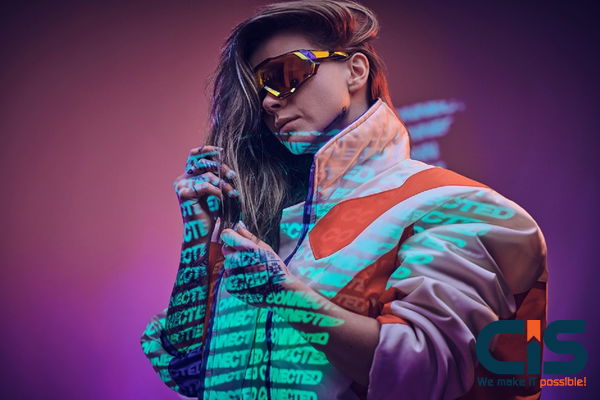
Automated Machine Learning: Advantages
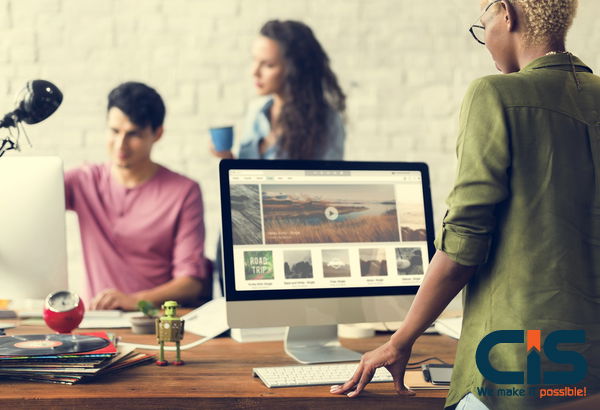
Efficiency
Users of AutoML can transfer data to training algorithms and then look for the optimum neural network design for a given problem. The time needed to complete data science projects is significantly decreased as a result. With AutoML, many tasks that used to take hours can now be finished in a matter of minutes.
Scalability
Access to machine learning techniques and technologies is made possible via AutoML, making machine learning more widely available. By bridging the talent gap, autoML tools enable businesses to expand their AI deployments.
Error reduction
Before AutoML, data scientists had to do laborious, manual procedures with their data. These tiresome duties were frequently the cause of mistakes produced by people. Data scientists were able to automate time-consuming, repetitive activities thanks to autoML.
Why is AutoML important?
Experts in machine learning are in high demand. The enormous number of available positions that outnumber the number of qualified applicants demonstrates this. By automating complex tasks that might otherwise be too challenging for novices in the industry, AutoML is a tool.
Machine learning software that is simple to use and understand has been made possible through automation. This makes machine learning accessible to analysts, marketers, IT professionals, and other professionals that aren't data scientists. Machine learning that has been scaled across several industries might benefit all enterprises. This enables them to be more effective and efficient when it is needed.
AutoML is for whom?
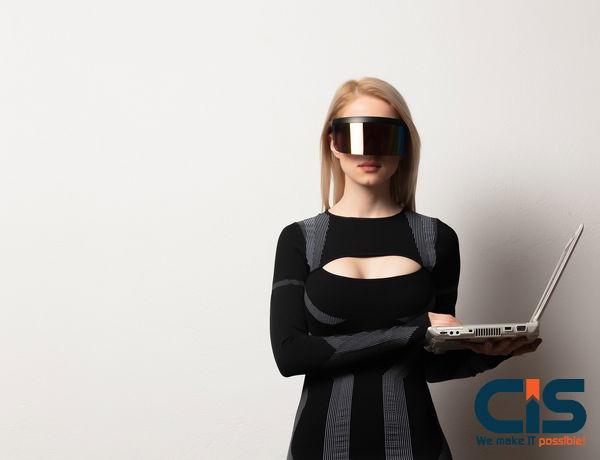
The use of AutoML is highly recommended for both experienced and new AI practitioners. The AutoML function in H2O is a wrapper that performs numerous modeling-related operations that would often need many lines. Users in multiple industries gain from this, including:
Financial services: Automation Technology is used by traditional financial institutions and fintech businesses to address issues with AML and transaction fraud, credit risk loss, trade failures, and client attrition.
Government: Government organizations are utilizing AI and AutoML to optimize massive data sets to lower fraud, waste, and abuse and improve supply chain management, cyber security, and human resources.
Health-AutoML: provides private and public healthcare professionals with the best data and practices to assist hospital operations, clinical applications, the life sciences, pharmacy, precision medicine, supply chain, finance, marketing, and human resources.
Relevant information: and best practices for supporting hospital operations, clinical applications, life sciences, biopharmacy, precision medicine, supply chain, finance, marketing, and human resources are available to the general public and healthcare professionals.
Insurance AutoML: is used by the insurance sector to close knowledge gaps, improve claims administration and precision pricing, reduce client turnover, and combat fraud.
Manufacturing: By applying demand forecasting, predictive machine maintenance, returns forecasting, and problem detection within their supply chains, leading manufacturers use AI/machine learning to reduce costs and streamline their operations.
Marketing: Marketing firms and agencies use AutoML to develop market forecasts, determine the best advertising locations, identify investment possibilities, target lead generation, or cross-sell campaigns, and implement funnel forecasts.
H2O Driverless AI & amp AutoML:
The AutoML tool from H2O streamlines the machine-learning process. This comprises the automatic training and adjustment of several models over a predetermined period.
H2O offers a range of model explanation techniques that may be used with both individual models and AutoML objects (groups or models). Automatic explanations can be produced with a single function call. The AutoML models can be explored and explained using this straightforward interface.
Automated Machine Learning vs. Other Technologies & Methodologies
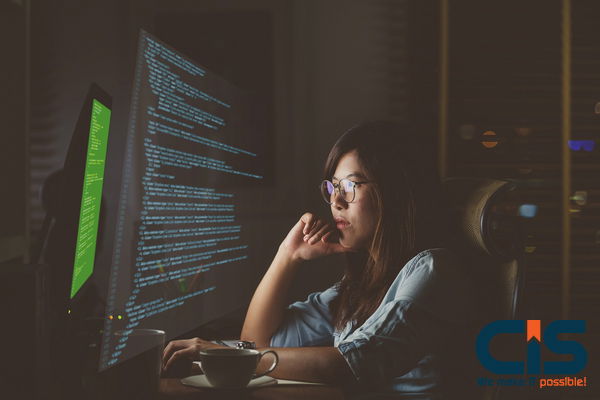
The stages involved in machine learning are automated using AutoML. Software with artificial intelligence mimics human thought.
Data science vs. autoML
Data science is anticipated to be replaced by autoML eventually. Therefore, businesses ought to invest. The "AutoML vs. Data Science" attitude is fundamentally incorrect. Without the aid of a data scientist, AutoML may use some components of the machine-learning pipeline. This does not, however, discount the importance of data science. By automating repetitive activities, AutoML is frequently used to expedite data science. Data scientists may devote more effort to solving complex technical issues thanks to autoML.
Statistic vs. AutoML
Prediction, statistics, populations, and hypotheses are the main focuses of autoML. The Machine Learning Lifecycle: What Is It? The technique of machine learning (ML) is frequently applied in data science initiatives. The ML cycle constantly improves data, models, and evaluations, even though it is not a simple procedure. The ML lifecycle can be used by organizations to aid in the creation of acquisition and management processes for value.
Phase 1 Data
High-quality data form the foundation of a good model. The first step in the machine learning cycle has data that can be utilized to train the model. These are what Phase 1's first stages entail.
Data collection
Large volumes of raw data must be gathered to fix any issues that may arise during model performance. Searching for information that relates to the project is a smart idea. The internet, databases, files, and mobile devices can all be used to collect data. Phase two will include processing the data. Therefore, the caliber of this stage will be less crucial.
Data preparation
Data organization and randomization are referred to as "data preparation." During this step, data can be examined to understand better the elements that might lead to a more effective output.
Data Cleaning
Cleaning raw data is referred to as "data cleaning." In order to fix problems and transform them into acceptable formats, this includes utilizing a range of filtering procedures. Data cleaning can fix errors, duplicates, and missing data. Data cleansing ensures that bad data does not affect the results of ML.
Read More: What Is Machine Learning? Different Fields Of Application For ML
Phase 2: Model
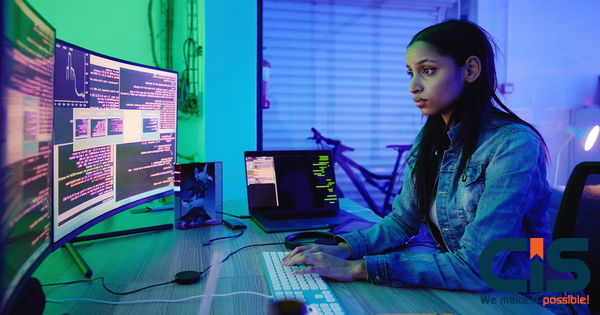
In phase two, using the data from phase one, the ML model is built.
Model development
Model development is the main component of the ML cycle. The selection and implementation of a baseline architecture are the first steps. Starting with a simple model is optional. For accurate results, use a straightforward model.
Training
Datasets are used during training to improve the model's performance and produce favorable results. It's critical to comprehend how a model operates with patterns, rules, and features.
Phase 3: Evaluation
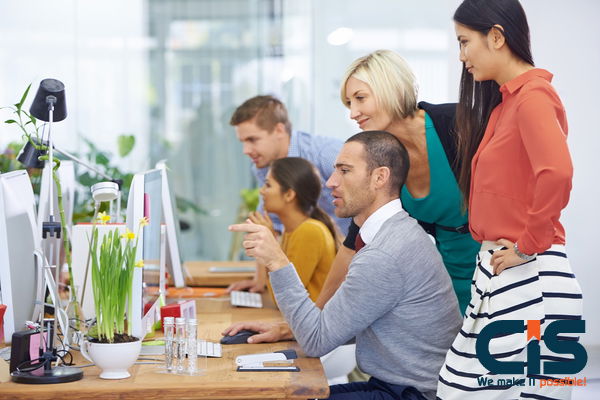
The model must be tested before it is put into use. During the evaluation step, the model's precision and accuracy are verified. Any errors will be identified, and their causes will be discussed thoroughly. To ascertain whether the solution achieves the objectives outlined at the project's outset, the assessment metrics will evaluate the various models.
Phase 4: Production
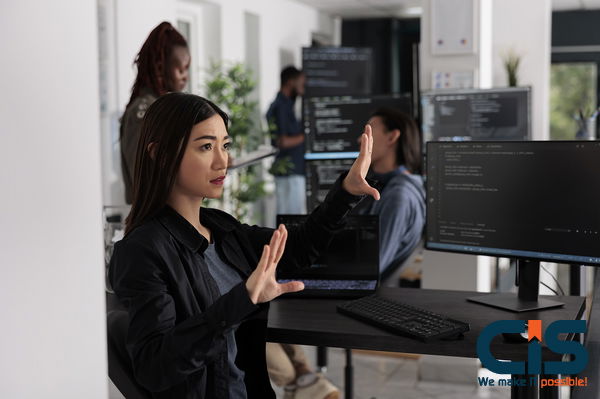
After the model has been evaluated and trained, it's time to make it. There are some challenges to overcome before the model can be deployed. ML monitoring should be performed frequently to ensure that the model runs smoothly and operates as expected. To ensure that the model works correctly, any new data must be checked. Production is a continuous testing phase that looks for potential problems and edge cases. Finally, the model can be deployed.
The Machine Learning Life Cycle has many Advantages
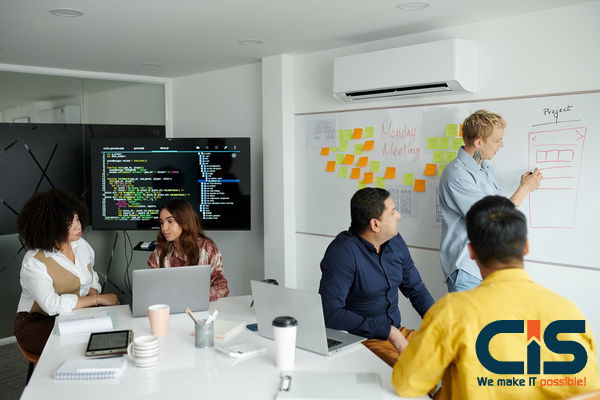
The ML lifecycle will aid teamwork and make it easier for non-technical folks to comprehend what is needed to finish a project. By unifying procedures and terminology and enabling the team to be more comprehensive, the ML lifecycle can also enhance performance and productivity.
Different types of Machine Learning
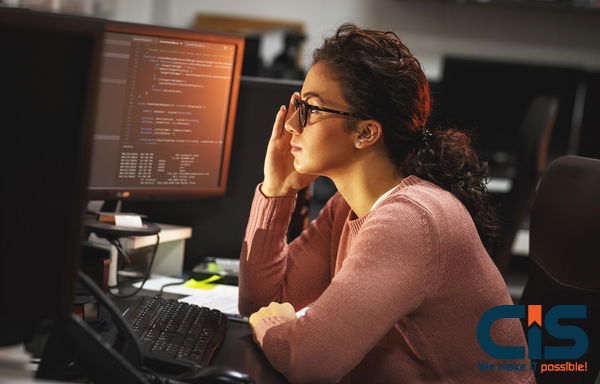
A subset of AI called "machine learning" enables a machine to learn from data and enhance its performance based on prior arrangements. Additionally, it predicts. Machine learning is known from a lot of data using a variety of algorithms. Data is fed into these algorithms to train them. They develop the model and do the task based on the training.
These machine learning (ML) methods can address various business issues, including regression, classification and forecasting, clustering, associations, and more.
- Machine learning may be categorized into four categories based on how it is carried out.
- machine learning under the supervision
- Automatic Machine Learning
- Machine Education Under Some Supervision Reinforcement Learning
- You will receive a thorough explanation of the various Machine kinds in this chapter.
Supervised Machine Learning
As the name suggests, supervised machine learning is based on supervision. The supervised learning technique uses the " labeled " dataset to train the machines. The devices then predict the output based on the training. According to the data, some inputs have been mapped to production. Another way to describe it is that after the machine has been trained with inputs and outputs, it is asked to predict the results using test data.
Let's have a look at an illustration of supervised learning. Consider a dataset of pictures of dogs and cats. The machine will first need to be trained to comprehend the photos. Cats' and dogs' tails vary in height, color, and shape.
Then, after providing it with a picture of a cat, we will ask it to identify the object and output the results. The machine has been programmed to examine each object's characteristics, including height, shape, and color. Then, it will recognize it as a cat. It will subsequently be classified as a cat. In supervised learning, the computer acknowledges objects in this way.
Classification
Classification algorithms can address issues like "Yes or No, Male or Female, Red or Blue, etc." when the output variable is categorical. The categorization algorithms can predict the categories in the data. Examples of practical categorization algorithms include email filtering and spam detection.
Regression
Regression methods can be used to tackle issues where input and output variables have linear connections. These algorithms are employed to forecast continuous output variables like market movements and weather forecasts.
Read More: Machine Learning And Deep Learning Are Becoming Increasingly Important For Businesses
Unsupervised Machine Learning
Compared to supervised learning, unsupervised learning is different. As implied by its name, it is not supervised. As a result, unsupervised machine learning refers to a technique in which the computer trains itself using unlabeled data and then guesses its results independently.
Unsupervised learning occurs when models are trained on unlabeled, unclassified input and act independently.
The primary goal of the unsupervised learning method is to classify and group unsorted data into groups based on patterns, differences, and other characteristics. The input dataset will be sent to machines with instructions to find hidden ways.
To better understand the idea, let's look at an example. Assume there are numerous fruit-related photos. They are entered into the computer learning model. The machine must identify patterns and classifications for the items because the model is ignorant of the images.
The computer can now recognize patterns and differences (such as differences in color and shape) and predict the outcome when tested using the test dataset.
The clustering technique is employed to find the pre-existing groups in the data. This is a technique for classifying items so that those with the most similarities are kept together and those from other groups have fewer or no similarities. Clustering algorithms can be demonstrated by categorizing clients according to their purchasing patterns.
- Some of the most well-liked clustering methods are listed below:
- Principal Component Analysis, Mean-Shift Algorithm, and the K-Means Clustering Algorithm
- Analysis of Independent Components
- Some of the most well-liked algorithms for learning association rules include Apriori and Eclat.
- Apriori Algorithm and Eclat are some of the most popular algorithms for Association rule learning.
An unsupervised learning technique called association rule learning uncovers fascinating connections between variables in huge datasets. This algorithm's goal is to map two data items in a way that maximizes profit by determining the relationship between them. This algorithm is mainly used in the continuous product, market basket analysis, web usage mining, and other fields.
Want More Information About Our Services? Talk to Our Consultants!
Conclusion
Machine learning (ML), a branch of artificial intelligence (AI), is the branch of computational science that is concerned with the analysis and interpretation of patterns and structures in data to enable learning, reasoning, and decision-making without the involvement of a human. Simply defined, machine learning enables users to send massive amounts of data into computer algorithms, which then analyze, recommend, and decide using only the supplied data. The algorithm can use the knowledge to improve its decision-making in the future if any corrections are found.The Importance of Machine Learning
All businesses depend on data to function. Making decisions based on data-driven insights might mean the difference between staying competitive or falling further behind. In order to harness the value of corporate and customer data and make decisions that keep a business ahead of the competition, machine learning may be the answer.