applications.
Advancements in Healthcare
Particularly during the outbreak, the healthcare sector has been in leading the way in IoT advancements, using wearable technologies and telemedicine for diagnosing and managing patients. The connected devices in healthcare enable doctors to gather data on patients and offer medical care to more patients without the threat of infection from visits in person.
Wearable medical devices may also measure blood pressure and heart rate and provide insulin to people who have diabetes. Healthcare organizations can also incorporate IoT technology into defibrillators, wheelchairs, or oxygen tanks to give medical professionals information that will allow them to understand the patient's condition better or work independently to enhance the patient's life quality.
What is Data Science?
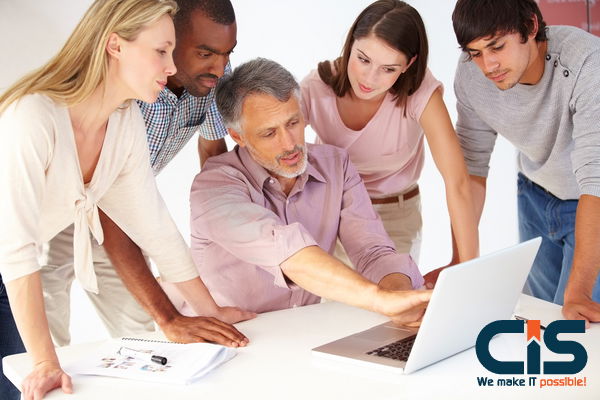
Data Science is a multidisciplinary discipline that blends Statistics programming, Computer Science, and domain knowledge to create applications to solve business-related problems. Data Science is a term that has been used to describe the field. Data Science has emerged with the growth in Big Data services, Computation Power, and Statistics. The main task of data scientists is to manifest solutions that impact society by offering practical solutions. As companies attempt to be data-driven, Data Science endeavors the most effective way to succeed in the highly competitive market.
Data science and business decision-making are now very likely to become a reality. It is the pillar of foundations for business in the modern age of information. Its capabilities go beyond inferring information. The findings gathered by curated analysis help improve effectiveness.
The decision-makers are occupied when determining the cross-over advantages of data science. These include but are not restricted to:
-
Fraud detection
-
Financial risk management
-
Cyberattack mitigation
-
Management and industrial mechanization
-
Systems for advanced warning for IT teams
Data is not only an asset but also a global currency. It is a tool to enhance the capabilities of a business, starting from supply chains for the organization's inventory, distribution networks, inventories, customer services, and a marketing channel. This method aims to decrease capital expenses by focusing on the outcomes of revenue.
Emerging Technologies in Data Science
Data science is a rapidly growing field that offers immense potential to grow more extensively. Recent developments and innovations make it distinct from other professions in greater than one. To fully grasp the potential this field offers, it is essential to understand the latest technologies in data science, which will shape the future and benefit everyone else.
Cloud Services
With the amount of data generated daily, it is an issue to find inexpensive storage and power solutions. Cloud computing and its services are a help. Cloud computing services are designed to store massive amounts of data at an affordable cost, allowing you to deal with the challenges in data storage in data science.
IoT
IoT is the term used to describe a system made up of different objects, such as individuals or devices with distinct IP addresses and access to the Internet. They are constructed to connect using the help of an internet connection. Smart meters and sensors, as well as other devices, are just a handful of advantages of the IoT as well as data science. We plan to develop this technology further to use it for predictive analytics. According to a report from Fortune Business Insights, the IoT devices market is anticipated to grow to $1.1 trillion by 2026.
Automated Machine Learning
Automated Machine Learning is also known as AutoML and has become an e-word. It is now being regarded as a tool to build more efficient models for machine learning. According to Gartner, over 40 percent of tasks within data science are expected to be automatized by 2020. Automating this data will help to fill in the shortage of quantity of talent, for example, data engineers, researchers, or data science researchers. Companies like Facebook have already implemented Automated Machine Learning.
AutoML (also known as Automated Machine Learning) aims to increase the accuracy of predictions and make the machine learning algorithms more refined. This allows one to concentrate more on finding solutions to difficult problems rather than creating workflows.
AR/VR Systems
AR refers to Augmented Reality, whereas VR is for Virtual Reality. The technology has already caught the attention of both individuals and businesses from all over the globe. Augmented real-time and virtual reality aim to improve the interactions between machines and humans. They can automate data analysis by using machine learning and Natural Language Processing (NLP) which assists data analysts and scientists in identifying patterns and creating shareable data. As per eMarketer, 42.9 million people use VR and 68.7 million use AR at least once each month.
Big Data
Big Data is the term used to describe a huge amount of data that could be structured or unstructured. These data sets are far too huge to be processed quickly by traditional methods, so advanced techniques must be used for similar purposes. Big Data boasts of technologies like dark data migration and strong security, which wouldn't be possible without this. Smart bots are also the consequence of processing massive data to analyze the required data. According to Big Data made easy, about 90% of the data on the planet has been generated within the last two years only instead of over a lengthy time.
What is Big Data Analytics?
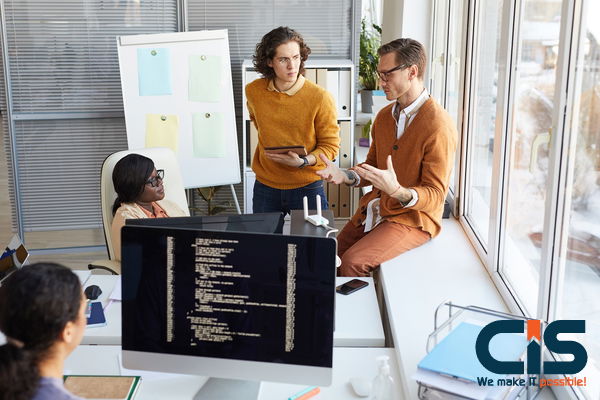
In its broadest definition, according to the most comprehensive definition, the term "big data analytics" refers to advanced analytics that entails sophisticated tools and applications that employ statistical algorithms and predictive modeling powered by high-performance analytical systems. Analyzing large and complicated data gathered from various sources, including digital platforms, social networks such as for weblogs, internet data surveys of customers' sales records, IoT sensors that collect data and sensors, etc.
The primary goal of analytics is to discover crucial information, such as customer preferences, patterns of data and their correlations, and market trends that assist businesses in making better business decisions.
Companies across the globe are recognizing the significance of running analytics software to analyze data flowing across the enterprise in various types and silos. Applications based on big data analytics help data analysts, statisticians, scientists, and professionals in predictive modeling to effectively analyze all types of untapped data flowing through the enterprise.
Integrating semi-structured, structured, and unstructured data visualization from enterprise allows businesses to obtain the required useful insights and use them to make business-related strategic decisions.
Trends Driving Progress in Big Data Analytics
Data analytics has emerged as the primary factor in competitive advantages over the past few years. Due to its significance and importance, businesses need to remain on top of the latest trends within the field of data analytics and the impact of these trends on various related technologies.
Hybrid Clouds
Cloud computing is a system that combines an on-premises private cloud and an outside cloud, which is orchestrated by two different interfaces. A hybrid cloud gives you great flexibility and greater possibilities for data deployment by transferring processes between public and private clouds. An enterprise requires a private cloud for the ability to adjust to the aspirational public cloud.
Data Fabric
A data fabric can be described as an architectural framework that collects data networks. This provides the same functionality across various devices, including on-premises and cloud-based environments. Data Fabric simplifies and integrates data storage across both on-premises and cloud environments to drive the digital revolution. It allows access and sharing of data within a distributed data environment.
XOps
The goal in XOps (data, model, model, platform) is to improve efficiency in scale and economics. Xps can be achieved through the implementation of DevOps top practices. This ensures effectiveness, reusability, and reproducibility while cutting down on technology, replication, and automation. These technologies will enable models to scale with a flexible design and a flexible orchestration of controlled systems.
Data Quality
Data quality is one of the top concerns of businesses in the coming years. The ratio is lower when companies are aware that data quality will be an important issue. On the contrary, this is not a problem for the companies. So far, businesses aren't focusing on the quality of the data generated by various mining tools, resulting in poor data management. If "Data" is their key decision-maker and plays a significant role, they may be making the wrong goals for their company or have targeted the wrong audience. It is the reason filtration is essential to reach real goals.
Want More Information About Our Services? Talk to Our Consultants!
Bringing IoT, Data Science, and Big Data Analytics Together
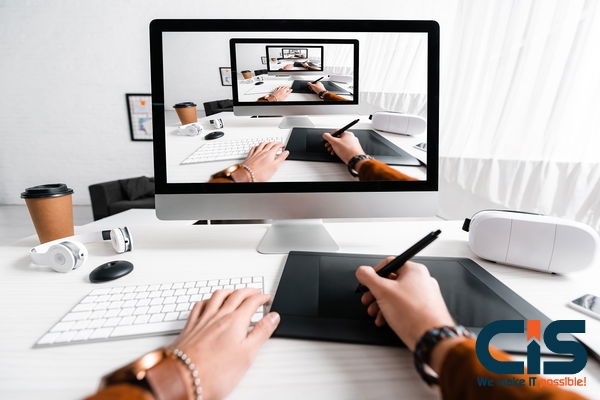
IoT requires advanced infrastructures that include sensors-based hardware, software programs, and operating system. Enterprises must manage the massive quantity of data. Analyzing it in real-time is an enormous challenge as the number of data increases in frequency every minute. This is where Big data can help. Numerous BI and big-data analysis tools and applications can handle the huge amounts of data transmitted by IoT devices that generate an unending stream of data. The IoT technology provides the data, from which Big data analytics draws the data to generate the information required by it.
The next-generation analytics database uses GPU technology, which reduces the size of infrastructure and hardware further. This allows IoT companies to connect the ever-growing quantity of data sets and provides immediate responses and adjust to changes in the market, addressing the problem of size without sacrificing performance. The application of Artificial Intelligence technologies like deep learning is a major advantage to gain insights quickly from the massive amounts of data. Through IoT, Big Data analytics could also be at the forefront of real-time decision-making, such as identifying patterns in agriculture crops by using drones in remote locations, detecting suspicious activity at ATMs, or anticipating drivers' behavior in connected vehicles.
With the growing popularity of the Internet of Things, we will also be seeing major cybersecurity concerns and safety concerns emerge since cybercriminals can hack into the electricity grid traffic systems and any other system connected to it that contains sensitive information that could shut down cities. Therefore, there will be a need for IoT devices to be protected from security breaches by using cloud-based solutions.