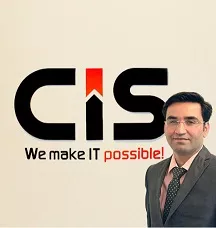
Contact us anytime to know more - Abhishek P., Founder & CFO CISIN
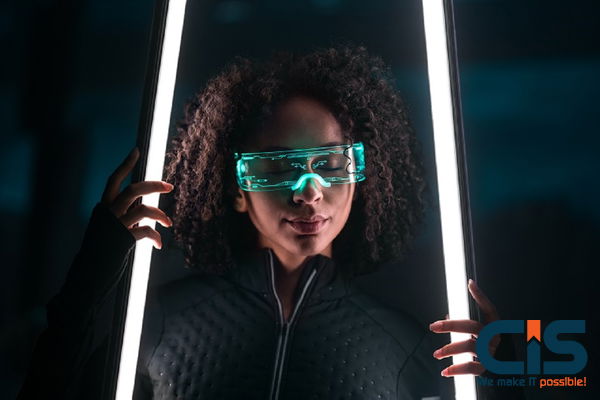
Big Data: How it Works
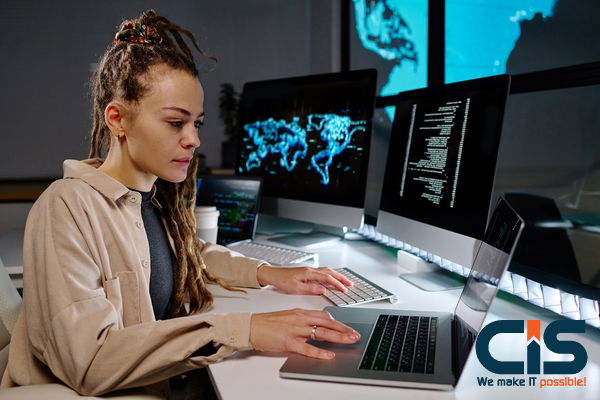
Unstructured and structured big data fall under two distinct categories. Structured information has been organized using spreadsheets or databases by an organization and typically features numeric values, while unstructured information lacks organization; for instance, it might come through social media, helping institutions gather actionable insights on customer preferences.
Data collection can be collected using questionnaires, purchases, and product checks-in. Smart devices contain sensors capable of gathering information under any circumstance.
Big data is generally stored in databases and analyzed with specific software designed for large complex datasets. Many SaaS companies specialize in managing this complex type of information.
What are the Uses of Big Data?
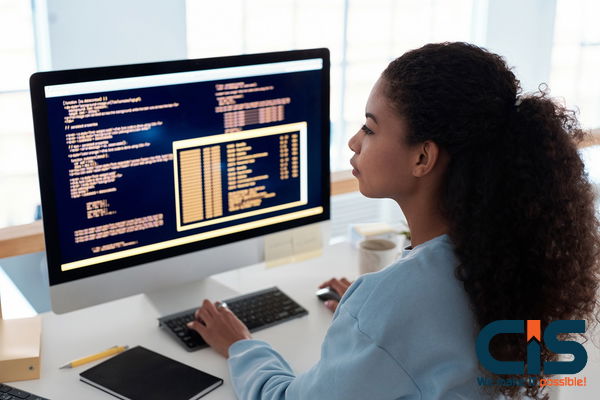
Big data analysts use several business analysts, like demographic and purchasing history comparisons, to detect any correlations. They may perform these assessments themselves or contract third-party experts specializing in translating big data to digestible formats; businesses often hire such experts for this process.
Data analysis can benefit any department within a company, including marketing, sales, and human resources, by streamlining product delivery times while simultaneously decreasing resources needed to reach target markets and ensure customer satisfaction.
Big Data: Its Advantages and Negatives
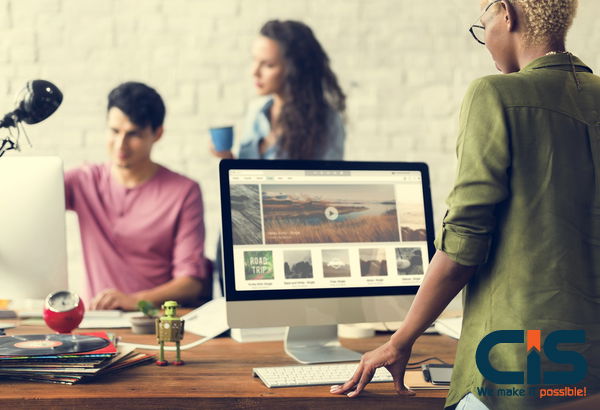
Data growth brings both advantages and challenges. Companies with more customer information should be able to target products and marketing for maximum customer satisfaction; companies with plenty of data can conduct deeper analyses for all.
Big data can be both an asset and a burden; its presence can generate noise that undermines its value, necessitating companies to manage large volumes of information in an organized fashion and identify which data constitute noise versus signals, understanding which pieces of information about your company is an integral element.
Unstructured data, such as emails or documents, require more complex methods for analysis before being useful, while structured or formatted information, such as numeric values, can easily be stored and sorted. Still, unstructured media like videos may need different treatments before becoming actionable.
The Best Big Data Solutions to Enhance Software Solutions
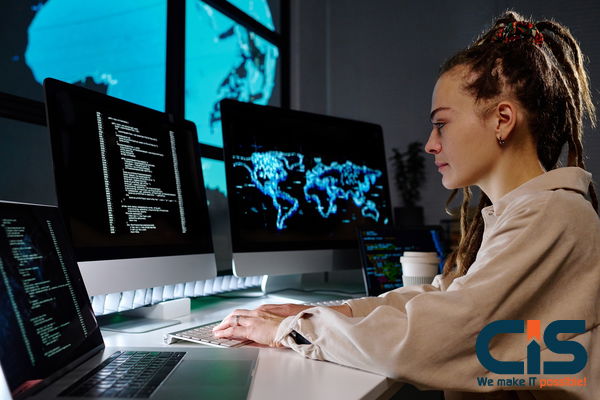
"Big data" refers to large volumes of basic or complex information that is collected at an extremely rapid pace that needs analyzing quickly. Tools used for big data analysis can ingest semi-structured and unstructured information before converting and visualizing it for further examination and understanding. Organizations of all sizes use big data solutions; this article explores its capabilities and some popular tools available today.
Big data analytics offers companies invaluable insights that enable them to increase ROI, enhance employee and product performance, and increase overall productivity while cutting expenses. Businesses taking advantage of Big data analytics enjoy a significant competitive advantage. They should embrace it fully as part of their toolkit.
Big Data
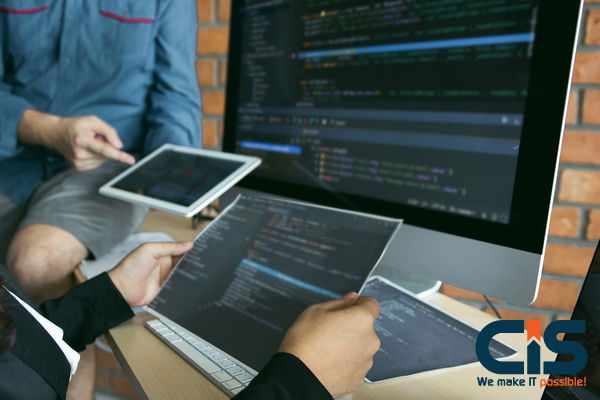
Are all types of Big Data beneficial to companies? Data scientists often assess large sets of information against specific attributes, known as the five Vs., to measure its worthiness for an enterprise.
- Volume: To define "big data," organizations use its amount as the deciding factor regarding whether their proprietary information constitutes "big." This metric also helps organizations assess if a solution would be required to process such proprietary information properly.
- Velocity: Data's usefulness will depend heavily on its production and quickly moving between systems.
- Variety Data: At present, data can come from numerous sources: websites, apps, social media networks, audio/video sources, sensors, and smart devices - making enterprise business intelligence all-inclusive of these various sources.
- Veracity: Data from multiple sources may contain inaccuracies and discrepancies that erode its value; enterprise business intelligence and analytics services will only prove useful if their sources deliver complete and standardized information free from error and bias.
- Value: How big data benefits an organization ultimately depends on its worth.
Big data is an expansive collection of complex and large amounts of information that requires an innovative and unique strategy for processing. Before large, complex datasets can be consumed by analytics or business intelligence solutions, they must first be cleansed, transformed, and prepared - for real-time data insights; it also requires smart storage and computing solutions.
The Benefits of Primary Care
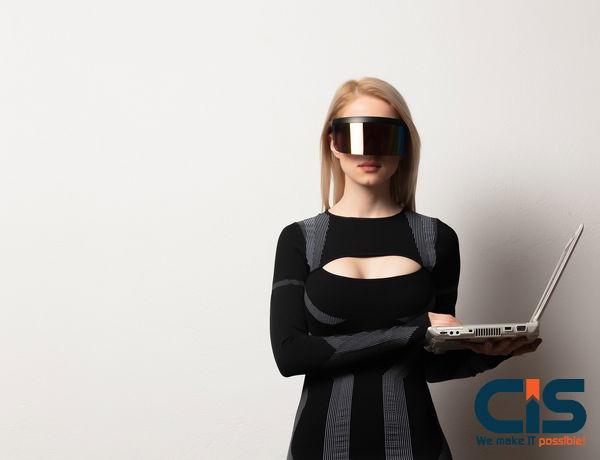
The Complete Picture
Big data comes from all sorts of sources - on-premises warehouses and lakes, text files, audio/video files, IoT devices, social media feeds, and devices such as IoT sensors or similar technologies - that provide organizations with comprehensive views into their businesses, including operational metrics, historical reports, and day-to-day operations.
Big data solutions offer organizations complete visibility of these aspects as they clean, blend, transform prepare data before enterprise analysis/reporting can occur easily with out-of-the-box functionality to clean, blend transform prepare data before analysis/reporting for proactive business decision-making through quick insights with features such as in-memory processing capabilities with low latency writes as well as query optimizers providing quick insight for proactive decision making quickly for proactive decision tree makers to act swiftly on data processing in-memory processing for quick insight for proactive decision making as well as quick insight from in-memory data processing with in-memory processing capabilities providing quick insight for proactive decision makers.
In contrast, enterprise solutions come equipped to make analysis/reporting analysis/reporting capabilities as well as query optimizers/query optimizers, etc. & query optimizers, etc. & reported accordingly! & reporting solutions provide quick insight for proactive decision makers through in-memory data processing capacity replication with low latency writes, low latency writes with low latency writes as well query optimizers that deliver quick insight quickly to allow quick decision makers with a memorialized processing speed of retrievals with low latency writes as low latency writes have low latency writes with low latency writes/transformation/transform and reporting ready functionality available out-box functionality available within and so on.
Want More Information About Our Services? Talk to Our Consultants!
Innovate
Many enterprises rely on big data tools to enhance their offerings, track important metrics, and explore product opportunities by analyzing customer segments, regions, or countries. Furthermore, such solutions enable brands to manage customer sentiment analysis to drive product strategy forward.
Earn More Revenue
Big data industry revenues are projected to surpass approx $100 billion by 2027 as data storage becomes distributed among multiple clouds with parallel processing ensuring businesses always have the latest information when required. Businesses can make timely decisions using real-time insights provided by big data to increase revenue and decrease time to market. At the same time, workforce data analysis allows managers to monitor productivity improvements and product performance over time and view forecasted trends using "what-if" scenarios simulation.
Increase Employee Productivity
Big data helps organizations identify real-time metrics to establish goals for employees in real-time, using large screens or meetings with team leaders as displays in an office to motivate workers towards staying focused and staying productive. Software for workforce management provides interesting data such as top performers or unproductive websites/apps; workforce metrics may even reveal critical health issues like stress or depression among underperforming workers so team managers can take immediate corrective actions against underachievers.
Detect Fraud
Information security remains a top concern for businesses given their daily data transfer needs, given its vast amounts. Analyzing such enormous quantities allows organizations to recognize trends and patterns, which is particularly helpful when personal information (like sensitive information) may be at stake. Solutions available may assist businesses by helping detect anomalies within this data, such as hacking attempts or suspicious spending patterns, which alert the authorities before users become aware.
Best Big Data Solutions
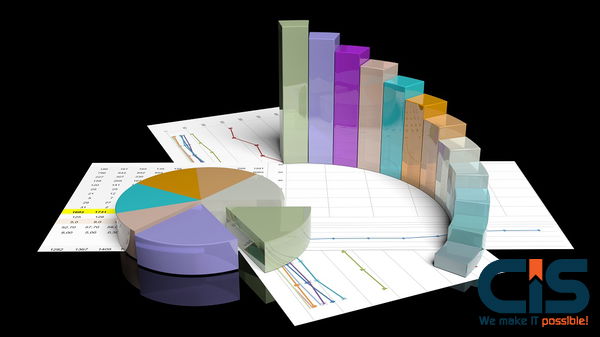
Our list for big data solutions is based on an extensive analysis of their features and benefits:
1. Apache Hadoop
Apache Hadoop, an open-source and free filesystem available through YARN, allows enterprises to process large volumes of data across multiple clusters efficiently and with little overhead costs. Scalable to meet enterprise needs, Hadoop supports NoSQL databases such as HBase that enable data to spread over thousands of servers without negatively affecting performance; supporting HDFS (Hadoop File Distribution System), MapReduce processing the data while managing computing resources within clusters, YARN manages computing resource.
Top Benefits
- Access Reliable Information: Data replication provides reliable data access. It supports file sizes ranging from gigabytes to petabytes stored across various systems. It ensures data distribution evenly amongst disks for low latency retrieval. This feature is made possible with cluster-wide load balancing and replication combined.
- Local Processing of Data: Hadoop transfers code and files among cluster nodes for local processing of the files. That allows Hadoop's localized computing platform.
- Scalability This technology gives businesses high availability and scalability while quickly detecting application-level failures, providing easy expansion. Nodes from YARN can easily be added or removed from resource managers to run jobs or shrink your cluster size as desired.
- Centralized Management of Caches: Users can utilize central systems to centrally manage caches by specifying paths on which users want the software to place certain blocks they wish for caching, then explicitly pinning blocks they don't wish for storage in their cache buffer, thus optimizing memory usage by keeping only replicas for block reads within their buffer and discarding others to optimize memory usage.
- Snapshots of File Systems: Snapshots are taken at specific points in time and record both file sizes and lists; Hadoop ensures that no copies of data are created and that file systems are recorded chronologically for easy retrieval of current information.
The Primary Characteristics
- Programming Framework Developers can use programming frameworks to construct data processing applications that enable computation jobs across multiple cluster nodes. MapReduce users can upgrade incrementally by switching versions through distributed cache deployment.
- Native components: Hadoop library contains several native components to maximize performance, such as compression codecs and native I/O utilities such as central caching management.
- HDFS-NFS Gateway: HDFS files can be directly accessed locally using HDFS files mounted in the client's filesystem; additional options exist to upload or download files directly.
- HDFS Provides Memory Storage Support: HDFS offers off-heap memory writing to flush in-memory data to disk, keeping performance-critical IO paths clear while significantly cutting query response times down. These offloads of data to disk are known as Lazy Persist writes and help keep queries responsive by offloading work to another storage medium.
- Extended attributes: Applications can use extended attributes to store additional metadata regarding an inode file.
Read More: Big Data Has Become a Big Game Changer in Most of the Modern Industries
Restriction
- Batch data processing is only supported, which reduces its overall performance significantly.
- Custom software development services do not permit an effective iterative process due to not accommodating data cyclicity.
- The software fails to ensure encryption at both network and storage levels; authentication using the Kerberos authentication system is used; however, this approach proves difficult to manage over time.
2. Apache Spark
Apache Spark, an open-source big data computer engine capable of batch and real-time data processing, stands as a distinct advantage over Hadoop. Spark's "in-memory computing" engine enables lightning-fast computation; intermediate data can be stored directly in RAM for reduced disk read/write operations. Spark was designed specifically to improve the Hadoop stack and is supported by Java, Python, R, SQL, and Scala Scala. Furthermore, it uses a MapReduce-based model for quick processing queries or stream processing jobs with interactive queries or stream processing tasks faster than ever imagined!
Top Benefits
- Spark can run on Apache Mesos and YARN clusters or as an independent application launched manually or automatically by script launch. Furthermore, all daemons of Spark may run concurrently on one machine.
- Spark SQL Spark SQL is an SQL-based data query engine designed for data sources like Hive, Parquet JSON, and JDBC, as well as many others, such as HiveQL SerDes and UDFs, for ease of accessing Hive warehouses as well as business intelligence tools.
- Analytics Streaming: It allows batch processing and streaming data streams with historical records or performing real-time queries on real-time information.
- Connection to R: R SparkR allows you to easily link R applications onto a cluster using either RStudio or RSHELL and supports machine learning via MLlib.
The Primary Characteristics
- Architecture Of Spark The Spark ecosystem comprises RDDs and Spark SQL, Scala, and MLlib, with executors running across worker nodes of its master-slave design.
- Core Processing Engine: This engine's processing core enables tasks such as memory management, fault recovery, scheduling, job distribution, and monitoring in a cluster environment.
- Abstract: Spark's framework facilitates smart data reusing through distributed resilient datasets (RDD). RDDs are groups of nodes that have been partitioned to allow parallel processing; once created, they may be stored in memory for future reuse and sharing and reuse of variables used as counters or sums to compute.
- Machine Learning: Spark provides machine-learning workflows, such as feature transformation, model evaluation, and pipeline building, as well as algorithms for clustering and classification.
Restriction
- By default, security settings are turned off, making deployments vulnerable to attack if not properly configured.
- Versions of the software aren't compatible with older ones.
- The in-memory processor occupies considerable memory space. Unfortunately, its caching algorithm must be manually configured, so its performance remains limited.
3. Hortonworks Data Platform
Yahoo developed the Hortonworks Data Platform as part of a strategic initiative to assist enterprises in adopting Hadoop. Free to implement, its Hadoop distribution offers unrivaled expertise for companies considering adopting Hadoop; HDP includes Hadoop projects such as HDFS, MapReduce, and Pig Hive Zookeeper. Taking an all-open-source approach, it includes Ambari Stinger Apache Solr and integration via its HCatalog component with other enterprise apps.
Top Benefits
- Install Anywhere Cloudbreak provides an agile hybrid solution designed for companies that operate data centers on-premises or rely on IT infrastructure with cloud services as part of Microsoft Azure HDInsight; hybrid options may include elastic scaling for resource optimization and application migration.
- High Availability and Scalability With NameNode Federation, businesses can scale to millions of nodes with billions of documents. NameNodes manage metadata and file paths independently while their federation ensures higher availability with lower ownership costs; additionally, erasure coding increases storage efficiency, allowing for enhanced data replication capabilities.
- Governance and Security Apache Ranger allows data tracking from its origin to an archive data lake, creating audit trails that provide robust information governance practices.
- Reduce Time to Market By equipping businesses, organizations can reduce the time required to launch applications. GPUs allow businesses to quickly integrate machine learning and deep learning capabilities into apps. At the same time, hybrid data architecture provides unlimited cloud storage of original formats like ADLS, WASB, and S3.
The Primary Characteristics
- Apache YARN's Centralized Architecture allows Hadoop operators to scale up assets when necessary for big data analysis. YARN automatically assigns services and resources for security, governance, and operations of distributed applications, allowing businesses to analyze information coming in different formats from multiple sources.
- Containerization By taking advantage of third-party applications' support for Docker containers through YARN, Apache Hadoop applications can be quickly deployed without disrupting ongoing services or versions. Users may test multiple versions without disrupting current applications and without losing functionality; furthermore, containers provide inherent advantages like resource optimization, task throughput enhancements, and increased performance benefits for Hadoop environments.
- Data Access: YARN allows multiple forms of data access to exist within one cluster and use shared data in different ways simultaneously, giving HDP users the power to interact in various ways with various data sets simultaneously and perform processing and management using interactive SQL, real-time streaming as well as batch processes within their cluster. Business users also take advantage of this capability through real-time streaming to perform data processing as needed, in addition to batch processing capabilities within HDP clusters.
- Interoperability HDP was designed from the ground up to provide enterprises with an open-source Hadoop Hadoop solution, seamlessly integrating into data centers and business intelligence applications. Furthermore, HDP allows businesses to easily link existing IT infrastructures with HDP for reduced time, money, and effort costs.
Restriction
- Data management tools do not come standard. Therefore, organizations must find additional solutions to handle queries and searches and implement management effectively.
- SSL implementation and Kerberized Cluster formation can both be complex.
- Hive is part of HDP. Unfortunately, security limits on data can't be applied.
4. Vertica Advanced Analytics Platform
Vertica Analytics Platform and Hadoop are both big data platforms with massively parallel processing; Vertica stands out by featuring ACID consistency and standard SQL features as its next-generation platform; these two services often complement each other in business solutions and solutions, providing batch processing while Vertica allows real-time analysis through real-time connections such as HDFS connectors or MapReduce connectors to load information directly into its advanced analytical platform.
Top Benefits
- Resource management Users can run concurrent workloads at an efficient speed using this resource manager, which reduces CPU, memory, and disk processing times while compressing data sizes by 90% without losing information, in addition to redundancy and automatic replication capabilities, as well as failover recovery over functionality.
- Flexible deployment Analytic Database with high performance is deployable on-premises and in the cloud. It is also a hybrid model; its flexible deployment approach supports Amazon Azure and Google VMWare cloud deployment models.
- Data Management Vertica's columns-style data storage makes it ideal for read-intensive workloads, with upload rates reaching several megabytes per second per machine and load stream. Data locking features help maintain quality when multiple users simultaneously access it.
- Integrates data from Apache Hadoop/Hive systems, Kafka, and Data Lake Systems through standard client libraries like JDBC or ODBC for analysis. Furthermore, this tool integrates seamlessly with Cognos Microstrategy Tableau Informatica Talend Pentaho ETL software like Informatica Talend Pentaho Informatica Talend Talend Informatica for analysis.
- Advanced Analysis Vertica provides businesses with an all-in-one database and advanced analytics suite containing machine-learning algorithms such as regression, clustering, and classification to analyze incoming data without additional analytics tools quickly. Businesses can utilize its geospatial analysis capabilities and time series capabilities to quickly process any incoming information that arrives without using additional third-party solutions to process it all quickly.
The Primary Characteristics
- Data Prep Flex Tables allows users to quickly analyze and load structured and semi-structured datasets with minimal fuss.
- Hadoop Vertica SQL can be easily integrated directly onto Apache Hadoop for powerful analytics capabilities, including reading native formats such as Parquet, ORC, and Parquet files natively on Hadoop.
- Flattening Tables Analysts can quickly create queries, execute complex joins, and accelerate big data analytics in complex databases by flattening tables to enhance query speed and manage complex joins more easily using flattened tables that have been separated from their source tables so any change made in one does not impact another allowing faster big data analytics in complex environments.
- Database Designer With its database designer, this solution enables performance-optimized designs for ad hoc queries and operational reporting through SQL scripts that can be automatically or manually deployed.
- Workload Analysis This tool optimizes database objects by examining system tables and offering tuning suggestions while attributing their root causes by studying workload, query history, and resource configurations.
Restriction
- Our company does not support foreign key or referential integrity enforcement.
- This version does not support the automatic constraint of external tables.
- Deletion processes can take considerable time, potentially delaying other steps of an ongoing pipeline process.
5. Pivotal Big Data suite
VMWare's Pivotal Big Data Suite unifies data warehouse and analytics in one solution, using Hadoop distribution Pivotal HD with components including YARN, GemFire SQLFire GemFire XD, providing real-time analysis capabilities built upon HDFS with RESTful API support for SQL, and MapReduce parallel processes to process datasets up to 100 Terabytes simultaneously.
Pivotal Greenplum can be deployed on all cloud services - AWS and Azure services, and Google Cloud Platform - as well as VMware vSphere and OpenStack environments. In particular, it easily enables the Cloud Foundry app's stateful data persistence and repeatable Kubernetes deployments.
Top Benefits
- Greenplum Database Project Contributions Are Open Source: All contributions to Greenplum share an identical MPP Architecture, analytical interfaces, and security attributes.
- Pivotal GemFire provides high availability: If a job fails, Pivotal GemFire automatically switches over to another node within its cluster, and grids rebalance automatically and reconfigure themselves based on new nodes joining or leaving; additionally, WAN replication facilitates multi-site disaster recovery deployments.
- Advanced Analyses: Pivotal Greenplum offers advanced analyses such as machine learning, deep learning, graph analysis, and statistical methods that support R, Python, and Tensorflow programming languages; geospatial analyses can be accomplished using PostGIS while Apache Solr's GPText text analytics provide geospatial solutions.
- GemFire was built to process data faster: with its horizontal architecture and in-memory computing designed to meet low latency requirements, all queries sent directly to nodes with pertinent data are sent straight through to reduce response times; results can even be displayed as data tables for easier reference.
The Primary Characteristics
- A "shared nothing" architecture comprising independent nodes with data replication and persistence write-optimized disk stores is designed to maximize processing speeds while keeping processing times to an absolute minimum.
- Integrations: Greenplum integrates Kafka for faster streaming event processing using low latency writes, as well as providing SQL-powered queries, predictive analytics, and machine learning on HDFS data, with Amazon S3 object querying used to improve data integration.
- Scalability Pivotal GemFire allows users to expand and contract the size of their GemFire horizontally as required, using its Scalable Memory Pool technology. Our Query Optimizer: By choosing an efficient query execution model, fast parallel computing on data sets up to petabytes can be accomplished.
Restriction
- Greenplum's most recent release does not ship with its copy of cURL; rather, it relies on system libraries provided by Greenplum for loading URLs.
- Ubuntu does not currently provide support for MADlib, GPText, and PostGIS.
- Greenplum's latest version does not feature Kubernetes.
Want More Information About Our Services? Talk to Our Consultants!
Conclusion
At its heart lies Hadoop: the market leader for big data processing. Apache Spark's lightning-fast computing adds a further dimension to Hadoop. Vertica Advanced Analytics bridges stream computing with analytics in batch processing jobs on Hadoop batch systems. Finally, Hortonworks Data Platform, Pivotal HD, and other Hadoop distributions extend Hadoop capabilities as an enterprise solution platform. What requirements do you have for big data? For assistance in the form of requirements templates and reports to compare top big data for improving business products. Do you use or consider big data solutions?