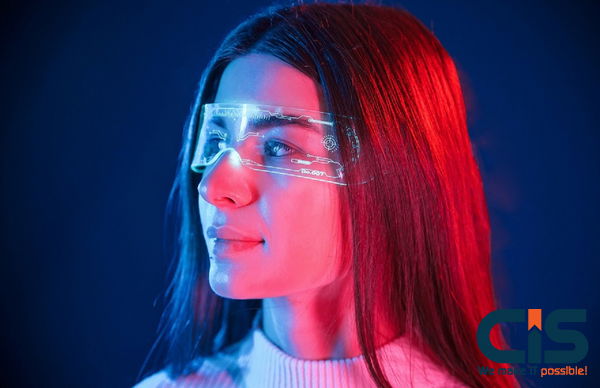
What is Machine Learning?
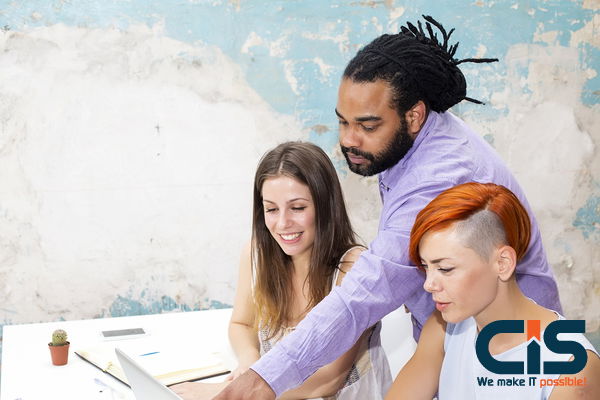
Machine learning is a branch of science that studies and develops AI. The focus is creating systems that can learn and grow through data analysis. Artificial Intelligence (AI) is closely related to Machine Learning (ML). AI machine learning applications can learn from data collected and increase accuracy without manual programming.
Machine learning: algorithms use patterns found in large amounts of data to make predictions and make data-based decisions. The more data ML processes, the better the algorithms and the more accurate the predictions and decisions they make.
Three main types of machine learning are defined today:
- Supervised Machine Learning: The algorithm is trained using predefined examples. The ML uses it to learn the correct conclusions when given new data.
- Unsupervised Machine Learning: The algorithm is trained using data without predefined rules. It must identify patterns and relationships to make future predictions.
- Reinforced Machine Learning: the technology is taught to create a series of decisions. The agent is trained to accomplish the goal within an uncertain, potentially complex environment.
How AI and ML Works
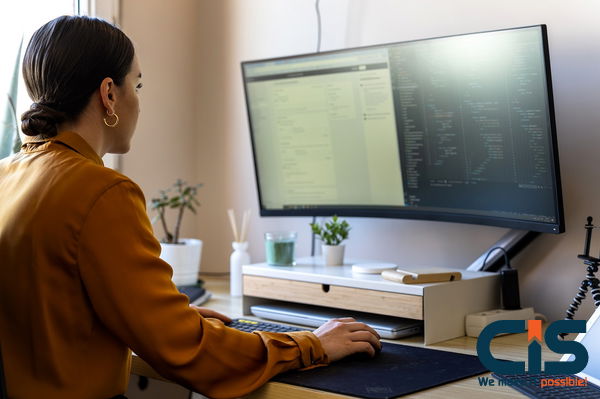
AI is the pursuit of intelligent building systems that can learn and reason like humans. This is "artificial intelligence" - Intelligence that doesn't come from nature. Machine learning is a branch of AI focusing on finding patterns within massive data sets. ML algorithms are used in various applications, from Netflix recommendations to diagnosis.
Artificial general Intelligence (AGI), or AGI, is the next step in AI. First, you must build a general-purpose AI. AGI features include the ability to learn unsupervised or even self-supervised and transfer learning. Deep learning is ML's most advanced area.
AI and Machine Learning in Mobile App Development
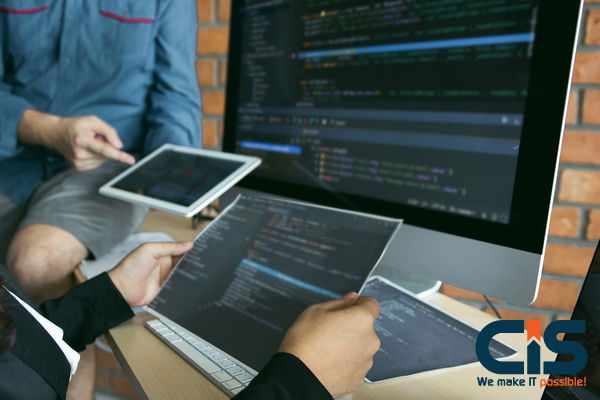
Artificial Intelligence and machine learning have taken over all tech-focused mobile app development areas. The demand for apps with AI and ML capabilities has grown as corporations and organizations show increasing interest in these technologies. Analysis suggests that AI will open up many opportunities for all types of businesses. AI and ML can offer various benefits, from improving product recommendations for an online shop to gaining deep insights into the business.
Medium-sized businesses and large corporations should invest in new technologies to gain a competitive advantage. AI and machine learning will become more critical in the future as mobile apps take over everything from healthcare to grocery delivery. This article aims to provide an overview of the mobile app trends crucial to business today. Here are some of AI and ML's most notable app development trends.
The Rise of Facial Recognition
In the past few years, facial recognition has seen an impressive increase. The topic has been in the news all year for good and bad reasons. Face recognition, for example, has improved data security because it reduces the risk of identity theft. Authoritarian countries like China use technology to track people down.
The positives of facial identification outweigh the negatives. Financial services companies, for example, rely on advanced facial recognition to prevent fraud. AI-enabled apps on mobile devices can verify that the correct person is using their mobile device or banking app. Most smartphones today have facial recognition features due to their increasing popularity.
These trends will have a significant impact on the way businesses use mobile applications. Face recognition is therefore becoming a substantial driver for next-generation app development. Fintech and dating apps are among the tech-based companies already experimenting with AI. Other services will likely adopt this technology to enhance customer experiences. Both B2B and B2C applications will use AI to upgrade their functionalities.
Voice Assistant Goes Mainstream
The voice assistant is the most popular AI-based consumer technology. Apple's Siri and Amazon's Alexa are all AI-based. These AI-based assistants are gaining popularity in both developed and emerging markets.
Voice assistants also have a growing presence in online searches. As technology advances, people prefer voice assistants to texts. Tech companies are constantly improving their voice assistants.
Apple's Siri, for example, allows you to customize your voice assistant. For instance, you could select different accents to detect, such as Indian, American, or British. Siri allows you to choose the type of voice assistant you prefer, including American female and Indian male voices, etc.
App developers are incorporating voice assistants worldwide to improve customer experience. AI-based Chatbots are one example of a technology that provides seamless customer service around the clock.
AI digital assistants took over customer service when lockdowns forced offices and call centers to close. Intelligent voice assistants have been used in industries as diverse as food service and banking to provide prompt customer support.
Search Engines Improved by Algorithms
Search engines are more important than ever as commercial and administrative activities have moved online. Search engines are improving thanks to the combination of deep machine learning and advanced web capabilities. Google and Microsoft use advanced algorithms to provide more relevant search results. These algorithms use machine learning to recognize patterns. Machine learning is the best way to engage users in mobile and web applications that rely on online searches.
Get a Free Estimation or Talk to Our Business Manager!
Machine Learning App Examples
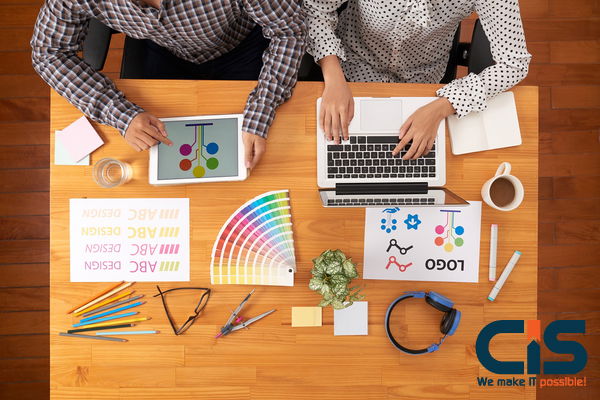
Machine learning is widely used in the development of apps today. Vast quantities of the solutions you use daily rely upon ML. Review some of the most popular machine learning implementations.
Netflix
Netflix is an excellent example of how machine learning can be used in software development. Netflix has the best content for you. It is exciting, and it matches your tastes. This is because artificial Intelligence and machine learning constantly learn about your preferences. Its robust recommendation system is based on them.
Netflix will recommend similar content to you if, for example, you watched a film yesterday. If you start watching a movie, stop, and give a negative review, the machine learning algorithms will understand that you do not like this content. They won't make similar recommendations.
Tinder
Tinder is a great example of machine learning in mobile apps. Contrary to what end users might think, the solution does not use spells to match them with their perfect partner. Machine learning analyzes how users swipe left and right and then provides more relevant suggestions based on collected data.
Snapchat
Snapchat is an excellent example of how machine learning and AI can be combined to create a powerful tool? The application uses AI, ML, and augmented reality to create a 3D mesh that can be deformed to apply filters.
This platform relies on machine learning, even though most users are unaware of it. The company uses this technology for many tasks, such as advertising, content discovery, spam moderating, etc.
There are many examples of a Machine learning app. Google Maps and Twitter are also examples of machine-learning applications. You can use them to learn how ML works and for some machine learning ideas.
Machine Learning Application Development: Industries That Can Benefit
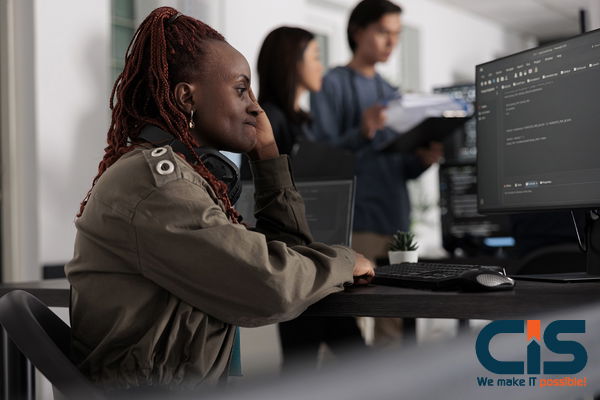
Machine learning is a solution that has slowly found its way to various industries. There are many use cases, and in this section, we'll discuss the areas where Machine learning technology is most valuable:
Transportation
The arrival of self-driving and smart cars has already changed our lives. Machine learning algorithms and artificial Intelligence are used to monitor traffic and predict change. Combining these technologies allows vehicles to drive autonomously.
Healthcare
In the healthcare sector, machine learning is used to develop critical processes such as disease discovery and treatment. These solutions analyze and collect patient data to diagnose the condition and suggest possible treatment options. Predictive health care is becoming more popular.
Finance
Finance industry data is vast and needs to be automated and analyzed to predict future data management, investments, and transactions. In the finance industry, machine learning and artificial Intelligence are used for fraud detection and prediction. Other industries use this technology for the same purpose.
Cybersecurity
Machine learning is also used in cyber security. Machine learning can be used to analyze incident reports, alerts, and other data to identify threats, improve security analysis, or even provide advice on response.
Manufacturing
Machine learning opens up new possibilities for manufacturing. Companies with assembly lines can use ML algorithms, sensors, and AI to predict maintenance needs. Implementing the technology can protect companies from unplanned downtime and production drops.
eCommerce
Machine learning is now widely used to make intelligent, data-driven predictions. This technology is already a boon to the eCommerce industry. Product recommendations tailored to the individual increase average order values and revenue.
Marketing
Machine learning can also be applied to the advertising industry. Ads can benefit from this technology by targeting users with highly personalized ads tailored to their needs. Machine learning and artificial Intelligence can be used in any industry. Automation adoption is revolutionizing our lives, sharing decisions between humans and technologies, and reducing risks of human error and delays that lead to wrong conclusions.
Read More: How to start implementing AI & ML to your existing mobile apps
A Step-By-Step Process For Building A Machine Learning App
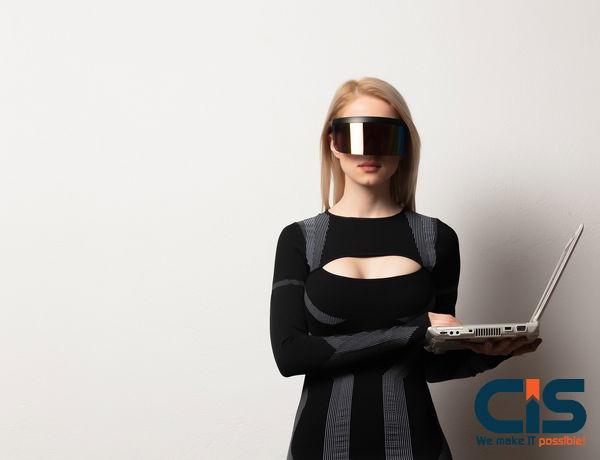
The development of machine learning applications is a series of steps. Follow the steps to create an app that uses machine learning:
- Problem-solving: How to frame a problem
- Data collection and cleaning
- Prepare data for ML application.
- Feature engineering
- Train a model
- Model accuracy: evaluate and improve
- Serve in a model in production.
Frame the Problem
You can begin developing a machine learning application by identifying your main implementation goal and the problem you want to solve with the technology. You must understand the problem and how machine learning should address it.
You can move forward by answering the following questions:
- What do you want to predict using ML? What is its primary purpose?
- What are the main features of the target?
- What data will you be using as input? Have you got it? Do you have to deal with binary classification or clustering?
- What are your expectations for improvement?
- What is the status of the feature?
- How will you measure the target feature of your product?
Remember that machine learning only recognizes patterns it has already seen. Data is, therefore, crucial to your goals.
Clean and Collect Data
Data collection is the next step after you've framed your problems. You can collect data either from your database or open data sources. You can clean the data after collecting it to remove any irrelevant inputs. This will directly impact the accuracy of the machine learning algorithms you use and future predictions.
Prepare Data for Machine Learning Application
It is essential to realize that machine learning only analyzes numbers. Convert all data to a format your ML can understand, like text or images. Create a data pipeline that consolidates data from different sources to be able to analyze it.
Feature Engineering
Sometimes, the row data does not have the specific characteristics needed to make machine learning algorithms work. You can do this by using feature engineering. This technique creates new features that combine two or more data elements in a more sensible and relevant way. Data preparation is the time-consuming component of developing a machine learning application.
Model Train the Model
Model training is the next step, improving your machine-learning algorithm's ability to analyze data. You need to divide the data in two: Training and Evaluation. You can use the second data set to see how well your model generalizes.
The algorithms will then learn to map the features and labels. Model training can be approached in many different ways. You can control the learning rate, regularization, and number of passes.
Optimize Model Accuracy By Evaluating And Evaluating
You need to assess how well the machine-learning algorithm is performing. You can use various accuracy metrics, such as under-fitting and overfitting. You can optimize your algorithms and data based on the results.
Serve in a Model in Production
The final step in developing a machine learning application is to deploy it into production. The algorithm is now ready for production. It would help to integrate the algorithm into your software to make accurate predictions and be practical. This is the most challenging part of the entire process and requires high technical expertise.
Data Privacy in Machine Learning App Development
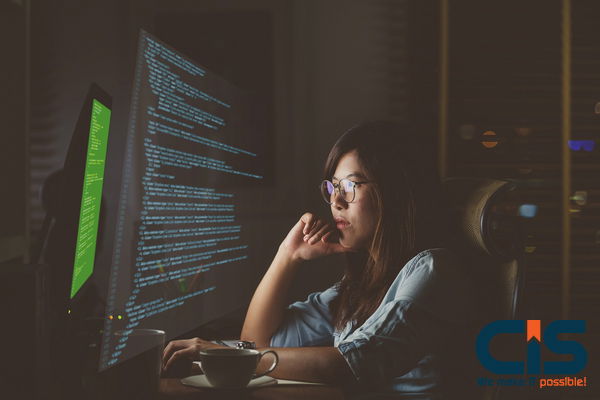
Working with large data sets is a part of machine learning. It is a challenge to create machine learning while considering data privacy. We'll look at some principles that can help you build a solution compliant with the modern requirements for data security.
Data Anonymization
Machine learning has several concerns, including the use of data that can identify a specific person. Data anonymization is one of the most critical requirements in privacy laws. You must choose the method to anonymize data when you build machine learning. When data is converted to a single number, one-way hashing is used so that the original data cannot be deduced from it.
GDPR
You will need to anonymize data that can identify an individual. General Data Protection Regulations (GDPR) clearly state that you cannot store this information without violating the regulations.
The Right To Be Forgotten
GDPR and CCPA require users to consent to the use of their data by business operations. Business models cannot use data if it does not have consent. You will need to comply with the rules of the "right to be forgotten." Users must be able to withdraw the support they have already given a company and demand that you erase all data you hold on them. It is difficult to remove data from a machine learning algorithm. It's, therefore, essential to design machine learning systems with "right to delete" requirements in mind.
The amount of data that is processed by machine learning can be overwhelming for companies who decide to develop Machine learning. Data privacy should be considered when designing machine learning algorithms to help you avoid future problems with local regulations.
A Technology Stack for Machine Learning App Development
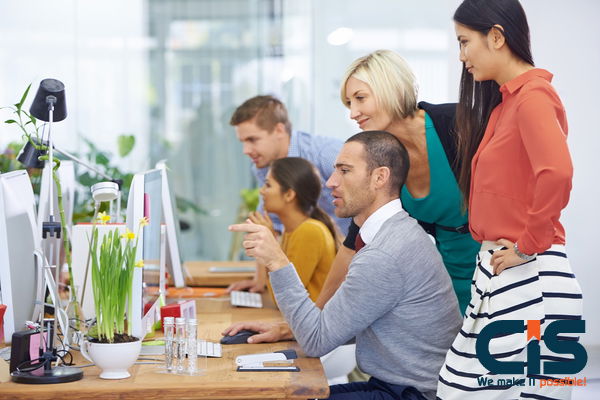
Machine learning applications can be built faster with the availability of frameworks, libraries, and APIs. It's okay to build algorithms from scratch. You can now choose from a wide range of machine-learning solutions to create powerful software that will facilitate your business goals and needs.
Review some of the most popular Machine Learning Frameworks:
TensorFlow
TensorFlow, a framework popularized by Google, is ideal for creating deep learning models. These models use artificial neuron networks to allow progressive learning and system improvement. The framework is built on a graph of computational nodes. Each node is a function that can be simple or complex.
PyTorch
PyTorch, which is directly competing with TensorFlow in terms of its capabilities to build deep learning models, offers a wide range of features. It is also part of the Torch deep-learning framework, which developers use to create deep neural networks and perform complicated computations.
Shogun
Shogun can be a good solution if you are interested in learning how to create a machine-learning app for research and educational purposes. The framework is capable of processing large data sets, and it supports vector machines to perform regression and classification.
Apache Mahout
Apache Mahout is a tool for mathematicians and data scientists who are looking for assistance with algorithm execution. This framework includes built-in algorithms to perform distributed linear algebra. It focuses on collaborative data grouping and classification.
KNIME
Konstanz Information Miner is an excellent software for data analysis, visualization, and modeling without the need to code. The framework is built on a modular data-pipeline concept that combines different components for machine learning and data mining as a single solution.
Weka
Weka is a collection of machine-learning algorithms that are primarily used for data mining. It can be used for a variety of functions, including regression, classification, and clustering. You can also prepare data, visualize it, or perform other tasks. It's an excellent tool for those who are just getting started in machine learning.
RapidMiner
RapidMiner provides a complete environment in which you can perform a variety of tasks, such as text mining, predictive analytics, machine learning, and deep learning. This solution is used widely for research, development, and education.
Machine Learning and Mobile App Development: What are the Benefits?
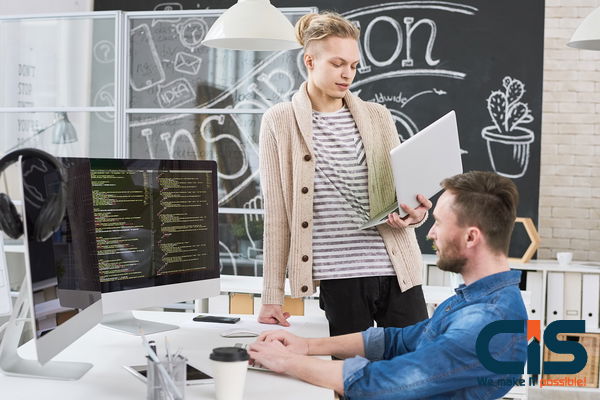
Personalization
Any machine-learning algorithm attached to your mobile app can analyze different sources of information, from social media activity to credit ratings, and provide recommendations to each user's device. Both mobile apps and machine learning web apps can be used for learning.
- Who are your clients?
- What are they interested in?
- What can they afford to spend?
- What are the words that they use to describe different products?
You can use this information to classify customer behavior and then target your marketing. Simply put, ML allows you to offer your customers and prospective customers more relevant and enticing content and give the impression that your mobile apps with AI have been customized for them.
Advanced Search
Machine learning app ideas let you optimize search options for your mobile applications. ML improves the user experience by making search results more contextual and intuitive. ML algorithms are able to learn from different queries made by customers and then prioritize results based on these queries.
Modern mobile applications can collect all user data, including search histories and typical behaviors. These data, along with search requests and behavioral data, can be used to rank products and services. Upgrades such as voice or gesture search can be implemented to improve the performance of an application.
Predicting User Behavior
The most significant advantage for marketers of machine learning app development is that they can get a better understanding of the preferences and behaviors of app users by inspecting different kinds of data regarding age, gender, location search history, app usage frequency, etc.
This data is critical to improving your marketing and application efforts. Amazon and Netflix's suggestions are based on the same principle; ML helps create customized recommendations for everyone.
More Relevant Advertisements
Many experts in the industry have argued that personalized experience for each customer is the best way to progress in this ever-expanding consumer market.
It can help you avoid debilitating customers by presenting them with services and products they don't want. Instead, You can focus all your efforts on creating ads tailored to each user's individual tastes and preferences.
Today, mobile app development companies can consolidate data using ML. This will save time and money spent on ineffective advertising and enhance the reputation of any brand.
Coca-Cola, for example, is known to customize its ads according to the demographic. This is done by analyzing what circumstances cause customers to mention the brand. It has then defined the best way of serving advertisements.
Increased Security Level
Machine learning can be used to streamline and secure mobile app authentication. Users can use features such as Image Recognition or Audio Recognition to store their biometric information as part of their security authentication on their mobile devices. ML can also help you establish access rights for customers.
Even malicious traffic and data can be prevented from reaching your device. Algorithms for Machine Learning applications detect and block suspicious activities.
How Do Developers Use Artificial Intelligence In Mobile Application Development?
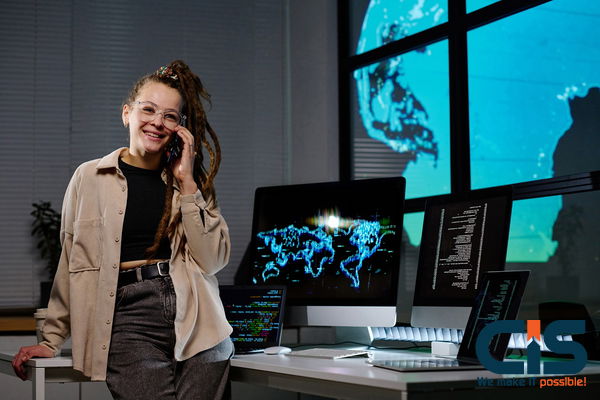
Let's take a closer look at the benefits of AI-powered mobile applications, which are endless for both users and mobile app developers. Artificial Intelligence is one of the best ways for developers to create realistic apps.
What are the best uses of:
- Mobile technology can incorporate machine learning as part of Artificial Intelligence.
- Predictive analysis is a method of analyzing large amounts of data to predict human behavior.
- Machine learning can be used to filter out unwanted data and improve security in mobile apps.
The machine learning technology allows an optical character recognition application (OCR) to recognize and remember characters that were skipped by the developer.
Machine learning is also applicable to Natural Language Processing apps. , which combines AI with Quality Assurance, reduces not only the time and effort required for development services but also the time spent on updating and testing.
What Are The Challenges And Solutions To Machine Learning?
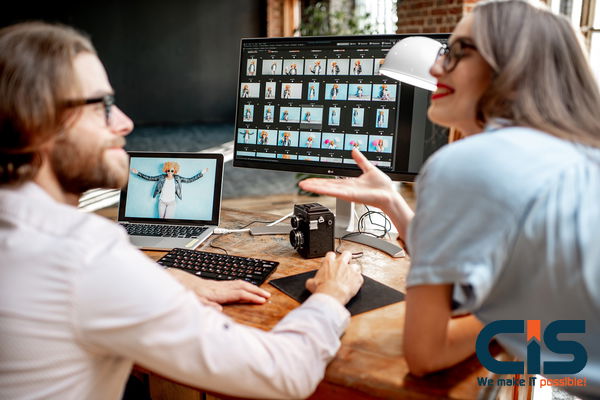
Machine learning is no different. It has its own set of challenges. Machine learning relies on the availability of sufficient data for training. As a benchmark for education, the training sample should be big enough to guarantee the fundamental perfection of machine learning algorithms.
Following are some of the methods that can be used to reduce the risk of misinterpretation by the mobile app or machine of any digital cues.
- Hard Sample Mining -When the sample size used for the analysis is not large enough, the machine should be able to confuse the objects. The device can learn to identify the main thing by comparing multiple objects.
- Data Enhancement -When an image is in question, in which a machine or mobile app is required to determine the central idea, the image should be modified while keeping the subject intact to enable the app to register a central object in various environments.
- Data Addition Impersonation -In a similar manner, specific data are nullified to only keep the information about significant objects. The machine memory will only contain information about the main thing and not the objects around it.
Want More Information About Our Services? Talk to Our Consultants!
Wrapping Up
Developing machine learning apps is a complex task requiring an in-depth understanding of the technology. It's best to hire an app development team who are experts in building machine learning apps if you need to know if you can do it yourself. Experts say that AI and ML will continue to grow in importance within the business world over the next few years. Artificial Intelligence and machine-learning-powered apps will become more mainstream in all industries as consumers and executives become more open-minded to cutting-edge technology. You must be ready to adopt these trends as an app developer and business owner to stay on top of the tech-driven market.