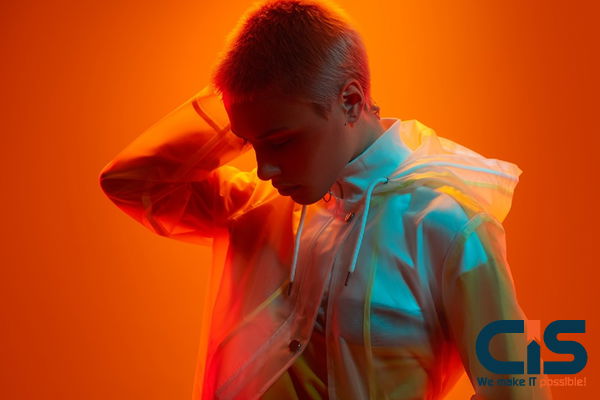
These are only a few of the changes in expectations. This is something that all employees in companies need to address. It doesn't matter if one of these digital heavyweights is a competitor. It's because disappointing customer expectations can be a gateway to competitors. This is a competitive advantage for competitors who take advantage.
Data is the foundation of digital companies. Digital companies can understand better and respond to customers, communicate better, and make more persuasive communications. Data handling creates competitive advantages that can transform entire industries. This data fixation is a trait passed down from generation to generation who grew up in the digital age. The optimal data flow is crucial for the organization's structure and internal design. Companies whose methods and organizations come from different times and have different paradigms will find it more challenging. Data is crucial for success.
This is a learning process that is essential for the long-term survival and growth of many businesses. These issues should be addressed by those in charge of companies. artificial intelligence (AI) is currently reshuffling the cards in markets like the insurance industry. Data is the foundation of AI applications.
Success is Dependent on the Data
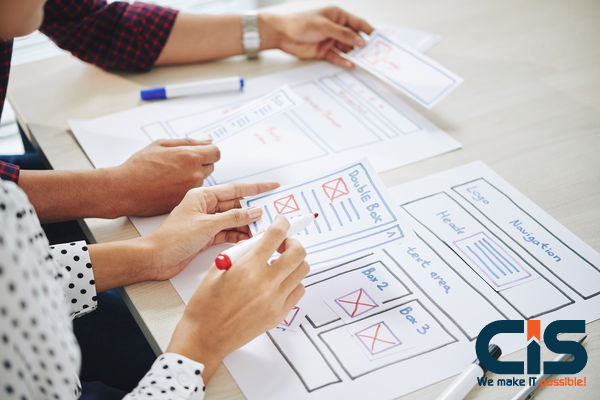
Although AI-based processes are not entirely new, the pace of their adoption is only now starting to pick up. These tools create new channels for offers, services, and communication. There are numerous uses: In the background of speech recognition apps, AI is at work. This is valid for programs that prepare documents for automatic processing by extracting information from them. A use case is crucial in insurance, especially when processing claims or applications.
In the areas of language, writing, and image recognition, AI is powerful. Appropriate processes and data structures are necessary for successful AI use. Data planning is crucial.
A company's data strategy establishes the basic guidelines for managing data. This approach handles concerns like data types, origin, administration style, use authorizations, deletion, and access. The foundations of any strategy can be established based on the data life cycle.
Phase 0. The Creation and Generation of Data
It is necessary to pay attention to the many data sources available. All data sources contribute to the continuous flow of information, whether business processes, IT systems or knowledge workers, customers, machines, or business processes. The necessary condition that all information can be used later is a requirement for decision-makers.
Phase 1. Managing Data, Recording it, and Storing it
There are two options for companies: centralized storage in data lakes or central storage systems like document management systems and business applications. Decentralized storage can be used in different ways on other devices and local devices. Both have their advantages which we will discuss in greater detail later.
Phase 2. Sharing Data
Data must travel from the point where it was created to the location where it will be processed. Data flow in a structured and defined way between IT systems. Access to portals, apps, and communication channels, such as email or collaboration platforms, is available to employees.
Phase 3. Using and Processing Data
Correctly interpreting data can make data valuable and meaningful. There are many ways to do this. This can be done in many ways, including creative processes, data analysis, and enrichment by experts. The study of extensive, unstructured data and its resulting forecasts using AI methods like machine learning is another option that has been becoming more important in recent years.
Phase 4. The Deletion of Data
Even though storage costs are falling into a black hole, data deletion is essential to the life cycle. For data protection purposes, this can be subject to regulatory requirements. Targeted cleaning can also improve data quality.
When managing their data, businesses must keep an eye on these procedures from a great height. The specifics are challenging to define. What competencies do businesses need to manage data effectively? What duties are necessary? What is a technology, organizational, and process blueprint? Companies must collect and process data following standardized guidelines. They must also use the information for professional purposes. Industrial businesses make use of components and raw materials. The finished product is what comes out of the process. The structure of present-day companies and insurance firms will be based on data flow. Planning centers are responsible for the creation of a structured approach.
A data platform refers to a mix of processes and technologies. It is designed to facilitate the use of data within a company. The forum will allow companies to share their experiences with networking and structuring data-based technologies and processes. This includes the initial data source and implementation of new AI-driven offers and services.
The development of uniform structures is one of the main issues with data management. To solve this issue, AI uses the juxtaposition of isolated silos. Contract documents are kept in the CRM solution, while customer service requests are kept in a different programme. Sales are unaware of marketing, and vice versa. Because of this, automating the personalization of customer interactions is challenging. Eliminating silos and establishing consistency in roles are crucial because of this. Then AI applications can show off their prowess.
How can companies move from their current structure to one that is based on data requirements? There are two ways to reach the goal, each with advantages and disadvantages.
The Way to The Data
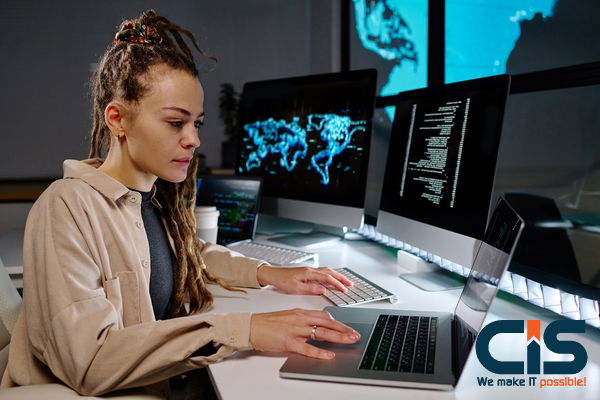
Approach 1. Parties involved implement processes that have taken a while to prepare and were carefully planned. The data platform is now ready to use after many rounds of coordination and years of planning.
Approach 2. The first Artificial Intelligence solution is followed by the second and third. Each project is tapped into again by the involved parties, who then design new processes and use different data formats.
The similarities between these two approaches can be easily seen in practice, despite their descriptions appearing overly simplistic. There is a chance with the first strategy of over-engineering. The experts might develop an idea for an ivory tower.
It is a system that can manage any situation. It takes a long time to implement. When put into practice, it eventually falls apart from every angle and comes to an end. The second strategy, however, needs to pay more attention to the strategic objectives of a data-driven business and instead places an excessive amount of emphasis on the operational level.
Quick implementation and immediate success can be accomplished through a patchwork of individual steps. This strategy works well for small-scale use cases but not for widespread adoption. Companies cannot benefit from "industrial" data processing with this strategy.
When developing a data platform, a middle ground is preferable. The bigger picture must be considered as each project is carried out. Therefore, building a data platform should be ongoing, permanent, adaptable, iterative, and agile.
Want More Information About Our Services? Talk to Our Consultants!
The people involved must closely or simultaneously coordinate several fields of action to produce operational results and lay strategic foundations. These include architecture, procedures, and consulting/use cases, among other topics. Competencies and organization are also included. The team faces many uncertainties, particularly during the early development projects. This is due to the low experience levels and high obstacles. Every new project needs to have all necessary skills, interfaces, and technologies available, as well as processes and methods that have been integrated into the system. Every stage introduces a new data platform that enables data to move largely automatically.
It is essential to make data profitable. This means that companies must adapt to ensure data flows smoothly. This is a tedious process but crucial for the company's viability in the future.