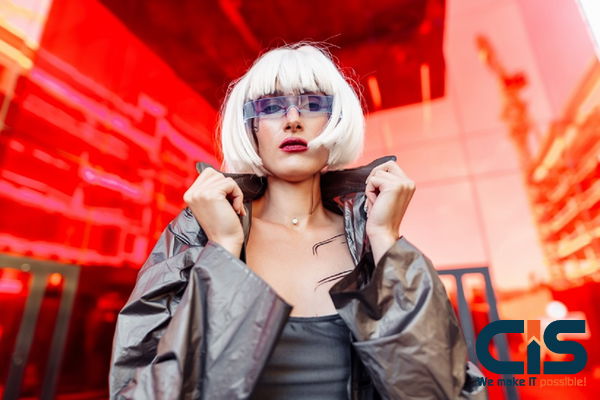
Artificial Intelligence's potential to transform how we work and live has made it an intriguing topic in recent months. It has led to much discussion surrounding its fundamentals, forms, mechanisms, and creative uses for AI use not forgetting its future. In this piece, we will delve deep into artificial intelligence's fundamentals, including forms, mechanisms, and creative applications, while discussing responsible AI use & its future potential.
What is AI?
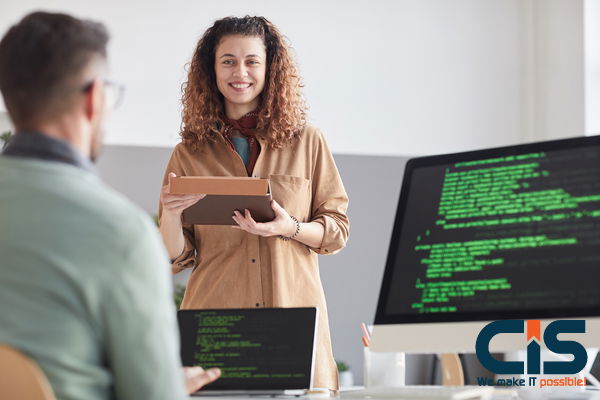
Artificial Intelligence, commonly referred to as AI, refers to a group of technologies that enable computers to perform complex tasks, including data analysis, question answering, visual perception, language understanding and translation, artistic creation, and video production - among many other capabilities. Artificial intelligence's incredible potential can include turning text prompts into high-quality images (like those seen here), removing background from photos more straightforwardly, and autonomous driving cars without human drivers using chatbots powered by artificial intelligence.
Which Key Trends Will Influence AI In The Future?
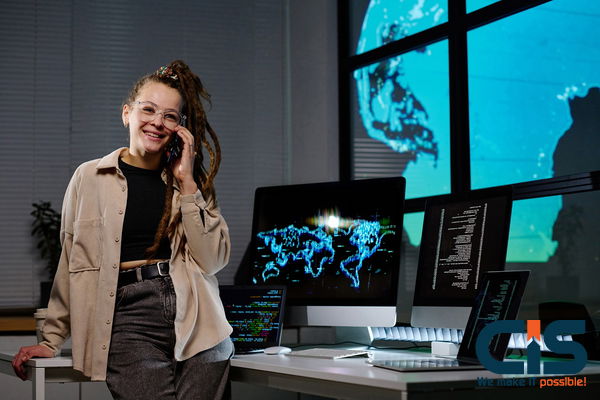
Before now, AI systems relied upon four main factors to advance:
- More processing power
- Data availability
- Better algorithms and tools
- Increased processing power for artificial intelligence solutions
All four areas remain areas for significant progress, although setting goals or deadlines for them is challenging. Blockchain technology and cryptography offer new solutions that make crowdsourcing AI models faster by increasing processing power as well as data availability.
Advances In Computing Power
Deep learning demands more processing power to address more intricate problems. Current technology often does not deliver results quickly enough, and therefore, more processing power may be necessary to produce results at an adequate pace. With new computing technologies available today, businesses can employ AI models that learn to solve harder-than-ever challenges with increasing success.
AI-Enabled Chips
By itself, even the most sophisticated CPU may not be sufficient to increase an AI model's effectiveness. Businesses require powerful processors to use AI applications like speech recognition, computer vision processing, and natural language processing. Chips equipped with artificial intelligence capabilities offer one solution: CPUs become "intelligent" enough to focus on task optimization independently while increasing productivity becoming vital components in new AI technologies that tackle more difficult challenges more rapidly than before.
Chips featuring artificial intelligence capabilities are becoming ever more expensive for companies like Google, Amazon, and Facebook, leading them to invest more and more money into startups developing these types of chips. Below is an overview of global equity funding available to startups developing AI-enabled chips.
AI chips will enable predictive analytics and faster query processing in next-gen databases and are essential in providing intelligence delivery systems in industries like healthcare and automotive. We have compiled an exhaustive, sortable list of businesses working on developing these chips.
Advances In GPUs
One of the most popular kinds of AI-enabled chips in the market is the GPU.
Simple processing power is needed to render an image, but it must be done quickly and on a big scale. Since GPUs can handle thousands of simple tasks at once, they are the best choice in these situations. Because new GPU technologies complete these easy tasks much more quickly, they produce images with higher quality.
These days, GPUs are powerful enough to be used for tasks other than image rendering, like machine learning and cryptocurrency mining. Although CPUs typically perform these tasks, data scientists have found that these are parallel, repetitive tasks. GPUs are, therefore, frequently utilized in AI models for effective learning.
Quantum Computing
Conventional computer systems operate using two states binary (0 and 1) for their operation. While quantum computing operates similarly, its application of quantum mechanics extends further; qubits rather than bits may be utilized within quantum systems both consisting of zeros and ones, but qubits contain both simultaneously along with another state that contains them both simultaneously making quantum computing quicker at performing some tasks while opening new possibilities; these tasks could include neural network optimizations or digital approximations applications as examples of tasks which benefit from using quantum technology.
IBM claims that within 10 years, a quantum computer featuring fifty to one hundred qubits will be possible to construct. Quantum computing holds much promise as an additional processing option when we consider that even its 50-qubit computer operates faster than all 500 current supercomputers combined.
Advances In Data Availability
As we've already established, data availability has steadily been on the increase over time. It is projected to rise further with the increased adoption of Internet of Things devices.
Advances In Algorithm Design
Artificial Intelligence models will always evolve as their capabilities increase, often rapidly. Improvements to algorithm designs make AI accessible and efficient for a wider range of users with lower technical expertise and are listed here as notable developments.
Explainable AI (XAI)
The complexity of AI models is one of their major shortcomings; understanding their workflow takes time and programming expertise; therefore, businesses typically rely on AI output without fully grasping how it operates.
Explainable AI (XAI) provides an answer by making these models accessible to everyone. Three major goals of XAI include:
- The impact of the AI model on developers and users
- How it affects data sources and results
- How inputs lead to output
AI models will soon be capable of diagnosing illnesses with great accuracy; doctors must, however, understand how AI makes its analysis through XAI to best communicate this situation to their patients. Our comprehensive guide can give more details click here if interested.
Transfer Learning
Transfer learning is a machine learning technique that enables users to leverage an artificial intelligence model already applied for another task, thus saving both time and resources by applying this strategy in certain instances. There may be various reasons to employ transfer learning:
- Some AI models require several weeks for proper functionality, and training is often difficult. Developers have an option when faced with new tasks to use a pre-trained AI model instead, cutting training time.
- Where there may not be enough data, businesses may turn to pre-trained models instead of solely depending on small amounts. To obtain accurate results more reliably and swiftly.
Trucks can also be identified through AI models trained on cars. Trucks will benefit from using this knowledge gained through cars instead of having to start from scratch themselves.
Reinforcement Learning (RL)
Reinforcement learning (RL), one branch of machine learning, can teach artificial agents to act in ways that maximize rewards for themselves. Contrary to traditional learning systems, reinforcement learning doesn't search for patterns to generate predictions; rather, RL learns through experience and makes decisions sequentially in order to maximize rewards for itself.
Google DeepMind AlphaGo has become synonymous with reinforcement learning (RL). It recently won two consecutive Go games against Ke Jie, widely considered the world's top Go player. As reinforcement learning advances further into autonomous vehicles and factories alike, its applications could only increase.
Self-Supervised Learning (Self-Supervision)
Self-supervised learning (also referred to as self-supervision) is one form of autonomous supervised learning that does not rely on humans labeling data; rather, it performs this labeling task autonomously. According to Yann LeCun, vice president and chief AI scientist for Facebook and chief AI scientist. Understanding human-level intelligence relies heavily on self-supervised learning.
Although self-supervised learning techniques have traditionally been limited to computer vision and natural language processing tasks such as image colorization or translation, their usage will likely grow substantially over time. Here are a few potential applications of this form of self-study learning:
- Healthcare: This method can be utilized both during monocular endoscopy and robotic surgeries for estimating dense depth estimation.
- Autonomous Driving: Off-roading allows us to assess terrain roughness and driving depth accurately.
Read more: 4 Types of AI - How Much Will They Transform Our World?
Advances In AI-Building Tools
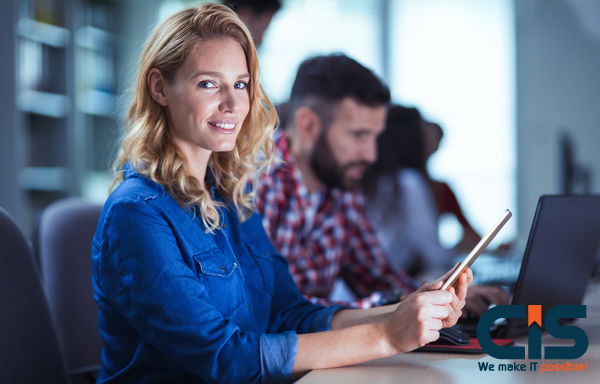
Although not new, these algorithms can speed up the creation process for models while aiding AI research and applications.
Neural Network Compatibility And Integration
Data scientists face an immense task when selecting and implementing the ideal neural network framework. With so many AI tools on the market available for neural network framework implementation, selecting one may prove challenging yet once trained using one AI tool, it may prove challenging to integrate models across frameworks once trained using another tool.
Microsoft, Amazon, and Facebook are joining forces to develop Open Neural Network Exchange (ONNX), an initiative that will facilitate trained neural network models' seamless integration across varying frameworks. It is anticipated that ONNX will eventually become essential equipment in this sector.
Automated Machine Learning
AutoML helps businesses address complex business problems quickly. Analysts no longer need to undergo lengthy manual machine learning training procedures with this technology while developing brand new models to address AI challenges quickly without spending too much time figuring out workflow; that way, more time can be dedicated to solving your case itself than trying to understand workflow details.
AutoML provides businesses with flexible models for various business scenarios through portability and data. Read our article to gain more knowledge of AutoML.
Advances In Collaboration In AI Model Building
Though tools have made model building simpler, creativity remains at the core of AI models. Companies can find thousands of data scientists willing to work on their problems by hosting data science competitions.
Prior to now, concerns over data confidentiality limited the expansion of such platforms. But thanks to modern encryption methods, businesses are now able to publically share data and leverage crowd intelligence without disclosing private details.
Key Trends In AI
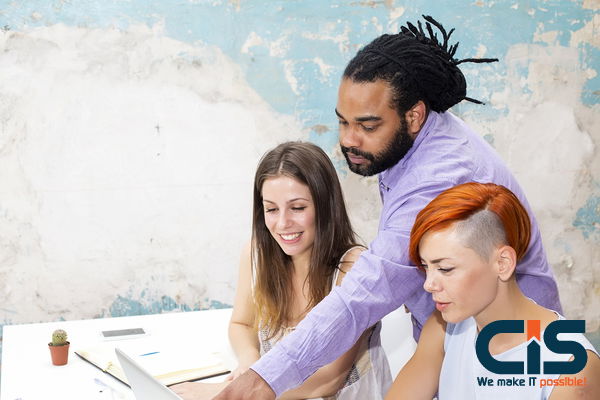
AI technology is rapidly progressing, and businesses must keep abreast of its latest trends and applications in this space. Examples include modern machine learning algorithms and developments in natural language processing as two examples that may profoundly transform multiple sectors of our economy and way of life. As the AI market matures in 2024, let's examine three trends from this sector.
Creative Or Generative AI
Artificial Intelligence (AI) refers to the process of producing original text, music, and image content using artificial intelligence techniques. Through generative AI, models are taught to recognize patterns and structures within preexisting data and apply that knowledge in creating original, one-of-a-kind content such as drawings, paintings, or any visual media form. Creative AI allows for the production of music using preexisting songs and techniques, innovating new compositions while keeping moral concerns about ownership of generated content at bay. Therefore, humans must maintain harmony between human creativity and artificial intelligence in creative fields such as music composition.
ChatGPT
As InstructGPT's sibling model, a chatbot designed to follow instructions, ChatGPT may sound familiar. Our marketing team uses this tool for client communications enhancement; though useful in terms of efficiency and support services, it must still be watched out for potentially false information when writing dialogue or providing customer service situations.
AI For Personalization
Artificial Intelligence has had an incredible impact on technology. Businesses using AI enable customers to enjoy a tailored customer experience tailored precisely to meet their individual needs, with online stores using it as part of personalized search results and past purchases to suggest products most likely appealing to individual users based on past search histories, purchases history or preferences; AI technology then suggests these products according to user behavior analysis something which helps increase customer satisfaction, loyalty, and user experience overall while improving the overall user journey. AI-powered personalization offers multiple potential advantages that make this an essential element of modern tech today.
Predictions For The Future Of AI
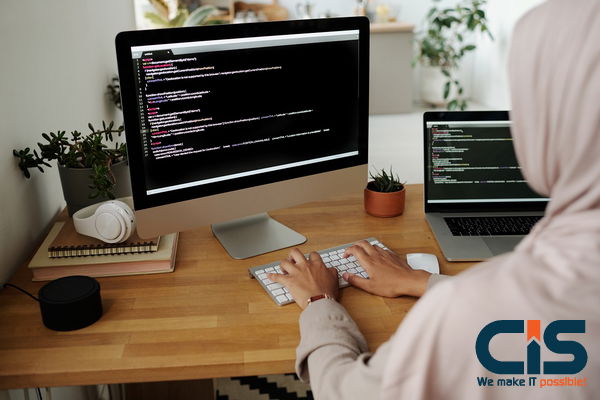
Technology will play an increasingly pivotal role in shaping our world, and we can anticipate breakthroughs down the road as it advances further.
How can we get ready for what's coming up? It's simple to raise awareness and continue learning new things. AI may seem unreal at times; however, its capacity for automating repetitive tasks and freeing human time may enable us to focus more creatively and imaginative projects than before. AI can benefit a broad array of professionals, such as physicians. AI may even save lives through the analysis of medical imaging. AI should become increasingly integral in transportation as well, becoming common in future models with cutting-edge tech over self-driving cars like Teslas that currently dominate this sector. Furthermore, it will streamline supply chains and logistics networks while increasing effectiveness and economy simultaneously.
Conclusion
Artificial Intelligence will revolutionize all organizations. While its effects might differ depending on your specific company's circumstances, most will follow a road map we outlined earlier in this book to implement and use AI technology effectively in your workplace. When applied correctly, AI opens up an amazing future where everyone will make better decisions thanks to data-rich computers that understand our environment not simply knowing when switches need turning on but understanding why as well. In the future, they may ask why there even need to be switches.
AI may not solve every business challenge your company is experiencing. Still, it has the power to change how your organization conducts itself radically. AI's impact can be felt across manufacturing and finance industries alike and results in previously unseen increases in productivity and efficiency. As more industries adopt and experiment with the technology, newer applications may emerge creating even larger changes than computing devices ever did including how we do business, receive medical diagnoses, carry out surgeries, and operate motor vehicles not forgetting computer vision technology financial, modeling, medical, imaging industrial processes already being transformed by AI.