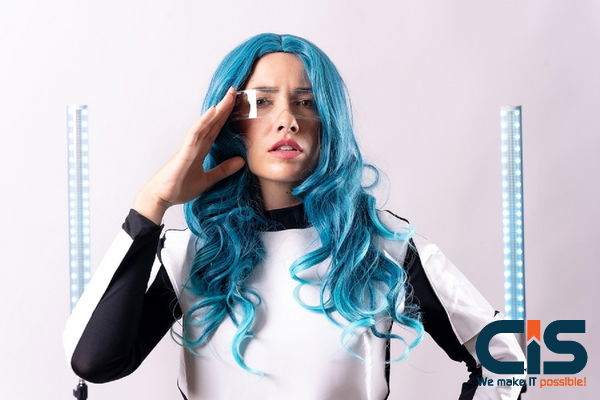
Frequently, as I work with initiative groups to foster the organization's digital transformation procedure, it isn't uncommon to discover critical goals, for example, "Deploy AI-based solutions for acknowledging xx% income learns," or "carry out AI to expand function and effectiveness."
These unclear, significant-level key objectives deceive an absence of comprehension of the abilities of AI-based processes and how they could truly enhance organizations. In this article we will learn more AI solutions for business in a wide range.
Artificial Intelligence can be identified as the automation of a cognitive process. The utilization of Artificial Intelligence Solutions processes upholds organizations in more ways than one, including automation of excess tasks, digitizing processes, as well as the quick analysis of data, especially huge data sets, and combining various data sets to acquire fast insights and knowledge that add to decision-making.
Moreover, AI processes empower flexibility in an unbendable conventional business environment. For instance, increasing hyper-customized client interactions and backing with digital gateways, for example, chatbots and virtual helpers.
In light of a 2022 review by McKinsey and Company, organizations are utilizing AI as a device for creating value, and these organizations intend to put much more into AI because of the Covid-19 pandemic and its speed increase of everything digital. Nonetheless, a new report by Gartner tracked down that just 53% of organizations had the option to move their AI confirmation of ideas to creation.
What is Artificial Intelligence?
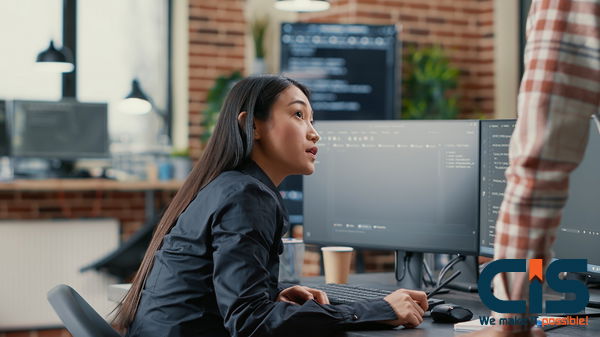
Plainly speaking, Artificial Intelligence is when machines can learn and settle on choices just like people. There are many kinds of Artificial Intelligence, including AI, where rather than being modified to figure out what to do, machines can notice, investigate and learn from data and slip-ups as much as our human minds can.
This innovation is impacting consumer items and has prompted critical leap forwards in medical services and physical science as well as altered businesses as different as manufacturing, money, and retail.
To a limited extent, because of the gigantic process of data we create each day and the computing power available, Artificial Intelligence has become popular lately. We may, in any case, be years from summing up AI-when a machine can do anything a human mind can do-however, AI in its present structure is as yet a fundamental piece of our reality.
A Catalyst For Digital Transformation
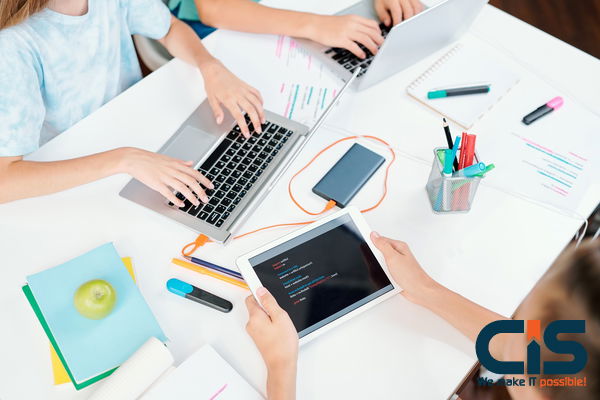
Digital transformation is an underlying update of an organization and its value chain. Artificial Intelligence systems are an impetus for digital transformation, empowering automation, and streamlining, as we are all keen on utilization of data to speed up Business Intelligence and further develop decision-making. Nonetheless, organizations commonly face a few boundaries that ruin AI execution.
Rather than review AI processes as an innovation execution, business pioneers should zero in on abilities and the potential business value that could be gotten from its execution. For instance, digitization of processes through digital robotics process automation(RPA) could understand huge functional proficiency results for the organization's functional environment.
Artificial Intelligence-based processes additionally make the possibility to increase and down business conditions, like the capacity to carry out hyper-customized client communications and backing at scale through digital channels.
Also, AI processes empower deep bits of knowledge from huge data sources or quickly consolidate huge datasets to determine smart insights. Organizations could use online media and CRM data to recognize new client segments or distinguish dynamic items or services that would speak to changing client inclinations.
To comprehend the abilities and value of Artificial Intelligence Solutions for business, chiefs first need to get what AI processes are. This doesn't mean acquiring specialized data on building the processes yet functioning data on what it is and how it very well may be carried out in the organization.
This incorporates understanding the difference between AI (automation of cognitive processes), machine learning (a way to get successful AI that offers PCs the capacity to finish undertakings with data without explicit programming), and deep learning (a specific method to carry out AI).
These terms are frequently utilized interchangeably in the business environment however convey particular implications and suggestions. In our present digital environment, data is democratized, and leaders who need to more deeply study AI systems can, without much of a stretch, access this data from solid sources, anyplace, whenever.
Want More Information About Our Services? Talk to Our Consultants!
Instructions to Deploy AI Solutions In Your Business and Conduct Artificial Intelligence Development
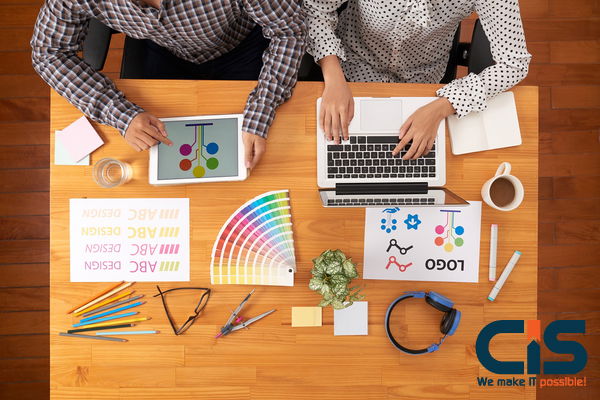
A beginning stage for any organization in AI solution deployment is to survey the current digital development of the organization, including the current innovation scene and accessibility of solid, evident data sources. At the core of AI systems are data, and this turns into a pre-imperative AI solution deploying, especially for any type of data analysis application.
An easier methodology is to start with off-the-rack solutions that are now in the market to drive speedier returns and acquaint the organization with AI-based systems. For instance, refined chatbots or virtual helpers fueled by AI and Ml have exhibited the capacity to drive critical improvements in client commitment and fulfillment.
Nonetheless, as organizations hope to speed up the digital transformation process with AI systems, it is basic to survey the network protection system to evaluate the dangers you might be opening up to with new innovation abilities.
Business pioneers need to guarantee that the perfect individuals and abilities are available to characterize, carry out and keep up with the AI systems conveyed. The jobs and abilities required are characterized as dependent on the solution methodology you need to carry out.
If your organization might want to set up an AI system for inward data analysis, recruiting in the necessary abilities would be basic to guarantee data protection and secrecy. Notwithstanding, if your organization chooses to make API joins for required AI processes, you may just need individuals with specialized data on APIs and networks to existing systems, either inner or outer assets. Also, there are various outside platforms that can speed up access to AI abilities and capacities.
Artificial Intelligence ought to be seen as a feature of the digital transformation process of your organization and executed just when it's a good idea for the business goals that business leaders are attempting to accomplish.
Regular Influences of Artificial Intelligence
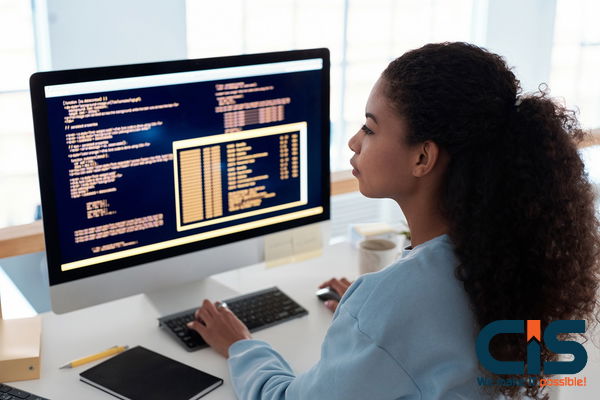
Numerous impacts of Artificial Intelligence are modifying the manner in which our regular routines look. If somebody from the 1950s went through time and showed up in 2019, they would wonder about the manner in which we utilize our cell phones to explore the city, how virtual digital assistants, for example, Alexa and Cortana, react to our questions and would be bewildered by our dependence on social media channels like Facebook, Instagram, and Twitter.
What is currently typical to us and controlled by AI would be absolutely unfamiliar to our companion from an earlier time. There's no question that digital Intelligence is a basic piece of our regular routines.
Our monetary foundations, legal infrastructure, media organizations, and insurance agencies are sorting out ways of utilizing Artificial Intelligence for their potential benefit. From error discovery to composing reports with normal language training and assessing law briefs, AI's scope is broad. Hence, AI can be used as a reliable to Financial Services Industry or financial management software solution too.
Artificial Intelligence Influences Business Process Management
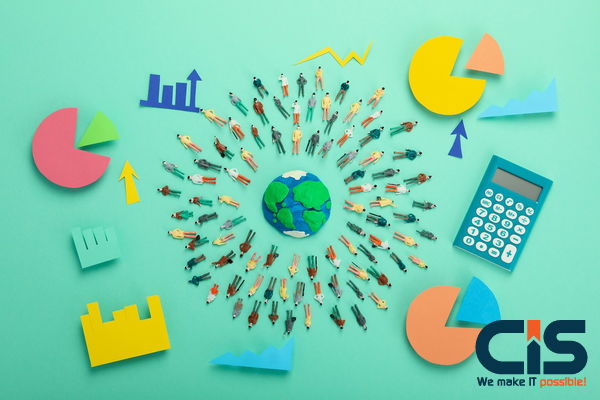
As business owners and trendsetters compete to arrive at the promise of artificial Intelligence to convey an upper hand as well as cost saving and time savings, the innovation is modifying enterprises from money to assembling new items, processes, and skills.
Organizations, for example, 150-year-old Heineken, are utilizing AI, the immense process of data they gather, and the Internet of Things to showcase choices and drives, further develop activities, and client support.
From overseeing global supply chains to enhancing delivery processes, Artificial Intelligence is helping organizations of all sizes and industries to further develop products and the bottom line functions at each phase of the business process management, from sourcing material to sales and bookkeeping to client assistance. It permits organizations to configure, create and convey items and services in excess of anyone's expectations.
Life-Saving AI
Artificial Intelligence isn't simply improving lives but it will save lives too. There are many organizations, including tech organizations, that are putting resources into and investigating ways that Artificial Intelligence can assist with further developing our medical care framework. From sorting out customized drug conventions to better analytic tools and even robots to aid medical procedures, AI is modifying our medical care system from its process to the care that these organizations give.
Notwithstanding the systems of our medical service, AI is probably going to be extremely instrumental in tackling the environmental concerns we are encountering because of global warming. From supporting a smart infrastructure to being more proficient with our regular resources and smart farming to taking care of a growing population, AI can expand our human efforts to tackle our worldwide difficulties. It's also a core part of our well-being and security systems.
Engaging AI in Business Process Management
Artificial Intelligence has been deployed to upgrade our lives too. Analysis with AI to form unique syntheses from books to music, build plans dependent on what's right now in the cabinet, and even to make show-stoppers are broadening the impact of AI on our lives. The AI-fueled recommendation engines of Netflix and Spotify assist with smoothing out our decision-making process when we are searching for a show to watch or tune.
Unmistakably if we removed AI, our reality would look different inside and out. As the current investments and analysis bring about extended and culminated use of AI, we can anticipate that the advanced technology should turn out to be significantly more important in our day-by-day presence, work environments, and society. The most effective method to track down the right Artificial Intelligence Solutions
Artificial Intelligence (AI) brings incredible new tools to fight dangerous actors. However, picking the right blend of libraries, test suites, and trading models when building AI security systems is profoundly reliant upon the circumstance. In case you're pondering over accepting AI in your security operations center (SOC), the below-mentioned inquiries and contemplations can assist with directing your decision-making.
What Problem are You Trying to Solve?
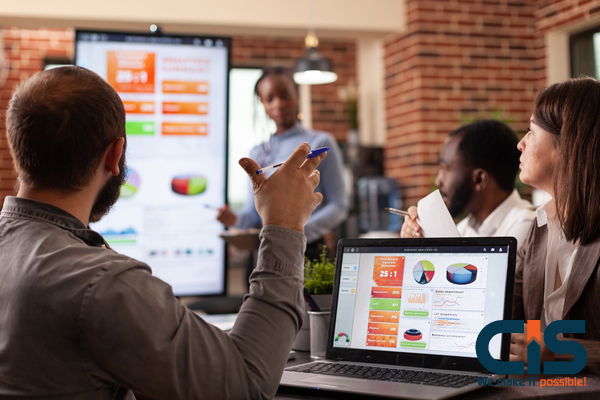
Spam recognition, intrusion discovery, malware identification, and regular language-based risk hunting are largely different issues that require diverse AI tools. Start by thinking about what sort of AI security systems you need.
Understanding the results assists you with testing data. Find out if you're tackling classification or regression problems, constructing a recommendation engine, or identifying oddities. Contingent upon the responses to those inquiries, you can apply one of four fundamental sorts of AI:
Supervised learning trains an algorithm dependent on model processes of data/outcome sets. The objective is to foster new inferences dependent on patterns induced from the sample results. Test data should be available and named. For instance, planning a spam location model by learning from tests named spam/nonspam is a decent use of supervised learning.
Unsupervised learning utilizes data that has not been named, arranged, or sorted. The machine is tested to distinguish patterns through the process, for example, group analysis, and the result is generally obscure. Unsupervised AI is acceptable for finding hidden samples and data but is a bad choice for a regression or classification issue. Network anomaly detection is a security issue that fits well in this type.
Semi-Supervised learning utilizes a mix of named and unlabeled data, ordinarily with the greater part being unlabeled. It is principally used to work on the nature of training sets. For exploit kit ID issues, we can track down some known exploit kits to prepare our model; however, there are numerous variations and obscure units that can't be marked. We can utilize semi-supervised calculations to resolve the issue.
Reinforcement learning looks for the ideal way to an ideal outcome by persistently rewarding improvement. The issue set is, by and large, small, and the training data is well understood.
An illustration of reinforcement learning is a generative adversarial network (GAN, for example, this trial from Cornell University wherein distance, estimated as right and mistaken pieces, is utilized as a loss capacity to scramble messages between two neural organizations and try not to listen in by an unapproved third neural organization.
Artificial Intelligence Depends on Good Data Just Like Business Intelligence
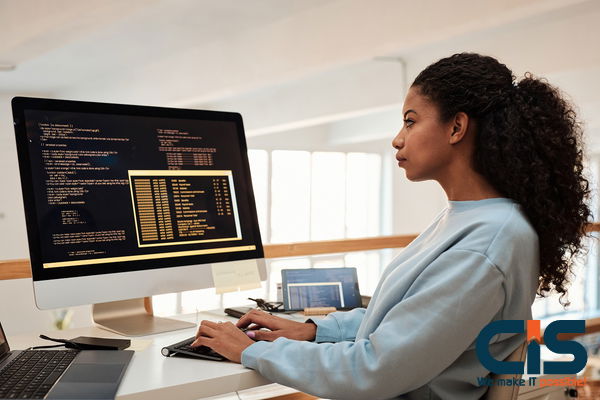
AI is predicated on learning from data, so having the right amount and quality is fundamental. Security pioneers ought to pose the following inquiries about their data sources to streamline their AI organizations:
- Is there sufficient data? You'll require an adequate amount to address all potential situations that a system will experience.
- Does the data contain patterns that AI systems can learn from? Great data indexes ought to have oftentimes repeating values, few out-of-range values, clear and obvious meanings, and persistence, meaning that they change little over time.
- Is the data inadequate? Are expected qualities missing? This can make misdirecting results.
- Is the data categorical or numeric in nature? This directs the decision of the classifier we can utilize.
Are Labels Available?
Is the data current? This is especially significant in AI security systems since risks change so rapidly. For instance, a malware recognition system that has been prepared on an old system will experience issues distinguishing new malware varieties.
Is the source of the data trusted? You would prefer not to prepare your model from openly available data in which you don't confide in. Data test harm is only one issue through which AI-based security models are compromised.
Picking the Right Platforms and Tools
There is a wide assortment of platforms and devices available, but how would you realize which is the right one for you? Pose the following inquiries to assist with educating your decision:
- How comfortable would you say you are in a given language?
- Does the tool coordinate well with your current environment?
- Is the apparatus appropriate for enormous data analysis?
- Does it offer built-in data parsing abilities that empower the model to comprehend the construction of data?
Does it Utilize a Graphical or order Line Interface?
Is it a complete AI platform or simply a bunch of libraries that you can use to assemble models? The last gives greater scalability but has a steeper learning curve and customer experience
Shouldn't something be said about the Algorithm?
You'll likewise have to choose an algorithm to utilize. Attempt a couple of various algorithms and compare them to figure out which offers the most dependable outcomes. Here are a few factors that can assist you with choosing which Algorithm to begin with:
What amount of data do you have, and is it of acceptable quality? Data with many missing qualities will convey lower-quality outcomes.
Is the learning problem unsupervised, supervised, or reinforcement learning? You'll need to coordinate with the data collection to the use case as portrayed previously.
Decide the kind of issue being addressed, like regression, anomaly detection, classification, or dimensionality reduction. There are diverse AI algorithms that turn out best for each kind of issue.
How significant is precision versus speed? In case approximations are satisfactory, you can get by with a more modest data set and lower-quality data. In case accuracy is of utmost importance, you'll need better data and more time to run the AI algorithms.
What amount of permeability do you require in the interaction? Algorithms that give decision trees show you unmistakably how the model arrived at a choice, while neural organizations are somewhat of a black box.
The most Effective Method to Train, Test, and Evaluate AI Security Systems:
Training models must be continually updated as new endeavors are found, so it's important to perform training on the fly. Be that as it may, training progressively opens up the danger of ill-disposed AI attacks in which attackers endeavor to disrupt the outcomes by presenting false input data.
While it is difficult to perform training offline, it is alluring to do when possible so the nature of the data can be controlled. When the training process is finished, the model can be delivered into creation.
One normal technique for testing trained models is to divide the data index and commit a part of the data - say, 70% - to training and the rest to testing. If the model is hearty, the yield from both data sets ought to be comparative.
A more refined methodology called cross-validation separates the data collection into groups of equivalent sizes and trains on everything except one of the groups. For instance, assuming the number of groups is "n," you would train n-1 groups and test with the one set that is left. This interaction is updated ordinarily, leaving out alternate groups for testing each time. Performance is calculated by averaging results across all repetitions.
The decision of evaluation metrics also relies upon the sort of issue you're attempting to address. For instance, a regression issue attempts to discover the scope of error between the real value and the anticipated value, so the metrics you may utilize incorporate mean outright mistake, root means an outright mistake, relative outright error, and relative squared mistake.
For a characterization issue, the goal is to figure out which categories new observations belong in - which requires an alternate solution of value metrics, like accuracy, precision, review, F1 score, and area under the curve (AUC).
Want More Information About Our Services? Talk to Our Consultants!
Organization on the Cloud or On-Premises?
Ultimately, you'll need to choose an area for the organization. Cloud Artificial Intelligence Solutions surely enjoy benefits, like the speed of provisioning, selection of devices, and the accessibility of outsider training data. Notwithstanding, you probably shouldn't share data in the cloud for security and compliance reasons. Consider these elements prior to picking whether to install on-premises or in a public cloud.
These are only a couple of the many variables to think about when building security systems with Artificial Intelligence. Keep in mind the best answer for one organization or security issue isn't really the best answer for everybody or each circumstance.