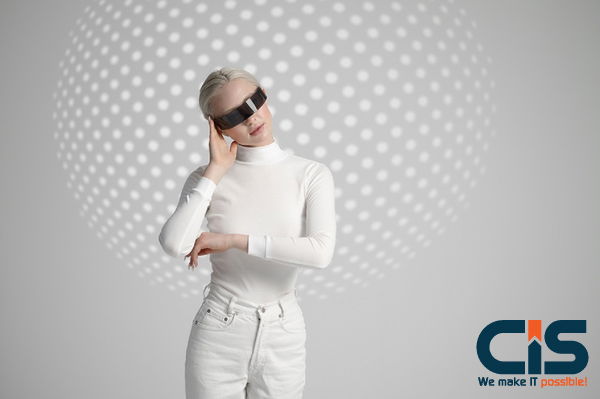
Artificial intelligence (AI) refers to technologies including Machine Learning (ML), deep learning, and other forms. Although often discussed interchangeably, AI/ML/DL technologies have enormously influenced healthcare industry services provision and assessment, with remarkable progress made despite many false starts during experimentation periods. Currently, two fundamental questions related to healthcare AI/M projects remain: 1. Investment levels 2) What projects use this form of Intelligence?
What Does the Phrase "Artificial Intelligence" Mean?
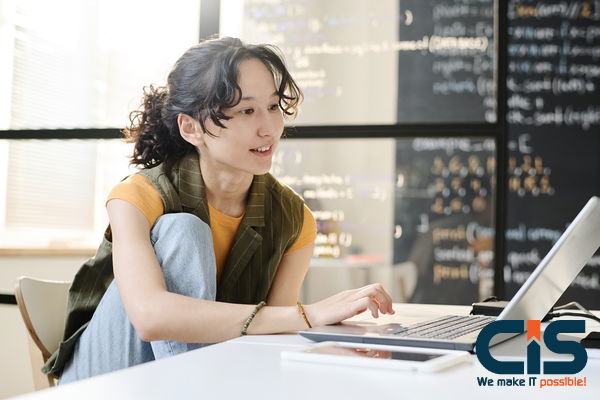
AI (Artificial Intelligence) is the art and science of designing intelligent machines through algorithms and rules that mimic cognitive processes, such as problem-solving and learning, to the extent possible. AI systems can anticipate issues or address them immediately as they arise - operating deliberately, intelligently, and adaptively as necessary.
AI also recognizes patterns from multidimensional datasets - for instance, it could convert patient medical records into one number that represents their likely diagnosis - while being dynamic autonomous adaptable systems learning new things as more data becomes available to adapt themselves accordingly and discovering new data continuously over time as new data becomes available - thus creating intelligent machines capable of learning as further information becomes available.
AI does not represent one ubiquitous technology; it encompasses many fields like deep learning, machine learning, and others that collectively add intelligence to software applications. machine learning (ML) algorithms help computer programs adapt to customer experience by improving themselves through repetition; classification options include unsupervised, supervised reinforcement learning (RL) and ongoing research into subfields such as self-supervised and multi-instance ml.
- Annotated data can enhance learning processes. for instance, they use X-ray images with tumour annotations to aid in recognizing them on new photos.
- Unsupervised learning means extracting information without labeling data directly, for instance, by categorizing patients who share similar symptoms to determine if there could be one root cause.
- Recurrent learning agents are instructed by experts or taught through trial and error to maximize rewards by adopting new strategies that maximize returns; many major AI breakthroughs of recent years were due to this form of AI education.
- Deep learning (dl) is an algorithmic class that utilizes numerous layers of interlinked processes, providing these processors with data samples from many examples to train themselves on. deep learning has quickly become one of the primary forms of artificial intelligence. it has driven significant improvement across fields like speech recognition and image analysis. but how can ai-augmented health systems become efficient and trustworthy?
Building AI systems for healthcare has many applications; unfortunately, they're often implemented without considering local context (clinical workflows, user needs, trust issues, and ethical implications). there are various approaches for creating artificial intelligence for health; unfortunately, too often, attempts are made at fitting square pegs into round holes by finding problems AI could solve without considering the entire environment.
Our approach to AI innovation in AI healthcare solutions stems from its ability to complement human intelligence rather than replace it. when developing AI systems for healthcare applications, the key lies in making sure vital human elements don't get left behind but instead focused and enhanced - AI innovations often result from understanding complex patient journeys as a human-centric journey map.
Develop and Design
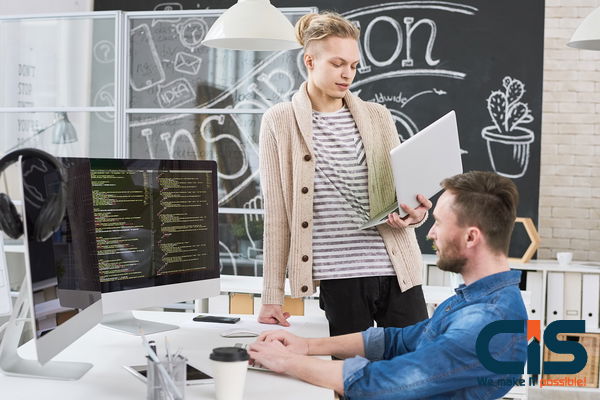
Prioritized AI solutions must first be designed and created for specific problems using an approach that prioritizes humanity while including relevant stakeholders in its creation and deployment.
Co-creation and Engagement of Stakeholders
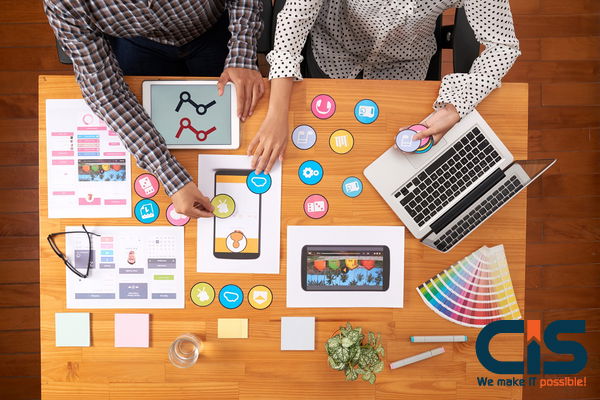
Create a team of multidisciplinary experts from social and computer scientists, operations leaders and research managers, clinical stakeholders (physicians, caregivers, and patients), and biomedical researchers as subject matter experts (subject matter champions and financiers). Multi-stakeholder teams bring technical, operational, and strategic expertise to identify problems, objectives, success metrics, and interim milestones.
AI Human Centric
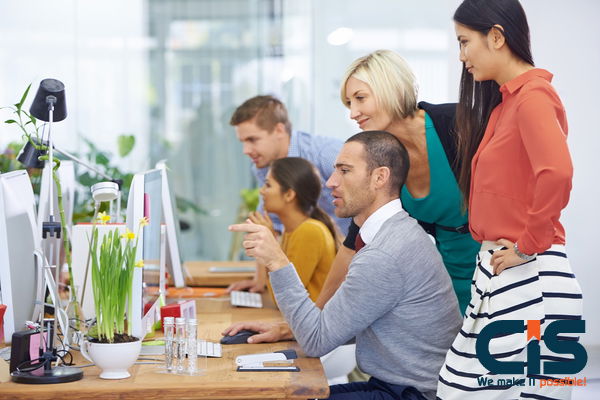
An ethnographic approach to AI marries AI technology with knowledge of health systems. First, through user-designed studies, identify significant problems. (We advise conducting qualitative research to understand if an issue is important, who it affects, why no steps have been taken to address it, and any barriers preventing AI integration).
Healthcare organizations face numerous requirements and constraints that necessitate AI integration; here, we examine their needs, workflows and conditions, and any barriers or facilitators. Once fundamental problems are defined, the next step should be identifying those AI could solve; any datasets available could assist in AI creation. AI systems could be integrated into an existing workflow to solve their specific user problems.
Experimentation
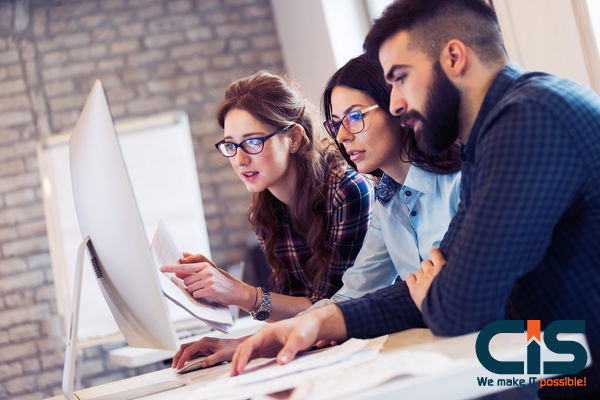
The focus should be piloting new AI experiments with stakeholders and tight feedback loops for rapid learning through experience and incremental change. Experiments allow simultaneous testing of different ideas enabling stakeholders to see which ones work and why, while experimentation and feedback help define the purpose, intended use, end users, and more for AI systems.
Validate and Evaluate
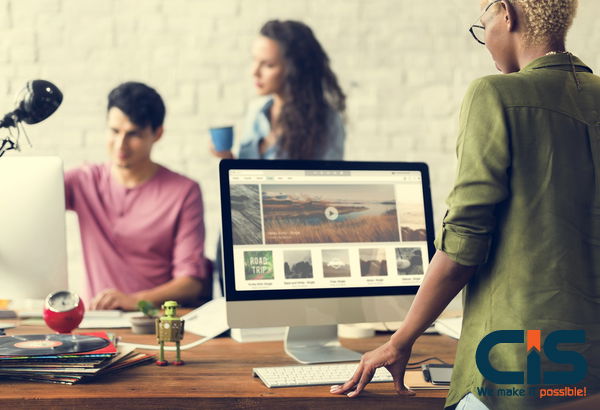
The next step should be validating and assessing AI tool predictions to understand their effectiveness in terms of statistical validity, economic value, and clinical utility. The evaluation must take place along three dimensions; statistical validity, economic value, and clinical utility must all be carefully considered when assessing such tools.
- Acknowledging AI's accuracy, reliability, and robustness is central to understanding its statistical validity. High performance in retrospective settings or silico alone does not demonstrate clinical impact.
- An algorithm should be evaluated on an external dataset with longitudinal and temporal validation data in real time to demonstrate clinical utility.
- The economic utility is the difference between benefits and costs when considering AI Systems.
Size and Dispersion
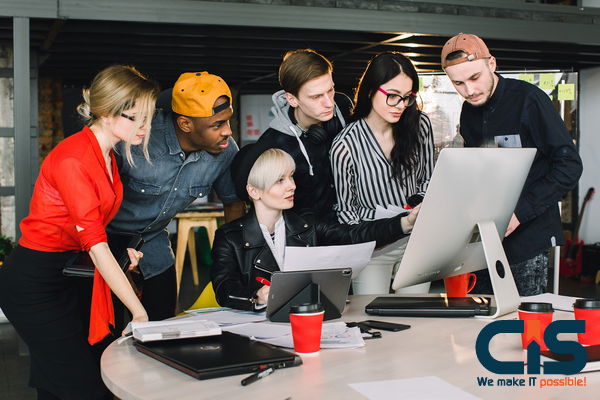
At first, AI systems were intended to address specific healthcare problems related to patients and their contexts. With scaling AI forward comes special considerations regarding deployment methods, model updates, and regulatory systems.
Related:- Is SAP an enterprise system? Explain
Maintain and Monitor
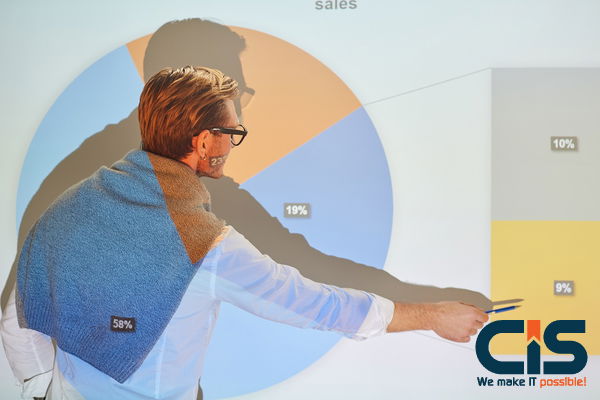
Once an AI system has been introduced into clinical settings, it must be carefully observed for risks or adverse events that might arise through post-market monitoring. AI developers, healthcare organizations, and regulatory agencies must collaborate in this endeavor by gathering and analyzing pertinent data about performance, risk, and safety in AI performance, and clinical risks and safety.
1. Providing Robotic Surgery
AI can be employed in numerous ways to advance healthcare. Collaborative robots equipped with AI have revolutionized surgery by decreasing risks related to bleeding, pain, and side effects; and helping surgeons perform more complex operations more accurately, which results in quicker patient recovery times.
Maastricht University medical center utilizes AI-powered robotics to stitch small blood vessels - some as small as 0.03 millimeters wide - using AI. Surgeons can access information in real-time about patient health status using this data source; prompt decisions can then be taken before, during, and post procedures to achieve positive outcomes for every treatment procedure.
2. Detecting Fraud
US Justice Department estimates that three percent (three) of healthcare claims are fraudulent, resulting in annual losses estimated to exceed $100 billion. AI allows healthcare professionals to detect invalid claims quicker, approve valid ones faster, and pay them. AI technology can also detect insurance fraud and protect patient privacy by helping detect patient data theft.
Harvard Pilgrim Health, an industry leader, is turning to artificial intelligence (AI) systems as part of its fight against healthcare fraud. AI-powered programs detect fraudulent behavior while flagging suspicious patterns of behavior.
3. Supporting Clinical Decisions
AI and machine learning (ML) are revolutionizing how clinical decision-makers make their choices. AI provides data that assists clinicians with diagnosing, treatment planning, and health management decisions, as well as data-intensive fields like radiology, pathology, and ophthalmology. AI may eventually automate specific tasks; using natural language processing, it could even translate clinical notes directly into EHRs so clinicians only have to enter data once.
4. Helping With Repetitive Jobs
Healthcare industries are rapidly moving toward Cognitive Assistants, which possess the capabilities for reasoning, analysis, and complete medical knowledge. Medical Sieve, one of many recently introduced algorithms, has already proven capable of aiding radiology and cardiology decisions while acting as a cognitive health assistant that analyzes radiology images faster to detect issues quickly.
Medical Sieve, an AI healthcare system that uses deep learning and medical data to enhance patient care, is one example of using deep Learning with healthcare data to advance diagnostics. Enlitic is another example that also utilizes this mix.
5. Changes to Online Consultations and Personal Consultations
Babylon App demonstrates how AI can revolutionize how physicians consult. Offering online healthcare and medical services with expert AI advice tailored specifically for each patient based on medical history and understanding, Babylon App provides online healthcare and medical services with all kinds of advice from its AI engine that helps physicians improve how they practice medicine.
AI-powered applications enable the user to report symptoms through speech recognition technology. Once processed, this app compares them against disease databases before taking into consideration history and circumstances before suggesting an individual treatment plan. Over 54% of mHealth users utilize AI or Robotics in healthcare to meet their medical needs, underscoring its increasing acceptance. This trend showcases its growing acceptance.
6. Manage Your Medication and Get Health Assistance
Sense.ly, a startup in the medical industry, created Molly as its inaugural digital nurse. Molly boasts a pleasant voice and friendly appearance while serving to monitor the health and treatment needs of her patients between medical visits.
Machine learning technology enables customized monitoring programs explicitly designed to manage chronic illnesses between medical visits; an app offers customized testing monitoring of chronic disease management. AI helps ensure patients take their medications on schedule by reminding them when to do so.
7. Drug Production
Clinical trials can be costly and lengthy processes; AI offers a faster and cheaper alternative - more cost-efficient drug development through speedier prototyping of pharmaceuticals.
Atomwise is an AI-powered network of supercomputers that mine databases of molecular structures to discover treatments, like Atomwise used by researchers in 2015 to discover existing drugs on the market that might help combat the Ebola outbreak. They found two medicines using Atomwise AI; their analysis typically takes several years but was completed quickly using this innovative system.
8. Precision Medicine
AI has had an immense influence on medicine. This technology helps identify patterns within massive medical information and genetic data databases to track disease mutations more precisely and identify genetic changes from therapy or natural causes that change DNA structure. AI informs doctors as they observe what occurs after changes happen to the DNA sequence.
9. Analyzing a Healthcare System
Information regarding medical institutions, doctors, and treatments can now easily be retrieved as healthcare bills increasingly move to digital format. Data mining enables hospitals to quickly generate reports that show errors they make when treating certain conditions; this allows hospitals to improve treatment methods while decreasing hospitalization rates.
Zorgprisma Publiek in the Netherlands has employed Watson technology to analyze data compiled from hospital invoices.
10. Automatic Image Diagnosis
Computer vision is one of AI's most promising applications within healthcare, used by hospitals and clinics alike to detect abnormalities in various forms of medical images such as CT scans or radiology studies, CT scans being incredibly beneficial in this respect; embodiments also being utilized for diagnosis tumors, liver, and kidney infections as well as improving cancer therapy treatment plans - among many other uses!
UVA University Hospital's tool provides an outstanding example of AI-powered visual perception. We are utilizing artificial Intelligence and machine learning in healthcare to analyze children's biopsy images for celiac disease vs. environmental enteropathy - with similar accuracy to doctors.
Let's explore how artificial intelligence (AI) technology is revolutionizing healthcare.
AI Types in Healthcare
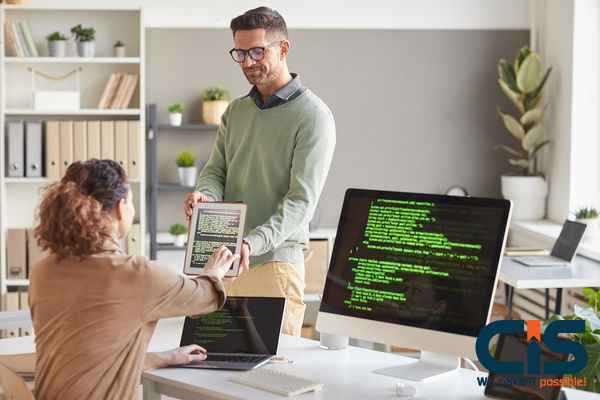
AI in healthcare refers to various technologies designed for specific uses and administrative tasks, all working together for tremendous industry success. AI technologies support multiple aspects of medical practice; discover some major systems here.
1. Machine Learning
Machine learning (ML) is an artificial intelligence practice widely utilized within the healthcare industry. Machine learning uses algorithms and data to mimic how humans learn.
Precision medicine has proven revolutionary within healthcare. Predictive analytics utilized by precision medicine uses patient attributes and prior treatment personalized experiences to predict which procedure would most suit a given individual patient based on an algorithm; most precision medicine applications utilize supervised learning utilizing known results as input data.
Deep learning -- using neural network models with multiple features or variables to predict patient outcomes of business models-- is the most complicated form of machine learning, capable of detecting potentially cancerous lesions on radiological images.
2. Natural Language Processing
Natural Language Processing, more commonly called NLP, encompasses text analysis and voice recognition, among other applications. NLP can be found across healthcare applications ranging from clinical documentation creation and classification through published research publications to unstructured patient notes that provide invaluable insight into improving methods, understanding quality issues, and offering better care services.
3. Robotic Process Automation
RPA (Robotic Process Automation) is a technology that automates business processes using robotic technologies to mimic and learn rules-based procedures. RPA systems are transparent, affordable, and straightforward to program compared with other generative AI solutions in healthcare; RPA's use in this sector automates complex tasks such as updating patient records or billing patients automatically.
4. Expert System Based on Rules
Rule-based expert systems represent one of the earliest forms of artificial Intelligence; they utilize knowledge-based rules to solve problems. Expert systems aim to transform expert human knowledge into hardcoded rules which can then be applied against input data.
Healthcare professionals often rely on rule-based systems to guide clinical decisions, which are easy to use and understand. But as more rules enter these systems, their business operation becomes unwieldy; recently, in healthcare, these approaches have been replaced with courses focused on machine learning and data.
AI Challenges in Healthcare
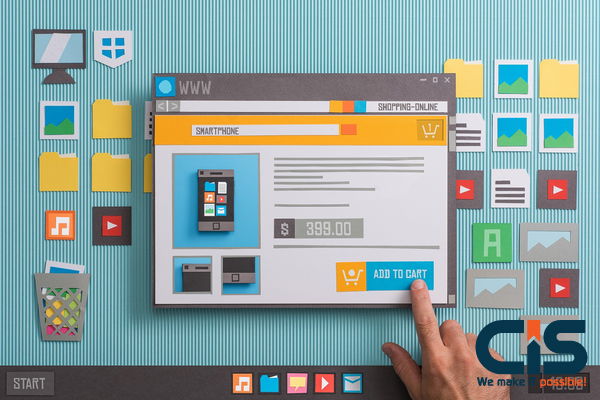
Though America spends more on healthcare than developed nations, its overall health outcomes remain inferior. Burnout among clinicians is another significant challenge. People of different generations desire tailored healthcare. Tom elaborates.
Millennials prefer consulting their doctor from their sofa while simultaneously ordering dinner, while other groups, such as baby boomers, may take an alternate approach; Baby boomers might select primary providers; we can utilize data and AI technologies to personalize care beginning with generational respect and within each generation (millennials or Gen Z for instance), we can personalize treatment according to its rules of engagement.
AI applications in the healthcare sector are constantly expanding, creating new challenges that must be met efficiently to realize AI's full potential in healthcare. From data security to quality issues, these challenges prevent AI from unleashing its full potential - so let's dive deeper into them to understand their effects on this industry better.
Data Access
Artificial intelligence in the healthcare sector presents several unique obstacles. Chief among them is data fragmentation due to patients visiting different healthcare providers, thus resulting in difficult-to-comprehend and expensive data sets and costly errors.
Privacy Issues
Implementing AI into healthcare involves meeting strict security measures to protect sensitive patient information in large amounts of collected data. Therefore, finding an ML/AI development company equipped with various security features for customer protection should be of prime importance.
Injuries and Errors
AI systems may make errors when detecting risks and creating treatment plans. If AI-powered computers prescribe incorrect medications to patients or fail to locate tumors on radiology images, there could be serious health repercussions.
AI: Healthcare Workers Should Not Fear It
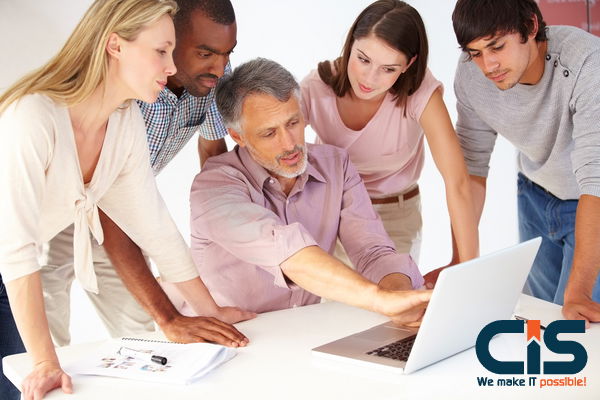
Artificial intelligence will enormously affect healthcare workers, but don't fret: computers won't replace healthcare providers anytime soon. Tom describes artificial Intelligence (AI) as an expert at pattern recognition - capable of sorting through vast amounts of data quickly to find something humans are unable to or would take years for. Humans excel at common sense, creativity, wisdom, and empathy - these characteristics all play an essential part in caring processes.
Clinicians must recognize AI's power and accept that things are changing quickly. An intelligent healthcare system won't take away jobs; instead, it will improve clinicians and their patients. All parties involved can easily claim victory.
AI in Healthcare: The Promise of Big Promises (Examples)
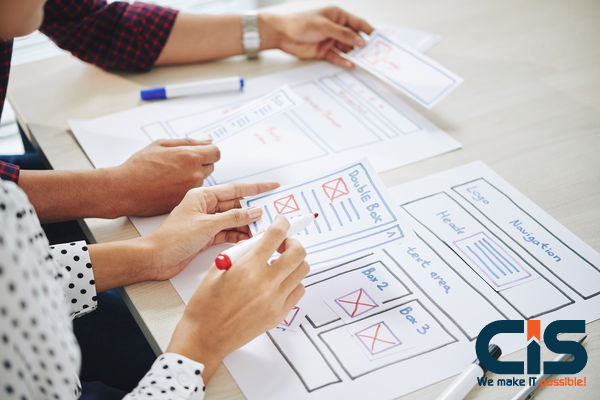
You acknowledge their terms by accepting our terms of service, privacy statement, and class action waiver. It's encouraging that many large healthcare organizations are now beginning to use AI in some capacity, as we have only just begun exploring its possibilities in improving healthcare delivery.
Organizations use machine learning and AI to anticipate future events, from emergency room volumes to optimizing staffing requirements. According to Tom, healthcare development teams have also adopted natural language processing to aid radiologists' work and enhance patient interpretation. He states:
Radiologists typically review images for one specific reason - why you sought an examination - yet often see something in the background, using natural language processing tools to identify secondary issues for further investigation that might otherwise go undetected.
AI's most significant contribution to healthcare lies in improving clinical workflows. AI adds immense value by automating and augmenting clinicians' work; AI can also automate repetitive tasks to boost health professionals and patients. Healthcare organizations will find success if they can redesign their processes and workflows while using AI/ML technologies to develop an intelligent healthcare system.
AI's Potential in Healthcare
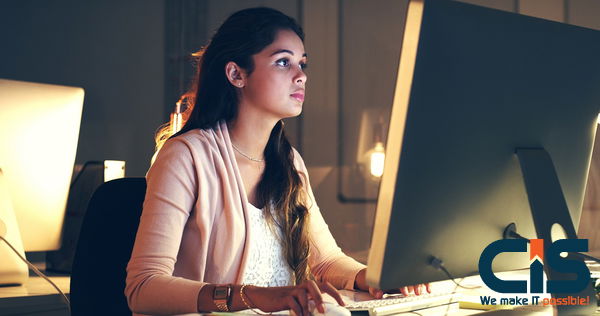
AI has already revolutionized healthcare maintenance practices and operations within hospitals and patient experiences. Medical units use this type of AI-powered predictive analysis for everything from designing effective breast cancer treatment plans for women to forecasting emergency room volumes - to name a few use cases!
AI represents only the start of an incredible journey. AI may provide next-generation radio instruments with enough accuracy and detail to replace tissue samples when necessary, helping providers better define cancer aggressivity and determine optimal treatments. AI technology also permits virtual biopsying capabilities while expanding on advanced radio mics.
Electronic health records can also assist healthcare providers in quickly and accurately identifying at-risk patients and infection patterns before symptoms appear. At the same time, AI's analytical abilities allow healthcare providers to have quick and precise alerts of conditions such as seizures or sepsis, requiring intense analysis of complex data sets.
Want More Information About Our Services? Talk to Our Consultants!
Conclusion
This revolutionary technological trend is revolutionizing healthcare delivery systems and leading to significant advances in patient care, leading to clinical excellence with noticeable advancements.
AI in healthcare is quickly gaining steam and seeing increased adoption, helping drive efficiency, improve patient care quality, optimize operations, and decrease system burden. Conversational AI will also play an integral part in making processes and workflows in this sector more streamlined, equitable, and tailored to individual patients' needs.