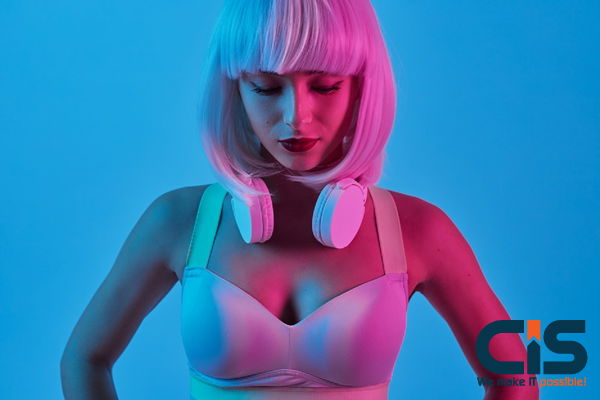
Let's get this party started! Let's set things in motion.
What Is Predictive Analytics For Customers?
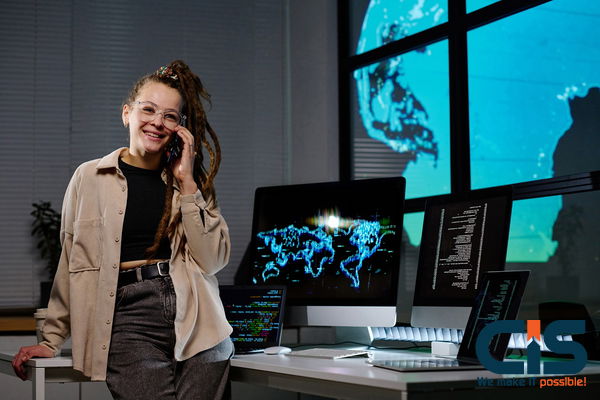
Predictive customer analytics involves analyzing and predicting future actions your customers take based on past behaviors, which involves employing historical data, machine-learning algorithms, and statistical algorithms.
Predictive Modeling Types
Models for predictive analytics use historical data to discover patterns and trends, using this insight to forecast future ones. Standard predictive analytics models include clustering, classification, and time series analyses.
Classification Models
Under supervised machine-learning models, classification models fall within this umbrella term. Classification models classify data based on historical records to describe relationships in a dataset and can serve as segmentation tools that segment customers and prospects into groups according to true/false or yes/no responses; typical applications of this type of model are credit risk evaluation and fraud detection; some common forms include logistic regression, decision tree, random forest and neural networks as classification models.
Clustering Models
Clustering models, used for unsupervised learning, group data according to similar attributes and can help an online store group customers into groups with similar characteristics and tailor marketing strategies. Standard clustering algorithms include K-means Clustering; Mean Shift Clustering; DBSCAN; Expectation Maximization Clustering using Gaussian Mixture Models (GMM); and Hierarchical Clustering.
Time Series Models
Time series models combine data inputs that change continuously with regularity (e.g., daily, weekly, or monthly). Plotting this dependent variable over time helps evaluate seasonality, trends, and cyclicality to assess seasonality or identify any transformation needed; for instance, the AR, MA (moving average), ARIMA, or ARMA models could all be employed here to predict call volume in different time slots, e.g., from call centers using time series models such as predictive call volume prediction to help make plans accordingly.
Customers Data: The Key To Success
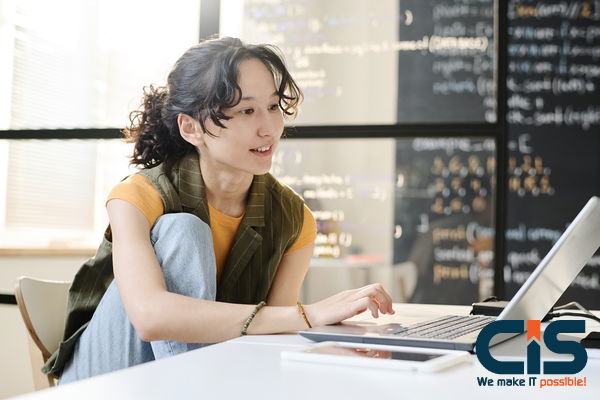
Predictive analytics thrive when organizations possess large quantities of customer data, such as social media posts, survey responses, transaction records, or customer interactions. With such rich resources available, they can gain a deeper insight into customers through data from sources like social media posts, survey responses, or transaction records, as well as customer interactions that provide vital customer interaction insights and identify trends as well as make predictions using this wealth of customer knowledge.
Case Study Anticipating Customers Preferences in E-CommerceTo illustrate the power of predictive analytics, we present a real-world example from an organization that successfully implemented it. They used it to create highly tailored product recommendations using data-driven approaches from preprocessing through feature engineering, model selection, and validation, ultimately leading to increased customer engagement and sales figures, as revealed through this case study.
What Are We Going To Do With It?
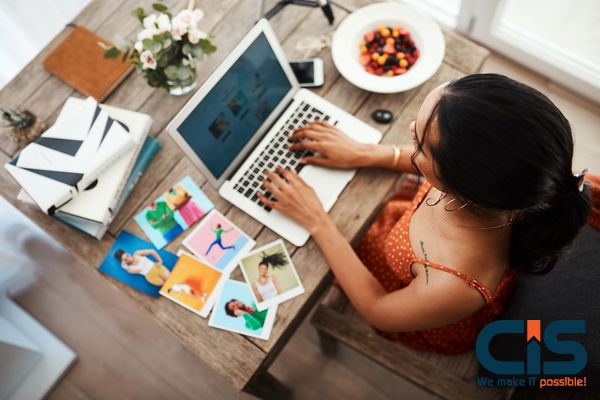
Predictive analytics offers companies an effective tool for personalizing product experiences for each customer segment based on preferences and behaviors. Discover popular personalization techniques such as content-based filters, collaborative filtering, and hybrid approaches; real-world examples demonstrate how personalizing customer interactions has led to higher customer retention and lifetime customer value increases.
Predictive analytics helps companies understand individual customer needs, market trends, and demand. With predictive models' help, businesses can better make informed decisions regarding product development and innovation by examining market data such as competitors' activities or customer feedback from previous surveys. Case studies demonstrate businesses that use predictive models successfully, creating innovative products while attaining competitive advantages or finding new markets.
Steps To Implement Predictive Analytics In Customer Intelligence
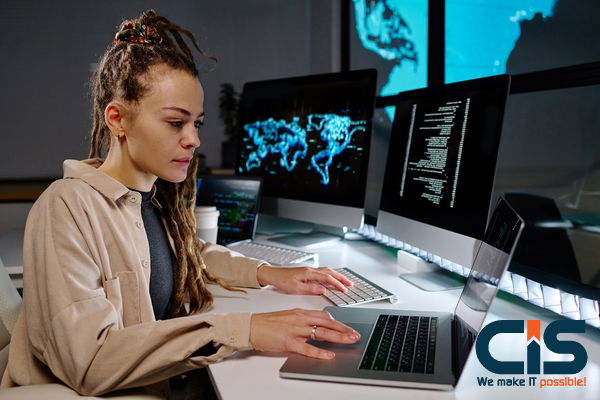
Implementing predictive analytics for customer intelligence will help you gain insight into your customers' behavior and tailor your marketing strategy. This includes:
- To implement customer intelligence, businesses must gather relevant data from different sources. These include customer data, social media data, and transactional data.
- Build Predictive Models: Businesses must use predictive analytics software to build models after gathering relevant data. The models must be designed to analyze customer behavior and predict future events.
- Validating and Testing Models - To ensure their accuracy and reliability, they must test and validate the predictive models. It involves comparing predictions from the model with actual outcomes.
- Integration of Models into Business Processes: Once the models are validated and tested, they can be integrated into business processes. It may be necessary to integrate predictive marketing tools into CRM systems or use these tools to create more effective marketing campaigns.
- Predictive models must be constantly monitored and updated to ensure accuracy and effectiveness. It is essential to analyze new data and update the models when necessary.
Predictive Customer Analytics: Benefits
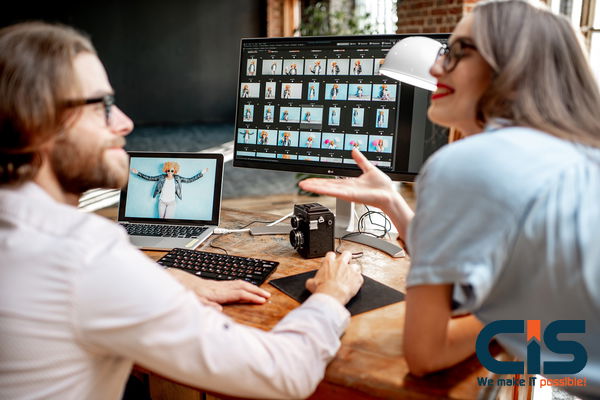
Predictive customer analytics can assist in many areas of SaaS business operations. Stay abreast of market trends quickly by reacting precisely when necessary - discover how predictive customer analytics could aid your SaaS firm!
Predict Future Customer Events By Understanding The Behavior Of Customers
Investigate and analyze customer data to detect trends. By conducting customer journey analysis, you'll discover how customers use and interact with your products - providing valuable insight into customers' needs and desires.
Building customer personas allows for a deeper insight into your clients. It can predict which users may become most essential for your business in the future, thus helping to maintain them and attract new ones.
Find High-Risk Clients Who Have Stuck In The Customer Journey
Predictive customer models can help prevent customer churn by identifying at-risk segments and helping address core causes of dissatisfaction while improving each customer's overall experience.
Use predictive analytics to target customers more likely to leave your brand to improve engagement by eliminating painful points in user journeys - and lower customer churn while simultaneously improving retention rates.
Customer Satisfaction And Loyalty Can Be Increased
Use predictive analytics to increase customer loyalty and satisfaction using real-time analytics. Real-time tracking lets you pinpoint their main drivers so they can be tracked efficiently.
Your data can help you pinpoint which parts of your business are successful and which aren't, helping you prioritize strengths and adjust accordingly.
Combining predictive analytics with customer surveys like CSAT and NPS will further your ability to understand customer needs and desires.
The Downsides Of Predictive Analytics
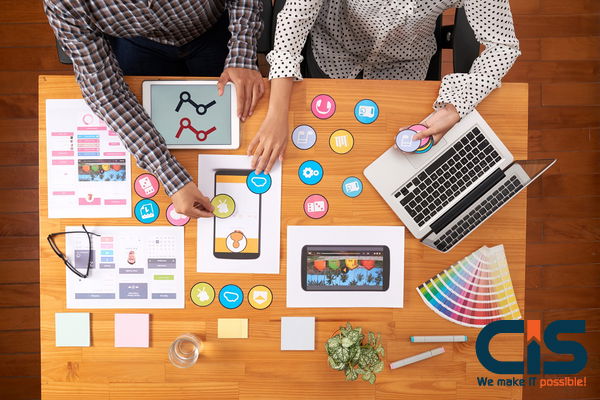
SaaS companies that want a deeper insight into customer behavior will find predictive analytics beneficial, although there may be drawbacks that should be kept in mind.
One of the greatest drawbacks to predictive analytics lies in its inability to accurately forecast complex human behaviors, with algorithms used unable to capture all factors influencing people's actions - emotions and motivations alike.
Predictive analytics software requires regular data updates; otherwise, it won't generate accurate predictions. Accurate predictions cannot be generated using predictive analytics software without an established process for collecting and updating this information regularly.
Companies using predictive analytics often assume they will have enough data available to them to generate valuable insights from predictive analyses. When data sets are incomplete or missing, their results become unreliable, and insight may become clouded or even obscured altogether; such incomplete sets also increase risks as decisions based on them may prove less successful in providing decisive answers.
The accuracy of data collection can also be an issue for companies. They utilize surveys to collect this information, yet companies know not all customers provide accurate or honest answers in surveys; some people may simply avoid providing honest responses out of fear they might give false answers that lead to poor business decisions. Data can also be misused inaccurately, leading to incorrect predictions and potentially poor decisions about future business endeavors.
What Are The Benefits Of Predictive Analytics For Customer Service?
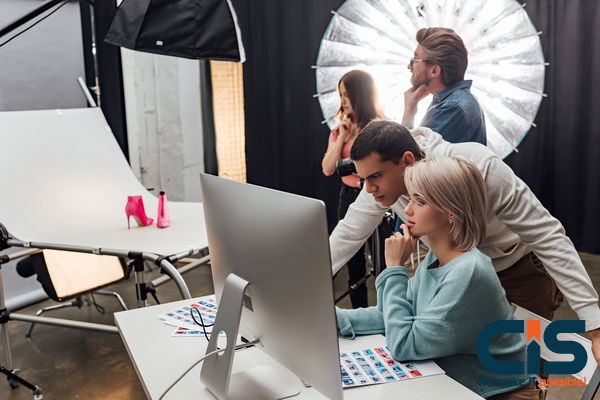
Predictive analysis involves collecting and using data to predict future events.
There are various approaches you can use predictive analytics for better customer service and the overall customer experience. In this blog, we explore five real-life examples where predictive analytics have successfully enhanced both.
Customer service has emerged as a crucial differentiator in today's hyper-competitive business environment. To provide optimal services to customers, one must understand their wants and needs first - but data collection and analysis may prove daunting when faced with large volumes of information.
Predictive analytics is a handy way of improving customer service by anticipating and fulfilling customer needs swiftly and accurately.
Read More: How AI Is Improving Predictive Analytics in Healthcare
Five Ways To Use Predictive Analytics In Customer Support
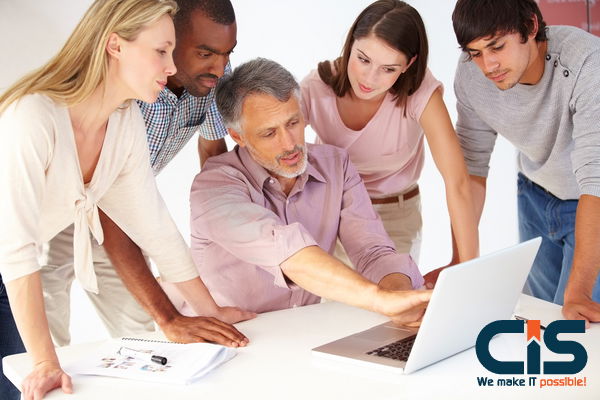
Identification Of Potential Problems
Predictive analytics in customer service can help identify issues by analyzing various data sources. Some examples include:
- How to collect customer feedback through a survey
- Re-examining service tickets to find complaints
- Monitor social media interaction
Predictive models can anticipate issues customers might experience. They can foresee defects in products, shipping delays, and service interruptions to prepare customers appropriately.
Imagine, for instance, one of your customers posts online about an order being late; predictive analytics could identify other customers experiencing similar difficulties and provide proactive resolution by communicating directly with those affected or issuing a statement.
Use Case:
Coca-Cola's Predictive Analytics Helps Identify Issues
Coca-Cola, the beverage giant, uses predictive analytics to detect potential disruptions in its supply chains that may impact production and distribution. Coca-Cola uses predictive analytics to identify potential supply chain disruptions that could impact its production and distribution processes.
Scale Personalization
By analyzing data about 24/7 customer services, predictive analytics can help personalize their experience. This is done by getting insights into each customer's preferences, behavior, and needs. Businesses can personalize experiences by understanding their customers.
Companies can, for example, use predictive analytics by analyzing a customer's past purchases and combining it with the current behavior of that shopper to predict their needs and provide unique product suggestions.
The recommendations may be given in several different ways.
- AI-powered bots can be programmed so that they initiate conversations with potential buyers. The chatbots will then display a particular item or the whole catalog. They can also offer to connect customers to product specialists.
- AI-backed agents are also available or can be the first to contact you. Instead of asking them to memorize the product details for a whole catalog, AI will help agents quickly find shoppers looking for perfect products based on previous and current shopping histories.
Use Case:
Netflix's Predictive Analytics Delivers Personalization On A Large Scale
Netflix's algorithm is one of the best-known examples. Netflix analyzes the viewing data of its users, searching for patterns in genres, actors, and directors. Then, it uses this information to find and recommend shows and films you might enjoy. The company's success is mainly due to its practice of mass personalization.
Chatbot Accuracy Can Be Improved
Chatbots can be trained to respond more accurately and personally using predictive analytics. Businesses can analyze customer feedback and inquiries to identify common themes and questions and evaluate the effectiveness of answers. This leads to a better customer experience.
This can be achieved at a fundamental level by giving a brief survey to users after each chatbot interaction. The business can see the performance of its chatbot and make improvements. Leading customer support solutions can provide chatbot suggestions based on the questions that have been received. You can then improve the experience of your chatbot and extend its capabilities.
Use Case:
Giesswein's Chatbot Uses Predictive Analytics For Training And Improving Its Performance
A European footwear and apparel company, uses its chatbot today to help with customer service. The program could not handle more than 30% of the incoming inquiries when first launched because they did not previously have a chatbot. After two weeks, the number of questions handled by this program increased to 71%. Then it jumped again to 89% after three months.
Giesswein's increased sales, customer satisfaction, and loyalty result from better customer responses.
Optimizing Resource Allocation
By analyzing data on customer demand and capacity, predictive analytics optimizes resource allocation for customer service. Businesses can better manage their agents to reduce wait times and meet demand.
For example, businesses can use predictive analytics to predict and identify high- or low-demand periods. This information can increase resources to provide quick service during busy hours and seasons or reduce them during slow times.
Use Case:
Delta Air Lines Optimizes Customer Service Resources Using Predictive Analytics
Delta Air Lines utilizes predictive analytics to predict customer demand. This is based on flight schedules, historical trends, and special events. These data can be used to predict how many agents will be needed for customer service at any given moment. Delta adjusts its staffing to ensure enough agents are on hand to answer customer questions during busy periods and avoids overstaffing in periods of low demand.
Predicting Customer Behavior
Predictive analytics allows you to analyze customer data and forecast future behaviors, such as whether a person is more likely to purchase a product or move on to another competitor.
It can first identify customers with high value and then predict the potential lifetime value of those clients to a company. Companies can identify customers likely to produce the highest revenue by analyzing their transaction histories, purchasing behavior, and feedback. This information can be used to determine the most important customers and offer exclusive offers or the same agent each time.
Predictive analytics can be used to analyze the data of a client's browsing or transactional behavior. Companies can use the information to re-engage the customer.
Use Predictive Analytics To Improve The Customer Experience And Retain Current Customers
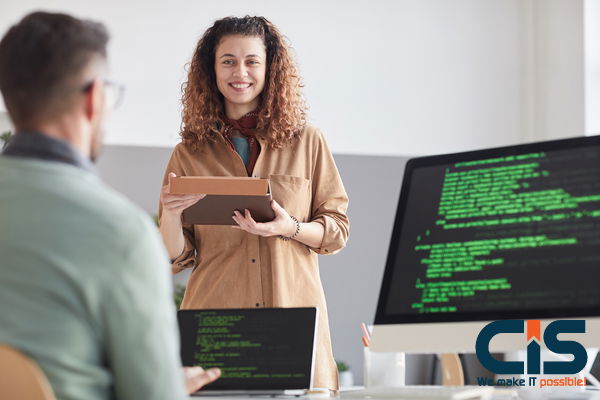
Predictive data analytics can boost customer satisfaction and achieve the best results in customer retention. How can you do this?
Personalized In-App Experiences For The Right Users At The Right Moment
By triggering personalized customer experiences in the app, you can get more out of your predictive analytics. You can increase customer engagement and drive revenue for your business. Predictive customer analytics can help you predict the future behaviors of customers by tracking their in-app activity. Using this data will help trigger personalized experiences in your app for users. When you give your users relevant and contextual experiences, they will feel valued.
It can be used for many purposes, including increasing engagement, encouraging upsells, promoting cross-sells, gathering feedback, or introducing new features. Customers are more inclined to take advantage of these opportunities when they receive personalized experiences.
You can also create a seamless, intuitive user experience using predictive data analytics.
You Can Adapt Your Messaging In The App Based On What Customers Want And Need
You can improve the messaging in your app by using predictive data analytics. You can develop messaging tailored to your customers' needs by tracking their behavior and preferences. You can predict what your customers will do in the future with predictive analytics. It is possible to predict which features will be popular with your customers and which might not.
Then you can customize your messaging in the app. For example, one message type for popular features may differ. Predictive data can be used to improve in-app marketing campaigns. Predictive data can also be used to develop in-app campaigns which are targeted and likely to resonate with each customer. You can use predictive customer data to determine the optimal time for sending promotional offers or messages.
Avoid Customer Loss By Reaching Out To Customers At Risk
Predictive customer analytics can be used to predict churn better. You can identify high-risk customers using customer feedback and in-app engagement data.
You can prevent them from leaving your business by proactively re-engaging them. Offer incentives and personalized assistance, like exclusive discounts or promotions. You can retain customers by offering them incentives and personalized help before it is too late.
Future Of Predictive Analytics In Customer Intelligence
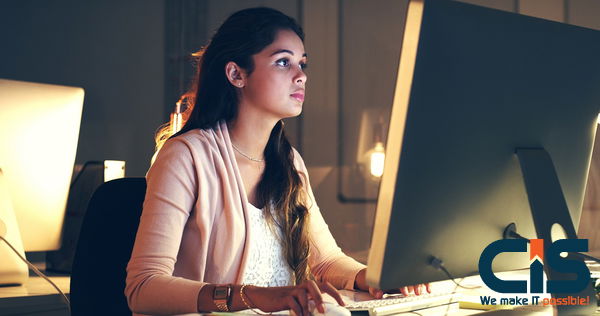
Machine learning and artificial Intelligence will revolutionize this field in the future. The need for software and tools that perform predictive analytics will continue to increase as businesses collect and analyze large volumes of data. Businesses will be pressured to use customer data responsibly, openly, and transparently.
Predictive Analytics plays a vital role in the customer intelligence process. It helps businesses gain insights into customer preferences and behavior. Businesses can gain an advantage by using predictive analytics. This includes identifying and analyzing potential customers, anticipating their needs, customizing customer experiences, and keeping customers.
Businesses must be mindful of the limitations and challenges of predictive analytics. These include data quantity and quality, reliance on models that are too predictive, and ethical issues. Businesses can improve their customer intelligence and increase profitability by considering these factors and using their power.
Wrapping Up
SaaS companies can use predictive customer analytics to gain better customer data insight and predict future behavior. These advantages allow you to improve products and services by anticipating customer needs.