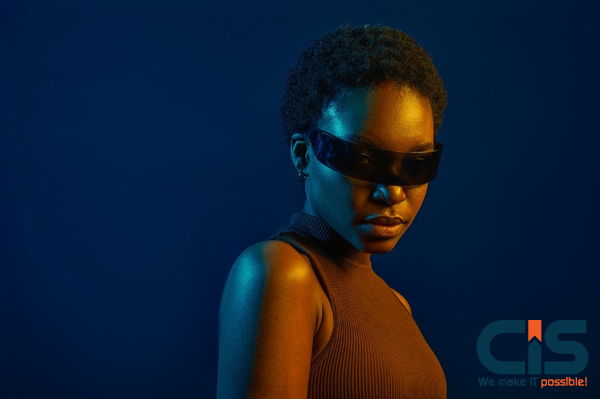
At an earlier time, not many years ago, the thought of consumers sharing details such as when they got you, what they had for breakfast, or even where they went on holiday would have seemed like a strange idea, to be honest. However, customer social norms have certainly changed, and expectations have increased.
The public is bombarded with ads for both products and services every day. The variety of choices is overwhelming. So what is it that makes people take a moment to pay attention?
When brands attempt to find out the answer as they try to answer it, they get more creative because of it. Indeed, many companies are embracing the advantages that big data can bring. For instance, in 2016, Starbucks introduced AI to email personalized offers to customers. Beyond adjusting drinks to suit your preferences, Starbucks uses its loyalty cards and application to collect and analyze customer data, including the place and time that purchases are made.
Big data isn't an entirely new concept. It's an idea that's been around for quite a while. However, the first prominent data analysts utilized spreadsheets written by hand and later manually analyzed the data. It's easy to imagine how long this process would take.
What Is Data Analytics?
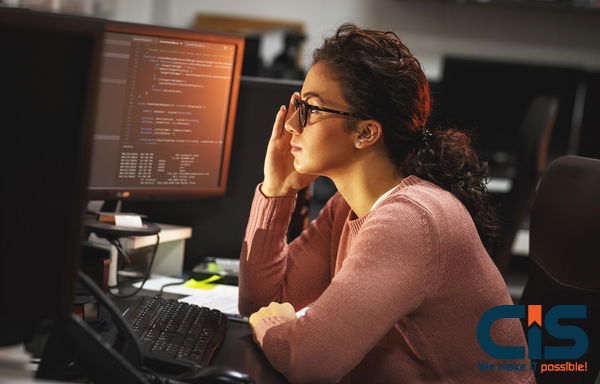
The term "data analytics" describes looking at data to conclude from the data they contain. Data analytics techniques allow users to analyze raw data and identify patterns that provide essential insights.
Nowadays, various techniques for data analysis use specialized systems and software that combine machine learning algorithms and automation capabilities, among others. Researchers and Analysts employ real-time data analytics methods to conduct their research. In addition, companies use data analytics to make informed decision-making.
Data visualization solutions can assist companies in gaining insight into their customers' needs, evaluating their advertising campaigns, personalizing content, developing content strategies, and creating products. As a result, businesses can use data analytics to boost their business and bottom performance.
For companies, the information they utilize could comprise historical data or any new data they collect for a particular project. They can also collect it from their clients and site visitors or buy it from different organizations. Information a company gathers about its customers is known as first-party data. Data obtained from an established company that has obtained it is referred to as second-party data. The aggregated data that a business purchases from a market is third-party data. The data that a company collects can include data about an audience's demographics, preferences, behavior, etc.
There are four main types of data analytics, and they are:
Descriptive Analytics: Describes the events that have transpired over a particular time. For instance, a sales increase or page views within a specific time, such as a quarter or month.
Predictive Analytics: Forecasts events that are likely to happen soon by analyzing data from the past.
Diagnostic Analytics: Analyze why a particular phenomenon (such as the increase or decrease in sales) occurs.
Prescriptive Analytics: Provides an idea for a particular method to take (e.g., an increase in the stock of a particular product) using data from the past.
Also, there is regression analysis. This term refers to various statistical techniques used to determine the relationships between dependent variables and one or more independent variables. This could give insight into the connections between variables and their future interactions.
Analyzing the previous data, we can make better business decision trees because we can anticipate consumer demand changes and understand why they happen. If used correctly will ultimately result in higher profits and a better bottom line.
But, the quality of data and efficient management of data matter significantly. Suppose your data are of low quality and reliable quality. In that case, it will be challenging to make the best business decision-making as you would. Therefore, data that is structured is essential.
Benefits of Data Analysis for Businesses in 2022
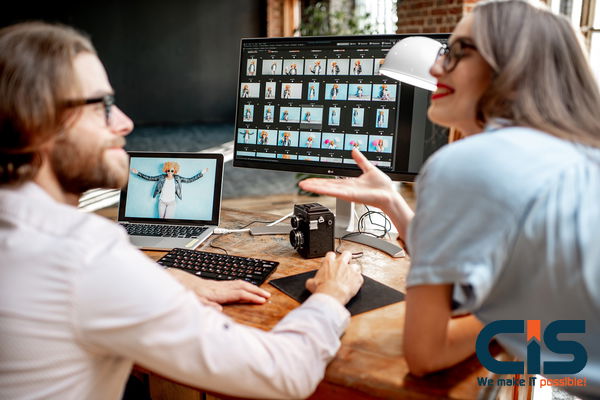
Once we've defined data analytics and described its various forms, we can consider the possible advantages it may bring to companies. There are many applications for data. Therefore, it's best to focus on a select few in this article.
Saves Time
Small business owners must wear multiple hats and manage their time between various duties and tasks. They need to be able to access the same resources as a more significant business goal, so they can't delegate responsibilities of a minor nature, at least not in the same way. Therefore, it is essential that the solutions and tools they use are effective and don't take up too much time.
Information is just as valuable insights as your skill in your interpretation. Small companies often contract out the task or employ consultants to handle the task. Machine learning, automation of processes, and mapping insights into business strategies are vital to boosting the efficiency of businesses.
Read More: Big Data Analytics Benefits - How To Analyse Big Data
Increase the Efficiency of the Work
Analytics allows you to analyze large quantities of data quickly and present the results in a structured manner to aid in achieving the organization's specific goals. In addition, it promotes a culture of cooperation and efficiency by allowing the management to communicate the analysis results with the employees. As a result, the weaknesses and areas for improvement within an organization become apparent, and action is taken to boost overall efficiency in the workplace, thereby increasing productivity.
Personalization of Products and Services
The days are gone when companies could offer customers the same items and services. Instead, customers want products and services that satisfy their specific needs.
Analytics can assist companies in keeping track of which product, service, or content is most liked by customers. They can then provide suggestions based on their preferences. For instance, when we look at Facebook, we generally view what we would like to see. All of this is possible thanks to the collection of data and analytics companies perform. In addition, data analytics may help provide specific services to customers depending on their needs.
Improved Customer Service
In the end, but not last least, data analytics can assist businesses in improving the quality of their customer service. First, it offers deep insights into customers' needs and what they prefer. Additionally, keeping data central and allowing the entire customer service staff to access it can lead to greater consistency in service quality.
Want More Information About Our Services? Talk to Our Consultants!
How can Businesses use Data Analytics?
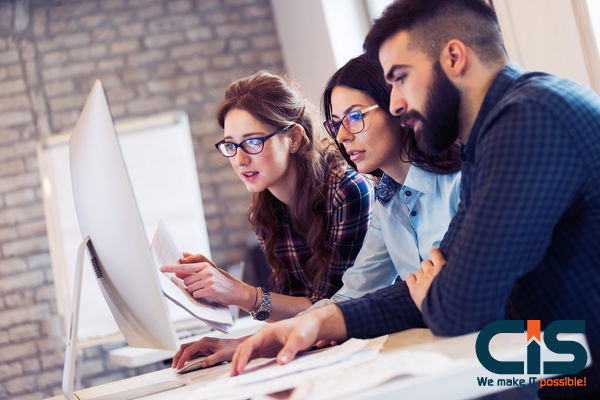
We've covered how data scientists--data engineers and scientists use analytical data. Yet, it's also worth taking a moment to consider how business processes can make the most practical use of data analytics. This is a daunting task because the amount of data required is significant. It is, therefore, crucial to think carefully about the applications that use data analysis.
As we've seen, finding accurate insights with no methods for data analysis is usually extremely difficult. This is a step-by-step guide to how companies can make use of data analytics and the most important questions to ask throughout the life cycle:
Planning and Strategy: Before doing anything other than data analytics, companies must ensure that they've got an overall strategy with clear objectives. They should ask themselves questions regarding their data requirements, for example, why they need to gather certain kinds of data (to gain insight into the customer's interactions, for instance) and what goals they want to accomplish.
Collecting Data: Once businesses know the need for data analysis services, they have to decide on the data sources they'll use, what particular data points they'll focus on, and how they'll collect the data. For example, some collect transaction and social media data. In contrast, others rely on high-tech sources, including GPS or RFID devices.
Ensuring Data is Relevant: We mentioned earlier that raw data could initially tell us nothing. Therefore, companies must ensure that the information they gather is high-quality and understandable. The simple act of rifling through massive amounts of data will not help and could be detrimental.
Making Effective use of Data: Businesses that use data analytics must carefully consider the best way to do this and provide the necessary resources needed for the job. For example, what metrics do you intend to implement? Certain companies employ internal data analysts who give them an edge over their competitors. However, hiring their own data experts will not likely work for smaller companies.
Presenting Data: Earlier, we discussed the importance of data visualizations to make findings more visible and easier to comprehend. Tools such as Tableau can assist businesses in visualizing data using graphs and charts. They can also be used as presentations. Examples include videos, webinars, and visually appealing infographics that are popular on sites such as LinkedIn.
Acting on New Insights: Getting all these insights from analytics on data is one thing. However, companies should have an action plan to put these insights into use. For example, how can these findings aid your company in improving its services to its customers? What are the best ways to use this information to gain new customers?
Top Data Analytics Tools to Use in 2022
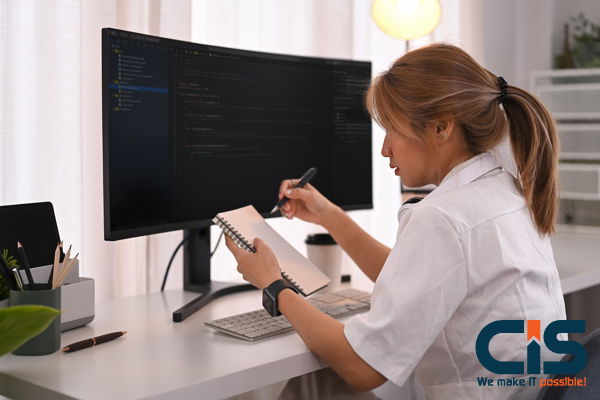
Numerous tools aid in this process of Data-Driven Decision-making. Selecting the appropriate tool can be difficult for data scientists and data analysts. The most common questions you may have in your mind include how many people use tools, how simple to use, and how it's placed in the marketplace. If you're an owner of a business, you may be concerned about the expense of such tools.
Here's a list of the top data analysis tools you can utilize for 2022 and beyond.
IBM
Big Blue offers a wide range of analytics solutions and tools. However, it is worth noting that Cognos Analytics with Watson is the leader in providing insights via data visualization. It utilizes its Watson AI and machine learning engine to combine data and give you comprehensive insight. In addition, the platform provides natural language processing, contextual forecasting, and predictive analytics. It also allows integration into social platforms.
MicroStrategy
The company describes its BI and Analytics platform as an opportunity to integrate "intelligence throughout." The platform connects over 200 data sources - including top platforms like Snowflake to real-time visualizations on PCs and Macs. In addition, it allows for location-based analysis. It offers self-service dashboards that can be used to perform sophisticated drill-down analyses.
Qlik
An established supplier within the BI and data analytics field, Qlik offers a moderately priced product, Qlik Sense, that provides robust functionality on-premises and in the cloud. It connects existing databases and information sources. It also offers self-service visualizations and reports that can be utilized across departments, groups, and functional areas. The platform is based on AI and ML to provide actionable intelligence.
SAS
An innovator in data analytics, SAS offers a sophisticated system that enables data-driven analytics. It has a range of programs that meet different needs. The visual analytics application is among the top available, with a sophisticated dashboard, a low-code framework, and AI/ML.
It is connected to various data sources, provides interactive data discovery, and supports augmented analytics, chat-enabled analytics, geo-location analytics, and more.
Apache Spark
Apache Spark is an open-source software framework that lets data analysts and scientists efficiently process massive datasets. Initially developed in 2012, it was transferred to the not-for-profit Apache Software Foundation. It was designed to analyze big unstructured datasets, and Spark distributes computationally heavy analysis tasks across various computers.
Spark is highly efficient in other similar frameworks (for instance, Apache Hadoop). Since it is based on RAM, not local memory, it's about 100 times more efficient than Hadoop. It's why it's frequently employed to develop large-scale machine learning models that require a lot of data.
Microsoft Power BI
In less than a decade, Power BI has been a newcomer in the world of tools for data analysis. Initially, it was an Excel plug-in but was developed in the early 2010s to become an entirely separate suite of business analysis tools. Power BI lets users build interactive reports and dashboards with a little learning curve.
Its primary selling point is its data connectivity, which works seamlessly with Excel (as one would expect from a Microsoft product); however, it also supports documents in text, SQL server, and cloud-based sources, such as Google and Facebook analytics.
Tableau at an Overview
Suppose you're looking to build interactive dashboards or visualizations without programming expertise. In that case, Tableau is one of the best commercial tools for available data analytics. It handles vast amounts of data more efficiently than other BI tools and is extremely easy to use. In addition, it features a visual drag-and-drop interface (another benefit over other tools for data analysis). However, as it needs a scripting layer, it cannot accomplish what Tableau can. In particular, there are better choices than Tableau for pre-processing data or creating more complicated calculations.
Want More Information About Our Services? Talk to Our Consultants!
The Key Takeaway
We've looked at the top well-known analysis tools currently available in this article. One of the most important things to learn is that no single tool can do everything. A skilled data analyst has a broad knowledge of various languages and programs.
If you've found one of these tools you didn't know about, why not investigate further? Try out the open-source tools (they're accessible in the end!) and learn about the others. Ultimately, it's helpful to understand which tools data analytics companies employ.